- 1Neuroimaging of Epilepsy Laboratory, Montreal Neurological Institute and Hospital, McGill University, Montreal, QC, Canada
- 2Department of Social Neuroscience, Max Planck Institute for Human Cognitive and Brain Sciences, Leipzig, Germany
Early imaging studies in temporal lobe epilepsy (TLE) focused on the search for mesial temporal sclerosis, as its surgical removal results in clinically meaningful improvement in about 70% of patients. Nevertheless, a considerable subgroup of patients continues to suffer from post-operative seizures. Although the reasons for surgical failure are not fully understood, electrophysiological and imaging data suggest that anomalies extending beyond the temporal lobe may have negative impact on outcome. This hypothesis has revived the concept of human epilepsy as a disorder of distributed brain networks. Recent methodological advances in non-invasive neuroimaging have led to quantify structural and functional networks in vivo. While structural networks can be inferred from diffusion MRI tractography and inter-regional covariance patterns of structural measures such as cortical thickness, functional connectivity is generally computed based on statistical dependencies of neurophysiological time-series, measured through functional MRI or electroencephalographic techniques. This review considers the application of advanced analytical methods in structural and functional connectivity analyses in TLE. We will specifically highlight findings from graph-theoretical analysis that allow assessing the topological organization of brain networks. These studies have provided compelling evidence that TLE is a system disorder with profound alterations in local and distributed networks. In addition, there is emerging evidence for the utility of network properties as clinical diagnostic markers. Nowadays, a network perspective is considered to be essential to the understanding of the development, progression, and management of epilepsy.
Introduction
Epilepsy is one of the most prevalent neurological disorders, affecting ~1% of the general population. Of patients treated with antiepileptic drugs, about one third never achieve remission. Drug-resistance should be identified early and treated effectively, as uncontrolled epilepsy is harmful to the brain, has devastating socio-economic consequences, and is associated with increased mortality (Leonardi and Ustun, 2002; Pugliatti et al., 2007; Tellez-Zenteno et al., 2007; Cascino, 2009; Coan and Cendes, 2013). Epilepsy is broadly defined by a state of recurrent spontaneous seizures, which arise when balance between excitation and inhibition is disrupted (Scharfman, 2007; Engel et al., 2013). Compelling evidence from animal models, experimental paradigms, and clinical work in humans indicates that specific cortical and subcortical networks play a fundamental role in the genesis and expression of seizures (Avoli and Gloor, 1982; Bear et al., 1996; Bertram, 1997; Spencer, 2002).
Temporal lobe epilepsy (TLE) is the most common drug-resistant epilepsy in adults. TLE is traditionally associated with mesiotemporal sclerosis, defined by cell loss and gliosis in the hippocampus, entorhinal cortex, and amygdala. Surgical resection of this epileptogenic lesion is the current treatment of choice (Wiebe et al., 2001), and leads to freedom from seizures in the majority of cases. Nevertheless, even in carefully selected cases, ~30% of surgical candidates continue to have seizures (Mcintosh et al., 2004; Bernhardt et al., 2010). Although reasons for surgical failure are not fully understood, electrophysiological and imaging data suggest that anomalies extending beyond the temporal lobe may have negative impact on outcome. This hypothesis has revived the concept of human epilepsy as a disorder of distributed neural networks (Spencer, 2002; Bonilha et al., 2007b; Elsharkawy et al., 2009; Engel et al., 2013).
In the past decade, advances in imaging acquisition and postprocessing have permitted in vivo mapping of the regional distribution of network abnormalities in TLE patients. In particular, quantitative structural MRI studies based on volumetry, voxel-based morphometry, cortical thickness mapping, and structural covariance analysis have shown widespread, coordinated, and progressive cortical gray matter loss in temporal and extra-temporal regions, such as the thalamus, fronto-limbic, and fronto-central neocortices (Bernasconi et al., 2003b, 2004; Natsume et al., 2003; Bonilha et al., 2004; Lin et al., 2007; Bernhardt et al., 2008, 2009, 2010, 2012; Keller and Roberts, 2008; Mcdonald et al., 2008b,c). Findings of gray matter alterations have been complemented by diffusion MRI data of the white matter. These studies have shown disruptions in inter-regional fiber diffusivity both within and beyond mesiotemporal and temporo-limbic networks, suggestive of decreased fiber arrangement and altered myelin membranes (Concha et al., 2005; Rodrigo et al., 2007; Yogarajah and Duncan, 2008; Ahmadi et al., 2009). Furthermore, studies based on both electrophysiological techniques as well as functional MRI have provided evidence for region-specific shifts in intrinsic functional networks (Bettus et al., 2009; Voets et al., 2012). More recently, reports of disruptions of inter-regional structural and functional connections in TLE have been complemented by graph-theoretical approaches (Liao et al., 2010; Bernhardt et al., 2011; Bonilha et al., 2012). These techniques, derived from complex system analysis, lend tools to characterize topological aspects that relate to the specialization and integration of inter-connected brain networks (Watts and Strogatz, 1998; Sporns et al., 2004; Bullmore and Sporns, 2009; Guye et al., 2010). In TLE, such approaches provide a novel window to study connectivity, and have begun showing alterations in higher-order network configurations.
The aim of this review is to summarize the current state of imaging evidence for network abnormalities in TLE. We will first outline findings that have provided insights into the topographical extent of regional structural abnormalities in TLE. We will then discuss studies on low-level inter-regional abnormalities, using connectivity mapping techniques such as seed-based structural MRI covariance, functional MRI connectivity, and diffusion MRI tractography. Subsequently, we will discuss graph-theoretical analyses to address the topological organization of brain networks in TLE. We will conclude by commenting on the potential clinical relevance of current network-based MRI analysis in TLE.
Regional Patterns of Structural Pathology
The hallmark lesion of TLE is hippocampal sclerosis. This lesion is characterized by various degrees of neuronal loss and gliosis within hippocampal subfields and the dentate gyrus (Sommer, 1880; Babb and Brown, 1987; Blumcke et al., 2002). In addition, up to 50% of TLE patients may show intense reorganization of neuronal networks, manifested by granule cell dispersion (Houser et al., 1992; Blumcke et al., 2002), selective loss of inhibitory neurons (De Lanerolle et al., 1989), as well as axonal sprouting (Babb et al., 1991). Histological reports of TLE patients and animal models of limbic epilepsy have consistently demonstrated that pathology is not limited to the hippocampus. Indeed, cell loss and gliosis may be found in proximal and even more distal temporo-limbic regions, including the amygdala (Yilmazer-Hanke et al., 2000), entorhinal cortex (Du et al., 1993, 1995), temporopolar (Choi et al., 1999; Meiners et al., 1999; Mitchell et al., 1999; Bothwell et al., 2001) and lateral temporal neocortices (Cavanagh and Meyer, 1956; Falconer et al., 1964; Turski et al., 1983; Clifford et al., 1987; Kuzniecky et al., 1987; Cavalheiro et al., 1991; Thom et al., 2009), as well as the thalamus (Turski et al., 1983; Clifford et al., 1987; Bertram et al., 2001; Sloan and Bertram, 2009). In animal models, tissue damage has been shown in extra-temporal neocortical regions, such as sensorimotor cortex, piriform, perirhinal, retrosplenial, and visual cortices. In humans, pathological data in regions remote from the temporal lobes in TLE is sparse. This is, in part, due to difficulties in obtaining immediate postmortem specimens and the surgical approach tailored to the temporal lobe. In their seminal postmortem study, Margerison and Corsellis described neuronal loss and gliosis in frontal and occipital cortices in about 20% of patients (Margerison and Corsellis, 1966). More recent autopsy reports have confirmed and further extended these observations by showing varying degrees of architectural abnormalities involving virtually all lobes (Eriksson et al., 2002; Blanc et al., 2011).
A large body of electro-clinical work suggests that the epileptogenic network in TLE is broad. Seizure activity may involve not only the hippocampus, but also several other subcortical and cortical structures, including the amygdala, entorhinal cortex, lateral temporal, inferior, as well as orbitofrontal cortices (Lieb et al., 1987, 1991) together with the medial thalamus (Cassidy and Gale, 1998; Rosenberg et al., 2006). The close spatial correspondence between histopathological alterations and electrophysiological anomalies in TLE has provided a strong motivation to study structural brain changes, which have been of a high clinical and scientific value in mapping causes and consequences of drug-resistant epilepsy. In particular, quantitative Magnetic Resonance Imaging (MRI) analysis has offered a unique perspective to study structural substrates of TLE in vivo and to gain further insights into their spatial patterns and clinical correlates (See Figure 1, for a schematic overview of structural MRI findings in TLE). Studies based on manual volumetric MRI analysis largely confirmed previous histological assessments, and provided a more comprehensive picture of the regional extent of structural abnormalities in TLE. Volumetric analysis demonstrated atrophy in multiple limbic structures, including the hippocampus, entorhinal cortex, amygdala (Cendes et al., 1993a,b; Bernasconi et al., 2001, 2003a,b), temporopolar, perirhinal, lateral temporal neocortices (Jutila et al., 2001; Moran et al., 2001; Sankar et al., 2008), and the thalamus (Dreifuss et al., 2001; Natsume et al., 2003; Bernhardt et al., 2012). In the hippocampus and thalamus, surface shape mapping has furthermore allowed localizing structural anomalies at a subregional level (Hogan et al., 2004; Kim et al., 2008; Bernhardt et al., 2012, in press). In the thalamus, for example, we found volume loss located primarily in mediodorsal segments (Bernhardt et al., 2012). Quantitative MRI postprocessing techniques, such as voxel-based morphometry (Bernasconi et al., 2004; Bonilha et al., 2004; Keller and Roberts, 2008) and analyses of cortical thickness have shown that TLE is associated with extensive regional neocortical abnormalities, encompassing not only mesiotemporal structures, but also prefrontal, fronto-central, cingulate, occipito-temporal, and lateral temporal neocortices (Lin et al., 2007; Bernhardt et al., 2008, 2009, 2010, 2012; Mcdonald et al., 2008c; Mueller et al., 2009b; Kemmotsu et al., 2011; Voets et al., 2011). Although the exact biological underpinnings of gray matter loss in different brain regions are not clear, they likely reflect a combination of neuronal loss and synaptic reorganization (Cascino et al., 1991; Sanabria et al., 2002; Blanc et al., 2011), possibly secondary to seizures (Sutula et al., 1988; Holmes, 2002; Cavazos et al., 2003). These findings have increased our understanding of whole-brain pathology associated with TLE. On the other hand, new techniques such as hippocampal and thalamic surface-shape mapping (Kim et al., 2008; Bernhardt et al., 2012) have allowed searching for fine-grained, subregional structural anomalies within temporo-limbic seizure networks. Importantly, the MRI-derived knowledge of pathology is in overall agreement with data from animal models and ex vivo studies. These studies collectively support the concept of TLE as a disorder of distributed neural networks.
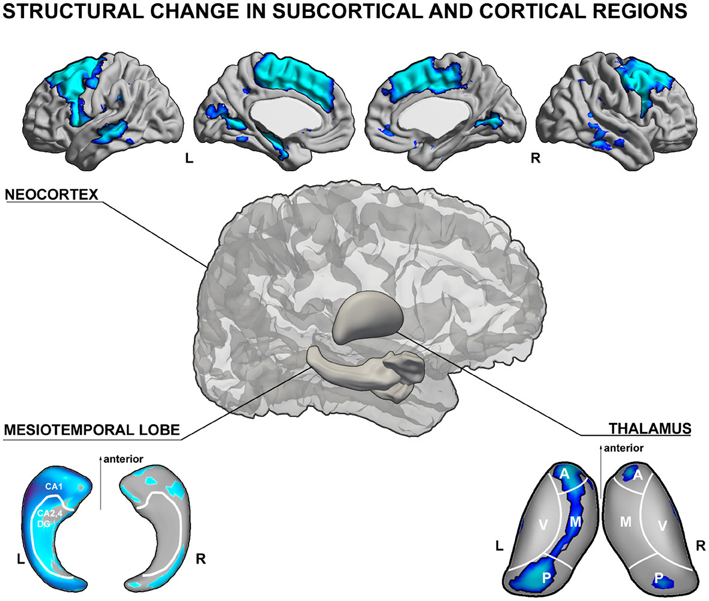
Figure 1. Schematic illustration of gray matter structural anomalies in temporal lobe epilepsy. (top) Results from MRI-based cortical thickness analysis, showing cortical thinning in left temporal lobe epilepsy (TLE) patients with hippocampal atrophy relative to healthy controls in mesial and lateral temporal as well as fronto-central neocortices (Bernhardt et al., 2010). (lower left) Patterns of atrophy in TLE patients relative to controls in CA1 subregions of the ipsilateral hippocampus (Kim et al., 2008) and (lower right) mediodorsal segments of the thalamus (Bernhardt et al., 2012), both generated using spherical harmonic surface-shape modeling techniques of manual MRI segmentations. The shown analyses have been generated using the SurfStat toolbox for Matlab (Worsley et al., 2009). Further details on the statistical procedures can be found in the original publications.
Structural Networks
Quantitative structural studies have provided a comprehensive mapping of structural pathology in TLE. Nevertheless, the commonly applied mass-univariate group comparisons provide only a snapshot of putative network abnormalities in TLE. Indeed, while such topographic maps may localize an ensemble of affected regions, they do not directly address how these regions inter-relate.
The term structural connectivity refers to anatomical associations between brain regions, defining the actual physical wiring (Stephan et al., 2000; Stone and Kotter, 2002; Sporns et al., 2005; Sporns, 2011). The gold standard to define such connections has been anterograde and retrograde tract-tracing techniques. Tracers show good accuracy and sensitivity, in particular for mapping long-range connections, and have resulted in a rich and detailed cartography of connectivity in several mammalian species (Felleman and Van Essen, 1991; Scannell et al., 1995; Modha and Singh, 2010). Their invasiveness however, limits their application to animal studies (Sporns, 2011).
In humans, two major indirect approaches have been employed to map structural networks: diffusion MRI tractography and structural MRI covariance (see Figures 2A–D). Structural networks derived from diffusion-weighted MRI data provide an approximation of the underlying white matter architecture (Le Bihan et al., 1986, 1996; Johansen-Berg and Behrens, 2006; Jbabdi and Johansen-Berg, 2011) by describing the directionality and magnitude of water diffusion at each imaging voxel. These data can be further processed by tractography algorithms (Mori et al., 1999; Behrens et al., 2003), which reconstruct fiber pathways running along plausible diffusion trajectories in voxel-space (Figure 2A). While somewhat challenged in regions where different fiber populations intersect (Behrens et al., 2003; Jones et al., 2012), such as the cortical gray matter, tractography can generate consistent results, particularly in deep white matter. Findings have shown overall a good correspondence with the animal tracing literature, and have been cross-validated by comparative sacrificial tracing studies in non-human primates (Mori et al., 1999; Parker et al., 2002; Dauguet et al., 2007). Moreover, it has been shown that factors such as fiber diameter and density, membrane permeability, myelination, as well as fiber packing (Beaulieu, 2002; Concha et al., 2010) can influence the directionality and magnitude of water displacement at a given voxel. Diffusion imaging may, thus, be used to assess microstructural and architectural integrity in vivo. The most widely used diffusion tensor parameters are fractional anisotropy (FA), an index of deviation of water diffusion from a random spherical displacement, and mean diffusion (MD), a scalar marker of bulk diffusion at each voxel.
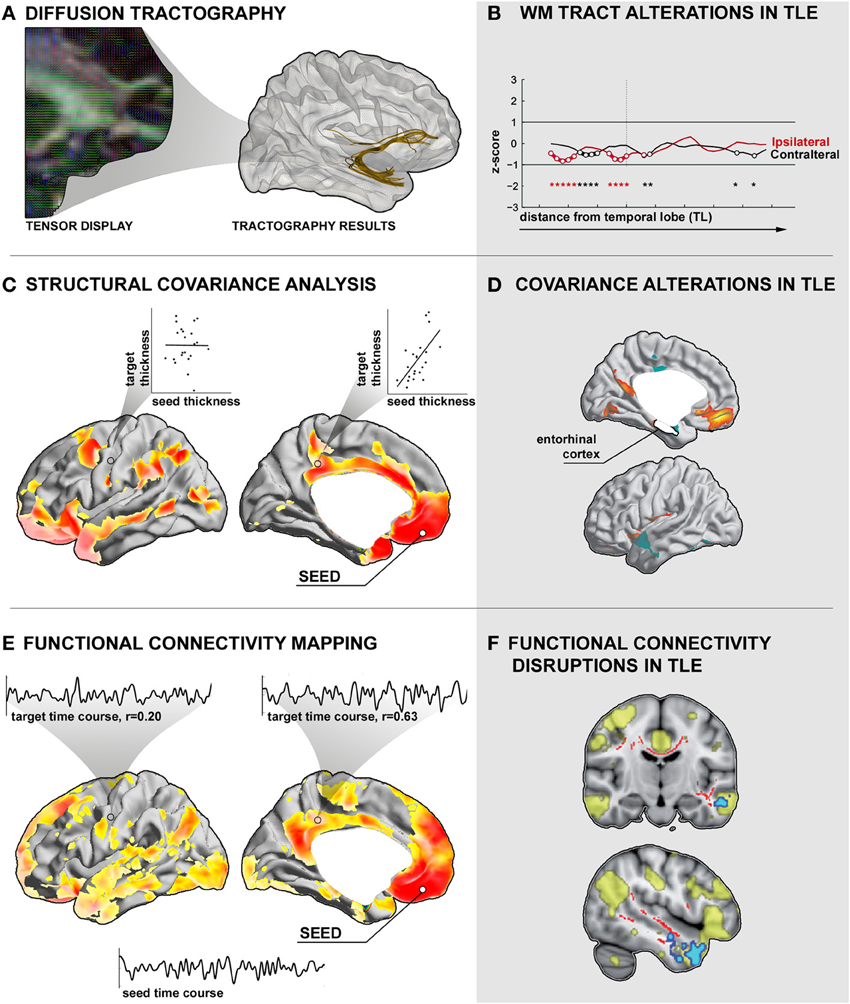
Figure 2. Assessment of inter-regional connectivity. (A) Diffusion tractography. Left: Illustration of diffusion tensor directions superimposed on a fractional anisotropy map derived from diffusion MRI. Right: Seed-based deterministic tractography of the uncinate fasciculus. (B) Altered mean diffusivity along the uncinate fasciculus tract in a group of patients with temporal lobe epilepsy (TLE) relative to controls (Concha et al., 2012). Prior to analysis, the tract was subdivided into bins with respect to the anatomical distance to the temporal and frontal lobes. (C) Structural covariance analysis. Shown is the cortical thickness correlation map of the left medial orbital cortex seed with the remaining cortical mantle in a group of healthy controls. High positive correlations are interpreted as connections, low correlations as absence of connections. (D) Structural covariance alterations in TLE patients relative to controls between an entorhinal cortex seed and target regions in medial orbitofrontal cortices (Bernhardt et al., 2008). (E) Functional connectivity between a left medial orbitofrontal cortex seed and the rest of the cortical mantle in healthy controls. Insets show exemplary time courses of the seed region with selected cortical target regions with high and low correlations, respectively. (F) Voxel-wise functional connectivity abnormalities in TLE, highlighting target regions with altered time-series correlation to a spatial component that closely matches the “default mode” network (Voets et al., 2012).
In TLE, previous diffusion MRI studies have consistently shown decreased FA in temporo-limbic tracts such as the fornix pathway (Concha et al., 2005; Ahmadi et al., 2009), parahippocampal fibers (Mcdonald et al., 2008a; Yogarajah and Duncan, 2008; Ahmadi et al., 2009), the uncinate fasciculus (Rodrigo et al., 2007; Diehl et al., 2008; Lin et al., 2008; Mcdonald et al., 2008a), and the cingulum bundle (Concha et al., 2008; Ahmadi et al., 2009), as well as in several frontal and posterior fiber tracts including the inferior and superior longitudinal fascicles (Focke et al., 2008; Lin et al., 2008; Mcdonald et al., 2008a; Ahmadi et al., 2009), the internal and external capsule (Arfanakis et al., 2002; Gross et al., 2006; Concha et al., 2008), and the corpus callosum (Arfanakis et al., 2002; Gross et al., 2006; Concha et al., 2008). Relative to the widespread pattern of FA changes, MD anomalies follow a more restricted distribution (Concha et al., 2005, 2008; Focke et al., 2008). In a recent study that assessed diffusion abnormalities along fiber tracts, our group could show that the effect size of MD alterations in TLE seems to decrease as a function of anatomical distance to the temporal lobe (Figure 2B), suggesting co-localization of these changes with the seizure focus (Concha et al., 2012).
The combined contribution of different microstructural and architectural properties to the diffusion signal precludes a straightforward, and universal biological interpretation of diffusion tensor indices and their alteration in disease (Jones et al., 2012). Diffusion MRI is, nevertheless, currently the only imaging method that can assess fiber architecture in vivo (Jones et al., 2012). Initial evidence from histopathological analysis of the fimbria-fornix pathways in operated TLE patients suggests that FA decreases may primarily reflect alterations in axonal membranes (Concha et al., 2010). MD changes, on the other hand, have been shown to vary with respect to the dynamics of seizure activity (Yu and Tan, 2008; Concha et al., 2012). Indeed, MD has been shown to decrease in the hyperacute phase after prolonged seizures or status epilepticus, likely due to intracellular cytotoxic edema. Conversely, few days following the subacute peri-ictal phase, MD may increase as a consequence of vasogenic edema (Scott et al., 2006). Neuronal loss and gliosis can lead to further MD increase as a consequence of the chronic expansion of the interstitial water content.
Structural networks may also be studied through covariance analysis of MRI-based morphological metrics, such as cortical thickness or gray matter volume (Bullmore et al., 1998; Mechelli et al., 2005; Lerch et al., 2006; Bernhardt et al., 2008, 2013). According to the framework of MRI covariance analysis, a high correlation in morphological markers between two regions across subjects can be interpreted as a network link, while a low correlation indicates no link (Figure 2C). Similar to diffusion tractography, this correlational framework does not infer direct anatomical connections between pairs of regions. Nonetheless, analyzing structural covariance may detect manifestations of persistent functional-trophic cross-talk, maturational inter-change, as well as common developmental and pathological influences (Lerch et al., 2006; Bullmore and Sporns, 2009; Zielinski et al., 2010; Bernhardt et al., 2011; Raznahan et al., 2011; Xia and He, 2011; Khundrakpam et al., 2012; Alexander-Bloch et al., 2013). One of the advantages of cortical thickness covariance analysis is the direct seeding from cortical gray matter regions in a high-resolution space that is in principle not limited by the imaging voxels of the underlying MR image, but by the sampling density of the points on the cortical mesh. Correlation analysis of structural features may furthermore represent a relatively pragmatic approach toward structural network mapping. In fact, the commonly used T1-weighted images, a standard component of every clinical imaging protocol, have a short acquisition time. Moreover, these images are generally unaffected by distortion and signal dropout artifacts in orbitofrontal and temporo-basal regions often occurring in echo-planar functional and diffusion MRI sequences.
In TLE, several recent covariance analyses have mapped abnormal structural correlations between mesiotemporal and neocortical regions (Bonilha et al., 2007a; Bernhardt et al., 2008; Mueller et al., 2009b), thalamic and neocortical regions (Mueller et al., 2009a; Bernhardt et al., 2012), and within cortico-cortical networks (Mueller et al., 2009a). Correlating the thickness of the entorhinal cortex to that of the neocortex, our group found decreased structural coordination between mesial temporal regions and lateral temporal neocortices, suggestive of a connectional breakdown within temporo-limbic circuits (Figure 2D; Bernhardt et al., 2008). Moreover, covariance analysis of thalamo-cortical circuits (Hetherington et al., 2007; Mueller et al., 2009a; Bernhardt et al., 2012) has shown coupled structural and metabolic change of the thalamus with neocortical (Bernhardt et al., 2012) and with mesiotemporal regions (Hetherington et al., 2007; Mueller et al., 2009a), emphasizing a key role of this structure in the pathological network of TLE.
Diffusion MRI and structural MRI covariance analysis tap into different facets of structural brain networks. While diffusion MRI analysis may be the method of choice to study white matter tracts, and their potential architectural disruptions, structural covariance analysis may sensitively assess alterations in the trophic-morphological coordination between gray matter regions. Both approaches have advanced our understanding of the fundamental architecture of inter-regional connections, and their disruptions in TLE.
Functional Networks
The study of functional networks helps to elucidate how a structural architecture gives rise to alterations in neurophysiological dynamics. The term functional connectivity refers to the strength of statistical dependencies of neurophysiological signals between regions (Figure 2E).
Functional connectivity can be determined from time-series measured by functional MRI (Friston et al., 1993, 1996; Focke et al., 2008; Smith, 2012) or electrophysiological techniques, such as electroencephalography (EEG) (Lopes Da Silva et al., 1989; Tononi et al., 1994; Lachaux et al., 1999). Although functional MRI and EEG have complimentary temporal/spatial resolution tradeoffs, they can also be combined (Lemieux et al., 2011). In short, functional MRI does not directly measure neural activity, but only activity-dependent hemodynamic alterations, and has a relatively low temporal resolution in the range of 1–2 s [but see Feinberg et al. (2010); Smith et al. (2012), for a recent example of increasing the temporal resolution in functional MRI acquisitions]. Yet, this technique offers high spatial resolution in the millimeter range and allows imaging the entire brain (Lemieux et al., 2011). EEG, on the other hand, has a superior temporal resolution (in the order of milliseconds) but suffers from neurophysiological signals limited to the scalp.
One way to assess functional connectivity between different brain regions is through analysis of task-free (or, resting-state) paradigms, functional acquisitions during which the subject does not perform any task (Biswal et al., 1995, 2010; Greicius et al., 2003; Smith et al., 2009). Functional connectivity analysis of such task-free datasets has allowed the identification of brain networks that show strong coupling of intrinsic, spontaneous brain activity. Ample recent resting-state functional MRI assessments have revealed networks which are generally reproducible across subjects (Damoiseaux et al., 2006) that closely correspond to brain systems engaging in specific tasks (Biswal et al., 1995; Smith et al., 2009; Laird et al., 2011). Several studies have furthermore begun to explore the relationship between low-frequency resting-state networks derived from functional MRI and those measured from EEG (De Pasquale et al., 2010; Jann et al., 2010; Musso et al., 2010; Yuan et al., 2012). Moreover, several studies in primates have suggested a close correspondence between intrinsic functional MRI connections and known anatomical pathways (Mantini et al., 2011; Shen et al., 2012). In turn, other studies have demonstrated the utility of resting-state patterns to generate regional parcellations of specific anatomical areas (Margulies et al., 2007; Mars et al., 2011). Finally, analysis of resting-state connectivity patterns may be sensitive to detect disruptions of brain organization in disease conditions (Greicius, 2008; Fox and Greicius, 2010; Kelly et al., 2012).
Several EEG and combined EEG-fMRI studies have shown dynamic alterations in functional activations and connectivity patterns related to epileptic spikes (Gotman et al., 2006; Kobayashi et al., 2006; Laufs et al., 2007; Ponten et al., 2007; Bettus et al., 2011). Resting-state functional EEG and functional MRI connectivity analyses in TLE have also quantified chronic, inter-ictal changes in functional networks (Waites et al., 2006; Bettus et al., 2009). These studies have mainly focused on assessing associations of intrinsic signals between regions known to be involved in seizure activity, particularly among medial temporal lobe structures. Bettus and colleagues reported decreased functional connectivity in mesiotemporal regions proximal to the seizure focus; interestingly, ipsilateral decreases co-occurred with increased functional connectivity in contralateral regions (Bettus et al., 2009). Findings of contralateral connectivity increases are suggestive of compensatory network reorganization. Several studies have also suggested functional connectivity alterations in regions that comprise the “default mode” network (Raichle et al., 2001; Greicius et al., 2003; Voets et al., 2012), or between this network and other brain regions (Frings et al., 2009; Liao et al., 2010; Zhang et al., 2010). The default mode network includes a collection of medial frontal, midline parietal, and lateral parietal regions that show increased activation in the absence of a specific tasks, and whose function may closely relate to internal thought processes such as memory and mind-wandering (Buckner and Carroll, 2007; Christoff et al., 2009).
Changes in inter-regional functional coupling are thought to represent compensatory mechanisms secondary to structural pathology and seizure-related activity. Combining structural and functional image analysis, for example, our group recently showed disruptions in functional connectivity between mesiotemporal regions and neocortical target networks (Figure 2F) that may relate to abnormal gray matter density and altered diffusivity of inter-connecting fiber tracts (Voets et al., 2012) suggestive of a complex derangement in the structural-functional cross-links. There is also evidence for abnormal signal interactions in epileptic patients without a visible lesion on MRI (Vlooswijk et al., 2011). In addition, functional abnormalities have even been shown in regions unaffected by epileptic discharges (Bettus et al., 2011), suggestive of a widespread pathological process that alter the whole-brain intrinsic functional network architecture in TLE.
Graph Theory—Modeling Network Topology
Conventional analysis approaches, mainly based on between-group comparisons have shown low-level regional and connectional alterations in TLE. These methods, however, are not tailored at capturing the complexity of whole-brain pathological interactions in TLE, which may affect higher-order, topological aspects of brain network organization.
Graph theory is a framework for the mathematical representation and analysis of complex systems. It has been applied to the analysis of artificial and biological networks (Watts and Strogatz, 1998). Graph theoretical analysis has recently attracted considerable attention in brain research because it provides a powerful formalism to quantitatively describe the topological organization of connectivity (Bullmore and Sporns, 2009; Guye et al., 2010; Bassett and Gazzaniga, 2011; Bullmore and Bassett, 2011; Alexander-Bloch et al., 2013). In graph theory terms, a network is a collection of nodes that are interconnected by edges. Nodes usually represent brain regions, while edges represent (structural or functional) connections. A pre-requisite to connectivity analysis is the proper designation of nodes as distinct gray matter regions. Various parcellation schemes have been proposed, including approximating Brodmann areas based on imaging-derived surrogates of myelination (Glasser and Van Essen, 2011; Bock et al., 2013), sulcation-based atlases (Van Essen, 2005; Desikan et al., 2006), high-resolution parcellations (Hagmann et al., 2008; Honey et al., 2009), as well as schemes that take the imaging voxels/vertices themselves as nodes (Lohmann et al., 2010; Tomasi and Volkow, 2011). In addition, several studies have also used data-driven techniques such as independent brain components to define network nodes (Yu et al., 2011b, 2013). Nodal definitions have shown to have a large influence on graph-theoretical parameters (Tohka et al., 2012), and the definition of reliable, biological meaningful parcellations schemes continues to be an active area of current research (Geyer et al., 2011; Glasser and Van Essen, 2011; Van Essen et al., 2012).
As described in previous sections, in vivo studies provide several definitions for network edges, both in the structural and functional domain (see Figure 3, for an example of functional and structural network generation). Accordingly, graph-theoretical analysis has been conducted across various modalities such as functional MRI (Salvador et al., 2005; He et al., 2009; Honey et al., 2009), electrophysiology (Stam, 2004; Bassett et al., 2006), diffusion-weighted MRI (Hagmann et al., 2007, 2008; Iturria-Medina et al., 2007; Gong et al., 2009), and structural covariance (He et al., 2007; Bassett et al., 2008; Chen et al., 2008; Bernhardt et al., 2011). Collectively, these studies have shown that the global topology of brain networks in healthy populations is neither random nor regular, but characteristic of a small-world. Small-world networks are defined by clusters of tightly inter-connected nodes, which are themselves linked to other clusters through few inter-connector links. This architecture results in overall short path lengths between individual nodes and an overall high degree of clustering (Watts and Strogatz, 1998), an architecture that enables both the specialization and integration of information transfer at relatively low wiring costs (Sporns et al., 2004). Graph-theoretical methods may be used to examine intermediary levels of organization. Communities (also called modules) are groups of nodes that are richly connected to one another within the larger framework of the entire network (Meunier et al., 2010; Bullmore and Bassett, 2011). Modularity is one of the most ubiquitous properties of complex, large-scale networks (Bullmore and Sporns, 2009), and modules may express some degree of hierarchical organization (Bassett et al., 2008; Meunier et al., 2010; Bullmore and Bassett, 2011). Theoretically, there are several advantages to a modular and hierarchical organization, including greater adaptability and robustness to changing environmental conditions (Meunier et al., 2010). Moreover, it has been suggested that the more modular and hierarchically organized a system is, the more diverse its functional activation patterns (Alexander-Bloch et al., 2010; Kaiser and Hilgetag, 2010). Modularity may be undermined by disease processes, as suggested by disrupted modularity in schizophrenia (Bassett et al., 2008; Alexander-Bloch et al., 2010; Yu et al., 2011a), and frontal lobe epilepsy (Vaessen et al., 2012a).
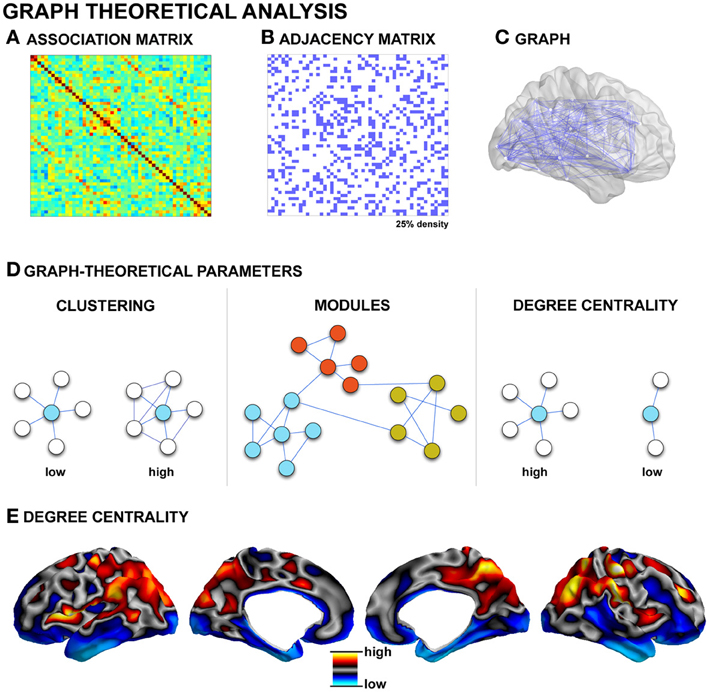
Figure 3. Graph-theoretical analysis. (A) Association matrix quantifying the degree of connectivity (derived from techniques such as diffusion MRI tractography, structural covariance, or functional connectivity, see Figure 2). (B) Matrices commonly undergo a thresholding procedure to remove spurious edges. In this example, the highest 25% of associations are preserved, leading to a binary adjacency matrix. (C) Each binary matrix is equivalent to an undirected graph. (D) Topological parameters such as the clustering coefficient and path length can then be measured; networks can be partitioned in modules based on groupings of connectivity among nodes; hubs can be identified, for example, as nodes with high degree centrality (i.e., a high number of connections). (E) Cortical degree centrality map, based on resting-state functional connectivity data from a single healthy control subject.
Besides the characterization of global and modular properties of large-scale networks, graph-theoretical techniques allow the localization of key regions within the network layout, so-called hubs, through centrality-based metrics (Bullmore and Sporns, 2009; Van Den Heuvel and Sporns, 2011; Zuo et al., 2012). According to formulations of centrality, hubs can be defined as regions with a high degree centrality, which means that they have a high number of connections to other nodes (Zuo et al., 2012); they can be identified on the basis of high betweeness centrality, which signifies they are located along pathways of efficient information flow (Zuo et al., 2012); finally, they can be identified through a high eigenvector centrality, which is a recursive formulation quantifying connections to other highly connected hubs (Lohmann et al., 2010; Zuo et al., 2012). Depending on their embedding in specific modules and connectivity profiles, hubs can be further classified as to whether they primarily mediate within- or between-module connectivity (Sporns et al., 2007). Assessing hubs promises to highlight critical key regions in structural and functional networks, and may thus provide a better understanding of their potential role in pathological processes (Bullmore and Sporns, 2012). In Alzheimer's disease, for example, functional hubs coincide with regions of high amyloid-beta deposition (Buckner et al., 2009). Central hubs may form a so-called rich club, a collection of mutually densely linked nodes with disproportionally high centrality (Van Den Heuvel and Sporns, 2011; Harriger et al., 2012). This architecture is thought to contribute to the robustness of the core constituents of the brain network. In humans, a rich club inferred from diffusion MRI has been shown to comprise lateral prefrontal, midline and lateral parietal, as well as the hippocampus, putamen, and thalamus (Van Den Heuvel and Sporns, 2011). In the macaque monkey, rich club regions have been shown to be preferentially located on short paths through the network, thereby contributing effectively to global communication (Harriger et al., 2012).
In focal epilepsy, relatively few studies have employed graph-theoretical analysis of brain networks derived from MRI (Liao et al., 2010; Bernhardt et al., 2011; Bonilha et al., 2012; Vaessen et al., 2012b). We previously showed that in drug-resistant TLE, structural networks derived from inter-regional MRI-based cortical thickness correlations are characterized by increased clustering and path length, a finding indicative of a more regular global topology (Bernhardt et al., 2011). These findings were complemented by the parallel observation of reduced network robustness, a measure of organizational stability (Bernhardt et al., 2011), and subtle alterations in the distribution of network hubs pointing toward a more paralimbic distribution in patients relative to controls. In a longitudinal study, we showed that structural network disruptions intensify over time. Furthermore, relating network parameters to postsurgical seizure outcome data indicated that patients who continued to have seizures after surgery had more marked network disruptions relative to those who became seizure-free (Bernhardt et al., 2011). These findings speak to the hypothesis that seizure recurrence after surgery may, in part, be related to an extended epileptogenic network (Ryvlin, 2003; Bernhardt et al., 2010). Our findings therefore suggest a possible clinical potential for network data in the presurgical workup.
Our finding of a more regularized topology of structural cortico-cortical networks in drug-resistant TLE closely resembled results from graph-theoretical analyses of intracerebral EEG recordings during focal seizures (Ponten et al., 2007; Kramer et al., 2008; Schindler et al., 2008) and scalp EEG data of generalized absence seizures (Ponten et al., 2009). Indeed, electrophysiological work has suggested that seizures may be associated with a sudden regularization of functional networks. It may, thus, be plausible that network synchronization during the ictal phase may influence mutual inter-cortical trophic exchanges, ultimately leading to progressive and long-lasting remodeling of inter-regional structural networks.
Few other studies assessed the topology of brain networks derived from MRI in TLE using graph-theoretical methods. A diffusion MRI tractography of structures belonging to the limbic network showed increased clustering and centrality in 12 patients with unilateral TLE (Bonilha et al., 2012). Analysis of whole-brain resting-state functional networks in patients with bilateral TLE has revealed both atypical low-level connectivity (i.e., increases in temporal and decreases in fronto-parietal connectivity) and topological disruptions, indicative of decreased clustering and path length (Liao et al., 2010). These studies have further demonstrated that TLE is associated with topological disruptions in large-scale structural and functional networks. Results have nevertheless been somewhat divergent, possibly as a result of heterogeneous patient populations and diverse network construction methods.
Conclusions and Future Directions
Advances in brain network construction and graph theoretical modeling have permitted a characterization of topological aspects of healthy and abnormal brain connectivity. While an extensive literature has shown regional as well as low-level connectional disruptions in TLE, studies focusing on disruptions of network topology have been so far rather sparse. The unified and elegant framework of graph theoretical analysis promises to further consolidate our understanding of how functional networks interact with their structural substrate. Moreover, given initial observations of a relationship between the extent of network damage and post-surgical seizure outcome, establishing subject-specific profiles of network properties has great potential to assist in clinical decision-making.
Conflict of Interest Statement
The authors declare that the research was conducted in the absence of any commercial or financial relationships that could be construed as a potential conflict of interest.
References
Ahmadi, M. E., Hagler, D. J. Jr., Mcdonald, C. R., Tecoma, E. S., Iragui, V. J., Dale, A.M., et al. (2009). Side matters: diffusion tensor imaging tractography in left and right temporal lobe epilepsy. AJNR. Am. J. Neuroradiol. 30, 1740–1747. doi: 10.3174/ajnr.A1650
Alexander-Bloch, A., Giedd, J. N., and Bullmore, E. (2013). Imaging structural co-variance between human brain regions. Nat. Rev. Neurosci. 14, 322–336. doi: 10.1038/nrn3465
Alexander-Bloch, A. F., Gogtay, N., Meunier, D., Birn, R., Clasen, L., Lalonde, F., et al. (2010). Disrupted modularity and local connectivity of brain functional networks in childhood-onset schizophrenia. Front. Syst. Neurosci. 4:147. doi: 10.3389/fnsys.2010.00147
Arfanakis, K., Hermann, B. P., Rogers, B. P., Carew, J. D., Seidenberg, M., and Meyerand, M. E. (2002). Diffusion tensor MRI in temporal lobe epilepsy. Magn. Reson. Imaging 20, 511–519. doi: 10.1016/S0730-725X(02)00509-X
Avoli, M., and Gloor, P. (1982). Interaction of cortex and thalamus in spike and wave discharges of feline generalized penicillin epilepsy. Exp. Neurol. 76, 196–217. doi: 10.1016/0014-4886(82)90112-1
Babb, T. L., and Brown, W. J. (1987). “Pathological findings in epilepsy,” in Surgical Treatment of the Epilepsies, ed J. Engel Jr. (New York, NY: Raven), 511–540.
Babb, T. L., Kupfer, W. R., Pretorius, J. K., Crandall, P. H., and Levesque, M. F. (1991). Synaptic reorganization by mossy fibers in human epileptic fascia dentata. Neuroscience 42, 351–363. doi: 10.1016/0306-4522(91)90380-7
Bassett, D. S., Bullmore, E., Verchinski, B. A., Mattay, V. S., Weinberger, D. R., and Meyer-Lindenberg, A. (2008). Hierarchical organization of human cortical networks in health and schizophrenia. J. Neurosci. 28, 9239–9248. doi: 10.1523/JNEUROSCI.1929-08.2008
Bassett, D. S., and Gazzaniga, M. S. (2011). Understanding complexity in the human brain. Trends Cogn. Sci. 15, 200–209. doi: 10.1016/j.tics.2011.03.006
Bassett, D. S., Meyer-Lindenberg, A., Achard, S., Duke, T., and Bullmore, E. (2006). Adaptive reconfiguration of fractal small-world human brain functional networks. Proc. Natl. Acad. Sci. U.S.A. 103, 19518–19523. doi: 10.1073/pnas.0606005103
Bear, J., Fountain, N. B., and Lothman, E. W. (1996). Responses of the superficial entorhinal cortex in vitro in slices from naive and chronically epileptic rats. J. Neurophysiol. 76, 2928–2940.
Beaulieu, C. (2002). The basis of anisotropic water diffusion in the nervous system - a technical review. NMR Biomed. 15, 435–455. doi: 10.1002/nbm.782
Behrens, T. E., Johansen-Berg, H., Woolrich, M. W., Smith, S. M., Wheeler-Kingshott, C. A., Boulby, P. A., et al. (2003). Non-invasive mapping of connections between human thalamus and cortex using diffusion imaging. Nat. Neurosci. 6, 750–757. doi: 10.1038/nn1075
Bernasconi, N., Andermann, F., Arnold, D. L., and Bernasconi, A. (2003a). Entorhinal cortex MRI assessment in temporal, extratemporal, and idiopathic generalized epilepsy. Epilepsia 44, 1070–1074. doi: 10.1046/j.1528-1157.2003.64802.x
Bernasconi, N., Bernasconi, A., Caramanos, Z., Antel, S. B., Andermann, F., and Arnold, D. L. (2003b). Mesial temporal damage in temporal lobe epilepsy: a volumetric MRI study of the hippocampus, amygdala and parahippocampal region. Brain 126, 462–469. doi: 10.1093/brain/awg034
Bernasconi, N., Bernasconi, A., Caramanos, Z., Dubeau, F., Richardson, J., Andermann, F., et al. (2001). Entorhinal cortex atrophy in epilepsy patients exhibiting normal hippocampal volumes. Neurology 56, 1335–1339. doi: 10.1212/WNL.56.10.1335
Bernasconi, N., Duchesne, S., Janke, A., Lerch, J., Collins, D. L., and Bernasconi, A. (2004). Whole-brain voxel-based statistical analysis of gray matter and white matter in temporal lobe epilepsy. Neuroimage 23, 717–723. doi: 10.1016/j.neuroimage.2004.06.015
Bernhardt, B. C., Bernasconi, N., Concha, L., and Bernasconi, A. (2010). Cortical thickness analysis in temporal lobe epilepsy: reproducibility and relation to outcome. Neurology 74, 1776–1784. doi: 10.1212/WNL.0b013e3181e0f80a
Bernhardt, B. C., Bernasconi, N., Kim, H., and Bernasconi, A. (2012). Mapping thalamocortical network pathology in temporal lobe epilepsy. Neurology 78, 129–136. doi: 10.1212/WNL.0b013e31823efd0d
Bernhardt, B. C., Chen, Z., He, Y., Evans, A. C., and Bernasconi, N. (2011). Graph-theoretical analysis reveals disrupted small-world organization of cortical thickness correlation networks in temporal lobe epilepsy. Cereb. Cortex 21, 2147–2157. doi: 10.1093/cercor/bhq291
Bernhardt, B. C., Klimecki, O. M., Leiberg, S., and Singer, T. (2013). Structural covariance networks of dorsal anterior insula predict females' individual differences in empathic responding. Cereb. Cortex doi: 10.1093/cercor/bht072. [Epub ahead of print].
Bernhardt, B. C., Kim, H., and Bernasconi, N. (in press). Patterns of subregional disease progression in temporal lobe epilepsy. Neurology.
Bernhardt, B. C., Worsley, K. J., Besson, P., Concha, L., Lerch, J. P., Evans, A. C., et al. (2008). Mapping limbic network organization in temporal lobe epilepsy using morphometric correlations: insights on the relation between mesiotemporal connectivity and cortical atrophy. Neuroimage 42, 515–524. doi: 10.1016/j.neuroimage.2008.04.261
Bernhardt, B. C., Worsley, K. J., Kim, H., Evans, A. C., Bernasconi, A., and Bernasconi, N. (2009). Longitudinal and cross-sectional analysis of atrophy in pharmacoresistant temporal lobe epilepsy. Neurology 72, 1747–1754. doi: 10.1212/01.wnl.0000345969.57574.f5
Bertram, E. H. (1997). Functional anatomy of spontaneous seizures in a rat model of limbic epilepsy. Epilepsia 38, 95–105. doi: 10.1111/j.1528-1157.1997.tb01083.x
Bertram, E. H., Mangan, P. S., Zhang, D., Scott, C. A., and Williamson, J. M. (2001). The midline thalamus: alterations and a potential role in limbic epilepsy. Epilepsia 42, 967–978. doi: 10.1046/j.1528-1157.2001.042008967.x
Bettus, G., Guedj, E., Joyeux, F., Confort-Gouny, S., Soulier, E., Laguitton, V., et al. (2009). Decreased basal fMRI functional connectivity in epileptogenic networks and contralateral compensatory mechanisms. Hum. Brain Mapp. 30, 1580–1591. doi: 10.1002/hbm.20625
Bettus, G., Ranjeva, J. P., Wendling, F., Benar, C. G., Confort-Gouny, S., Regis, J., et al. (2011). Interictal functional connectivity of human epileptic networks assessed by intracerebral EEG and BOLD signal fluctuations. PLoS ONE 6:e20071. doi: 10.1371/journal.pone.0020071
Biswal, B., Yetkin, F. Z., Haughton, V. M., and Hyde, J. S. (1995). Functional connectivity in the motor cortex of resting human brain using echo-planar MRI. Magn. Reson. Med. 34, 537–541. doi: 10.1002/mrm.1910340409
Biswal, B. B., Mennes, M., Zuo, X. N., Gohel, S., Kelly, C., Smith, S. M., et al. (2010). Toward discovery science of human brain function. Proc. Natl. Acad. Sci. U.S.A. 107, 4734–4739. doi: 10.1073/pnas.0911855107
Blanc, F., Martinian, L., Liagkouras, I., Catarino, C., Sisodiya, S. M., and Thom, M. (2011). Investigation of widespread neocortical pathology associated with hippocampal sclerosis in epilepsy: a postmortem study. Epilepsia 52, 10–21. doi: 10.1111/j.1528-1167.2010.02773.x
Blumcke, I., Thom, M., and Wiestler, O. D. (2002). Ammon's horn sclerosis: a maldevelopmental disorder associated with temporal lobe epilepsy. Brain Pathol. 12, 199–211.
Bock, N. A., Hashim, E., Janik, R., Konyer, N. B., Weiss, M., Stanisz, G. J., et al. (2013). Optimizing T(1)-weighted imaging of cortical myelin content at 3.0T. Neuroimage 65, 1–12. doi: 10.1016/j.neuroimage.2012.09.051
Bonilha, L., Nesland, T., Martz, G. U., Joseph, J. E., Spampinato, M. V., Edwards, J. C., et al. (2012). Medial temporal lobe epilepsy is associated with neuronal fibre loss and paradoxical increase in structural connectivity of limbic structures. J. Neurol. Neurosurg. Psychiatr. 83, 903–909. doi: 10.1136/jnnp-2012-302476
Bonilha, L., Rorden, C., Castellano, G., Pereira, F., Rio, P. A., Cendes, F., et al. (2004). Voxel-based morphometry reveals gray matter network atrophy in refractory medial temporal lobe epilepsy. Arch. Neurol. 61, 1379–1384. doi: 10.1001/archneur.61.9.1379
Bonilha, L., Rorden, C., Halford, J. J., Eckert, M., Appenzeller, S., Cendes, F., et al. (2007a). Asymmetrical extra-hippocampal grey matter loss related to hippocampal atrophy in patients with medial temporal lobe epilepsy. J. Neurol. Neurosurg. Psychiatr. 78, 286–294. doi: 10.1136/jnnp.2006.103994
Bonilha, L., Yasuda, C. L., Rorden, C., Li, L. M., Tedeschi, H., De Oliveira, E., et al. (2007b). Does resection of the medial temporal lobe improve the outcome of temporal lobe epilepsy surgery? Epilepsia 48, 571–578. doi: 10.1111/j.1528-1167.2006.00958.x
Bothwell, S., Meredith, G. E., Phillips, J., Staunton, H., Doherty, C., Grigorenko, E., et al. (2001). Neuronal hypertrophy in the neocortex of patients with temporal lobe epilepsy. J. Neurosci. 21, 4789–4800.
Buckner, R. L., and Carroll, D. C. (2007). Self-projection and the brain. Trends Cogn. Sci. 11, 49–57. doi: 10.1016/j.tics.2006.11.004
Buckner, R. L., Sepulcre, J., Talukdar, T., Krienen, F. M., Liu, H., Hedden, T., et al. (2009). Cortical hubs revealed by intrinsic functional connectivity: mapping, assessment of stability, and relation to Alzheimer's disease. J. Neurosci. 29, 1860–1873. doi: 10.1523/JNEUROSCI.5062-08.2009
Bullmore, E., and Sporns, O. (2009). Complex brain networks: graph theoretical analysis of structural and functional systems. Nat. Rev. Neurosci. 10, 186–198. doi: 10.1038/nrn2575
Bullmore, E., and Sporns, O. (2012). The economy of brain network organization. Nat. Rev. Neurosci. 13, 336–349. doi: 10.1038/nrn3214
Bullmore, E. T., and Bassett, D. S. (2011). Brain graphs: graphical models of the human brain connectome. Annu. Rev. Clin. Psychol. 7, 113–140. doi: 10.1146/annurev-clinpsy-040510-143934
Bullmore, E. T., Woodruff, P. W., Wright, I. C., Rabe-Hesketh, S., Howard, R. J., Shuriquie, N., et al. (1998). Does dysplasia cause anatomical dysconnectivity in schizophrenia? Schizophr. Res. 30, 127–135. doi: 10.1016/S0920-9964(97)00141-2
Cascino, G. D. (2009). Temporal lobe epilepsy is a progressive neurologic disorder: time means neurons! Neurology 72, 1718–1719. doi: 10.1212/WNL.0b013e3181a4e465
Cascino, G. D., Jack, C. R. Jr., Parisi, J. E., Sharbrough, F. W., Hirschorn, K. A., Meyer, F. B., et al. (1991). Magnetic resonance imaging-based volume studies in temporal lobe epilepsy: pathological correlations. Ann. Neurol. 30, 31–36. doi: 10.1002/ana.410300107
Cassidy, R. M., and Gale, K. (1998). Mediodorsal thalamus plays a critical role in the development of limbic motor seizures. J. Neurosci. 18, 9002–9009.
Cavalheiro, E. A., Leite, J. P., Bortolotto, Z. A., Turski, W. A., Ikonomidou, C., and Turski, L. (1991). Long-term effects of pilocarpine in rats: structural damage of the brain triggers kindling and spontaneous recurrent seizures. Epilepsia 32, 778–782. doi: 10.1111/j.1528-1157.1991.tb05533.x
Cavanagh, J. B., and Meyer, A. (1956). Aetiological aspects of Ammon's horn sclerosis associated with temporal lobe epilepsy. Br. Med. J. 2, 1403–1407. doi: 10.1136/bmj.2.5006.1403
Cavazos, J. E., Zhang, P., Qazi, R., and Sutula, T. P. (2003). Ultrastructural features of sprouted mossy fiber synapses in kindled and kainic acid-treated rats. J. Comp. Neurol. 458, 272–292. doi: 10.1002/cne.10581
Cendes, F., Andermann, F., Gloor, P., Evans, A., Jones-Gotman, M., Watson, C., et al. (1993a). MRI volumetric measurement of amygdala and hippocampus in temporal lobe epilepsy. Neurology 43, 719–725. doi: 10.1212/WNL.43.4.719
Cendes, F., Andermann, F., Gloor, P., Lopes-Cendes, I., Andermann, E., Melanson, D., et al. (1993b). Atrophy of mesial structures in patients with temporal lobe epilepsy: cause or consequence of repeated seizures? Ann. Neurol. 34, 795–801. doi: 10.1002/ana.410340607
Chen, Z. J., He, Y., Rosa-Neto, P., Germann, J., and Evans, A. C. (2008). Revealing modular architecture of human brain structural networks by using cortical thickness from MRI. Cereb. Cortex 18, 2374–2381. doi: 10.1093/cercor/bhn003
Choi, D., Na, D. G., Byun, H. S., Suh, Y. L., Kim, S. E., Ro, D. W., et al. (1999). White-matter change in mesial temporal sclerosis: correlation of MRI with PET, pathology, and clinical features. Epilepsia 40, 1634–1641. doi: 10.1111/j.1528-1157.1999.tb02050.x
Christoff, K., Gordon, A. M., Smallwood, J., Smith, R., and Schooler, J. W. (2009). Experience sampling during fMRI reveals default network and executive system contributions to mind wandering. Proc. Natl. Acad. Sci. U.S.A. 106, 8719–8724. doi: 10.1073/pnas.0900234106
Clifford, D. B., Olney, J. W., Maniotis, A., Collins, R. C., and Zorumski, C. F. (1987). The functional anatomy and pathology of lithium-pilocarpine and high- dose pilocarpine seizures. Neuroscience 23, 953–968. doi: 10.1016/0306-4522(87)90171-0
Coan, A. C., and Cendes, F. (2013). Epilepsy as progressive disorders: what is the evidence that can guide our clinical decisions and how can neuroimaging help? Epilepsy Behav. 26, 313–321. doi: 10.1016/j.yebeh.2012.09.027
Concha, L., Beaulieu, C., Collins, D. L., and Gross, D. W. (2008). White matter diffusion abnormalities in temporal lobe epilepsy with and without mesial temporal sclerosis. J. Neurol. Neurosurg. Psychiatr. 80, 312–319. doi: 10.1136/jnnp.2007.139287
Concha, L., Beaulieu, C., and Gross, D. W. (2005). Bilateral limbic diffusion abnormalities in unilateral temporal lobe epilepsy. Ann. Neurol. 57, 188–196. doi: 10.1002/ana.20334
Concha, L., Kim, H., Bernasconi, A., Bernhardt, B. C., and Bernasconi, N. (2012). Spatial patterns of water diffusion along white matter tracts in temporal lobe epilepsy. Neurology 79, 455–462. doi: 10.1212/WNL.0b013e31826170b6
Concha, L., Livy, D. J., Beaulieu, C., Wheatley, B. M., and Gross, D. W. (2010). In vivo diffusion tensor imaging and histopathology of the fimbria-fornix in temporal lobe epilepsy. J. Neurosci. 30, 996–1002. doi: 10.1523/JNEUROSCI.1619-09.2010
Damoiseaux, J. S., Rombouts, S. A., Barkhof, F., Scheltens, P., Stam, C. J., Smith, S. M., et al. (2006). Consistent resting-state networks across healthy subjects. Proc. Natl. Acad. Sci. U.S.A. 103, 13848–13853. doi: 10.1073/pnas.0601417103
Dauguet, J., Peled, S., Berezovskii, V., Delzescaux, T., Warfield, S. K., Born, R., et al. (2007). Comparison of fiber tracts derived from in vivo DTI tractography with 3D histological neural tract tracer reconstruction on a macaque brain. Neuroimage 37, 530–538. doi: 10.1016/j.neuroimage.2007.04.067
De Lanerolle, N. C., Kim, J. H., Robbins, R. J., and Spencer, D. D. (1989). Hippocampal interneuron loss and plasticity in human temporal lobe epilepsy. Brain Res. 495, 387–395. doi: 10.1016/0006-8993(89)90234-5
De Pasquale, F., Della Penna, S., Snyder, A. Z., Lewis, C., Mantini, D., Marzetti, L., et al. (2010). Temporal dynamics of spontaneous MEG activity in brain networks. Proc. Natl. Acad. Sci. U.S.A. 107, 6040–6045. doi: 10.1073/pnas.0913863107
Desikan, R. S., Segonne, F., Fischl, B., Quinn, B. T., Dickerson, B. C., Blacker, D., et al. (2006). An automated labeling system for subdividing the human cerebral cortex on MRI scans into gyral based regions of interest. Neuroimage 31, 968–980. doi: 10.1016/j.neuroimage.2006.01.021
Diehl, B., Busch, R. M., Duncan, J. S., Piao, Z., Tkach, J., and Luders, H. O. (2008). Abnormalities in diffusion tensor imaging of the uncinate fasciculus relate to reduced memory in temporal lobe epilepsy. Epilepsia 49, 1409–1418. doi: 10.1111/j.1528-1167.2008.01596.x
Dreifuss, S., Vingerhoets, F. J., Lazeyras, F., Andino, S. G., Spinelli, L., Delavelle, J., et al. (2001). Volumetric measurements of subcortical nuclei in patients with temporal lobe epilepsy. Neurology 57, 1636–1641. doi: 10.1212/WNL.57.9.1636
Du, F., Eid, T., Lothman, E. W., Köhler, C., and Schwarcz, R. (1995). Preferential neuronal loss in layer III of the medial entorhinal cortex in rat models of temporal lobe epilepsy. J. Neurosci. 15, 6301–6313.
Du, F., Whetsell, W. O., Abou-Khalil, B., and Blumenkopf, B. (1993). Preferential neuronal loss in layer III of the entorhinal cortex in patients with temporal lobe epilepsy. Epilepsy Res. 16, 223–233. doi: 10.1016/0920-1211(93)90083-J
Elsharkawy, A. E., Alabbasi, A. H., Pannek, H., Oppel, F., Schulz, R., Hoppe, M., et al. (2009). Long-term outcome after temporal lobe epilepsy surgery in 434 consecutive adult patients. J. Neurosurg. 110, 1135–1146. doi: 10.3171/2008.6.JNS17613
Engel, J. Jr., Thompson, P. M., Stern, J. M., Staba, R. J., Bragin, A., and Mody, I. (2013). Connectomics and epilepsy. Curr. Opin. Neurol. 26, 186–194. doi: 10.1097/WCO.0b013e32835ee5b8
Eriksson, S. H., Rydenhag, B., Uvebrant, P., Malmgren, K., and Nordborg, C. (2002). Widespread microdysgenesis in therapy-resistant epilepsy–a case report on post-mortem findings. Acta Neuropathol. (Berl.) 103, 74–77. doi: 10.1007/s004010100426
Falconer, M. A., Serafetinides, E. A., and Corsellis, J.A.N. (1964). Etiology and pathogenesis of temporal lobe epilepsy. Arch. Neurol. 10, 233–248. doi: 10.1001/archneur.1964.00460150003001
Feinberg, D. A., Moeller, S., Smith, S. M., Auerbach, E., Ramanna, S., Gunther, M., et al. (2010). Multiplexed echo planar imaging for sub-second whole brain FMRI and fast diffusion imaging. PLoS ONE 5:e15710. doi: 10.1371/journal.pone.0015710
Felleman, D. J., and Van Essen, D. C. (1991). Distributed hierarchical processing in the primate cerebral cortex. Cereb. Cortex 1, 1–47. doi: 10.1093/cercor/1.1.1
Focke, N. K., Yogarajah, M., Bonelli, S. B., Bartlett, P. A., Symms, M. R., and Duncan, J. S. (2008). Voxel-based diffusion tensor imaging in patients with mesial temporal lobe epilepsy and hippocampal sclerosis. Neuroimage 40, 728–737. doi: 10.1016/j.neuroimage.2007.12.031
Fox, M. D., and Greicius, M. (2010). Clinical applications of resting state functional connectivity. Front. Syst. Neurosci. 4:19. doi: 10.3389/fnsys.2010.00019
Frings, L., Schulze-Bonhage, A., Spreer, J., and Wagner, K. (2009). Remote effects of hippocampal damage on default network connectivity in the human brain. J. Neurol. 256, 2021–2029. doi: 10.1007/s00415-009-5233-0
Friston, K. J., Frith, C. D., Fletcher, P., Liddle, P. F., and Frackowiak, R. S. (1996). Functional topography: multidimensional scaling and functional connectivity in the brain. Cereb. Cortex 6, 156–164. doi: 10.1093/cercor/6.2.156
Friston, K. J., Frith, C. D., Liddle, P. F., and Frackowiak, R. S. (1993). Functional connectivity: the principal-component analysis of large (PET) data sets. J. Cereb. Blood Flow Metab. 13, 5–14. doi: 10.1038/jcbfm.1993.4
Geyer, S., Weiss, M., Reimann, K., Lohmann, G., and Turner, R. (2011). Microstructural parcellation of the human cerebral cortex - from Brodmann's post-mortem map to in vivo mapping with high-field magnetic resonance imaging. Front. Hum. Neurosci. 5:19. doi: 10.3389/fnhum.2011.00019
Glasser, M. F., and Van Essen, D. C. (2011). Mapping human cortical areas in vivo based on myelin content as revealed by T1- and T2-weighted MRI. J. Neurosci. 31, 11597–11616. doi: 10.1523/JNEUROSCI.2180-11.2011
Gong, G., He, Y., Concha, L., Lebel, C., Gross, D. W., Evans, A. C., et al. (2009). Mapping anatomical connectivity patterns of human cerebral cortex using in vivo diffusion tensor imaging tractography. Cereb. Cortex 19, 524–536. doi: 10.1093/cercor/bhn102
Gotman, J., Kobayashi, E., Bagshaw, A. P., Benar, C. G., and Dubeau, F. (2006). Combining EEG and fMRI: a multimodal tool for epilepsy research. J. Magn. Reson. Imaging 23, 906–920. doi: 10.1002/jmri.20577
Greicius, M. (2008). Resting-state functional connectivity in neuropsychiatric disorders. Curr. Opin. Neurol. 21, 424–430. doi: 10.1097/WCO.0b013e328306f2c5
Greicius, M. D., Krasnow, B., Reiss, A. L., and Menon, V. (2003). Functional connectivity in the resting brain: a network analysis of the default mode hypothesis. Proc. Natl. Acad. Sci. U.S.A. 100, 253–258. doi: 10.1073/pnas.0135058100
Gross, D. W., Concha, L., and Beaulieu, C. (2006). Extratemporal white matter abnormalities in mesial temporal lobe epilepsy demonstrated with diffusion tensor imaging. Epilepsia 47, 1360–1363. doi: 10.1111/j.1528-1167.2006.00603.x
Guye, M., Bettus, G., Bartolomei, F., and Cozzone, P. J. (2010). Graph theoretical analysis of structural and functional connectivity MRI in normal and pathological brain networks. MAGMA 23, 409–421. doi: 10.1007/s10334-010-0205-z
Hagmann, P., Cammoun, L., Gigandet, X., Meuli, R., Honey, C. J., Wedeen, V. J., et al. (2008). Mapping the structural core of human cerebral cortex. PLoS Biol. 6:e159. doi: 10.1371/journal.pbio.0060159
Hagmann, P., Kurant, M., Gigandet, X., Thiran, P., Wedeen, V. J., Meuli, R., et al. (2007). Mapping human whole-brain structural networks with diffusion MRI. PLoS ONE 2:e597. doi: 10.1371/journal.pone.0000597
Harriger, L., Van Den Heuvel, M. P., and Sporns, O. (2012). Rich club organization of macaque cerebral cortex and its role in network communication. PLoS ONE 7:e46497. doi: 10.1371/journal.pone.0046497
He, Y., Chen, Z. J., and Evans, A. C. (2007). Small-world anatomical networks in the human brain revealed by cortical thickness from MRI. Cereb. Cortex 17, 2407–2419. doi: 10.1093/cercor/bhl149
He, Y., Wang, J., Wang, L., Chen, Z. J., Yan, C., Yang, H., et al. (2009). Uncovering intrinsic modular organization of spontaneous brain activity in humans. PLoS ONE 4:e5226. doi: 10.1371/journal.pone.0005226
Hetherington, H. P., Kuzniecky, R. I., Vives, K., Devinsky, O., Pacia, S., Luciano, D., et al. (2007). A subcortical network of dysfunction in TLE measured by magnetic resonance spectroscopy. Neurology 69, 2256–2265. doi: 10.1212/01.wnl.0000286945.21270.6d
Hogan, R. E., Wang, L., Bertrand, M. E., Willmore, L. J., Bucholz, R. D., Nassif, A. S., et al. (2004). MRI-based high-dimensional hippocampal mapping in mesial temporal lobe epilepsy. Brain 127, 1731–1740. doi: 10.1093/brain/awh197
Holmes, G. L. (2002). Seizure-induced neuronal injury: animal data. Neurology 59, S3–S6. doi: 10.1212/WNL.59.9_suppl_5.S3
Honey, C. J., Sporns, O., Cammoun, L., Gigandet, X., Thiran, J. P., Meuli, R., et al. (2009). Predicting human resting-state functional connectivity from structural connectivity. Proc. Natl. Acad. Sci. U.S.A. 106, 2035–2040. doi: 10.1073/pnas.0811168106
Houser, C. R., Swartz, B. E., Walsh, G. O., and Delgado-Escueta, A. V. (1992). “Granule cell disorganization in the dentate gyrus: possible alterations of neuronal migration in human temporal lobe epilepsy,” in Molecular Neurobiology of Epilepsy, eds J. Engel Jr., C. Wasterlain, E. A. Cavalheiro, U. Heinemann, and G. Avanzini (Amsterdam: Elsevier Science), 41–47.
Iturria-Medina, Y., Canales-Rodriguez, E. J., Melie-Garcia, L., Valdes-Hernandez, P. A., Martinez-Montes, E., Aleman-Gomez, Y., et al. (2007). Characterizing brain anatomical connections using diffusion weighted MRI and graph theory. Neuroimage 36, 645–660. doi: 10.1016/j.neuroimage.2007.02.012
Jann, K., Kottlow, M., Dierks, T., Boesch, C., and Koenig, T. (2010). Topographic electrophysiological signatures of FMRI Resting State Networks. PLoS ONE 5:e12945. doi: 10.1371/journal.pone.0012945
Jbabdi, S., and Johansen-Berg, H. (2011). Tractography: where do we go from here? Brain Connect. 1, 169–183. doi: 10.1089/brain.2011.0033
Johansen-Berg, H., and Behrens, T. E. (2006). Just pretty pictures? What diffusion tractography can add in clinical neuroscience. Curr. Opin. Neurol. 19, 379–385. doi: 10.1097/01.wco.0000236618.82086.01
Jones, D. K., Knosche, T. R., and Turner, R. (2012). White matter integrity, fiber count, and other fallacies: the do's and don'ts of diffusion MRI. Neuroimage 73, 239–254. doi: 10.1016/j.neuroimage.2012.06.081
Jutila, L., Ylinen, A., Partanen, K., Alafuzoff, I., Mervaala, E., Partanen, J., et al. (2001). MR volumetry of the entorhinal, perirhinal, and temporopolar cortices in drug-refractory temporal lobe epilepsy. AJNR. Am. J. Neuroradiol. 22, 1490–1501.
Kaiser, M., and Hilgetag, C. C. (2010). Optimal hierarchical modular topologies for producing limited sustained activation of neural networks. Front. Neuroinform. 4:8. doi: 10.3389/fninf.2010.00008
Keller, S. S., and Roberts, N. (2008). Voxel-based morphometry of temporal lobe epilepsy: an introduction and review of the literature. Epilepsia 49, 741–757. doi: 10.1111/j.1528-1167.2007.01485.x
Kelly, C., Biswal, B. B., Craddock, R. C., Castellanos, F. X., and Milham, M. P. (2012). Characterizing variation in the functional connectome: promise and pitfalls. Trends Cogn. Sci. 16, 181–188. doi: 10.1016/j.tics.2012.02.001
Kemmotsu, N., Girard, H. M., Bernhardt, B. C., Bonilha, L., Lin, J. J., Tecoma, E. S., et al. (2011). MRI analysis in temporal lobe epilepsy: cortical thinning and white matter disruptions are related to side of seizure onset. Epilepsia 52, 2257–2266. doi: 10.1111/j.1528-1167.2011.03278.x
Khundrakpam, B. S., Reid, A., Brauer, J., Carbonell, F., Lewis, J., Ameis, S., et al. (2012). Developmental changes in organization of structural brain networks. Cereb. Cortex 23, 2072–2085. doi: 10.1093/cercor/bhs187
Kim, H., Besson, P., Colliot, O., Bernasconi, A., and Bernasconi, N. (2008). Surface-based vector analysis using heat equation interpolation: a new approach to quantify local hippocampal volume changes. Med. Image. Comput. Comput. Assist. Interv. 11, 1008–1015. doi: 10.1007/978-3-540-85988-8_120
Kobayashi, E., Bagshaw, A. P., Benar, C. G., Aghakhani, Y., Andermann, F., Dubeau, F., et al. (2006). Temporal and extratemporal BOLD responses to temporal lobe interictal spikes. Epilepsia 47, 343–354. doi: 10.1111/j.1528-1167.2006.00427.x
Kramer, M. A., Kolaczyk, E. D., and Kirsch, H. E. (2008). Emergent network topology at seizure onset in humans. Epilepsy Res. 79, 173–186. doi: 10.1016/j.eplepsyres.2008.02.002
Kuzniecky, R. I., De La Sayette, V., Ethier, R., Melanson, D., Andermann, F., Berkovic, S. F., et al. (1987). Magnetic resonance imaging in temporal lobe epilepsy: pathological correlations. Ann. Neurol. 22, 341–347. doi: 10.1002/ana.410220310
Lachaux, J. P., Rodriguez, E., Martinerie, J., and Varela, F. J. (1999). Measuring phase synchrony in brain signals. Hum. Brain Mapp. 8, 194–208. doi: 10.1002/(SICI)1097-0193(1999)8:4<194::AID-HBM4>3.0.CO;2-C
Laird, A. R., Fox, P. M., Eickhoff, S. B., Turner, J. A., Ray, K. L., Mckay, D. R., et al. (2011). Behavioral interpretations of intrinsic connectivity networks. J. Cogn. Neurosci. 23, 4022–4037. doi: 10.1162/jocn_a_00077
Laufs, H., Hamandi, K., Salek-Haddadi, A., Kleinschmidt, A. K., Duncan, J. S., and Lemieux, L. (2007). Temporal lobe interictal epileptic discharges affect cerebral activity in “default mode” brain regions. Hum. Brain Mapp. 28, 1023–1032. doi: 10.1002/hbm.20323
Le Bihan, D., Breton, E., Lallemand, D., Grenier, P., Cabanis, E., and Laval-Jeantet, M. (1986). MR imaging of intravoxel incoherent motions: application to diffusion and perfusion in neurologic disorders. Radiology 161, 401–407.
Le Bihan, D., Turner, R., Douek, P., and Patronas, N. (1996). Diffusion MR imaging: clinical application. AJNR Am. J. Neuroradiol. 159, 591–599.
Lemieux, L., Daunizeau, J., and Walker, M. C. (2011). Concepts of connectivity and human epileptic activity. Front. Syst. Neurosci. 5:12. doi: 10.3389/fnsys.2011.00012
Leonardi, M., and Ustun, T. B. (2002). The global burden of epilepsy. Epilepsia 43(Suppl. 6), 21–25. doi: 10.1046/j.1528-1157.43.s.6.11.x
Lerch, J. P., Worsley, K., Shaw, W. P., Greenstein, D. K., Lenroot, R. K., Giedd, J., et al. (2006). Mapping anatomical correlations across cerebral cortex (MACACC) using cortical thickness from MRI. Neuroimage 31, 993–1003. doi: 10.1016/j.neuroimage.2006.01.042
Liao, W., Zhang, Z., Pan, Z., Mantini, D., Ding, J., Duan, X., et al. (2010). Altered functional connectivity and small-world in mesial temporal lobe epilepsy. PLoS ONE 5:e8525. doi: 10.1371/journal.pone.0008525
Lieb, J. P., Dasheiff, R. M., and Engel, J. Jr. (1991). Role of the frontal lobes in the propagation of mesial temporal lobe seizures. Epilepsia 32, 822–837. doi: 10.1111/j.1528-1157.1991.tb05539.x
Lieb, J. P., Hoque, K., Skomer, C. E., and Song, X. W. (1987). Inter-hemispheric propagation of human mesial temporal lobe seizures: a coherence/phase analysis. Electroencephalogr. Clin. Neurophysiol. 67, 101–119. doi: 10.1016/0013-4694(87)90033-2
Lin, J. J., Riley, J. D., Juranek, J., and Cramer, S. C. (2008). Vulnerability of the frontal-temporal connections in temporal lobe epilepsy. Epilepsy Res. 82, 162–170. doi: 10.1016/j.eplepsyres.2008.07.020
Lin, J. J., Salamon, N., Lee, A. D., Dutton, R. A., Geaga, J. A., Hayashi, K. M., et al. (2007). Reduced neocortical thickness and complexity mapped in mesial temporal lobe epilepsy with hippocampal sclerosis. Cereb. Cortex 17, 2007–2018. doi: 10.1093/cercor/bhl109
Lohmann, G., Margulies, D. S., Horstmann, A., Pleger, B., Lepsien, J., Goldhahn, D., et al. (2010). Eigenvector centrality mapping for analyzing connectivity patterns in fMRI data of the human brain. PLoS ONE 5:e10232. doi: 10.1371/journal.pone.0010232
Lopes Da Silva, F., Pijn, J. P., and Boeijinga, P. (1989). Interdependence of EEG signals: linear vs. nonlinear associations and the significance of time delays and phase shifts. Brain Topogr. 2, 9–18. doi: 10.1007/BF01128839
Mantini, D., Gerits, A., Nelissen, K., Durand, J. B., Joly, O., Simone, L., et al. (2011). Default mode of brain function in monkeys. J. Neurosci. 31, 12954–12962. doi: 10.1523/JNEUROSCI.2318-11.2011
Margerison, J. H., and Corsellis, J. A. (1966). Epilepsy and the temporal lobes. A clinical, electroencephalographic and neuropathological study of the brain in epilepsy, with particular reference to the temporal lobes. Brain 89, 499–530. doi: 10.1093/brain/89.3.499
Margulies, D. S., Kelly, A. M., Uddin, L. Q., Biswal, B. B., Castellanos, F. X., and Milham, M. P. (2007). Mapping the functional connectivity of anterior cingulate cortex. Neuroimage 37, 579–588. doi: 10.1016/j.neuroimage.2007.05.019
Mars, R. B., Jbabdi, S., Sallet, J., O'Reilly, J. X., Croxson, P. L., Olivier, E., et al. (2011). Diffusion-weighted imaging tractography-based parcellation of the human parietal cortex and comparison with human and macaque resting-state functional connectivity. J. Neurosci. 31, 4087–4100. doi: 10.1523/JNEUROSCI.5102-10.2011
Mcdonald, C. R., Ahmadi, M. E., Hagler, D. J., Tecoma, E. S., Iragui, V. J., Gharapetian, L., et al. (2008a). Diffusion tensor imaging correlates of memory and language impairments in temporal lobe epilepsy. Neurology 71, 1869–1876. doi: 10.1212/01.wnl.0000327824.05348.3b
Mcdonald, C. R., Hagler, D. J. Jr., Ahmadi, M. E., Tecoma, E., Iragui, V., Dale, A. M., et al. (2008b). Subcortical and cerebellar atrophy in mesial temporal lobe epilepsy revealed by automatic segmentation. Epilepsy Res. 79, 130–138. doi: 10.1016/j.eplepsyres.2008.01.006
Mcdonald, C. R., Hagler, D. J. Jr., Ahmadi, M. E., Tecoma, E., Iragui, V., Gharapetian, L., et al. (2008c). Regional neocortical thinning in mesial temporal lobe epilepsy. Epilepsia 49, 794–803. doi: 10.1111/j.1528-1167.2008.01539.x
Mcintosh, A. M., Kalnins, R. M., Mitchell, L. A., Fabinyi, G. C., Briellmann, R. S., and Berkovic, S. F. (2004). Temporal lobectomy: long-term seizure outcome, late recurrence and risks for seizure recurrence. Brain 127, 2018–2030. doi: 10.1093/brain/awh221
Mechelli, A., Friston, K. J., Frackowiak, R. S., and Price, C. J. (2005). Structural covariance in the human cortex. J. Neurosci. 25, 8303–8310. doi: 10.1523/JNEUROSCI.0357-05.2005
Meiners, L. C., Witkamp, T. D., De Kort, G. A., Van Huffelen, A. C., Van Der, G. Y., Jansen, G. H., et al. (1999). Relevance of temporal lobe white matter changes in hippocampal sclerosis. Magnetic resonance imaging and histology. Invest. Radiol. 34, 38–45. doi: 10.1097/00004424-199901000-00006
Meunier, D., Lambiotte, R., and Bullmore, E. T. (2010). Modular and hierarchically modular organization of brain networks. Front. Neurosci. 4:200. doi: 10.3389/fnins.2010.00200
Mitchell, L. A., Jackson, G. D., Kalnins, R. M., Saling, M. M., Fitt, G. J., Ashpole, R. D., et al. (1999). Anterior temporal abnormality in temporal lobe epilepsy: a quantitative MRI and histopathologic study. Neurology 52, 327–336. doi: 10.1212/WNL.52.2.327
Modha, D. S., and Singh, R. (2010). Network architecture of the long-distance pathways in the macaque brain. Proc. Natl. Acad. Sci. U.S.A. 107, 13485–13490. doi: 10.1073/pnas.1008054107
Moran, N. F., Lemieux, L., Kitchen, N. D., Fish, D. R., and Shorvon, S. D. (2001). Extrahippocampal temporal lobe atrophy in temporal lobe epilepsy and mesial temporal sclerosis. Brain 124, 167–175. doi: 10.1093/brain/124.1.167
Mori, S., Crain, B. J., Chacko, V. P., and Van Zijl, P. C. (1999). Three-dimensional tracking of axonal projections in the brain by magnetic resonance imaging. Ann. Neurol. 45, 265–269. doi: 10.1002/1531-8249(199902)45:2<265::AID-ANA21>3.0.CO;2-3
Mueller, S. G., Laxer, K. D., Barakos, J., Cheong, I., Finlay, D., Garcia, P., et al. (2009a). Involvement of the thalamocortical network in TLE with and without mesiotemporal sclerosis. Epilepsia. doi: 10.1111/j.1528-1167.2009.02413.x
Mueller, S. G., Laxer, K. D., Barakos, J., Ian, C., Garcia, P., and Weiner, M. W. (2009b). Widespread neocortical abnormalities in temporal lobe epilepsy with and without mesial sclerosis. Neuroimage 46, 353–359. doi: 10.1016/j.neuroimage.2009.02.020
Musso, F., Brinkmeyer, J., Mobascher, A., Warbrick, T., and Winterer, G. (2010). Spontaneous brain activity and EEG microstates. A novel EEG/fMRI analysis approach to explore resting-state networks. Neuroimage 52, 1149–1161. doi: 10.1016/j.neuroimage.2010.01.093
Natsume, J., Bernasconi, N., Andermann, F., and Bernasconi, A. (2003). MRI volumetry of the thalamus in temporal, extratemporal, and idiopathic generalized epilepsy. Neurology 60, 1296–1300. doi: 10.1212/01.WNL.0000058764.34968.C2
Parker, G. J., Stephan, K. E., Barker, G. J., Rowe, J. B., Macmanus, D. G., Wheeler-Kingshott, C. A., et al. (2002). Initial demonstration of in vivo tracing of axonal projections in the macaque brain and comparison with the human brain using diffusion tensor imaging and fast marching tractography. Neuroimage 15, 797–809. doi: 10.1006/nimg.2001.0994
Ponten, S. C., Bartolomei, F., and Stam, C. J. (2007). Small-world networks and epilepsy: graph theoretical analysis of intracerebrally recorded mesial temporal lobe seizures. Clin. Neurophysiol. 118, 918–927. doi: 10.1016/j.clinph.2006.12.002
Ponten, S. C., Douw, L., Bartolomei, F., Reijneveld, J. C., and Stam, C. J. (2009). Indications for network regularization during absence seizures: weighted and unweighted graph theoretical analyses. Exp. Neurol. 217, 197–204. doi: 10.1016/j.expneurol.2009.02.001
Pugliatti, M., Beghi, E., Forsgren, L., Ekman, M., and Sobocki, P. (2007). Estimating the cost of epilepsy in Europe: a review with economic modeling. Epilepsia 48, 2224–2233.
Raichle, M. E., Macleod, A. M., Snyder, A. Z., Powers, W. J., Gusnard, D. A., and Shulman, G. L. (2001). A default mode of brain function. Proc. Natl. Acad. Sci. U.S.A. 98, 676–682. doi: 10.1073/pnas.98.2.676
Raznahan, A., Lerch, J. P., Lee, N., Greenstein, D., Wallace, G. L., Stockman, M., et al. (2011). Patterns of coordinated anatomical change in human cortical development: a longitudinal neuroimaging study of maturational coupling. Neuron 72, 873–884. doi: 10.1016/j.neuron.2011.09.028
Rodrigo, S., Oppenheim, C., Chassoux, F., Golestani, N., Cointepas, Y., Poupon, C., et al. (2007). Uncinate fasciculus fiber tracking in mesial temporal lobe epilepsy. Initial findings. Eur. Radiol. 17, 1663–1668. doi: 10.1007/s00330-006-0558-x
Rosenberg, D. S., Mauguiere, F., Demarquay, G., Ryvlin, P., Isnard, J., Fischer, C., et al. (2006). Involvement of medial pulvinar thalamic nucleus in human temporal lobe seizures. Epilepsia 47, 98–107. doi: 10.1111/j.1528-1167.2006.00375.x
Ryvlin, P. (2003). Beyond pharmacotherapy: surgical management. Epilepsia 44(Suppl. 5), 23–28. doi: 10.1046/j.1528-1157.44.s.5.4.x
Salvador, R., Suckling, J., Schwarzbauer, C., and Bullmore, E. (2005). Undirected graphs of frequency-dependent functional connectivity in whole brain networks. Philos. Trans. R. Soc. Lond. B Biol. Sci. 360, 937–946. doi: 10.1098/rstb.2005.1645
Sanabria, E. R., Da Silva, A. V., Spreafico, R., and Cavalheiro, E. A. (2002). Damage, reorganization, and abnormal neocortical hyperexcitability in the pilocarpine model of temporal lobe epilepsy. Epilepsia 43(Suppl. 5), 96–106. doi: 10.1046/j.1528-1157.43.s.5.31.x
Sankar, T., Bernasconi, N., Kim, H., and Bernasconi, A. (2008). Temporal lobe epilepsy: differential pattern of damage in temporopolar cortex and white matter. Hum. Brain Mapp. 29, 931–944. doi: 10.1002/hbm.20437
Scannell, J. W., Blakemore, C., and Young, M. P. (1995). Analysis of connectivity in the cat cerebral cortex. J. Neurosci. 15, 1463–1483.
Scharfman, H. E. (2007). The neurobiology of epilepsy. Curr. Neurol. Neurosci. Rep. 7, 348–354. doi: 10.1007/s11910-007-0053-z
Schindler, K. A., Bialonski, S., Horstmann, M. T., Elger, C. E., and Lehnertz, K. (2008). Evolving functional network properties and synchronizability during human epileptic seizures. Chaos 18, 033119. doi: 10.1063/1.2966112
Scott, R. C., King, M. D., Gadian, D. G., Neville, B. G., and Connelly, A. (2006). Prolonged febrile seizures are associated with hippocampal vasogenic edema and developmental changes. Epilepsia 47, 1493–1498. doi: 10.1111/j.1528-1167.2006.00621.x
Shen, K., Bezgin, G., Hutchison, R. M., Gati, J. S., Menon, R. S., Everling, S., et al. (2012). Information processing architecture of functionally defined clusters in the macaque cortex. J. Neurosci. 32, 17465–17476. doi: 10.1523/JNEUROSCI.2709-12.2012
Sloan, D. M., and Bertram, E. H. 3rd. (2009). Changes in midline thalamic recruiting responses in the prefrontal cortex of the rat during the development of chronic limbic seizures. Epilepsia 50, 556–565. doi: 10.1111/j.1528-1167.2008.01790.x
Smith, S. M. (2012). The future of FMRI connectivity. Neuroimage 62, 1257–1266. doi: 10.1016/j.neuroimage.2012.01.022
Smith, S. M., Fox, P. T., Miller, K. L., Glahn, D. C., Fox, P. M., Mackay, C. E., et al. (2009). Correspondence of the brain's functional architecture during activation and rest. Proc. Natl. Acad. Sci. U.S.A. 106, 13040–13045. doi: 10.1073/pnas.0905267106
Smith, S. M., Miller, K. L., Moeller, S., Xu, J., Auerbach, E. J., Woolrich, M. W., et al. (2012). Temporally-independent functional modes of spontaneous brain activity. Proc. Natl. Acad. Sci. U.S.A. 109, 3131–3136. doi: 10.1073/pnas.1121329109
Sommer, W. (1880). Erkrankung des ammonshorns als aetiologisches moment der epilepsie. Arch. Psychiatr. Nervenkr. 10, 631–675. doi: 10.1007/BF02224538
Spencer, S. S. (2002). Neural networks in human epilepsy: evidence of and implications for treatment. Epilepsia 43, 219–227. doi: 10.1046/j.1528-1157.2002.26901.x
Sporns, O. (2011). The human connectome: a complex network. Ann. N.Y. Acad. Sci. 1224, 109–125. doi: 10.1111/j.1749-6632.2010.05888.x
Sporns, O., Chialvo, D. R., Kaiser, M., and Hilgetag, C. C. (2004). Organization, development and function of complex brain networks. Trends Cogn. Sci. 8, 418–425. doi: 10.1016/j.tics.2004.07.008
Sporns, O., Honey, C. J., and Kotter, R. (2007). Identification and classification of hubs in brain networks. PLoS ONE 2:e1049. doi: 10.1371/journal.pone.0001049
Sporns, O., Tononi, G., and Kotter, R. (2005). The human connectome: a structural description of the human brain. PLoS Comput. Biol. 1:e42. doi: 10.1371/journal.pcbi.0010042
Stam, C. J. (2004). Functional connectivity patterns of human magnetoencephalographic recordings: a ‘small-world' network? Neurosci. Lett. 355, 25–28. doi: 10.1016/j.neulet.2003.10.063
Stephan, K. E., Hilgetag, C. C., Burns, G. A., O'neill, M. A., Young, M. P., and Kotter, R. (2000). Computational analysis of functional connectivity between areas of primate cerebral cortex. Philos. Trans. R. Soc. Lond. B Biol. Sci. 355, 111–126. doi: 10.1098/rstb.2000.0552
Stone, J. V., and Kotter, R. (2002). Making connections about brain connectivity. Trends Cogn. Sci. 6, 327–328. doi: 10.1016/S1364-6613(02)01943-5
Sutula, T., He, X. X., Cavazos, J., and Scott, G. (1988). Synaptic reorganization in the hippocampus induced by abnormal functional activity. Science 239, 1147–1150. doi: 10.1126/science.2449733
Tellez-Zenteno, J. F., Dhar, R., Hernandez-Ronquillo, L., and Wiebe, S. (2007). Long-term outcomes in epilepsy surgery: antiepileptic drugs, mortality, cognitive and psychosocial aspects. Brain 130, 334–345. doi: 10.1093/brain/awl316
Thom, M., Eriksson, S., Martinian, L., Caboclo, L. O., Mcevoy, A. W., Duncan, J. S., et al. (2009). Temporal lobe sclerosis associated with hippocampal sclerosis in temporal lobe epilepsy: neuropathological features. J. Neuropathol. Exp. Neurol. 68, 928–938. doi: 10.1097/NEN.0b013e3181b05d67
Tohka, J., He, Y., and Evans, A. C. (2012). The impact of sampling density upon cortical network analysis: regions or points. Magn. Reson. Imaging 30, 978–992. doi: 10.1016/j.mri.2012.02.029
Tomasi, D., and Volkow, N. D. (2011). Functional connectivity hubs in the human brain. Neuroimage 57, 908–917. doi: 10.1016/j.neuroimage.2011.05.024
Tononi, G., Sporns, O., and Edelman, G. M. (1994). A measure for brain complexity: relating functional segregation and integration in the nervous system. Proc. Natl. Acad. Sci. U.S.A. 91, 5033–5037. doi: 10.1073/pnas.91.11.5033
Turski, W. A., Cavalheiro, E. A., Schwarz, M., Czuczwar, S. J., Kleinrok, Z., and Turski, L. (1983). Limbic seizures produced by pilocarpine in rats: behavioural, electroencephalographic and neuropathological study. Behav. Brain Res. 9, 315–335. doi: 10.1016/0166-4328(83)90136-5
Vaessen, M. J., Braakman, H. M., Heerink, J. S., Jansen, J. F., Debeij-Van Hall, M. H., Hofman, P. A., et al. (2012a). Abnormal modular organization of functional networks in cognitively impaired children with frontal lobe epilepsy. Cereb. Cortex 23, 1997–2006. doi: 10.1093/cercor/bhs186
Vaessen, M. J., Jansen, J. F., Vlooswijk, M. C., Hofman, P. A., Majoie, H. J., Aldenkamp, A. P., et al. (2012b). White matter network abnormalities are associated with cognitive decline in chronic epilepsy. Cereb. Cortex 22, 2139–2147. doi: 10.1093/cercor/bhr298
Van Den Heuvel, M. P., and Sporns, O. (2011). Rich-club organization of the human connectome. J. Neurosci. 31, 15775–15786. doi: 10.1523/JNEUROSCI.3539-11.2011
Van Essen, D. C. (2005). A Population-Average, Landmark- and Surface-based (PALS) atlas of human cerebral cortex. Neuroimage 28, 635–662. doi: 10.1016/j.neuroimage.2005.06.058
Van Essen, D. C., Glasser, M. F., Dierker, D. L., and Harwell, J. (2012). Cortical parcellations of the macaque monkey analyzed on surface-based atlases. Cereb. Cortex 22, 2227–2240. doi: 10.1093/cercor/bhr290
Vlooswijk, M. C., Vaessen, M. J., Jansen, J. F., De Krom, M. C., Majoie, H. J., Hofman, P. A., et al. (2011). Loss of network efficiency associated with cognitive decline in chronic epilepsy. Neurology 77, 938–944. doi: 10.1212/WNL.0b013e31822cfc2f
Voets, N. L., Beckmann, C. F., Cole, D. M., Hong, S., Bernasconi, A., and Bernasconi, N. (2012). Structural substrates for resting network disruption in temporal lobe epilepsy. Brain. doi: 10.1093/brain/aws137
Voets, N. L., Bernhardt, B. C., Kim, H., Yoon, U., and Bernasconi, N. (2011). Increased temporolimbic cortical folding complexity in temporal lobe epilepsy. Neurology 76, 138–144. doi: 10.1212/WNL.0b013e318205d521
Waites, A. B., Briellmann, R. S., Saling, M. M., Abbott, D. F., and Jackson, G. D. (2006). Functional connectivity networks are disrupted in left temporal lobe epilepsy. Ann. Neurol. 59, 335–343. doi: 10.1002/ana.20733
Watts, D. J., and Strogatz, S. H. (1998). Collective dynamics of ‘small-world' networks. Nature 393, 440–442. doi: 10.1038/30918
Wiebe, S., Blume, W. T., Girvin, J. P., and Eliasziw, M. (2001). A randomized, controlled trial of surgery for temporal-lobe epilepsy. N. Engl. J. Med. 345, 311–318. doi: 10.1056/NEJM200108023450501
Worsley, K. J., Taylor, J. E., Carbonell, F., Chung, M. K., Duerden, E., Bernhardt, B., et al. (2009). SurfStat: a matlab toolbox for the statistical analysis of univariate and multivariate surface and volumetric data using linear mixed effects models and random field theory. Neuroimage 47, S102. doi: 10.1016/S1053-8119(09)70882-1
Xia, M., and He, Y. (2011). Magnetic resonance imaging and graph theoretical analysis of complex brain networks in neuropsychiatric disorders. Brain Connect. 1, 349–365. doi: 10.1089/brain.2011.0062
Yilmazer-Hanke, D. M., Wolf, H. K., Schramm, J., Elger, C. E., Wiestler, O. D., and Blumcke, I. (2000). Subregional pathology of the amygdala complex and entorhinal region in surgical specimens from patients with pharmacoresistant temporal lobe epilepsy. J. Neuropathol. Exp. Neurol. 59, 907–920.
Yogarajah, M., and Duncan, J. S. (2008). Diffusion-based magnetic resonance imaging and tractography in epilepsy. Epilepsia 49, 189–200. doi: 10.1111/j.1528-1167.2007.01378.x
Yu, J. T., and Tan, L. (2008). Diffusion-weighted magnetic resonance imaging demonstrates parenchymal pathophysiological changes in epilepsy. Brain Res. Rev. 59, 34–41. doi: 10.1016/j.brainresrev.2008.04.008
Yu, Q., Plis, S. M., Erhardt, E. B., Allen, E. A., Sui, J., Kiehl, K. A., et al. (2011a). Modular organization of functional network connectivity in healthy controls and patients with schizophrenia during the resting state. Front. Syst. Neurosci. 5:103. doi: 10.3389/fnsys.2011.00103
Yu, Q., Sui, J., Rachakonda, S., He, H., Gruner, W., Pearlson, G., et al. (2011b). Altered topological properties of functional network connectivity in schizophrenia during resting state: a small-world brain network study. PLoS ONE 6:e25423. doi: 10.1371/journal.pone.0025423
Yu, Q., Sui, J., Liu, J., Plis, S. M., Kiehl, K. A., Pearlson, G., et al. (2013). Disrupted correlation between low frequency power and connectivity strength of resting state brain networks in schizophrenia. Schizophr. Res. 143, 165–171. doi: 10.1016/j.schres.2012.11.001
Yuan, H., Zotev, V., Phillips, R., Drevets, W. C., and Bodurka, J. (2012). Spatiotemporal dynamics of the brain at rest–exploring EEG microstates as electrophysiological signatures of BOLD resting state networks. Neuroimage 60, 2062–2072. doi: 10.1016/j.neuroimage.2012.02.031
Zhang, Z., Lu, G., Zhong, Y., Tan, Q., Liao, W., Wang, Z., et al. (2010). Altered spontaneous neuronal activity of the default-mode network in mesial temporal lobe epilepsy. Brain Res. 1323, 152–160. doi: 10.1016/j.brainres.2010.01.042
Zielinski, B. A., Gennatas, E. D., Zhou, J., and Seeley, W. W. (2010). Network-level structural covariance in the developing brain. Proc. Natl. Acad. Sci. U.S.A. 107, 18191–18196. doi: 10.1073/pnas.1003109107
Keywords: TLE, connectivity, MRI, graph-theory, connectome
Citation: Bernhardt BC, Hong S, Bernasconi A and Bernasconi N (2013) Imaging structural and functional brain networks in temporal lobe epilepsy. Front. Hum. Neurosci. 7:624. doi: 10.3389/fnhum.2013.00624
Received: 21 March 2013; Accepted: 09 September 2013;
Published online: 01 October 2013.
Edited by:
Yong He, Beijing Normal University, ChinaReviewed by:
Qingbao Yu, The Mind Research Network, USAXiaobo Li, Albert Einstein College of Medicine, USA
Copyright © 2013 Bernhardt, Hong, Bernasconi and Bernasconi. This is an open-access article distributed under the terms of the Creative Commons Attribution License (CC BY). The use, distribution or reproduction in other forums is permitted, provided the original author(s) or licensor are credited and that the original publication in this journal is cited, in accordance with accepted academic practice. No use, distribution or reproduction is permitted which does not comply with these terms.
*Correspondence: Boris C. Bernhardt, Neuroimaging of Epilepsy Laboratory, Montreal Neurological Institute and Hospital, McGill University, 3801 University Street, H3A2B4, Montreal, QC, Canada e-mail: boris@bic.mni.mcgill.ca