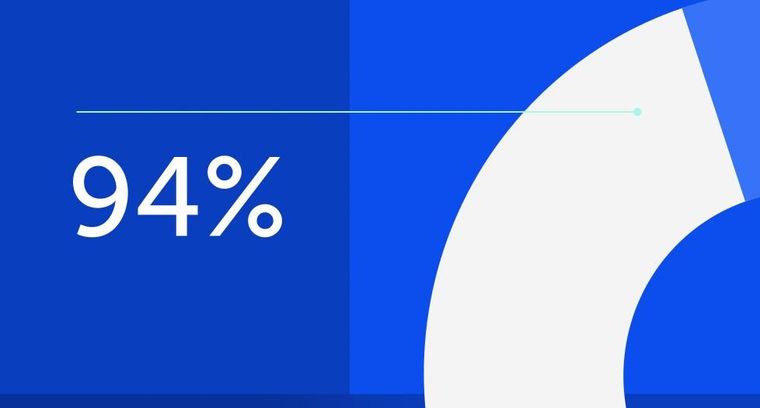
94% of researchers rate our articles as excellent or good
Learn more about the work of our research integrity team to safeguard the quality of each article we publish.
Find out more
REVIEW article
Front. Hum. Neurosci., 25 May 2016
Sec. Cognitive Neuroscience
Volume 10 - 2016 | https://doi.org/10.3389/fnhum.2016.00239
This article is part of the Research TopicBrain Oscillations in Human CommunicationView all 16 articles
Effective interpersonal communication depends on the ability to perceive and interpret nonverbal emotional expressions from multiple sensory modalities. Current theoretical models propose that visual and auditory emotion perception involves a network of brain regions including the primary sensory cortices, the superior temporal sulcus (STS), and orbitofrontal cortex (OFC). However, relatively little is known about how the dynamic interplay between these regions gives rise to the perception of emotions. In recent years, there has been increasing recognition of the importance of neural oscillations in mediating neural communication within and between functional neural networks. Here we review studies investigating changes in oscillatory activity during the perception of visual, auditory, and audiovisual emotional expressions, and aim to characterize the functional role of neural oscillations in nonverbal emotion perception. Findings from the reviewed literature suggest that theta band oscillations most consistently differentiate between emotional and neutral expressions. While early theta synchronization appears to reflect the initial encoding of emotionally salient sensory information, later fronto-central theta synchronization may reflect the further integration of sensory information with internal representations. Additionally, gamma synchronization reflects facilitated sensory binding of emotional expressions within regions such as the OFC, STS, and, potentially, the amygdala. However, the evidence is more ambiguous when it comes to the role of oscillations within the alpha and beta frequencies, which vary as a function of modality (or modalities), presence or absence of predictive information, and attentional or task demands. Thus, the synchronization of neural oscillations within specific frequency bands mediates the rapid detection, integration, and evaluation of emotional expressions. Moreover, the functional coupling of oscillatory activity across multiples frequency bands supports a predictive coding model of multisensory emotion perception in which emotional facial and body expressions facilitate the processing of emotional vocalizations.
Effective communication is crucial for the formation and maintenance of social relationships in complex societies. Emotional communication is a complex process where the expression and perception of emotional signals exchanges information about internal affective states. While some of these signals can be expressed through verbal means, much of our emotional communication occurs nonverbally through changes in facial, body, and vocal expressions. Therefore, our ability to perceive and interpret nonverbal expressions of emotion can have a profound impact on the quality of our social interactions, affecting our mental health and wellbeing. To this end, deficits in emotion perception have been observed in a number of neurological and psychiatric conditions (Phillips et al., 2003; Garrido-Vásquez et al., 2011) and may negatively correlate with subjective quality of life in a number of these conditions (i.e., Phillips et al., 2010, 2011; Fulford et al., 2014). Despite this importance, the neural mechanisms and dynamics underpinning the perception of emotional cues within and between sensory modalities is poorly understood. This review explores the functional role of neural oscillations in mediating neural communication within and between sensory modalities in order to facilitate the detection, integration, and evaluation of emotional expressions.
Emotions are commonly defined as brief, coordinated neural, physiological, and behavioral responses to relevant events (Scherer, 2000). These responses can manifest behaviorally as changes in facial expression, body language, tone of voice (prosody), or any combination thereof. Thus, emotion perception can be described as the process of detecting salient signals, integrating those signals with prior knowledge of emotional meaning, and evaluating the integrated representation within the context of the current environment. According to current models, emotion perception of visual (Adolphs, 2002a; De Gelder, 2006), auditory (Schirmer and Kotz, 2006; Wildgruber et al., 2009; Kotz and Paulmann, 2011), and audiovisual (Brück et al., 2011) signals unfolds in three fast yet distinct stages: detection, integration, and evaluation.
The first stage consists of early perceptual processing in what are traditionally considered modality-specific cortices. For visual expressions of emotion, this includes regions of the occipito-temporal cortex, most notably the fusiform gyrus (Adolphs, 2002a,b; Vuilleumier and Pourtois, 2007) with distinct subregions of the fusiform gyrus responding preferentially to facial and body expressions (Schwarzlose et al., 2005), and extriate body area (Grèzes et al., 2007; Kret et al., 2011; Meeren et al., 2013). Early detection of complex acoustic cues occurs in the belt region of the primary auditory cortex (Woods and Alain, 2009) and later in multiple voice-sensitive areas in the temporal lobe (Belin et al., 2004; Wiethoff et al., 2008; Ethofer et al., 2009, 2011; Pernet et al., 2015).
Following the extraction of low-level visual and acoustic features, a more detailed representation of the emotional expression is generated in the superior temporal sulcus (STS). Evidence from neuroimaging research suggests a functional subdivision within the STS with face-sensitive regions in the posterior terminal ascending branch and voice-sensitive regions in the trunk section (Kreifelts et al., 2009). Functional differentiation between middle and anterior regions of the STS has also been noted during the perception of emotional vocal expressions (Kotz and Paulmann, 2011). Receiving input from both visual and auditory cortices, the STS also plays a key role in audiovisual integration (i.e., Calvert et al., 2000, 2001; Beauchamp et al., 2004a,b; Stevenson et al., 2007; Stevenson and James, 2009). To this end, facial and vocal expressions activate overlapping face- and voice-sensitive regions within the STS, suggesting that the STS is essential for the integration of audiovisual emotional information (Robins et al., 2009; Watson et al., 2013, 2014).
In the final stage, the behavioral and motivational significance of the expression is interpreted and evaluated within the inferior frontal gyrus (IFG; Frühholz and Grandjean, 2013a) and orbitofrontal cortex (OFC; Adolphs, 2002b; Kotz et al., 2012). Involved in the processing of reward and punishment (Kringelbach and Rolls, 2004; Rolls, 2004), the OFC is thought to be involved in the representation of stimulus value across sensory modalities. Thus, during emotion perception, the OFC may be responsible for evaluating the emotional value of the expression within the context of the current environment.
In addition to these cortical regions, many studies also support a key role for subcortical structures such as the amygdala and basal ganglia in the perception of emotions. For example, the amygdala has been implicated in the processing of facial (i.e., Phillips et al., 1997; Blair et al., 1999; Whalen et al., 2001, 2013; Williams et al., 2004), body (Hadjikhani and de Gelder, 2003; Grèzes et al., 2007), and vocal (Fecteau et al., 2007; Frühholz and Grandjean, 2013b) expressions in both early and late stages of emotion perception. Studies also support a key role for the basal ganglia in the processing of facial (Adolphs, 2002b) and vocal (Kotz et al., 2003) expressions. Furthermore, deep brain stimulation of the basal ganglia, specifically the subthalamic nucleus, can impair emotion perception from facial and vocal expressions (Péron et al., 2010a,b). Given the importance of the basal ganglia in other aspects of emotion processing (i.e., subjective feeling and production of emotional expressions), it has been proposed that the basal ganglia coordinates the synchronization of different components of emotion processing (Péron et al., 2013). While the amygdala appears to be involved in both the early and late stages of emotion perception, consistent with dual-pathway models of emotion processing (LeDoux, 1996), basal ganglia activity is more often observed in the later stages as a function of attention (Kotz et al., 2012).
In sum, the perception of emotion from facial, body and vocal expressions involves a distributed neural network of cortical and subcortical structures. The question then becomes, how does the brain selectively attend to and integrate these signals across space and time in order to give rise to a unified representation of an emotional expression?
The investigation of such rapid online processing of dynamic changes in sensory input requires adequate methods to capture neural information processing in real time. Electroencephalography (EEG) and magnetoencephalography (MEG) are particularly well suited for the study of emotion perception due to their millisecond temporal resolution.
Results from event-related potential (ERPs) suggest differentiation between emotional and neutral facial expressions within 120 ms of stimulus onset (Eimer and Holmes, 2002; Eimer et al., 2003). The time course of emotion-related effects on evoked responses to vocal expressions depends on the stimulus type with earlier effects for affective bursts such as laughs and screams (Liu et al., 2012) compared to changes in emotion prosody (Paulmann and Kotz, 2008; Paulmann et al., 2012, 2013; Pell et al., 2015). These early ERP effects are thought to reflect rapid detection of salience. Visual (Stefanics et al., 2012) and auditory (Schirmer et al., 2005) deviance detection, in the form of the mismatch negativity (MMN) is observed at approximately 200 ms, supporting the idea that integration of emotional signals occurs at this stage. Across domains, emotional expressions also elicit a sustained positivity (the late positive component or LPC) beginning between 300–400 ms post-stimulus, reflecting the interpretation and evaluation of emotional significance (visual, Eimer and Holmes, 2007; auditory, Paulmann et al., 2013).
Taken together, findings from ERP studies largely support the staged models of visual and auditory emotion perception and further establish a time course for the stages of detection, integration, and evaluation of emotional expressions. While studies of ERPs have undoubtedly advanced our understanding of the time course and neural bases of emotion perception, they can only provide limited insight into the dynamic interaction within and between nodes of functional neural networks. That is, we know substantially more about when and where certain processes may occur, than about how these processes arise and unfold within the human brain.
Neural oscillations, which reflect rhythmic fluctuations in the synchronization of neuronal populations, provide a measure of the dynamic interactions within and between regions involved in the different stages of emotion perception. Changes in oscillatory activity are commonly analyzed by treating the on-going EEG (or MEG) signal as the sum of pure sinusoids, which are separated into characteristic frequency bands each associated with distinct cognitive and computational operations. Decomposing the EEG/MEG signal into its constituent sinusoids, allows for the measurement of changes in power (amplitude) and phase within and between each frequency band, at different time points and in different brain regions. While increases or decreases in power—referred to as event-related synchronization (ERS) or desynchronization (ERD) respectively—indicate changes in neural synchronization within a specific node or region, phase coherence across brain regions reflects synchrony between brain regions that make up a functional neural network (Bastiaansen et al., 2012). According to one hypothesis, phase coherence enables the effective communication between neuronal populations (Fries, 2005). Moreover, cross-frequency coupling may facilitate the integration of information across different spatial and temporal scales (Canolty and Knight, 2010). Thus, neural oscillations can provide an index of the dynamic interaction between brain regions involved in emotion perception as well as a plausible mechanism by which the brain can integrate rapidly changing emotional information from facial, body, and vocal expressions. To date, the majority of studies investigating emotion perception have focused solely on power changes. Thus, this review will primarily focus on ERS and ERD, while noting the critical importance of phase coherence and cross-frequency coupling in elucidating the functional dynamics of emotion perception within and between sensory modalities.
Facial expressions are by far the most commonly studied means of emotional communication. In a typical study, participants are presented with images of facial expressions and asked to respond to the emotion (explicit) or identity/gender (implicit) of the face. Using this type of paradigm, studies have found changes in oscillatory activity across multiple frequency bands during the perception of emotion from facial expressions.
Delta oscillations have been implicated in a wide range of processes including the perception of faces and facial expressions (Knyazev, 2012; Güntekin and Başar, 2015). While frontal delta synchronization is characteristic of many more “cognitive” tasks, face processing is associated with delta synchronization over more posterior regions (Güntekin and Başar, 2009). Moreover, emotional expressions appear to induce stronger delta synchronization than neutral expressions over occipito-parietal regions, which is suggested to reflect stimulus updating (Balconi and Lucchiari, 2006; Balconi and Pozzoli, 2007, 2008, 2009). Effects of emotion on delta oscillations have also been observed over fronto-central regions, correlating with behavioral measures emotional involvement (Knyazev et al., 2009b). Of note here is that the studies observing occipito-parietal delta synchronization have typically used passive viewing paradigms while Knyazev et al. (2009a) used both implicit (gender identification) and explicit (emotion categorization) tasks. Thus, emotion may differentially affect delta responses to facial expressions depending on task demands. Together these findings suggest a role for delta oscillations in the perception of emotional facial expressions; yet the functional significance of delta synchronization in this context remains unclear. Further research is needed in order to determine more precisely the functional role of delta oscillations within the context of emotion perception.
Most commonly associated with memory encoding and retrieval (Klimesch, 1999), theta band oscillations are thought to play a key role in the processing of emotion (Knyazev, 2007). To this end, recent studies have shown enhanced theta synchronization for emotional compared to neutral facial expressions, suggesting that theta oscillations may facilitate the rapid encoding of emotionally salient sensory information. For instance, Balconi and colleagues have observed enhanced theta synchronization over predominantly right frontal regions of the scalp between 150–250 ms extending into the later time window of 250–350 ms which they suggest reflects the orienting of attention toward the emotional significance of the stimulus during the early stages of conceptual processing (Balconi and Lucchiari, 2006; Balconi and Pozzoli, 2009). Similar results were reported by Knyazev et al. (2009b, 2010), who found increased early theta ERS over right frontal regions during the implicit processing of emotional facial expressions, that is, when participants performed a gender categorization task in which attention was directed away from the emotional content of the stimulus. Furthermore, these authors observed a second distinct theta ERS between 230–350 ms that was greater when the emotional content of the stimulus was processed explicitly during an emotion categorization task. Source localization revealed differential activation in the right parietal cortex (angry) and insula (happy) in the early time window and left temporal lobe (angry) and bilateral PFC (happy) in the later time window. Interestingly, some studies have also observed theta synchronization over more posterior (occipital and occipito-parietal) regions within a similar (early) time window (i.e., Başar et al., 2006; Balconi and Pozzoli, 2007, 2008), an effect that increases as a function of visual awareness (Zhang et al., 2012). However, the extent to which this theta synchronization is emotion-specific may be called into question on the basis of a study by González-Roldan et al. (2011) showing no effect of emotion on theta synchronization during an explicit task. Instead, the authors observed a main effect of intensity on theta ERS between 200–400 ms over frontal, central, and parietal regions. This may suggest that theta synchronization in response to emotional facial expressions may reflect facilitated encoding of the biological or motivational significance rather than the emotional quality of the expression per se. That is, emotional expressions (relative to neutral expressions) contain more behaviorally relevant sensory information, which reduces uncertainty, resulting in stronger neural synchronization in the theta frequency. This enhanced theta synchronization facilitates the dynamic between brain regions involved in the early detection and integration of static emotional facial expressions.
As first noted by Berger (1929), neural oscillations in the alpha frequency band show strong synchronization over occipital regions in the absence of visual stimulation (i.e., with eyes closed). Based on further evidence showing alpha ERS over cortical regions not necessary for a given task, alpha synchronization was initially taken as an indicator of cortical idling (Pfurtscheller et al., 1996). However, more recent hypotheses suggest that alpha synchronization serves an active role in the inhibition of task-irrelevant brain regions (Klimesch et al., 2007; Jensen and Mazaheri, 2010). The rhythmic fluctuation of alpha oscillations thus produces temporal windows in which neurons are more or less likely to fire. Larger amplitudes (reflecting stronger inhibition) result in smaller temporal windows and thus more precise timing of neuronal firing. Smaller amplitudes, associated with release of inhibition, result in greater cortical excitability over longer temporal intervals. Within the context of emotion perception, alpha oscillations may be involved in the selective attention to emotionally salient social cues through active inhibition of task-irrelevant regions and pathways. It is notable, however, that many studies using static faces have found no difference in alpha synchronization between emotional and neutral expressions (Balconi and Lucchiari, 2006; Balconi and Pozzoli, 2007, 2008, 2009). Differences in alpha power emerge more reliably when comparing expressions of positive and negative valence. While perception of negative emotional expressions was associated with right-lateralized alpha ERD, perception of positive emotional expressions was associated with left-lateralized alpha ERD (Balconi and Ferrari, 2012). Although greater when facial expressions were presented supraliminally, these valence-specific differences were also observed when expressions were presented subliminally. Further support for these findings comes from a study by Del Zotto et al. (2013) showing valence-specific lateralization of frontal alpha power in a patient with cortical blindness. Results from this study showed that alpha ERD was greatest for fear compared to happy expressions over right frontal regions even though the patient could not report seeing the stimuli. Other evidence suggests that alpha synchronization over posterior regions may also differentiate between stimuli of negative and positive valence (Başar et al., 2006), though this effect was only observed when selecting the stimuli with the most extreme valence ratings for analysis.
While studies using static stimuli highlight the roles of valence in alpha responses to facial expressions, they have two important limitations. Firstly, in naturalistic human communication, facial expressions are inherently dynamic and therefore the extent to which these findings would be valid in naturalistic settings is unclear. Secondly, although providing a rough estimate as to the topographical distribution of alpha ERD, these studies only provide limited insight into the patterns of functional connectivity underpinning the perception of emotion from facial expressions. Addressing these issues, a recent MEG study used dynamic facial expressions to explore changes in spatial connectivity during emotion perception (Popov et al., 2013). Findings from this study provide evidence for two stages of upper alpha desynchronization during facial emotion perception: a pre-recognition stage associated with increased alpha power over frontal and sensorimotor regions and decreased alpha power over occipital regions followed by a post-recognition stage associated with the reversed pattern. Moreover, these power changes were associated with inverse patterns of functional connectivity, suggesting that alpha synchronization and desynchronization may regulate the exchange of information between visual and sensorimotor. That these effects were stronger in response to emotional compared to neutral expressions implies that emotion may enhance the functional coupling, facilitating recognition of facial expressions of emotion.
Oscillatory activity in the beta frequency is typically associated with sensorimotor processing (Brovelli et al., 2004). However, recent evidence suggests a broader role for beta synchronization in the maintenance of current sensory, motor, and cognitive sets (Engel and Fries, 2010). Beta band oscillations have also been implicated in the perception of emotion from facial expressions. However, the direction, time course, and topography of beta modulation vary considerably between studies. For example, Güntekin and Başar (2007a) found increased beta power for angry compared to happy expressions over frontal and central regions. In a similar study including occipital electrodes, however, the authors found no main effect of emotion on beta band activity (Güntekin and Başar, 2007b). Thus, it seems that only fronto-central beta synchronization reflects differentiation between emotional and neutral facial expressions. Other evidence suggests that such differences in beta synchronization may also be modulated by attention. To this end, asymmetry in resting-state parietal beta band activity has been negatively correlated with attentional bias towards angry facial expressions (Schutter et al., 2001).
Given the importance of beta oscillations in the perception of biological motion, which is thought to be critical for social cognition in naturalistic environments (Pavlova, 2012). Jabbi et al. (2015) used MEG to compare evoked beta band activity in response to dynamic and static facial expressions. Perhaps unsurprisingly, greater beta power was observed for dynamic compared to static facial expressions in occipital, superior temporal and sensorimotor cortices. When comparing dynamic emotional to neutral expressions, the authors found stronger beta power in regions such as the amygdala, STS, and OFC. Furthermore, beta power in the left STS was negatively correlated with the time course of fearful facial expressions but positively correlated with the time course of happy facial expressions. These emotion-specific differences suggest that the observed changes in beta power were not solely due to the processing of biological motion. Although this study investigates evoked rather than induced oscillatory activity, its findings support a putative role for beta oscillations—particularly within the STS—in tracking the temporal dynamics of facial expressions of emotion.
Reflecting neuronal communication on a more local scale, gamma oscillations have been implicated in a number of cognitive processes including feature integration (Singer and Gray, 1995) and sensory selection (Fries et al., 2002). Within the context of emotion perception, event-related gamma synchronization has been commonly used to explore the functional dynamics underpinning the conscious and unconscious processing of emotional facial expressions. Studies investigating the spatial and temporal dynamics of emotion perception support a dual-pathway model of emotion perception consisting of a cortical and subcortical pathway (i.e., LeDoux, 1996). Accordingly, in an MEG study, Luo et al. (2007, 2009) have reported that fearful expressions elicit early gamma band activity in the amygdala followed by later responses in the occipital, parietal, and prefrontal cortices. These authors have also observed a later attention-dependent gamma response localized to the amygdala, presumably due to feedback from prefrontal regions (Luo et al., 2010). However, these studies, as with any EEG or MEG study reporting activation from deep, subcortical structures, should be considered respect to the current limitations in source analysis techniques. Although greater for supraliminally-presented facial expressions, gamma synchronization is also observed in response to facial expressions processed subliminally (Balconi and Lucchiari, 2008; Luo et al., 2009), suggesting that gamma synchronization can be influenced by emotion even in the absence of visual awareness.
These findings are supported by intracranial studies showing localized gamma synchronization in brain regions implicated in emotion processing—most notably, the amygdala and OFC. Recording intracranial field potentials from the amygdala of pre-surgical epileptic patients, Sato et al. (2011) found increased gamma synchronization in the amygdala for fearful compared to neutral facial expressions. The early time course of gamma synchronization (50–150 ms) supports the presence of a subcortical pathway involved in the rapid detection of emotionally salient facial features. Gamma synchronization has also been observed over prefrontal cortices during the later stage of emotional face perception. Consistent with findings from functional neuroimaging studies demonstrating functional subdivisions between medial and lateral regions of the OFC (i.e., Kringelbach and Rolls, 2004), gamma responses in the lateral OFC are greater in response to negative emotions (Jung et al., 2011). However, this effect only occurred when attention was explicitly directed to the emotional quality of the expressions. Thus, during an implicit processing task, no responses in the lateral OFC were observed. Moreover, Jung et al. (2011) observed increased gamma band activity in the medial OFC only in response to target stimuli, regardless of emotional valence. These finding suggest that the medial-lateral distinction between sub-regions of the OFC cannot be explained simply in terms of valence but may instead reflect the processing of relative value within the context of the current environment. Recent studies have also observed differential effects of attention on gamma band activity in a network of brain regions the amygdala and OFC during the perception of emotional facial expressions (Müsch et al., 2014). Thus, gamma synchronization in the OFC may reflect the attention-dependent binding of emotionally salient stimuli with internal representations of their motivational significance.
Taken together, the current evidence supports the idea that the perception of emotional facial expressions is mediated by the synchronization of neural oscillations across multiple frequency bands (Güntekin and Başar, 2014). Overall, it appears that lower frequency bands may coordinate patterns of long-range connectivity necessary for the encoding and selection of emotionally salient facial features while higher frequency bands may be associated with the integration of these features at multiple stages of emotion processing.
Within the auditory domain, emotion can be communicated via affective bursts (laughs, screams, cries, etc.) or more subtle changes in tone of voice, or emotion prosody. While both convey important affective information, perception of emotion from these two types of vocal expressions occurs along different time scales and may rely on different patterns of neural activity and connectivity. Although very few studies have investigated the role of neural oscillations in perception of emotion from either type of vocal expression, current evidence suggests that theta synchronization may play a particularly important role in facilitating the detection of emotionally salient vocal cues.
A considerable body of research suggests that theta band oscillations drive the processing of slow acoustic changes in speech perception (Peelle and Davis, 2012). To explore the role of oscillatory activity in the detection of emotional prosodic change, Chen et al. (2012) used a cross-splicing procedure to artificially combine vocalizations spoken in angry and neutral prosodies. Thus, vocalizations could change from neutral to angry, angry to neutral, or remain constant. In this paradigm, detection of prosodic change was associated with an increase in fronto-central theta synchronization between 100–600 ms. Furthermore, for angry prosodies only, theta synchronization was modulated by intensity with greater power for high compared to low intensity vocalizations. Subsequent research by the same group has extended these findings, showing increased theta synchronization for neutral to angry change compared to no change for both implicit and explicit tasks suggesting that the emotional content of the stimulus may facilitate the detection of acoustic change (Chen et al., 2014). In this study, significant beta desynchronization was also observed between 400–750 ms, but only when the task required explicit processing of emotional change, which the authors interpret as re-integration of the cross-spliced portion of the sentence with its preceding context. Although these findings provide preliminary support for the role of theta synchronization and beta desynchronization in the detection of emotion prosody, the precise temporal and spatial dynamics of these effects needs to be addressed in order to provide a better characterization of the function of these frequency bands in vocal emotion perception.
With regards to affective bursts, what little evidence there is suggests that gender differences may also influence theta band activity. In a study by Bekkedal et al. (2011), the authors found no main effect of emotion on frontal theta synchronization. Instead, they found an interaction between emotion and gender such that women showed increased theta synchronization for angry expressions over bilateral anterior regions while men showed increased theta synchronization for expressions of pleasure over right anterior regions. As noted by the authors, this gender difference in theta synchronization may be due to differences in arousal, although behavioral measures would certainly be needed to support this claim. Moreover, the wide time intervals used for analysis (500 ms) make the functional interpretation of these gender differences in theta synchronization difficult and may partially account for the absence of any statistically significant differences in other frequency bands.
Though few in number, the existing studies suggest that theta synchronization may facilitate the perception of emotion from vocal expressions. Consistent with findings from the speech literature, theta synchronization appears to mediate the detection of acoustic change, an effect which is modulated by emotion. Additionally, beta desynchronization may also play a role in vocal emotion perception, but only when explicitly attending to the change in prosody. Thus, theta synchronization may be involved in the detection of emotionally significant acoustic features during vocal emotion perception while beta desynchronization may facilitate the integration of these features with contextual information.
In natural environments, emotion perception requires the integration of emotional cues from both visual and auditory modalities. Based on current models of visual and auditory emotion perception, it could be hypothesized that multisensory emotional expressions are integrated in a convergent manner such that visual and auditory cues are processed separately in modality-specific cortices, integrated into a coherent multisensory percept the STS, and evaluated in the PFC. However, it is important to note that facial and vocal expressions occur along different temporal scales with changes in facial expression often preceding changes in vocal expressions. Therefore, based on dynamic changes in facial and body expressions, the brain can generate predictions about the timing and content of forthcoming vocal expressions. Evidence from ERP studies suggests that emotional facial expressions elicit stronger (i.e., more reliable) predictions than neutral expressions (Jessen and Kotz, 2011; Jessen et al., 2012; Ho et al., 2015; Kokinous et al., 2015), resulting in facilitated processing of predicted emotional vocalizations. Together with recent proposals suggesting that neural oscillations play an important role in multisensory processing (Schroeder et al., 2008; Senkowski et al., 2008; Arnal and Giraud, 2012), this suggests that neural synchronization may facilitate the processing of multisensory emotional expressions through: (i) the selective binding of emotionally-salient sensory input from different modalities; and (ii) the formation and modification of sensory predictions.
Many earlier studies of multisensory emotion perception relied on the use of static facial expressions paired with words or phrases spoken in emotional or neutral prosody. In one such study, Chen et al. (2010) sought to determine whether multisensory integration effects could be observed in the primary sensory cortices during emotional face-voice processing. Using MEG, the authors recorded changes in oscillatory activity during visual, auditory, and audiovisual processing of emotional expressions. However, no integration effects were observed in either visual or auditory cortices. While this finding is interpreted as absence of audiovisual integration in primary sensory cortices, it could also be explained by the absence of predictive visual information since visual and auditory cues were presented simultaneously (see Vroomen and Stekelenburg, 2010). Interestingly, however, the authors observed alpha synchronization over superior frontal and cingulate cortices, which may suggest that increasing the amount of information available to the sensory systems via multiple modalities reduces the cognitive demand on prefrontal regions (Schelenz et al., 2013). Other studies using static facial expressions have found cross-modal interactions in other frequency bands and brain regions. For instance, by presenting participants with static fearful and neutral facial expressions paired with congruent vocal expressions, Hagan et al. (2009) demonstrated supra-additive increases in oscillatory activity in the STS, with theta and gamma bands contributing most to the increase in broadband activity. Subsequent research by the same group showed that supra-additive increases in the STS occurred in both congruent and incongruent conditions (albeit later in the incongruent condition), suggesting automatic integration of emotional facial and vocal expressions (Hagan et al., 2013). Consistent with these findings, other studies have observed theta synchronization during the integration of facial and prosodic change (Chen et al., 2015). Together, these findings suggest that oscillatory activity in the alpha and theta frequency bands drive the integration of facial and vocal expressions. Thus, without predictive visual information, theta synchronization in the STS may facilitate the feedforward integration of visual and auditory input into a coherent percept, reducing the processing demands on prefrontal regions involved in the interpretation and evaluation of the expression.
Although these studies using static facial expressions have undoubtedly contributed to our understanding of audiovisual integration of emotional expression, their findings could be challenged on the grounds of ecological validity. Therefore, more recent studies have moved towards the use of dynamic facial, body, and vocal expression in order to explore the oscillatory correlates of emotion perception in more naturalistic environments. In among the first to do so, Jessen and Kotz (2011) presented participants with video clips of dynamic facial, body, and vocal expressions. Using EEG, the authors found significant decreases in both alpha and beta power for audiovisual compared to the sum of auditory- and visual-only conditions with additional suppression for emotional compared to neutral expressions. These findings were replicated in a subsequent study, which also showed that while beta suppression for the contrast between multimodal and unimodal conditions was localized to the premotor cortex, suppression for the contrast between emotional and neutral conditions was localized to the posterior parietal cortex (Jessen et al., 2012). Since previous studies have demonstrated beta suppression in these regions during the processing of biological motion (Muthukumaraswamy et al., 2006; Muthukumaraswamy and Singh, 2008), it could be argued that the observed differences in beta power are due to differences in the motion content between emotional and neutral expressions. However, for the stimuli used in these studies, there was no difference in the motion content before the onset of the vocal expression (see Jessen and Kotz, 2011) making it unlikely that beta suppression was an artifact of differences in motion content. Instead, beta oscillations may play a broader role in the predictive coding of audiovisual information (i.e., Arnal and Giraud, 2012). Furthermore, the observed differences in beta ERD between emotional and neutral expressions provide support for the hypothesis that emotional expressions generate stronger cross-modal predictions compared to neutral expressions (Jessen and Kotz, 2013).
Taken together, these studies support previous research suggesting that neural oscillations play an important role in multisensory processing. Furthermore, these findings show that the emotional content of the stimulus may facilitate flexible integration of facial, body, and vocal expressions. The simultaneous presentation of visual and auditory expressions results in synchronization of theta oscillations in the STS (i.e., the STS) and alpha oscillations over prefrontal regions, suggesting that theta synchronization mediates the integration of audiovisual emotional expressions. Previous evidence suggests that multimodal expressions generally are more easily recognizable than unimodal expressions (Collignon et al., 2008; Tanaka et al., 2010; Föcker et al., 2011), frontal alpha synchronization may reflect relative inhibition of regions needed to resolve any remaining uncertainty with regards to the emotional content of the stimulus. Since this effect was observed in emotion categorization tasks, it is possible that different task demands will induce different spatial and temporal patterns of alpha synchronization. In contrast, the natural temporal delay between visual and auditory expressions enables the brain use changes in facial and body expression to generate predictions about the timing and content of forthcoming vocal expressions. Thus, cross-modal prediction results in ERD, particularly in the alpha and beta frequencies. These findings support the idea that multisensory integration and cross-modal prediction are distinct yet interactive mechanisms underpinning the multisensory emotion perception (Jessen and Kotz, 2013, 2015).
Nonverbal emotion perception is driven by dynamic, context-dependent interactions within and between brain regions involved in the detection, integration, and evaluation of emotional expressions. Where and when such interactions occur depends on the sensory modality (or modalities) through which the emotion is expressed as well as the emotional quality of the stimulus itself. However, emotional expressions are dynamic events that continuously evolve over time. Therefore, the neural system(s) supporting emotion perception must be able to flexibly adapt to and integrate rapidly changing sensory input from multiple modalities. Based on the reviewed evidence, we propose that neural synchronization underpins the selective attention to and the flexible binding of emotionally salient sensory input across different spatial and temporal scales. Furthermore, neural oscillations provide a mechanism through which emotional facial and body expressions can predictively modulate the processing of subsequent vocal expressions.
The recognition of an expression as “emotional” requires the selective binding of emotionally relevant sensory information. However, individual features of an emotional expression can occur at different points in time and are processed in spatially distinct regions of the brain. Thus, the brain is challenged with the task of binding only those features belonging to the same event across both space and time. One mechanism through which this may occur is the synchronization of neural oscillations, which creates temporal windows in which information belonging to the same event can be selected and integrated (Singer and Gray, 1995). Moreover, coherence between distinct neuronal populations may enable the flexible neuronal communication across different regions of the brain (Fries, 2005). Consistent with this idea, current evidence suggests that the synchronization of neural oscillations supports the selection and integration of sensory information within and between modalities (Senkowski et al., 2008; van Atteveldt et al., 2014). Gamma band oscillations, in particular, are thought to be important for sensory binding and feature integration on a local scale (Tallon-Baudry and Bertrand, 1999). As previously discussed, perception of emotion from facial expressions results in increased gamma band synchronization, suggesting that gamma band oscillations may mediate the rapid integration of emotionally salient sensory input. However, gamma band synchronization may be modulated by lower-frequency oscillations. Since lower frequency bands represent the activity of larger neuronal populations and longer temporal windows, such cross-frequency coupling between low and high frequency oscillations may enable the integration of information across different spatial and temporal scales (Canolty and Knight, 2010).
Natural communicative signals exhibit strong regularities that enable the brain to generate predictions about forthcoming sensory information within and between sensory modalities. This process may be mediated by the functional coupling of neural oscillations, which can facilitate the efficient allocation of processing resources to the predicted sensory input. For instance, synchronization of low-frequency oscillations may coordinate the allocation of processing resources, via high-frequency oscillations, at the phase in which the predicted sensory input occurs (Hyafil et al., 2015). As an example, the natural temporal delay between visual and acoustic speech signals provides a means through which the visual signal can alter the phase of ongoing neural oscillations such that the expected acoustic signal occurs at the phase of optimal neuronal excitability (Schroeder et al., 2008). While the phase of low-frequency oscillations may create temporal windows for the selection of relevant sensory information, higher-frequency beta and gamma oscillations may be involved in the transmission of top-down predictions (both formal and temporal) and bottom-up prediction errors, respectively (Arnal et al., 2011; Arnal and Giraud, 2012). If this is indeed the case, then it follows that neural oscillations, particularly within these frequency bands, may facilitate the predictive coding of nonverbal communicative signals such as dynamic facial, body, and vocal expressions. In this respect, emotion perception is similar to other forms of perception, with emotion acting as a highly salient source of relevant information that must be encoded and integrated with other sources of sensory information.
Although early on, Charles Darwin recognized the equal importance of facial, body, and vocal expressions in emotional communication, research over the past 50 years has focused predominantly on the perception of emotion from facial expressions. Thus, the role of neural oscillations in emotion perception has primarily been studied by presenting participants with images of static facial expressions. While this approach has yielded some valuable results, it does not necessarily reflect how emotions are expressed and perceived in natural human communication.
In everyday life, emotional expressions are dynamic, characterized by changes in facial expression, body language, and prosody unfolding over time. To this end, previous functional neuroimaging research has shown distinct neural pathways involved in the perception of emotion from static and dynamic facial expressions (i.e., Kilts et al., 2003). Consistent with these findings, results from Jabbi et al. (2015) suggest that oscillatory activity in the beta frequency band may track dynamic changes in sensory input facilitating the differentiation of emotional expressions. Although the use of dynamic facial expressions adds an additional level of stimulus complexity, it also affords greater ecological validity, which can improve our understanding of the neural dynamics underpinning naturalistic emotion perception. Moreover, the dynamic nature of emotional expressions enables the brain to use incoming sensory input to generate predictions about future events. Future studies using methods such as dynamic causal modeling (DCM) can be used to compare convergent and predictive coding models of multisensory emotion perception.
A second issue relates to the fact that facial expressions are also not the only means of emotional communication. Changes in emotional body language (De Gelder, 2006) and prosody (Schirmer and Kotz, 2006) also provide important information about one’s emotional state. Compared to facial expressions, however, little is known about the oscillatory dynamics underpinning the perception of emotion from body and vocal expressions. Therefore, further research into: (i) the perception of emotion from dynamic body and vocal expressions; and (ii) the integration of emotional expressions from multiple modalities is needed if we are to understand the neural bases of emotion perception in human social interactions.
Each emotion is associated with a unique physiological, cognitive, and behavioral profile that serves an adaptive and, in social species, a communicative function. Therefore, it is likely that distinct patterns of neural activity and connectivity drive the expression and perception of different emotions.
One of the broadest distinctions between emotions is that of valence, which categorizes emotions as positive (pleasant) or negative (unpleasant). Within the brain, some have proposed that the right hemisphere is dominant for the processing of negative emotions while the left hemisphere is dominant for positive emotions (Ahern and Schwartz, 1979, 1985; Silberman and Weingartner, 1986). Although valence-specific asymmetry has primarily been discussed within the context of emotional experience, studies in healthy individuals and in patients with unilateral brain damage suggest that there may also be hemispheric asymmetry in the perception of emotion (i.e., Jansari et al., 2000; Adolphs et al., 2001), though this may be influenced by task demands (Kotz et al., 2003, 2006). Consistent with this hypothesis, there is preliminary support for valence-specific hemispheric asymmetry of alpha desynchronization during the emotion perception (i.e., Balconi and Ferrari, 2012). However, given support for alternative hypotheses such as the approach-withdrawal model of hemispheric lateralization (Davidson, 1992), future studies examining patterns of coherence across brain regions during the perception of positive and negative emotions are needed in order to elucidate the functional dynamics underpinning the differentiation of emotional valence.
Since each emotion serves a distinct function, it has been hypothesized that there may be different, yet partially overlapping, neural pathways specialized for the processing of different emotions (i.e., LeDoux, 2000). Thus, we may expect specific patterns of neural synchronization during the perception of different emotions. In support of this idea, distinct spatial and temporal patterns of theta (Knyazev et al., 2009b) and gamma (Luo et al., 2007) band activity have been observed in response to different emotions. So although perception of different emotions may rely partially overlapping networks, further investigations into patterns of neural synchronization and coherence may reveal subtle changes in functional dynamics that enable us to differentiate between emotions.
Due to the interaction between neurophysiological and environmental factors, individual differences can have a profound effect on how we perceive and interpret nonverbal expressions of emotion. Underlying these individual differences are changes in functional coupling that can be investigated by examining patterns of neural synchrony. To this end, gender differences are reflected in beta (Güntekin and Başar, 2007b) and theta (Knyazev et al., 2010) synchronization in response to emotional facial expressions. Furthermore, alpha desynchronization has been negatively associated with extraversion (Fink, 2005) and hostility (Knyazev et al., 2009b) and positively associated with anxiety (Knyazev et al., 2008) and depression (Knyazev et al., 2015). Individual differences have also been observed in the theta band, with reduced frontal theta synchronization in individuals with high levels of anxiety (Knyazev et al., 2008) and depression (Knyazev et al., 2015) and increased theta synchronization in those scoring high on measures of emotional intelligence (Knyazev et al., 2013). Additionally, hostility has been associated with gender differences in alpha and theta synchronization over posterior regions (Knyazev et al., 2009b) while dominance motivation is associated with delta/beta asymmetry (Hofman et al., 2013). Taken together, these findings suggest that changes in patterns of neural synchronization may mediate individual differences in the perception of emotional expressions.
Deficits in the ability to accurately perceive and interpret emotions have been observed in a number of neurological and psychiatric conditions, the neural bases of which remain poorly understood. By enabling us to look beyond the activity of specific brain regions into the dynamics of functional neural networks, investigations into changes in neural synchronization and coherence can advance our understanding of the specific impairments associated with different clinical conditions. Work in this area has already begun with studies showing reduced theta synchronization during perception of emotional facial expressions in individuals with schizophrenia (Ramos-Loyo et al., 2009; Csukly et al., 2014). Schizophrenia has also been associated with abnormal patterns of alpha synchronization (Ramos-Loyo et al., 2009; Popov et al., 2014), though this may be improved through targeted training in facial affect recognition (Popova et al., 2014). Other studies have found that oscillatory responses to facial expressions in the gamma band differentiate between unipolar and bipolar depression; while individuals with unipolar depression show reduced gamma power in response to sad facial expressions, those with bipolar show increased gamma band activity in response to highly arousing emotions (Liu et al., 2014). Finally, adolescents with Autism Spectrum Disorder show reduced interregional beta synchronization in response to angry facial expressions, suggesting that impairments in functional connectivity within networks involved in emotion processing may contribute to the deficits in facial emotion perception observed in autism (Leung et al., 2014). Thus, a better characterization of oscillatory responses to emotional expressions may aid in the diagnosis and treatment of a number of clinical conditions.
From the reviewed studies, it is clear that the perception of facial, body, and vocal expressions of emotion is mediated by oscillatory activity in multiple frequency bands. Although research on delta synchronization has been primarily restricted to the visual domain, the important delta oscillations and their functional coupling with higher (beta/gamma) frequency bands, in basic biological, cognitive, and emotional processes highlights the need for further research into the functional role of delta oscillations in emotion perception within and between sensory modalities. Across modalities, theta synchronization most consistently differentiates between emotional and neutral expressions and may reflect the initial encoding and derivation of emotional significance. Changes in alpha power have been primarily observed in studies with a visual component, with some evidence of valence-specific lateralization over frontal regions. Based on the hypothesis that alpha synchronization reflects active inhibition of task irrelevant brain regions (Klimesch et al., 2007), modulation of alpha power may reflect sensory selection and inhibition of behaviorally relevant sensory information. Although evidence is still inconclusive as to the role of beta oscillations in emotion perception, changes in beta power are more likely to be observed in studies using dynamic stimuli or in those involving shifts in attention, consistent with the idea that beta band activity reflects the maintenance of the current cognitive or sensorimotor set (Engel and Fries, 2010). Gamma synchronization has been observed in emotion processing regions such as the amygdala, STS, and OFC, suggesting that oscillatory activity in this frequency band is associated with the binding of emotionally salient sensory input. The modulation of specific frequency bands by emotion enables the selective detection, integration, and evaluation of emotional signals through coordinated changes in effective connectivity. From a predictive coding perspective, the emotional quality of the expression may act as a particularly salient source of information, strengthening the precision of sensory predictions through enhanced neural synchronization. However, further research, particularly in the auditory and audiovisual domains, is clearly necessary to gain a deeper understanding of the neural dynamics underpinning the perception of emotion within and between sensory modalities.
Main contribution by first author (AES). All the other authors contributed equally to this work.
The authors declare that the research was conducted in the absence of any commercial or financial relationships that could be construed as a potential conflict of interest.
Adolphs, R. (2002a). Neural systems for recognizing emotion. Curr. Opin. Neurobiol. 12, 169–177. doi: 10.1016/s0959-4388(02)00301-x
Adolphs, R. (2002b). Recognizing emotion from facial expressions: psychological and neurological mechanisms. Behav. Cogn. Neurosci. Rev. 1, 21–62. doi: 10.1177/1534582302001001003
Adolphs, R., Jansari, A., and Tranel, D. (2001). Hemispheric perception of emotional valence from facial expressions. Neuropsychology 15, 516–524. doi: 10.1037/0894-4105.15.4.516
Ahern, G. L., and Schwartz, G. E. (1979). Differential lateralization for positive versus negative emotion. Neuropsychologia 17, 693–698. doi: 10.1016/0028-3932(79)90045-9
Ahern, G. L., and Schwartz, G. E. (1985). Differential lateralization for positive and negative emotion in the human brain: EEG spectral analysis. Neuropsychologia 23, 745–755. doi: 10.1016/0028-3932(85)90081-8
Arnal, L. H., and Giraud, A. L. (2012). Cortical oscillations and sensory predictions. Trends Cogn. Sci. 16, 390–398. doi: 10.1016/j.tics.2012.05.003
Arnal, L. H., Wyart, V., and Giraud, A. L. (2011). Transitions in neural oscillations reflect prediction errors generated in audiovisual speech. Nat. Neurosci. 14, 797–801. doi: 10.1038/nn.2810
Balconi, M., and Ferrari, C. (2012). Subliminal and supraliminal processing of facial expression of emotions: brain oscillation in the left/right frontal area. Brain Sci. 2, 85–100. doi: 10.3390/brainsci2020085
Balconi, M., and Lucchiari, C. (2006). EEG correlates (event-related desynchronization) of emotional face elaboration: a temporal analysis. Neurosci. Lett. 392, 118–123. doi: 10.1016/j.neulet.2005.09.004
Balconi, M., and Lucchiari, C. (2008). Consciousness and arousal effects on emotional face processing as revealed by brain oscillations. A γ band analysis. Int. J. Psychophysiol. 67, 41–46. doi: 10.1016/j.ijpsycho.2007.10.002
Balconi, M., and Pozzoli, U. (2007). Event-related oscillations (EROs) and event-related potentials (ERPs) comparison in facial expression recognition. J. Neuropsychol. 1, 283–294. doi: 10.1348/174866407x184789
Balconi, M., and Pozzoli, U. (2008). Event-related oscillations (ERO) and event-related potentials (ERP) in emotional face recognition. Int. J. Neurosci. 118, 1412–1424. doi: 10.1080/00207450601047119
Balconi, M., and Pozzoli, U. (2009). Arousal effect on emotional face comprehension. Frequency band changes in different time intervals. Physiol. Behav. 97, 455–462. doi: 10.1016/j.physbeh.2009.03.023
Başar, E., Güntekin, B., and Öniz, A. (2006). Principles of oscillatory brain dynamics and a treatise of recognition of faces and facial expressions. Prog. Brain Res. 159, 43–62. doi: 10.1016/s0079-6123(06)59004-1
Bastiaansen, M., Mazaheri, A., and Jensen, O. (2012). “Beyond ERPs: oscillatory neuronal dynamics,” in The Oxford Handbook of Event-Related Potential Components, eds S. J. Luck, and E. S. Kappenman (New York, NY: Oxford University Press), 31–50.
Beauchamp, M. S., Argall, B. D., Bodurka, J., Duyn, J. H., and Martin, A. (2004a). Unraveling multisensory integration: patchy organization within human STS multisensory cortex. Nat. Neurosci. 7, 1190–1192. doi: 10.1038/nn1333
Beauchamp, M. S., Lee, K. E., Argall, B. D., and Martin, A. (2004b). Integration of auditory and visual information about objects in superior temporal sulcus. Neuron 41, 809–823. doi: 10.1016/s0896-6273(04)00070-4
Bekkedal, M. Y. V., Rossi, J. III, and Panksepp, J. (2011). Human brain EEG indices of emotions: delineating responses to affective vocalizations by measuring frontal theta event-related synchronization. Neurosci. Biobehav. Rev. 35, 1959–1970. doi: 10.1016/j.neubiorev.2011.05.001
Belin, P., Fecteau, S., and Bédard, C. (2004). Thinking the voice: neural correlates of voice perception. Trends Cogn. Sci. 8, 129–135. doi: 10.1016/j.tics.2004.01.008
Berger, H. (1929). Über das elektrenkephalogramm des menschen. Eur. Arch. Psychiatry Clin. Neurosci. 87, 527–570. doi: 10.1007/bf01797193
Blair, R. J. R., Morris, J. S., Frith, C. D., Perrett, D. I., and Dolan, R. J. (1999). Dissociable neural responses to facial expressions of sadness and anger. Brain 122, 883–893. doi: 10.1093/brain/122.5.883
Brovelli, A., Ding, M., Ledberg, A., Chen, Y., Nakamura, R., and Bressler, S. L. (2004). α oscillations in a large-scale sensorimotor cortical network: directional influences revealed by Granger causality. Proc. Natl. Acad. Sci. U S A 101, 9849–9854. doi: 10.1073/pnas.0308538101
Brück, C., Kreifelts, B., and Wildgruber, D. (2011). Emotional voices in context: a neurobiological model of multimodal affective information processing. Phys. Life Rev. 8, 383–403. doi: 10.1016/j.plrev.2011.10.002
Calvert, G. A., Campbell, R., and Brammer, M. J. (2000). Evidence from functional magnetic resonance imaging of crossmodal binding in the human heteromodal cortex. Curr. Biol. 10, 649–657. doi: 10.1016/s0960-9822(00)00513-3
Calvert, G. A., Hansen, P. C., Iversen, S. D., and Brammer, M. J. (2001). Detection of audio-visual integration sites in humans by application of electrophysiological criteria to the BOLD effect. Neuroimage 14, 427–438. doi: 10.1006/nimg.2001.0812
Canolty, R. T., and Knight, R. T. (2010). The functional role of cross-frequency coupling. Trends Cogn. Sci. 14, 506–515. doi: 10.1016/j.tics.2010.09.001
Chen, Y. H., Edgar, J. C., Holroyd, T., Dammers, J., Thönneßen, H., Roberts, T. P. L., et al. (2010). Neuromagnetic oscillations to emotional faces and prosody. Eur. J. Neurosci. 31, 1818–1827. doi: 10.1111/j.1460-9568.2010.07203.x
Chen, X., Pan, Z., Wang, P., Yang, X., Liu, P., You, X., et al. (2015). The integration of facial and vocal cues during emotional change perception: EEG markers. Soc. Cogn. Affect. Neurosci. doi: 10.1093/scan/nsv083 [Epub ahead of print].
Chen, X., Pan, Z., Wang, P., Zhang, L., and Yuan, J. (2014). EEG oscillations reflect task effects for the change detection in vocal emotion. Cogn. Neurodyn. 9, 351–358. doi: 10.1007/s11571-014-9326-9
Chen, X., Yang, J., Gan, S., and Yang, Y. (2012). The contribution of sound intensity in vocal emotion perception: behavioral and electrophysiological evidence. PLoS One 7:e30278. doi: 10.1371/journal.pone.0030278
Collignon, O., Girard, S., Gosselin, F., Roy, S., Saint-Amour, D., Lassonde, M., et al. (2008). Audio-visual integration of emotion expression. Brain Res. 1242, 126–135. doi: 10.1016/j.brainres.2008.04.023
Csukly, G., Stefanics, G., Komlósi, S., Czigler, I., and Czobor, P. (2014). Event-related theta synchronization predicts deficit in facial affect recognition in schizophrenia. J. Abnorm. Psychol. 123, 178–189. doi: 10.1037/a0035793
Davidson, R. J. (1992). Anterior cerebral asymmetry and the nature of emotion. Brain Cogn. 20, 125–151. doi: 10.1016/0278-2626(92)90065-t
De Gelder, B. (2006). Towards the neurobiology of emotional body language. Nat. Rev. Neurosci. 7, 242–249. doi: 10.1038/nrn1872
Del Zotto, M., Deiber, M. P., Legrand, L. B., De Gelder, B., and Pegna, A. J. (2013). Emotional expressions modulate low alpha and α oscillations in a cortically blind patient. Int. J. Psychophysiol. 90, 358–362. doi: 10.1016/j.ijpsycho.2013.10.007
Eimer, M., and Holmes, A. (2002). An ERP study on the time course of emotional face processing. Neuroreport 13, 427–431. doi: 10.1097/00001756-200203250-00013
Eimer, M., and Holmes, A. (2007). Event-related brain potential correlates of emotional face processing. Neuropsychologia 45, 15–31. doi: 10.1016/j.neuropsychologia.2006.04.022
Eimer, M., Holmes, A., and McGlone, F. P. (2003). The role of spatial attention in the processing of facial expression: an ERP study of rapid brain responses to six basic emotions. Cogn. Affect. Behav. Neurosci. 3, 97–110. doi: 10.3758/cabn.3.2.97
Engel, A. K., and Fries, P. (2010). β-band oscillations-signalling the status quo? Curr. Opin. Neurobiol. 20, 156–165. doi: 10.1016/j.conb.2010.02.015
Ethofer, T., Bretscher, J., Gschwind, M., Kreifelts, B., Wildgruber, D., and Vuilleumier, P. (2011). Emotional voice areas: anatomic location, functional properties and structural connections revealed by combined fMRI/DTI. Cereb. Cortex 22, 191–200. doi: 10.1093/cercor/bhr113
Ethofer, T., Van De Ville, D., Scherer, K., and Vuilleumier, P. (2009). Decoding of emotional information in voice-sensitive cortices. Curr. Biol. 19, 1028–1033. doi: 10.1016/j.cub.2009.04.054
Fecteau, S., Belin, P., Joanette, Y., and Armony, J. L. (2007). Amygdala responses to nonlinguistic emotional vocalizations. Neuroimage 36, 480–487. doi: 10.1016/j.neuroimage.2007.02.043
Fink, A. (2005). Event-related desynchronization in the EEG during emotional and cognitive information processing: differential effects of extraversion. Biol. Psychol. 70, 152–160. doi: 10.1016/j.biopsycho.2005.01.013
Föcker, J., Gondan, M., and Röder, B. (2011). Preattentive processing of audio-visual emotional signals. Acta Psychol. (Amst) 137, 36–47. doi: 10.1016/j.actpsy.2011.02.004
Fries, P. (2005). A mechanism for cognitive dynamics: neuronal communication through neuronal coherence. Trends Cogn. Sci. 9, 474–480. doi: 10.1016/j.tics.2005.08.011
Fries, P., Schröder, J.-H., Roelfsema, P. R., Singer, W., and Engel, A. K. (2002). Oscillatory neuronal synchronization in primary visual cortex as a correlate of stimulus selection. J. Neurosci. 22, 3739–3754.
Frühholz, S., and Grandjean, D. (2013a). Processing of emotional vocalizations in bilateral inferior frontal cortex. Neurosci. Biobehav. Rev. 37, 2847–2855. doi: 10.1016/j.neubiorev.2013.10.007
Frühholz, S., and Grandjean, D. (2013b). Amygdala subregions differentially respond and rapidly adapt to threatening voices. Cortex 49, 1394–1403. doi: 10.1016/j.cortex.2012.08.003
Fulford, D., Peckham, A. D., Johnson, K., and Johnson, S. L. (2014). Emotion perception and quality of life in bipolar I disorder. J. Affect. Disord. 152–154, 491–497. doi: 10.1016/j.jad.2013.08.034
Garrido-Vásquez, P., Jessen, S., and Kotz, S. A. (2011). Perception of emotion in psychiatric disorders: on the possible role of task, dynamics and multimodality. Soc. Neurosci. 6, 515–536. doi: 10.1080/17470919.2011.620771
González-Roldan, A. M., Martínez-Jauand, M., Muñoz-García, M. A., Sitges, C., Cifre, I., and Montoya, P. (2011). Temporal dissociation in the brain processing of pain and anger faces with different intensities of emotional expression. Pain 152, 853–859. doi: 10.1016/j.pain.2010.12.037
Grèzes, J., Pichon, S., and de Gelder, B. (2007). Perceiving fear in dynamic body expressions. Neuroimage 35, 959–967. doi: 10.1016/j.neuroimage.2006.11.030
Güntekin, B., and Başar, E. (2007a). Emotional face expressions are differentiated with brain oscillations. Int. J. Psychophysiol. 64, 91–100. doi: 10.1016/j.ijpsycho.2006.07.003
Güntekin, B., and Başar, E. (2007b). Gender differences influence brain’s α oscillatory responses in recognition of facial expressions. Neurosci. Lett. 424, 94–99. doi: 10.1016/j.neulet.2007.07.052
Güntekin, B., and Başar, E. (2009). Facial affect manifested by multiple oscillations. Int. J. Psychophysiol. 71, 31–36. doi: 10.1016/j.ijpsycho.2008.07.019
Güntekin, B., and Başar, E. (2014). A review of brain oscillations in perception of faces and emotional pictures. Neuropsychologia 58, 33–51. doi: 10.1016/j.neuropsychologia.2014.03.014
Güntekin, B., and Başar, E. (2015). Review of evoked and event-related delta responses in the human brain. Int. J. Psychophysiol. doi: 10.1016/j.ijpsycho.2015.02.001 [Epub ahead of print].
Hadjikhani, N., and de Gelder, B. (2003). Seeing fearful body expressions activates the fusiform cortex and amygdala. Curr. Biol. 13, 2201–2205. doi: 10.1016/j.cub.2003.11.049
Hagan, C. C., Woods, W., Johnson, S., Calder, A. J., Green, G. G. R., and Young, A. W. (2009). MEG demonstrates a supra-additive response to facial and vocal emotion in the right superior temporal sulcus. Proc. Natl. Acad. Sci. U S A 106, 20010–20015. doi: 10.1073/pnas.0905792106
Hagan, C. C., Woods, W., Johnson, S., Green, G. G. R., and Young, A. W. (2013). Involvement of right STS in audio-visual integration for affective speech demonstrated using MEG. PLoS One 8:e70648. doi: 10.1371/journal.pone.0070648
Ho, H. T., Schröger, E., and Kotz, S. A. (2015). Selective attention modulates early human evoked potentials during emotional face-voice processing. J. Cogn. Neurosci. 27, 798–818. doi: 10.1162/joc_a_00734
Hofman, D., Terburg, D., van Wielink, L., and Schutter, D. J. L. G. (2013). Coalescence of dominance motivation and responses to facial anger in resting-state and event-related electrophysiology. Neuroimage 79, 138–144. doi: 10.1016/j.neuroimage.2013.04.088
Hyafil, A., Giraud, A. L., Fontolan, L., and Gutkin, B. (2015). Neural cross-frequency coupling: connecting architectures, mechanisms and functions. Trends Neurosci. 38, 725–740. doi: 10.1016/j.tins.2015.09.001
Jabbi, M., Kohn, P. D., Nash, T., Ianni, A., Coutlee, C., Holroyd, T., et al. (2015). Convergent BOLD and α-band activity in superior temporal sulcus and frontolimbic circuitry underpins human emotion cognition. Cereb. Cortex 25, 1878–1888. doi: 10.1093/cercor/bht427
Jansari, A., Tranel, D., and Adolphs, R. (2000). A valence-specific lateral bias for discriminating emotional facial expressions in free field. Cogn. Emot. 14, 341–353. doi: 10.1080/026999300378860
Jensen, O., and Mazaheri, A. (2010). Shaping functional architecture by oscillatory alpha activity: gating by inhibition. Front. Hum. Neurosci. 4:186. doi: 10.3389/fnhum.2010.00186
Jessen, S., and Kotz, S. A. (2011). The temporal dynamics of processing emotions from vocal, facial and bodily expressions. Neuroimage 58, 665–674. doi: 10.1016/j.neuroimage.2011.06.035
Jessen, S., and Kotz, S. A. (2013). On the role of crossmodal prediction in audiovisual emotion perception. Front. Hum. Neurosci. 7:369. doi: 10.3389/fnhum.2013.00369
Jessen, S., and Kotz, S. A. (2015). Affect differentially modulates brain activation in uni-and multisensory body-voice perception. Neuropsychologia 66, 134–143. doi: 10.1016/j.neuropsychologia.2014.10.038
Jessen, S., Obleser, J., and Kotz, S. A. (2012). How bodies and voices interact in early emotion perception. PLoS One 7:e36070. doi: 10.1371/journal.pone.0036070
Jung, J., Bayle, D., Jerbi, K., Vidal, J. R., Hénaff, M. A., Ossandon, T., et al. (2011). Intracerebral γ modulations reveal interaction between emotional processing and action outcome evaluation in the human orbitofrontal cortex. Int. J. Psychophysiol. 79, 64–72. doi: 10.1016/j.ijpsycho.2010.09.014
Kilts, C. D., Egan, G., Gideon, D. A., Ely, T. D., and Hoffman, J. M. (2003). Dissociable neural pathways are involved in the recognition of emotion in static and dynamic facial expressions. Neuroimage 18, 156–168. doi: 10.1006/nimg.2002.1323
Klimesch, W. (1999). EEG alpha and theta oscillations reflect cognitive and memory performance: a review and analysis. Brain Res. Rev. Res. Rev. 29, 169–195. doi: 10.1016/s0165-0173(98)00056-3
Klimesch, W., Sauseng, P., and Hanslmayr, S. (2007). EEG alpha oscillations: the inhibition-timing hypothesis. Brain Res. Rev. 53, 63–88. doi: 10.1016/j.brainresrev.2006.06.003
Knyazev, G. G. (2007). Motivation, emotion and their inhibitory control mirrored in brain oscillations. Neurosci. Biobehav. Rev. 31, 377–395. doi: 10.1016/j.neubiorev.2006.10.004
Knyazev, G. G. (2012). EEG delta oscillations as a correlate of basic homeostatic and motivational processes. Neurosci. Biobehav. Rev. 36, 677–695. doi: 10.1016/j.neubiorev.2011.10.002
Knyazev, G. G., Bocharov, A. V., Levin, E. A., Savostyanov, A. N., and Slobodskoj-Plusnin, J. Y. (2008). Anxiety and oscillatory responses to emotional facial expressions. Brain Res. 1227, 174–188. doi: 10.1016/j.brainres.2008.06.108
Knyazev, G. G., Bocharov, A. V., Savostyanov, A. N., and Slobodskoy-Plusnin, J. (2015). Predisposition to depression and implicit emotion processing. J. Clin. Exp. Neuropsychol. 37, 701–709. doi: 10.1080/13803395.2015.1061483
Knyazev, G. G., Bocharov, A. V., and Slobodskoj-Plusnin, J. Y. (2009a). Hostility- and gender-related differences in oscillatory responses to emotional facial expressions. Aggress. Behav. 35, 502–513. doi: 10.1002/ab.20318
Knyazev, G. G., Mitrofanova, L. G., and Bocharov, A. V. (2013). Emotional intelligence and oscillatory responses to emotional facial expressions. Hum. Physiol. 39, 371–377. doi: 10.1134/s0362119713030110
Knyazev, G. G., Slobodskoj-Plusnin, J. Y., and Bocharov, A. V. (2009b). Event-related delta and theta synchronization during explicit and implicit emotion processing. Neuroscience 164, 1588–1600. doi: 10.1016/j.neuroscience.2009.09.057
Knyazev, G. G., Slobodskoj-Plusnin, J. Y., and Bocharov, A. V. (2010). Gender differences in implicit and explicit processing of emotional facial expressions as revealed by event-related theta synchronization. Emotion 10, 678–687. doi: 10.1037/a0019175
Kokinous, J., Kotz, S. A., Tavano, A., and Schröger, E. (2015). The role of emotion in dynamic audiovisual integration of faces and voices. Soc. Cogn. Affect. Neurosci. 10, 713–720. doi: 10.1093/scan/nsu105
Kotz, S. A., Hasting, A. S., and Paulmann, S. (2012). “On the orbito-striatal interface in (acoustic) emotional processing,” in The Evolution of Emotional Communication: From Sounds in Nonhuman Mammals to Speech and Music in Man, eds E. Altenmüller, S. Schmidt and E. Zimmermann (New York, NY: Oxford University Press), 229–240.
Kotz, S. A., Meyer, M., Alter, K., Besson, M., von Cramon, D. Y., and Friederici, A. D. (2003). On the lateralization of emotional prosody: an event-related functional MR investigation. Brain Lang. 86, 366–376. doi: 10.1016/s0093-934x(02)00532-1
Kotz, S. A., Meyer, M., and Paulmann, S. (2006). Lateralization of emotional prosody in the brain: an overview and synopsis on the impact of study design. Prog. Brain Res. 156, 285–294. doi: 10.1016/s0079-6123(06)56015-7
Kotz, S. A., and Paulmann, S. (2011). Emotion, language and the brain. Lang. Linguist. Compass 5, 108–125. doi: 10.1111/j.1749-818x.2010.00267.x
Kreifelts, B., Ethofer, T., Shiozawa, T., Grodd, W., and Wildgruber, D. (2009). Cerebral representation of non-verbal emotional perception: fMRI reveals audiovisual integration area between voice- and face-sensitive regions in the superior temporal sulcus. Neuropsychologia 47, 3059–3066. doi: 10.1016/j.neuropsychologia.2009.07.001
Kret, M. E., Pichon, S., Grèzes, J., and de Gelder, B. (2011). Similarities and differences in perceiving threat from dynamic faces and bodies. An fMRI study. Neuroimage 54, 1755–1762. doi: 10.1016/j.neuroimage.2010.08.012
Kringelbach, M. L., and Rolls, E. T. (2004). The functional neuroanatomy of the human orbitofrontal cortex: evidence from neuroimaging and neuropsychology. Prog. Neurobiol. 72, 341–372. doi: 10.1016/j.pneurobio.2004.03.006
LeDoux, J. (1996). The Emotional Brain: The Mysterious Underpinnings of Emotional Life. New York, NY: Simon and Schuster.
LeDoux, J. E. (2000). Emotion circuits in the brain. Annu. Rev. Neurosci. 23, 155–184. doi: 10.1146/annurev.neuro.23.1.155
Leung, R. C., Ye, A. X., Wong, S. M., Taylor, M. J., and Doesburg, S. M. (2014). Reduced α connectivity during emotional face processing in adolescents with autism. Mol. Autism 5:51. doi: 10.1186/2040-2392-5-51
Liu, T. Y., Chen, Y. S., Su, T. P., Hsieh, J. C., and Chen, L. F. (2014). Abnormal early γ responses to emotional faces differentiate unipolar from bipolar disorder patients. Biomed Res. Int. 2014:906104. doi: 10.1155/2014/906104
Liu, T., Pinheiro, A. P., Deng, G., Nestor, P. G., McCarley, R. W., and Niznikiewicz, M. A. (2012). Electrophysiological insights into processing nonverbal emotional vocalizations. Neuroreport 23, 108–112. doi: 10.1097/WNR.0b013e32834ea757
Luo, Q., Holroyd, T., Jones, M., Hendler, T., and Blair, J. (2007). Neural dynamics for facial threat processing as revealed by γ band synchronization using MEG. Neuroimage 34, 839–847. doi: 10.1016/j.neuroimage.2006.09.023
Luo, Q., Holroyd, T., Majestic, C., Cheng, X., Schechter, J., and Blair, R. J. (2010). Emotional automaticity is a matter of timing. J. Neurosci. 30, 5825–5829. doi: 10.1523/JNEUROSCI.BC-5668-09.2010
Luo, Q., Mitchell, D., Cheng, X., Mondillo, K., Mccaffrey, D., Holroyd, T., et al. (2009). Visual awareness, emotion and γ band synchronization. Cereb. Cortex 19, 1896–1904. doi: 10.1093/cercor/bhn216
Meeren, H. K., de Gelder, B., Ahlfors, S. P., Hämäläinen, M. S., and Hadjikhani, N. (2013). Different cortical dynamics in face and body perception: an MEG study. PLoS One 8:e71408. doi: 10.1371/journal.pone.0071408
Müsch, K., Hamamé, C. M., Perrone-Bertolotti, M., Minotti, L., Kahane, P., Engel, A. K., et al. (2014). Selective attention modulates high-frequency activity in the face-processing network. Cortex 60, 34–51. doi: 10.1016/j.cortex.2014.06.006
Muthukumaraswamy, S. D., Johnson, B. W., Gaetz, W. C., and Cheyne, D. O. (2006). Neural processing of observed oro-facial movements reflects multiple action encoding strategies in the human brain. Brain Res. 1071, 105–112. doi: 10.1016/j.brainres.2005.11.053
Muthukumaraswamy, S. D., and Singh, K. D. (2008). Modulation of the human mirror neuron system during cognitive activity. Psychophysiology 45, 896–905. doi: 10.1111/j.1469-8986.2008.00711.x
Paulmann, S., Bleichner, M., and Kotz, S. A. (2013). Valence, arousal and task effects in emotional prosody processing. Front. Psychol. 4:345. doi: 10.3389/fpsyg.2013.00345
Paulmann, S., and Kotz, S. A. (2008). Early emotional prosody perception based on different speaker voices. Neuroreport 19, 209–213. doi: 10.1097/WNR.0b013e3282f454db
Paulmann, S., Jessen, S., and Kotz, S. A. (2012). It’s special the way you say it: an ERP investigation on the temporal dynamics of two types of prosody. Neuropsychologia 50, 1609–1620. doi: 10.1016/j.neuropsychologia.2012.03.014
Pavlova, M. A. (2012). Biological motion processing as a hallmark of social cognition. Cereb. Cortex 22, 981–995. doi: 10.1093/cercor/bhr156
Peelle, J. E., and Davis, M. H. (2012). Neural oscillations carry speech rhythm through to comprehension. Front. Psychol. 3:320. doi: 10.3389/fpsyg.2012.00320
Pell, M. D., Rothermich, K., Liu, P., Paulmann, S., Sethi, S., and Rigoulot, S. (2015). Preferential decoding of emotion from human non-linguistic vocalizations versus speech prosody. Biol. Psychol. 111, 14–25. doi: 10.1016/j.biopsycho.2015.08.008
Pernet, C. R., McAleer, P., Latinus, M., Gorgolewski, K. J., Charest, I., Bestelmeyer, P. E. G., et al. (2015). The human voice areas: spatial organisation and inter-individual variability in temporal and extra-temporal cortices. Neuroimage 119, 164–174. doi: 10.1016/j.neuroimage.2015.06.050
Péron, J., Biseul, I., Leray, E., Vicente, S., Le Jeune, F., Drapier, S., et al. (2010a). Subthalamic nucleus stimulation affects fear and sadness recognition in Parkinson’s disease. Neuropsychology 24, 1-8. doi: 10.1037/a0017433
Péron, J., Frühholz, S., Vérin, M., and Grandjean, D. (2013). Subthalamic nucleus: a key structure for emotional component synchronization in humans. Neurosci. Biobehav. Rev. 37, 358–373. doi: 10.1016/j.neubiorev.2013.01.001
Péron, J., Grandjean, D., Le Jeune, F., Sauleau, P., Haegelen, C., Drapier, D., et al. (2010b). Recognition of emotional prosody is altered after subthalamic nucleus deep brain stimulation in Parkinson’s disease. Neuropsychologia 48, 1053–1062. doi: 10.1016/j.neuropsychologia.2009.12.003
Pfurtscheller, G., Stancák, A., and Neuper, C. (1996). Event-related synchronization (ERS) in the alpha band - an electrophysiological correlate of cortical idling: a review. Int. J. Psychophysiol. 24, 39–46. doi: 10.1016/s0167-8760(96)00066-9
Phillips, M. L., Drevets, W. C., Rauch, S. L., and Lane, R. (2003). Neurobiology of emotion perception II: implications for major psychiatric disorders. Biol. Psychiatry 54, 515–528. doi: 10.1016/s0006-3223(03)00171-9
Phillips, L. H., Henry, J. D., Scott, C., Summers, F., Whyte, M., and Cook, M. (2011). Specific impairments of emotion perception in multiple sclerosis. Neuropsychology 25, 131–136. doi: 10.1037/a0020752
Phillips, L. H., Scott, C., Henry, J. D., Mowat, D., and Bell, J. S. (2010). Emotion perception in Alzheimer’s disease and mood disorder in old age. Psychol. Aging 25, 38–47. doi: 10.1037/a0017369
Phillips, M. L., Young, A. W., Senior, C., Brammer, M., Andrew, C., Calder, A. J., et al. (1997). A specific neural substrate for perceiving facial expressions of disgust. Nature 389, 495–498. doi: 10.1038/39051
Popov, T., Miller, G. A., Rockstroh, B., and Weisz, N. (2013). Modulation of α power and functional connectivity during facial affect recognition. J. Neurosci. 33, 6018–6026. doi: 10.1523/JNEUROSCI.2763-12.2013
Popova, P., Popov, T. G., Wienbruch, C., Carolus, A. M., Miller, G. A., and Rockstroh, B. S. (2014). Changing facial affect recognition in schizophrenia: effects of training on brain dynamics. Neuroimage Clin. 6, 156–165. doi: 10.1016/j.nicl.2014.08.026
Popov, T. G., Rockstroh, B. S., Popova, P., Carolus, A. M., and Miller, G. A. (2014). Dynamics of alpha oscillations elucidate facial affect recognition in schizophrenia. Cogn. Affect. Behav. Neurosci. 14, 364–377. doi: 10.3758/s13415-013-0194-2
Ramos-Loyo, J., González-Garrido, A. A., Sánchez-Loyo, L. M., Medina, V., and Basar-Eroglu, C. (2009). Event-related potentials and event-related oscillations during identity and facial emotional processing in schizophrenia. Int. J. Psychophysiol. 71, 84–90. doi: 10.1016/j.ijpsycho.2008.07.008
Robins, D. L., Hunyadi, E., and Schultz, R. T. (2009). Superior temporal activation in response to dynamic audio-visual emotional cues. Brain Cogn. 69, 269–278. doi: 10.1016/j.bandc.2008.08.007
Rolls, E. T. (2004). The functions of the orbitofrontal cortex. Brain Cogn. 55, 11–29. doi: 10.1016/S0278-2626(03)00277-X
Sato, W., Kochiyama, T., Uono, S., Matsuda, K., Usui, K., Inoue, Y., et al. (2011). Rapid amygdala γ oscillations in response to fearful facial expressions. Neuropsychologia 49, 612–617. doi: 10.1016/j.neuropsychologia.2010.12.025
Schelenz, P. D., Klasen, M., Reese, B., Regenbogen, C., Wolf, D., Kato, Y., et al. (2013). Multisensory integration of dynamic emotional faces and voices: method for simultaneous EEG-fMRI measurements. Front. Hum. Neurosci. 7:729. doi: 10.3389/fnhum.2013.00729
Scherer, K. R. (2000). “Psychological models of emotion” in The Neuropsychology of Emotion, ed J. Board (Oxford, NY: Oxford University Press), 137–162.
Schirmer, A., and Kotz, S. A. (2006). Beyond the right hemisphere: brain mechanisms mediating vocal emotional processing. Trends Cogn. Sci. 10, 24–30. doi: 10.1016/j.tics.2005.11.009
Schirmer, A., Striano, T., and Friederici, A. D. (2005). Sex differences in the preattentive processing of vocal emotional expressions. Neuroreport 16, 635–639. doi: 10.1097/00001756-200504250-00024
Schroeder, C. E., Lakatos, P., Kajikawa, Y., Partan, S., and Puce, A. (2008). Neuronal oscillations and visual amplification of speech. Trends Cogn. Sci. 12, 106–113. doi: 10.1016/j.tics.2008.01.002
Schutter, D. J. L. G., Putman, P., Hermans, E., and van Honk, J. (2001). Parietal electroencephalogram α asymmetry and selective attention to angry facial expressions in healthy human subjects. Neurosci. Lett. 314, 13–16. doi: 10.1016/s0304-3940(01)02246-7
Schwarzlose, R. F., Baker, C. I., and Kanwisher, N. (2005). Separate face and body selectivity on the fusiform gyrus. J. Neurosci. 25, 11055–11059. doi: 10.1523/jneurosci.2621-05.2005
Senkowski, D., Schneider, T. R., Foxe, J. J., and Engel, A. K. (2008). Crossmodal binding through neural coherence: implications for multisensory processing. Trends Neurosci. 31, 401–409. doi: 10.1016/j.tins.2008.05.002
Silberman, E. K., and Weingartner, H. (1986). Hemispheric lateralization of functions related to emotion. Brain Cogn. 5, 322–353. doi: 10.1016/0278-2626(86)90035-7
Singer, W., and Gray, C. M. (1995). Visual feature integration and the temporal correlation hypothesis. Annu. Rev. Neurosci. 18, 555–586. doi: 10.1146/annurev.neuro.18.1.555
Stefanics, G., Csukly, G., Komlósi, S., Czobor, P., and Czigler, I. (2012). Processing of unattended facial emotions: a visual mismatch negativity study. Neuroimage 59, 3042–3049. doi: 10.1016/j.neuroimage.2011.10.041
Stevenson, R. A., Geoghegan, M. L., and James, T. W. (2007). Superadditive BOLD activation in superior temporal sulcus with threshold non-speech objects. Exp. Brain Res. 179, 85–95. doi: 10.1007/s00221-006-0770-6
Stevenson, R. A., and James, T. W. (2009). Audiovisual integration in human superior temporal sulcus: inverse effectiveness and the neural processing of speech and object recognition. Neuroimage 44, 1210–1223. doi: 10.1016/j.neuroimage.2008.09.034
Tallon-Baudry, C., and Bertrand, O. (1999). Oscillatory γ activity and its role in object representation. Trends Cogn. Sci. 3, 151–162. doi: 10.1016/s1364-6613(99)01299-1
Tanaka, A., Koizumi, A., Imai, H., Hiramatsu, S., Hiramoto, E., and de Gelder, B. (2010). I feel your voice. Cultural differences in the multisensory perception of emotion. Psychol. Sci. 21, 1259–1262. doi: 10.1177/0956797610380698
van Atteveldt, N., Murray, M. M., Thut, G., and Schroeder, C. E. (2014). Multisensory integration: flexible use of general operations. Neuron 81, 1240–1253. doi: 10.1016/j.neuron.2014.02.044
Vroomen, J., and Stekelenburg, J. J. (2010). Visual anticipatory information modulates multisensory interactions of artificial audiovisual stimuli. J. Cogn. Neurosci. 22, 1583–1596. doi: 10.1162/jocn.2009.21308
Vuilleumier, P., and Pourtois, G. (2007). Distributed and interactive brain mechanisms during emotion face perception: evidence from functional neuroimaging. Neuropsychologia 45, 174–194. doi: 10.1016/j.neuropsychologia.2006.06.003
Watson, R., Latinus, M., Noguchi, T., Garrod, O., Crabbe, F., and Belin, P. (2013). Dissociating task difficulty from incongruence in face-voice emotion integration. Front. Hum. Neurosci. 7:744. doi: 10.3389/fnhum.2013.00744
Watson, R., Latinus, M., Noguchi, T., Garrod, O., Crabbe, F., and Belin, P. (2014). Crossmodal adaptation in right posterior superior temporal sulcus during face-voice emotional integration. J. Neurosci. 34, 6813–6821. doi: 10.1523/JNEUROSCI.4478-13.2014
Whalen, P. J., Raila, H., Bennett, R., Mattek, A., Brown, A., Taylor, J., et al. (2013). Neuroscience and facial expressions of emotion: the role of amygdala-prefrontal interactions. Emot. Rev. 5, 78–83. doi: 10.1177/1754073912457231
Whalen, P. J., Shin, L. M., McInerney, S. C., Fischer, H., Wright, C. I., and Rauch, S. L. (2001). A functional MRI study of human amygdala responses to facial expressions of fear versus anger. Emotion 1, 70-83. doi: 10.1037/1528-3542.1.1.70
Wiethoff, S., Wildgruber, D., Kreifelts, B., Becker, H., Herbert, C., Grodd, W., et al. (2008). Cerebral processing of emotional prosody—influence of acoustic parameters and arousal. Neuroimage 39, 885–893. doi: 10.1016/j.neuroimage.2007.09.028
Wildgruber, D., Ethofer, T., Grandjean, D., and Kreifelts, B. (2009). A cerebral network model of speech prosody comprehension. Int. J. Speech Lang. Pathol. 11, 277–281. doi: 10.1080/17549500902943043
Williams, M. A., Morris, A. P., McGlone, F., Abbott, D. F., and Mattingley, J. B. (2004). Amygdala responses to fearful and happy facial expressions under conditions of binocular suppression. J. Neurosci. 24, 2898–2904. doi: 10.1523/jneurosci.4977-03.2004
Woods, D. L., and Alain, C. (2009). Functional imaging of human auditory cortex. Curr. Opin. Otolaryngol. Head Neck Surg. 17, 407–411. doi: 10.1097/MOO.0b013e3283303330
Keywords: emotion, nonverbal communication, multisensory, cross-modal prediction, neural oscillations
Citation: Symons AE, El-Deredy W, Schwartze M and Kotz SA (2016) The Functional Role of Neural Oscillations in Non-Verbal Emotional Communication. Front. Hum. Neurosci. 10:239. doi: 10.3389/fnhum.2016.00239
Received: 11 January 2016; Accepted: 09 May 2016;
Published: 25 May 2016.
Edited by:
Anne Keitel, University of Glasgow, UKReviewed by:
Didier Grandjean, University of Geneva, SwitzerlandCopyright © 2016 Symons, El-Deredy, Schwartze and Kotz. This is an open-access article distributed under the terms of the Creative Commons Attribution License (CC BY). The use, distribution and reproduction in other forums is permitted, provided the original author(s) or licensor are credited and that the original publication in this journal is cited, in accordance with accepted academic practice. No use, distribution or reproduction is permitted which does not comply with these terms.
*Correspondence: Ashley E. Symons, YXNobGV5LnN5bW9uc0BtYW5jaGVzdGVyLmFjLnVr
Disclaimer: All claims expressed in this article are solely those of the authors and do not necessarily represent those of their affiliated organizations, or those of the publisher, the editors and the reviewers. Any product that may be evaluated in this article or claim that may be made by its manufacturer is not guaranteed or endorsed by the publisher.
Research integrity at Frontiers
Learn more about the work of our research integrity team to safeguard the quality of each article we publish.