- 1Department of Psychology, Perceptual-Motor Dynamics Laboratory, CAP Center for Cognition, Action, and Perception, University of Cincinnati, Cincinnati, OH, USA
- 2Movement to Health Laboratory, EuroMov, Montpellier-1 University, Montpellier, France
Traditional theories of cognitive science have typically accounted for the organization of human behavior by detailing requisite computational/representational functions and identifying neurological mechanisms that might perform these functions. Put simply, such approaches hold that neural activity causes behavior. This same general framework has been extended to accounts of human social behavior via concepts such as “common-coding” and “co-representation” and much recent neurological research has been devoted to brain structures that might execute these social-cognitive functions. Although these neural processes are unquestionably involved in the organization and control of human social interactions, there is good reason to question whether they should be accorded explanatory primacy. Alternatively, we propose that a full appreciation of the role of neural processes in social interactions requires appropriately situating them in their context of embodied-embedded constraints. To this end, we introduce concepts from dynamical systems theory and review research demonstrating that the organization of human behavior, including social behavior, can be accounted for in terms of self-organizing processes and lawful dynamics of animal-environment systems. Ultimately, we hope that these alternative concepts can complement the recent advances in cognitive neuroscience and thereby provide opportunities to develop a complete and coherent account of human social interaction.
Introduction
To note that human beings are “social creatures” is to risk making a dramatic understatement. Consider examples of everyday behavior in which individuals appear to be acting entirely on their own. Consider sitting at an intersection while waiting for the light to change. Although you are alone in the car, as evidenced by your singing along to the radio, in some real sense this is “social behavior.” In obeying the rules of the road, and stopping at the light when it is red, your behavior is organized by social convention. In singing along to the radio, you engage in a behavior of immense social significance with roots in thousands of years of cultural tradition. That our natural behaviors are so deeply about the behavior of other human beings makes considerable demands on theories of social interaction.
The past 20 years of cognitive neuroscience has met these demands with research on neurological structures proposed as mechanisms serving the many functions of social cognition and joint action (Gallese, 2003; Newman-Norland et al., 2007; Graf et al., 2009). The activity of such neural networks, most notably mirror neuron systems (Gallese et al., 1996; Iacoboni et al., 1999; see Rizzolatti and Craighero, 2004, for review), have been implicated across the full range of social phenomena, from coordinated spatio-temporal interactions (e.g., imitation; Iacoboni, 2005) to the ability to understand one another's cognitive and emotional states (e.g., empathy; Gallese, 2001). Although these neural structures are unquestionably part of a complete account, basing a theory of human behavior solely on neural processes, to the exclusion of all others, implicitly assumes a causal primacy.
In line with the focus of this special issue, online and real-time social interactions involve a host of processes that serve as important constraints in shaping and organizing social behavior and cognition. In this paper, we introduce a set of concepts from dynamical systems theory (e.g., Kugler and Turvey, 1987; Saltzman and Kelso, 1987; Turvey, 1990; Kelso, 1995; Warren, 2006) that provide a means for capturing how organized behavior emerges, or “self-organizes,” from these rich contexts of constraint. To detail how these concepts might apply to social interactions, we explore interpersonal rhythmic coordination as an example of self-organization in joint action (see Marsh et al., 2006, 2009; Richardson et al., 2008, 2010; Schmidt and Richardson, 2008 for more detailed reviews). Consistent with the embodied-embedded perspective (e.g., Brooks, 1991; Clark, 1998; van Dijk et al., 2008), we argue for a theory of social interaction that situates the activity of neural structures in a context of continuous interaction with a myriad of other natural processes. Ultimately, we hope that this set of alternative concepts can serve as a complementary framework (see Kelso and Enstrøm, 2006) to traditional theories and advance the development of a complete and coherent account of human behavior and mind.
Social Neuromechanics
The field of cognitive science has customarily explained human behavior as the output of a computational system. Generally, these theories contend that behavior is organized and controlled via cognitive processes manifest in the activities of the central nervous system, which form representations of the environment from incoming sensory stimulation, and plan actions in accordance with these representations (e.g., von Eckardt, 1993; Kawato, 1999). In short, neural mechanisms are the crucial causal link between the organism and its environment. The same explanatory principles are applied in theories of social behavior. Functions necessary for social interaction, such as predicting the actions of co-actors, are met with representational mechanisms carried out in neural processing (e.g., Hommel et al., 2001; Blakemore and Frith, 2005; Sebanz and Knoblich, 2009).
Research in support of these principles has produced an abundance of information detailing neurological mechanisms that might support such representational processes. Comprehensive review of this literature is beyond the scope of this article, and has already been provided elsewhere (e.g., Rizzolatti and Craighero, 2004; Newman-Norland et al., 2007; Graf et al., 2009). The forthcoming argument concerning the nature of these neural processes, however, requires at a minimum an appreciation of the basic findings underlying concepts such as “common-coding” and “co-representation.”
Converging evidence supports that several structures across the human brain comprise a network which underlies social functions (Grafton and Hamilton, 2007; Newman-Norland et al., 2007; Bekkering et al., 2009). For instance, several studies have supported the involvement of the superior temporal sulcus (STS) in the perception of biological motion and goal-directed movements (e.g., Grossman and Blake, 2000; Puce and Perrett, 2003; Schultz et al., 2004). Specifically, increased activity in the STS has been associated with point-light displays of goal-directed or biological movements as contrasted against random point-light movements (Bonda et al., 1996; Grossman and Blake, 2000). Interestingly, the STS shows increased activity not only with stimuli depicting human movement, but also with stimuli depicting non-human objects that appear to reflect animate movement (Blakemore et al., 2003; Schultz et al., 2004). For instance, Schultz et al. (2005) manipulated the degree to which the movements of geometrical shapes were correlated with one another, and found that increases in this “interactivity parameter” were met with greater ratings of animacy and increased activity in the STS. Thus, the function of the STS (i.e., detection of goal-directed movement) is argued to underlie the acquisition of information critical to sustaining social interactions (Puce and Perrett, 2003).
Although social interactions doubtlessly involve the STS, it was the discovery of neural structures involved in both the perception and production of goal-directed actions that transformed neurological accounts of joint action (for reviews see Rizzolatti and Craighero, 2004; Rizzolatti and Sinigaglia, 2010). Briefly, these “mirror neurons” were originally discovered in the premotor cortex of macaque monkeys when cells became active during both execution and observation of object-directed actions (di Pellegrino et al., 1992). Subsequent research has detailed the different actions coded by these neurons (e.g., power vs. precision grips) and revealed the extent to which these neurons were “congruent,” requiring an exact match between observed and executed action (Gallese et al., 1996; Rizzolatti et al., 1996a).
Structures with similar mirror properties have been discovered in the human brain (Fadiga et al., 1995; Rizzolatti et al., 1996b; Iacoboni et al., 1999; Decety et al., 2002). The human mirror system is a more complex network with areas displaying mirror properties in both frontal and parietal regions (Rizzolatti and Craighero, 2004). Whereas the frontal regions are involved in coding for the goal of the action, the parietal regions are involved in coding for the kinematics of the movement (Iacoboni et al., 1999). Subsequent research yielded a number of interesting facts about the mirror system and extended its functional properties to accounts of imitation (Iacoboni, 2005), complementary joint action (Newman-Norland et al., 2007), empathy (Gallese, 2001), and deficits associated with autism spectrum disorder (Sebanz et al., 2005).
The discovery of the mirror system fit nicely with concurrent theoretical work proposing that action observation and execution share a common representational domain (Prinz, 1990, 1997; Hommel et al., 2001). That is, via activation of mirror systems, observation of a co-actor's behavior engages the same representational structures necessary to produce similar or complementary behaviors. This “common-coding” (i.e., representational equivalence) between action observation and execution constitutes an inherent linkage between co-actors during social interactions. Hence, these neural structures are considered the mechanism supporting covert motor simulations (Graf et al., 2009), allowing co-actors to “co-represent” a common task, infer, and understand the action goals of their partners, predict future behaviors, and establish coordination during joint actions (Knoblich and Sebanz, 2006; Sebanz and Knoblich, 2009).
Although it remains unclear as to how mirror systems perform these social-cognitive functions, several plausible models are possible. For instance, Kilner and colleagues (2007a) propose that the human mirror system, including the STS and both parietal and frontal mirror neuron centers, comprises a representational hierarchy capable of processing equivalent to empirical Bayesian inference. This account suggests reciprocal couplings between levels of the hierarchy, which represent kinematics of the observed movement (STS), motor commands which gave rise to the observed movements (parietal mirror neurons), and action goals which gave rise to those motor commands (frontal mirror neurons), respectively. Generative processing gives rise to predictions of motor commands given a goal, and likewise, predictions of observed kinematics given motor commands. Whereas backward connections convey predictions from higher to lower levels, forward connections convey errors in prediction from lower to higher levels. Predicted kinematics can be compared to the observed kinematics and the error in prediction can then be used to adjust higher-level representations. By minimizing error across this reciprocal processing, an observer can, therefore, derive the most likely goal of an observed action.
In addition to support from neuroscience, a number of behavioral studies have also yielded evidence consistent with common-coding theories of joint action (Brass et al., 2001; Kilner et al., 2003, 2007b; Sebanz et al., 2003, 2005; Vogt et al., 2003; Press et al., 2005; Stanley et al., 2007). Generally, these studies reveal action-observation effects in which the observation of a co-actor's behaviors, or knowledge of the co-actors task, affects the production of one's own action. For instance, Press et al. (2005) had participants produce a hand-opening gesture in response to a visual stimulus. They found slower reaction times when the stimuli depicted a hand in an incongruent configuration (i.e., closed) than in a congruent configuration (i.e., open). These data are consistent with a common-coding account in that the observation of an incongruent behavior (i.e., a closed hand) activates representations that conflict with the motor commands planned for execution (i.e., opening the hand). The resolution of this conflict is expressed in longer reaction times.
In a similar example with later relevance, Kilner et al. (2003, also see Stanley et al., 2007) had participants produce rhythmic arm movements in the horizontal plane while watching the movements of a confederate. Participants produced greater variability in the uninstructed plane of their movements (i.e., more vertical movement variability) when confederate movements were in the incongruent plane (i.e., vertical movements of the confederate). Again, these “motor contagion” effects (Blakemore and Frith, 2005) are consistent with the theory of common-coding and the known properties of mirror systems.
This evidence from both neurological and behavioral research has garnered widespread support for a “neuromechanistic” account of social behavior (but see Jacob and Jeannerod, 2005; Dinstein et al., 2008; Hickok, 2009). In summary, this account holds that the activity of specific neural structures constitutes the causal organization of social interactions. To be sure, evidence in favor of an inherently social brain has considerably advanced scientific accounts of the inherently social nature of human mind and behavior. Without doubting the validity of the scientific findings offered in support of this account, there is reason to question whether a theory of social interaction should rely on these neural processes exclusively. Exclusive explanatory recourse to the activity of neural structures fails to appreciate the complete behavioral contexts in which these structures have emerged and in which they perform their functions. We contend that contextualizing these neural processes in the full set of embodied-embedded constraints on behavior will provide the basis for a grounded understanding of human social interaction.
In what follows we synthesize an argument made across several recent works (Marsh et al., 2009a; Richardson et al., 2010; Schmidt et al., 2011) that supports an alternative set of explanatory principles in approaching the organization of human behavior. Specifically, we argue that applying concepts from the dynamical systems framework (e.g., Kugler and Turvey, 1987; Kelso, 1995; Strogatz, 2003; Warren, 2006) to a theory of social interaction offers an opportunity to appropriately situate the activities of the nervous system in the context of a complete social system. Ultimately, we hope that these alternative theoretical principles can complement the recent advances in social-cognitive neuroscience, and thus provide new windows into understanding human social behavior.
Dynamics in Action
Juarrero (2000) begins her treatment of human behavior with a consideration of concepts of causality. She notes that the approach favored in traditional cognitive science has addressed the organization of behavior with the Newtonian notion of efficient (i.e., billiard-ball) causality to the exclusion of other conceptualizations of causal relationships (e.g., Aristotle's final, formal, and material causes). An exclusive reliance on efficient causality is reflected in an exclusive recourse to the causal powers of componential functions (i.e., neural mechanisms) to explain the order of behavioral states (e.g., walking, talking, playing chess). Although the notion of efficient cause-and-effect is a powerful explanatory tool, and necessarily part of any complete account, staunch reliance on efficient causality alone underlies many of the theoretical and philosophical issues associated with computational accounts to human behavior (e.g., Turvey and Shaw, 1979; Searle, 1980; Jordan, 1998; Juarrero, 2000).
The explanatory framework promoted by the dynamical systems approach operates under an alternative conceptualization of causality. Behavior is not understood as the “output” of efficient, mechanistic operations on “inputs.” Rather, behavior is said to self-organize across reciprocal relations between local componential interactions and the global behavioral state of the system (Haken, 1977/1983; Kugler et al., 1980; Kugler and Turvey, 1987; Thelen and Smith, 1994; Kelso, 1995; Strogatz, 2003; van Orden et al., 2003). The term self-organization is used to refer to patterns of behavioral order that emerge naturally from the free interplay of forces and mutual influences between components. That is, behavior is considered an emergent pattern that results from the balance of constraints that coordinate interactions of the systems components. Such patterns, and the nature of constraints that give rise to them, can be understood by studying the system's dynamics, or the lawful evolution of the system's behavior.
An introduction to these concepts is perhaps best accomplished through a well-known example of self-organization in a simple physical system, such as the Rayleigh–Benard instability (e.g., Kelso, 1995; van Orden et al., 2003). In this phenomenon a relatively thin layer of oil in a pan is heated from below. Applying heat from below creates a temperature differential between the hot oil at the bottom of the pan and the cool oil at the top. Within a range of temperature gradients, random collisions between the individual molecules of oil are sufficient to transfer the energy from the source of the heat at the bottom of the pan to the oil's surface where it can be dispersed. As the heat from below is increased, however, random collisions between the molecules no longer suffice to dispense the incoming energy. Past a certain critical point random motion of the oil molecules gives way to orderly “convection rolls”; currents that move the molecules from the bottom of the pan to the surface with adjacent rolls turning in opposite directions (i.e., clockwise vs. counter-clockwise).
Again, dynamical systems theory attempts to account for how behavior emerges as a result of coupling enforced on componential interactions by relevant constraints. In the example, the system components (i.e., oil molecules) are coordinated into a collective, orderly behavior (i.e., convection rolls) in accordance with thermodynamic constraints (i.e., properties affecting energy dissipation). All factors that affect the temperature gradient (e.g., viscosity of the oil, size of the pan, degree of heat applied) can be said to constrain system behavior. For instance, a given degree of heat applied might, or might not, be sufficient to produce convection roll behavior dependent on the viscosity of the oil, the size of the pan, or the coolness of the air above the pan. Thus, the balance of the relevant constraints determines which behavioral state the system will adopt (e.g., random motion vs. convection rolls), and manipulations of these constraints (e.g., changing the temperature differential) drive the system through its behavioral states.
To be clear, the dynamical systems approach does not assert that mechanical, efficient causal processes are inconsequential to an account of system behavior. Convection roll behavior certainly could not exist without physical interaction between oil molecules. What this approach contends is that efficient causal interactions between components are themselves subject to the constraints imposed at the level of the complete system. That is, global behavioral regularities are understood as the expression of interaction-dominants dynamics (see van Orden et al., 2003). Unlike a truly mechanical, component-dominant system (e.g., watch, engine, computer), in which behavior is the final output of efficient causal links between functionally independent mechanisms, an interaction-dominant system entails a functional interdependence between components. The activity of each component is dependent on the activity of the coupled components. Thus, the interaction itself is critical to an account of the observed behavior. Physical interactions of the oil molecules are constrained to a collective, synergetic structure by the thermodynamic forces imposed in the experimental preparation. It is in this sense that behavior is self-organized. Global behavioral order is the result of a dynamical balance between lawful processes that coordinate componential relationships. Accordingly, the dynamical systems approach aims to capture behavioral regularities at the level of the complete system and to understand how those behavioral regularities emerge, are sustained, and eventually destroyed, in the terms of interaction-dominant dynamics (e.g., van Orden et al., 2003; Kello et al., 2007; Kello and van Orden, 2009; Anderson et al., in press).
These theoretical aspirations are no different in the case of human behaviors; provide an account of how system components (e.g., limbs, dance partners, societies) are coupled to produce states of behavioral regularity (e.g., walking, waltzing, warring). Central to this account of human behavior is the concept of synergies or coordinative structures (e.g., Bernstein, 1967; Turvey et al., 1978; Kelso, 1984). A synergy is a temporarily assembled, task-specific, functional coupling between a system's componential degrees-of-freedom (see Kelso, 2009). Conceptually similar to the example of the convection roll behavior, the system's components are coupled such that they behave as a complete functional unit by virtue of the constraints inherent in the behavioral task. Just as oil molecules self-organize into convection rolls in accordance with thermodynamic constraints, the componential degrees-of-freedom of the human motor system (e.g., neurons, muscles, limbs) self-organize into synergetic units in accordance with the constraints imposed in the animal-environment relationship.
The concept of synergies has been thoroughly applied in the domain of motor control as a potential resolution to the need to coordinate a multiple-effector body in a complex environment to realize behavioral goals. For instance, Saltzman and Kelso (1987) state that motor degrees-of-freedom are coordinated to behave as synergies by the dynamical constraints of a given task. Organizing the motor system into synergetic structures greatly simplifies the problems of motor control. Once coordinated to behave as a functional unit, the individual degrees-of-freedom do not need to be controlled independently of one another, and perturbations applied to a component are automatically compensated by the coupled components (e.g., Kelso, 1984; Latash et al., 2002; Riley et al., 2011). Although the details are too involved for full review here, this kind of task-dynamic understanding also enables one to model how the motor system is organized into synergistic structures specifically tailored to the dynamics of a given task, such as reaching to grasp a cup and returning the cup to the mouth to drink (Saltzman and Kelso, 1987).
More recently, Warren (2006) has proposed an extension of these principles to explain how animals might control their relationship to their environment via perceptual information. Warren argues that the animal and the environment should be understood as componential systems and that the animal and the environment together comprise a behavioral synergy when coupled to one another through mechanical and informational constraints inherent in the particular task. That is, the relatively high-dimensional interactions that exist between an animal and its environment underlie the emergence of a relatively low-dimensional behavior. Under this conception, agents accomplish behavioral goals by learning the control laws that map physical forces and perceptual information to the dynamics of the action system. Accordingly, the adaptive human behavior can be modeled as emerging from the lawful interactions between perceptual and motor variables by using low-dimensional dynamical models (i.e., differential equations). For example, Warren and Fajen (2003, 2008) demonstrated how goal-directed paths of locomotion can emerge from properties of a person's action system and his or her surrounding environment. Using elementary dynamical components, such as dynamical (point) attractors to explain the paths toward a target and dynamical repellers to explain paths of obstacle avoidance, they modeled the self-organized emergence of rather complex aspects of locomotion, such as route selection and route switching.
Thus, these general explanatory principles of self-organization and emergence have been extended from dynamical systems theory and instantiated in several theories of human behavior, such as coordination dynamics (Kelso, 1995) and behavioral dynamics (Warren, 2006). Given the promising application of these theoretical principles to understanding how individual actors coordinate and control their behavior in their environments, several researchers have recently argued for a dynamical approach to human social behavior (Marsh et al., 2009; Schmidt et al., 2011). Although there is not yet a general dynamical theory of social behavior, the study of coordination dynamics (i.e., spatio-temporal entrainment) has provided a particularly interesting example of self-organizing processes in human social interaction in the case of interpersonal rhythmic coordination (e.g., Kelso, 1995; Marsh et al., 2006; Schmidt and Richardson, 2008).
Dynamics in Joint Action
Rhythmic coordination is a foundational aspect of behavior for organisms that propel themselves through their respective environments. von Holst (1937/1973) described the coordination between the fins of his Labrus fish with concepts such as “maintenance tendency” and “magnet effect” (see Amazeen et al., 1995). He observed that the patterns of coordination between fins seemed to be the result of a dynamic balance between “competition” (i.e., each fin maintains its own intrinsic frequency) and “cooperation” (i.e., fins move together). Later research by Kelso and his colleagues (Kelso, 1981, 1984; Haken et al., 1985; see Kelso, 1995) demonstrated that similar intrapersonal rhythmic limb coordination in humans could be explained using dynamical systems principles (for review, see Schmidt and Richardson, 2008).
Specifically, Kelso's (1981, 1984) experiments demonstrated that participants naturally produced only two forms of bimanual rhythmic coordination. In “inphase” coordination, the limbs were synchronized as they went through their respective oscillatory cycles. In the case of finger movements, this mode corresponds to when each finger reached the top of its swing upward, and the bottom of its swing downward, at the same time. In “antiphase” coordination, the limbs were offset from one another by a half-cycle (i.e., one finger up and one finger down simultaneously). Interestingly, inphase coordination is more stable (i.e., less variable) than antiphase coordination, and thus, can be performed across a broader range of frequencies. Indeed, participants instructed to produce antiphase coordination spontaneously transitioned to the inphase mode at faster movement frequencies (irrespective of movement frequency the opposite transition does not occur, people do not transition from inphase to antiphase coordination; see Schmidt and Richardson, 2008). Most interestingly, Haken et al. (1985) demonstrated that modeling “relative phase” as the collective variable for a system of coupled oscillators could capture these coordination dynamics in a simple and elegant manner.
Foregoing a complete technical description (see Pikovsky et al., 2001), the movement of an oscillatory limb at any point in its cycle can be described using the “phase angle” (θ) of its circular trajectory on the position-by-velocity phase space (see Figure 1). That is, the beginning of each rhythmic cycle (θ = 0°) corresponds to the limb being at a maximal, end-point position (e.g., finger completely up), but with zero velocity (e.g., no longer moving upward, and yet to move downward). As the limb moves through its rhythmic cycle, it speeds up and slows down to reach its opposite end-point position (e.g., finger completely down) which corresponds to the half-cycle (i.e., θ = 180°). The limb again speeds up and slows down, now in the opposite direction, to return to its starting position (i.e., θ = 360°/0°).
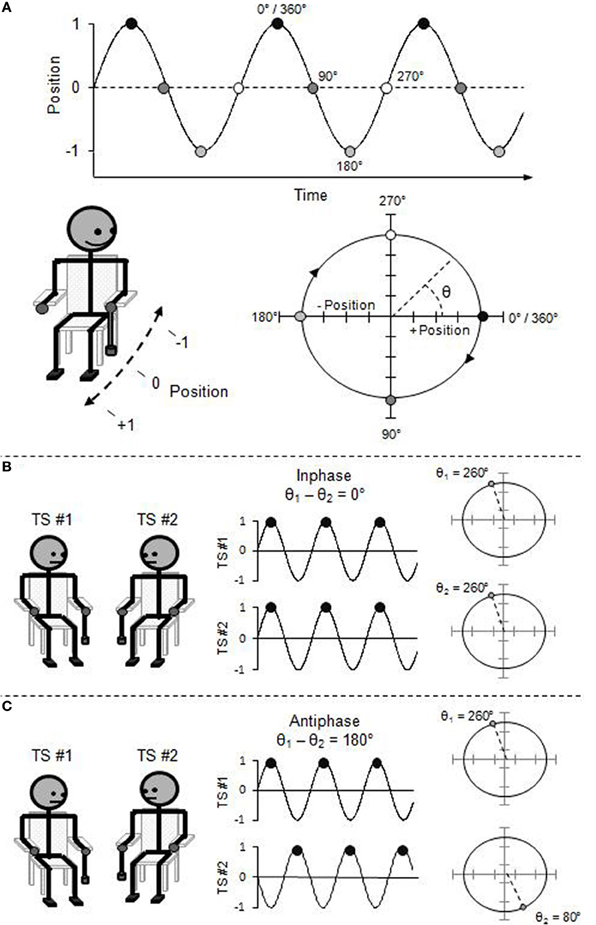
Figure 1. (A) Rhythmic pendulum swinging expressed in phase angle and represented as a position time-series and a position-by-velocity phase space. (B) Inphase interpersonal coordination expressed in relative phase angle and represented as a time-series and a phase pace. (C) Antiphase interpersonal coordination expressed in relative phase angle.
Thus, the “relative phase angle” (ϕ), which is simply the difference between the phase angle of two oscillators (ϕ = θ1 − θ2), captures the collective behavioral state of the system of coupled oscillators. Two oscillators coordinated in the inphase mode will be at the same phase angle of their respective cycles at the same time, and thus, the relative phase over time will be zero (i.e., ϕ = 0°). Oscillators coordinated in the antiphase mode will be in opposite phase angle of their respective cycles over time, and thus, the relative phase will be offset by a half-cycle (i.e., ϕ = 180°).
Kelso and colleagues (1990) extended the earlier model (Haken et al., 1985) in order to model relative phase dynamics as a function of two terms with striking similarity to von Holst's conception: the degree of coupling between the two oscillatory components (i.e., magnet effect) and the difference in the inherent frequency of each component (i.e., maintenance tendency).
This model Equation 1 captures the “competition” in the detuning term (Δω) as the simple difference in the intrinsic frequency of each oscillator (Δω = ω1 − ω2), while the “cooperation” between the two oscillators is captured by the sine functions (−a sinϕ − 2b sin2ϕ), with coupling strength indexed by b/a. The relative balance of these two constraints affects the system dynamics (see Schmidt and Richardson, 2008, for details).
In the language of synergetics (Haken, 1977/1983; see Schöner and Kelso, 1988), this model captures the lawful change in the collective state of the system, or “order parameter,” as a function of the systems relevant constraints, or “control parameters.” Manipulating either of the control parameters (i.e., coupling and detuning) gives rise to predictable changes in the dynamics of the order parameter (i.e., relative phase). Holding the coupling constant and varying the detuning term produces a “phase lag” in which the oscillator with the slower natural frequency lags slightly behind the other. Holding the detuning constant and varying the coupling affects the relative stability of the attractor states. At lower levels of coupling the antiphase attractor state becomes unstable, and only the inphase attractor remains.
The coordination dynamics proscribed by this model fit well with empirical observations of intrapersonal bimanual coordination (see Kelso, 1995). With respect to social behavior, research has provided ample evidence that the same coordination dynamics govern rhythmic behaviors between individuals (Schmidt et al., 1990, 1998; Amazeen et al., 1995; Richardson et al., 2007). Pairs of participants intentionally coordinating rhythmic limb movements are naturally constrained (i.e., without practice) to the inphase and antiphase modes, and exhibit the same relative stabilities as in intrapersonal coordination (Schmidt et al., 1990, 1998; Schmidt and Turvey, 1994). Moreover, research has demonstrated that experimental manipulations of the detuning and coupling parameters yield results consistent with Equation 1. (e.g., Schmidt and Turvey, 1994; Amazeen et al., 1995; Richardson et al., 2007). For instance, having participants swing pendulums with different natural frequencies produces the expected phase lag in the relative phase between participants (Amazeen et al., 1995). Similarly, manipulations of the strength of visual coupling, such as decreasing attention (Temprado and Laurent, 2004), the degree of visual tracking (Schmidt et al., 2007), or the amplitude of the observed movement (Varlet et al., in press), all reduce the stability of coordination.
The same coordination dynamics are evident even in spontaneous (i.e., uninstructed) coordination between interacting individuals (e.g., Schmidt and O'Brien, 1997; Richardson et al., 2005, 2007; Oullier et al., 2008), and between an individual and an environmental stimulus (Schmidt et al., 2007; Lopresti-Goodman et al., 2008). For instance, Richardson et al. (2005) found that the same inphase/antiphase dynamic occurred between two people who were engaged in an interpersonal task, but not instructed to coordinate their movements. As in intentionally produced coordination, increasing the degree of visual coupling between participants is met with a predicted increase in coordination stability (Richardson et al., 2007). Additionally, these same dynamics appear to govern simultaneous states of coordination at interpersonal and intrapersonal scales of rhythmic activity (Coey et al., 2011). In a compelling example of synergetic coupling at the interpersonal scale, Harrison and Richardson (2009) have shown that pairs of participants can be coordinated to behave as single functional unit by virtue of simple mechanical constraints. In this study, participants walked and jogged while joined together (one behind the other) via a foam appendage. The leg movements of the pairs not only spontaneously became coordinated, but also exhibited a distinct preference for certain quadrapedal gait patterns (i.e., pace, trot), with differences in gait preference being a function of the differences in gait stability.
Research also suggests that these coordination dynamics might be applicable to the motor contagion effects mentioned previously. Recall, Kilner et al. (2003) found increased variability in the non-dominant plane of movement when participants observed a movement in the incongruent direction (e.g., swinging arm horizontally, confederate oscillating vertically). This additional movement in the uninstructed plane was interpreted as error induced in an executed motor commands brought on by observation of the incongruent movement. Alternatively, Richardson and colleagues (2009) demonstrated that the additional movement in the uninstructed plane is in fact rhythmically coordinated with the movements of the observed confederate. Rather than being extraneous and random “error,” the movements in the non-instructed plane contained coherent oscillations that were coordinated with the observed movement. Thus, these results suggest that this “interference” effect is best understood as the result of observers recruiting all available degrees-of-freedom (e.g., up-down movements) to successfully achieve the task goal and stabilize coordination with the observed movements.
Research has also demonstrated that these coordination dynamics are linked to other, higher-order aspects of human social interactions, such as rapport and social connectedness (e.g., Hove and Risen, 2009; Miles et al., 2009; Paladino et al., 2010; Miles et al., 2010a). For instance, Miles and colleagues (2009) found greater third-party ratings of rapport for stimuli depicting the naturally stable modes (i.e., inphase and antiphase) as compared to other coordination patterns. More recently, Miles et al. (2011) employed a minimal-group manipulation and found increased spontaneous coordination when participants observed the rhythmic movements of a confederate who was labeled as an out-group member. Furthermore, several studies have demonstrated that rhythmic coordination dynamics might also differ in conditions marked by social dysfunction (i.e., autism, Marsh et al., 2009; Isenhower et al., 2012; schizophrenia, Varlet et al., 2012).
At first blush, the finding that rhythmic coordination dynamics are the same between co-actors as they are within an individual actor might not seem to make an overwhelming contribution toward resolving the questions of modern social-cognitive neuroscience (Sebanz and Knoblich, 2009). Whereas the traditional questions concern how we might understand one another's goals and predict one another's future behaviors, these data seem to only speak to joint actions at the most base spatio-temporal level. Furthermore, a representational account of such coordination, based in cognitive timing simulations, is certainly not unthinkable (e.g., Semjen and Ivry, 2001; Ivry and Richardson, 2002), and arguably could even be based in the activity of mirror neuron systems (Sebanz and Knoblich, 2009).
What the study of coordination dynamics has provided, however, is considerable evidence that certain aspects of social interactions can be addressed in the language of self-organizing, dynamical processes. In brief, what matters for the organization of rhythmic interpersonal coordination is a coupling between two oscillatory components strong enough to overcome intrinsic differences (i.e., different natural frequencies). This coupling can be realized across neuromuscular linkages within a person (Kelso, 1984; Haken et al., 1985) or via informational linkages (i.e., visual, auditory, or haptic couplings) between two people (Schmidt et al., 1990; Repp and Penel, 2004; Lagarde and Kelso, 2006; Richardson et al., 2007; Harrison and Richardson, 2009; van der Wel et al., 2011). Thus, the findings of coordination dynamics research support the larger theoretical conclusion that behavioral regularity in joint actions can be understood as an emergent property of the lawful constraints that bind co-actors to behave as a unified, functional whole.
Dynamics in Dynamics
As mentioned at the outset of this article, the challenge to a theory of social interaction is the inherent “interrelatedness” of human behavior. As detailed above, the traditional explanatory framework in cognitive science has been extended to social interaction through inherently social neural structures (i.e., mirror neuron systems) and the effects these structures have on behavior (i.e., motor contagion). The evidence in favor of this representational account makes a persuasive case that these neurological mechanisms play a foundational role in supporting the cognitive functions necessary to sustain social interactions. In contrast, the dynamical approach has applied an alternative set of explanatory concepts to the understanding of joint action. As evidenced in the example of interpersonal rhythmic coordination, this general framework suggests that the behavioral regularities of joint actions can be understood as the result of synergetic coupling between co-actors enforced via mechanical and informational task constraints. Rather than assert theoretical superiority for either of these accounts, we hope to open a dialogue as to how these two frameworks might complement and inform one another. In line with the focus of this special issue, we begin this dialogue by suggesting that situating the activity of a social brain in the constraints inherent in real-time and online social interactions is necessary for a full appreciation of the nervous system's role in the organization of social behavior and cognition.
Consistent with the embodied-embedded perspective (e.g., Brooks, 1991; Beer, 1995; Hutchins, 1995; Clark, 1998; Gibbs, 2006), this proposal simply asserts that the constraints provided in the fact that social interactions exist between agents manifest in physical bodies and within a physical environment is critical to grounding social cognition. A common criticism of representational theories of human behavior is that they involve a “loan of intelligence” (e.g., Dennett, 1978; Turvey et al., 1981; Jordan, 1998). This framework likens the operation of the central nervous system to a computational machine (e.g., von Eckardt, 1993; Kawato, 1999); hence “representational mechanisms.” Although the efficient causal linkages from neural component to neural component may explain how sensory signals might be mechanistically processed to produce action commands, the claim that these processes are “understood” or “interpreted” by the agent leaves looming conceptual issues (Searle, 1980; Chalmers, 1996; Jordan, 1998). How is it that these physical brain processes can generate an experience of meaning? How can these meaningful experiences cause changes in physical processes to enact intentional behavior? Although the neural processes carried out by networks such as mirror systems are undoubtedly meaningfully tied to social behavior, the conceptual problem is the same for the representational account of social cognition. For instance, the predictive coding account (Kilner et al., 2007a) discussed previously details the efficient causal mechanism by which an observed behavior might be contrasted against a predictive model in order to reduce error in prediction. Although this account is perfectly sound in terms of the mechanistic processing it proposes, the question as to how an actor arrives at an understanding of co-actors goals remains unanswered.
We propose that theories of social interaction can begin to approach a solution to these conceptual issues by complementing the traditional account with the concepts of the dynamical systems approach, such as self-organization and interaction-dominant dynamics. To be certain, this proposal is neither meant to stand “against” the representational or neuro-cognitive approach, nor do we mean to imply that the questions traditionally addressed by cognitive research (e.g., thoughts, goals, intentions) are irrelevant to an account of social behavior. In the example of interpersonal rhythmic coordination, although the interacting participants achieve coordination via informational couplings, it is equally true that this coordination must be manifest in systems capable of detecting, and thereby coupling through, said perceptual information (Schmidt et al., 2011). Moreover, the participants' intentional states are equally important (Kloos and van Orden, 2010). An uncooperative participant, who chooses to attend to the geometrical patterns of the carpet rather than the movement patterns of their co-actor, will not yield rhythmic coordination. Thus, the conceptual approach proposed here is not intended to circumvent the importance of either neural structures or cognitive processes. What we do contend, however, is that social-cognitive processes, and the neural processes thought to underlie them, only gain full meaning when appropriately contextualized in the myriad of natural processes in which they have evolved and in which they are sustained.
As implied by the term “dynamics,” the explanatory goal of dynamical systems approach is to describe the necessary relationship (i.e., law) that dictates how the collective state of a system will change given the constraints imposed on its behavior. In the example of rhythmic coordination, the dynamical approach captures lawful behavioral regularity that generalizes across systems with radically different mechanical configurations. The same coordination dynamics are evident in systems comprised of a single human nervous system (i.e., intrapersonal coordination), multiple human nervous systems (i.e., interpersonal coordination), multiple “diminished” nervous systems (e.g., fireflies, Hanson, 1978; crickets, Walker, 1969), and no nervous systems at all (e.g., pendulum clocks, Huygens, 1673/1986). These dynamics characterize patterns of rhythmic coordination between oscillators whether the coordination is intended or spontaneous. Moreover, these coordination dynamics are evident both in the behavior of limb movements and in the patterns of neural activity that accompany such movements (e.g., Schöner and Kelso, 1988).
Thus, the dynamical approach manages to parsimoniously generalize across different systems precisely because its explanatory principles address the organization of behavior at the functional level of the system. A system of coupled oscillators obeys lawful coordination dynamics whether the oscillators are coupled to one another via neural linkages (e.g., human co-actors) or via physical vibrations (e.g., coupled metronomes). What is necessary is that the system components be sufficiently coupled and be able to freely interact. This does not imply that a system of coupled oscillators comprised of human participants is cognitively equivalent to a system of coupled metronomes, nor that all social interactions can be understood in terms of rhythmic coordination dynamics, but rather that both systems exhibit the same coordination dynamics because systems are subject to the same lawful constraints and share the same functional organization.
It is also important to reiterate that the dynamical systems approach is not solely concerned with observed macro-level behavior, but is equally interested in uncovering the dynamic stabilities of more micro-level neural activity (e.g., Tognoli et al., 2007; Lindenberger et al., 2009; Dumas et al., 2010). Of particular interest, given the current discussion, is research investigating neural processes involved in sensori-motor coordination (Jantzen and Kelso, 2007; Jantzen et al., 2009; Kelso, 2012). This research program has revealed that neural dynamics exhibit oscillatory patterns that can be examined and understood with the principles of coordination dynamics. For instance, Tognoli and colleagues (2007) instructed pairs of participants to produce rhythmic finger oscillations while fitted with electroencephalogram (EEG) recording equipment. A varying degree of spontaneous entrainment emerged between participants during conditions in which they could see one another's movements. A spectral analysis of the EEG data revealed a two-component peak in neural activity within a certain frequency band (9.2–11.5 Hz) that was specific to the degree of entrainment. Whereas the first component of this “phi complex” was associated with the degree of unsynchronized rhythmic movements, the second was associated with synchronization. Interestingly, the brain topography in the EEG data suggested that the brain structures associated with the human mirror neuron system might play a role in these phi dynamics, highlighting the possible relationships between neural and behavioral dynamics.
Again, the dynamical approach is not against the theoretical notion that the activities of the central nervous system are a meaningful and necessary part of human behavior. What the dynamical approach does suggest is a different conceptualization of these neural structures. Whereas the traditional approach conceptualizes these structures as “mechanisms,” the dynamical approach conceptualizes them as self-organizing synergies. That is, neural structures and their activities are considered to be part of the synergetic relationship by which an organism sustains its functional interactions with its environment. This claim holds that neural components are temporarily assembled into functional units by task-specific constraints (Kelso, 2009). This conception of neural activity seems to fit well with the findings suggesting that the same neural structures are involved in several different functional relationships, rather than having one context-independent function (i.e., neural reuse; see Anderson, 2010).
This conception offers an opportunity to situate the activities of the nervous system within the context of constraints at the level of the complete human behavioral system. At the most fundamental level, the dynamics of the natural environment provides a rich set of constraints on behavior. For instance, the physical sciences have revealed a set of lawful processes that govern the motion of physical bodies (i.e., the law of gravity) and transformations of energy (i.e., the laws of thermodynamics). These lawful processes lie behind the large-scale, long-term behavioral stabilities of all physical systems, including the body in which the nervous system has evolved. Similarly, the specific properties of the body (e.g., weights of limbs, joint linkages), in the context of the properties of the environment, yield natural, intrinsic bodily dynamics that constrain behaviors. These embodied-embedded constraints on human behavior provide fundamental sources of behavioral stability that are argued to underlie human cognition (e.g., van Dijk et al., 2008; Richardson et al., 2010; Schmidt et al., 2011). Kelso (2002) has proposed that the processes of self-organization in human behavior might also provide the critical foundation for consciousness and agency to emerge. Similarly, Jordan and Ghin (2006, 2007) have recently argued that self-organization and “contextual emergence” provide a theoretically grounded basis to understand intentionality, anticipation, and cognition. These authors suggest that the work of the micro-scale, componential interactions of the system (e.g., neural dynamics) are “naturally and necessarily about” the macro-scale, functional wholes in which they sustain themselves. Thus, these micro-scale activities are meaningful embodiments of the behavioral context in which they emerge. These embodiments constitute “virtual content” (i.e., intentions and goals) when the macro-scale behavioral wholes they are “about” involve prospective relationships to the environment and other organisms (e.g., predator-prey relations). Hence, the dynamical processes of embodiment in embedded self-sustaining systems constitute a natural, grounded basis for phenomenal meaning (i.e., contextual aboutness) that might not only underlie physical co-action or social movement coordination, but also more uniquely human, social behaviors (e.g., symbolic communication; see Streeck and Jordan, 2009).
Conclusion and Future Directions
So, what then is the role of the central nervous system in the organization of human social interactions? We do not proclaim to have the definitive answer to this question. Current trends in neuroscience suggest that certain neural networks underlie the social-cognitive abilities necessary to sustain social interactions. Ample evidence from both neurological and behavioral studies provides compelling reasons to accept that the activities of these neural structures are critical to a full account of social interaction. Accounts of social interactions relying exclusively on these neurological mechanisms, however, are likely to encounter the same philosophical issues inherent in representational approaches in general. Although efficient causal mechanisms can certainly accomplish their proposed computational processing, the question remains of how these computations ever give rise to the meaningful experiences we refer to when we claim to “know” the intention of our co-actors or are able to “predict” their future behavior.
Although these philosophical issues are not easily overcome, we propose that the explanatory concepts and descriptive tools of dynamical systems theory can serve as a complementary framework to the recent advances made in social-cognitive neuroscience. Rather than insist that either framework has superior explanatory access to the processes underlying human social interaction, we propose that the representational and dynamical approaches form a complementary pair (Pattee, 1978, 1982; Kelso and Enstrøm, 2006). As such, the contribution of the dynamical approach is to provide the set of concepts by which the neural “mechanisms” involved in the organization of social behavior can be understood as emergent mechanisms; embodied reflections of lawful dynamics.
Central to accomplishing this contribution is, of course, providing empirical evidence of how such concepts can frame a general theory of social interactions. Although the previous investigations of periodic behaviors has provided evidence that the dynamical approach is applicable to the spatio-temporal organization of social actions, this alone does not constitute the foundation for a general theory. As Sebanz and Knoblich (2009) rightly insist, the dynamical approach must be empirically extended to accounts of non-periodic actions. To this end, future research should focus on investigating the behavioral dynamics of a broader range of everyday social activities, including more discrete, goal-directed, and complementary joint actions, and the time-evolving neural activity that takes place during such interaction.
Thankfully such research endeavors have already begun. For instance, several researchers have started to investigate the behavioral dynamics and coordination that occurs between individuals who are dancing together, telling jokes, or simply conversing (e.g., Boker and Rotondo, 2002; Shockley et al., 2003; Dale and Spivey, 2006; Sandamirskaya and Schöner, 2006; Schmidt et al., 2011). Others have begun to investigate coordination patterns in more goal-directed interaction tasks (e.g., Mottet et al., 2001; Newman-Norland et al., 2008), including how individual actors might couple in ways that give rise to functional roles that collectively stabilize group behavior (e.g., Goldstone et al., 2008; Richardson et al., 2011).
What is still needed, however, are specific investigations of what neural synergies emerge during these kinds of behaviors and how the time-evolving behavior of these neural synergies is specific to the physical dynamics and constraints of a joint action task. We are excited at the prospect of what discoveries such studies may hold for understanding the role of the brain in shaping social behavior and cognition. We foresee that future research on the dynamics of social interaction will maintain that the neurological mechanisms promoted by social neuroscience are inherently and necessarily meaningful when appropriately situated and grounded in the myriad of natural constraints that shape behavioral order. In short, we believe that social neuroscience should be directed toward investigating how neural structures are functional embodiments of the self-organizing dynamics in which they have evolved and in which they exist, and how the activity of the nervous system is part of the synergistic animal-environment systems that sustain and support complex human behavior. This can be achieved by exploring the manner in which neural processes are entailed and modified by the dynamics of real-time social action and support the physical and informational couplings that self-organize perception, action, and cognition.
Conflict of Interest Statement
The authors declare that the research was conducted in the absence of any commercial or financial relationships that could be construed as a potential conflict of interest.
References
Amazeen, P. G., Schmidt, R. C., and Turvey, M. T. (1995). Frequency detuning of the phase entrainment dynamics of visually coupled rhythmic movements. Biol. Cybern. 72, 511–518.
Anderson, M. L. (2010). Neural reuse: a fundamental organizational principle of the brain. Behav. Brain Sci. 33, 245–313.
Anderson, M. L., Richardson, M. J., and Chemero, A. (in press). Eroding the boundaries of cognition: implications of embodiment. Top. Cogn. Sci.
Beer, R. (1995). A dynamical systems perspective on agent-environment interaction. Artif. Intell. 72, 173–215.
Bekkering, H., de Druijn, E. R. A., Cuijpers, R. H., Newman-Norland, R., van Schie, H. T., and Meulenbroek, R. (2009). Joint action: neurocognitive mechanisms supporting human interaction. Top. Cogn. Sci. 1, 340–352.
Blakemore, S. J., Boyer, P., Pachot-Clouard, M., Meltzoff, A., Segebarth, C., and Decety, J. (2003). The detection of contingency and animacy from simple animations in the human brain. Cereb. Coretx 13, 837–844.
Blakemore, S. J., and Frith, C. (2005). The role of motor contagion in the prediction of action. Neuropsychologia 43, 260–267.
Boker, S. M., and Rotondo, J. L. (2002). “Symmetry building and symmetry breaking in synchronized movement,” in Mirror Neurons and the Evolution of Brain and Language, eds M. I. Stamenov and V. Gallese (Phildelphia: Benjamins), 163–171.
Bonda, E., Petrides, M., Ostry, D., and Evans, A. (1996). Specific involvement of human parietal systems and the amygdala in the perception of biological motion. J. Neurosci. 16, 3737–3744.
Brass, M., Bekkering, H., and Prinz, W. (2001). Movement observation affects movement execution in a simple response task. Acta Psychol. (Amst.) 106, 3–22.
Chalmers, D. J. (1996). The Conscious Mind: In Search of a Fundamental Theory. New York, NY: Oxford University Press.
Clark, A. (1998). “Embodiment and the philosophy of mind,” in Current Issues in Philosophy of Mind: Royal Institute of Philosophy Supplement, ed A. O'Hear (Cambridge: Cambridge University Press), 35–52.
Coey, C. A., Varlet, M., Schmidt, R. C., and Richardson, M. J. (2011). Effects of movement stability and congruency on the emergence of spontaneous interpersonal coordination. Exp. Brain Res. 211, 483–493.
Dale, R., and Spivey, M. J. (2006). Unraveling the dyad: using recurrence analysis to explore patterns of pyntactic coordination between children and caregivers in conversation. Lang. Learn. 56, 391–430.
Decety, J., Chaminade, T., Grezes, J., and Meltzoff, A. N. (2002). A PET exploration of the neural mechanisms involved in reciprocal imitation. Neuroimage 15, 265–272.
di Pellegrino, G., Fadiga, L., Fogassi, L., Gallese, V., and Rizzolatti, G. (1992). Understanding motor events: a neurophysiological study. Exp. Brain Res. 91, 176–180.
Dinstein, I., Thomas, C., Behrmann, M., and Heeger, D. J. (2008). A mirror up to nature. Curr. Biol. 18, R13–R18.
Dumas, G., Nadel, J., Soussignan, R., Martinerie, J., and Garnero, L. (2010). Inter-brain synchronization during social interaction. PLoS ONE 5:e12166. doi: 10.1371/journal.pone.0012166
Fadiga, L., Fogassi, L., Pavesi, G., and Rizzolatti, G. (1995). Motor facilitation during action observation: a magnetic stimulation study. J. Neurophysiol. 73, 2608–2611.
Fajen, B. R., and Warren, W. H. (2003). Behavioral dynamics of steering, obstacle avoidance, and route selection. J. Exp. Psychol. Hum. Percept. Perform. 29, 343–362.
Gallese, V. (2001). The ‘shared manifold’ hypothesis: from mirror neurons to empathy. J. Conscious. Stud. 8, 33–50.
Gallese, V. (2003). The roots of empathy: the shared manifold hypothesis and the neural basis of intersubjectivity. Psychopathology 36, 171–180.
Gallese, V., Fadiga, L., Fogassi, L., and Rizzolatti, G. (1996). Action recognition in the premotor cortex. Brain 119, 593–609.
Goldstone, R. L., Roberts, M. E., and Gureckis, T. M. (2008). Emergent processes in group behavior. Curr. Dir. Psychol. Sci. 17, 10–15.
Graf, M., Schutz-Bosbach, S., and Prinz, W. (2009). “Motor involvement in action and object perception,” in Grounding Sociality: Neurons, Mind, and Culture, eds G. R. Semin and G. Echterhoff (New York, NY: Psychology Press), 17–45.
Grafton, S. T., and Hamilton, A. F. (2007). Evidence for a distributed hierarchy of action representation in the brain. Hum. Mov. Sci. 26, 590–616.
Grossman, E. D., and Blake, R. (2000). Brain areas involved in perception of biological motion. J. Cogn. Neurosci. 12, 711–720.
Haken, H., Kelso, J. A. S., and Bunz, H. (1985). A theoretical model of phase transitions in human hand movements. Biol. Cybern. 51, 347–356.
Harrison, S. J., and Richardson, M. J. (2009). Horsing around: spontaneous four-legged coordination. J. Mot. Behav. 41, 519–524.
Hickok, G. (2009). Eight problems for the mirror neuron theory of action understanding in monkeys and humans. J. Cogn. Neurosci. 21, 1229–1243.
Hommel, B., Musseler, J., Aschersleben, G., and Prinz, W. (2001). The theory of event coding: a framework for perception and action planning. Behav. Brain Sci. 24, 849–937.
Hove, M. J., and Risen, J. L. (2009). It's all in the timing: interpersonal synchrony increases affiliation. Soc. Cogn. 27, 949–960.
Huygens, C. (1673/1986). The Pendulum Clock or Geometrical Demonstrations Concerning the Motion of Pendula as Applied to Clocks. Trans. R. J. Blackwell, Ames: Iowa State University Press.
Iacoboni, M., Woods, R. P., Brass, M., Bekkering, H., Mazziotta, J. C., and Rizzolatti, G. (1999). Cortical mechanisms of human imitation. Science 286, 256–2528.
Isenhower, R. W., Marsh, K. L., Richardson, M. J., Helt, M., Schmidt, R. C., and Fein, D. (2012). Rhythmic bimanual coordination is impaired in children with autism spectrum disorder. Res. Autism Spectr. Disord. 6, 25–31.
Ivry, R. B., and Richardson, T. C. (2002). Temporal control and coordination: the multiple timer model. Brain Cogn. 48, 117–132.
Jacob, P., and Jeannerod, M. (2005). The motor theory of social cognition: a critique. Trends Cogn. Sci. 9, 21–25.
Jantzen, K., and Kelso, J. A. S. (2007). “Neural coordination dynamics: a review,” in Handbook of Brain Connectivity, eds V. K. Jirsa and A. R. McIntosh (Heidelberg: Springer-Verlag), 1–39.
Jantzen, K., Steinberg, F. L., and Kelso, J. A. S. (2009). Coordination dynamics of large-scale neural circuitry underlying rhythmic sensorimotor behavior. J. Cogn. Neurosci. 21, 2420–2433.
Jordan, J. S. (1998). “Intentionality, perception, and autocatalytic closure: a potential means of repaying psychology's conceptual debt,” in Systems Theories and A Priori Aspects of Perception, eds J. S. Jordan (Amsterdam: North-Holland), 181–208.
Jordan, J. S., and Ghin, M. (2006). Proto-consciousness as a contextually emergent property of self-sustaining systems. Mind Matter 4, 45–68.
Jordan, J. S., and Ghin, M. (2007). The role of control in a science of consciousness: causality, regulation, and self-sustainment. J. Conscious. Stud. 14, 177–197.
Kawato, M. (1999). Internal models for motor control and trajectory planning. Curr. Opin. Neurobiol. 9, 718–727.
Kello, C. T., and van Orden, G. (2009). Soft-assembly of sensorimotor function. Nonlinear Dynamics Psychol. Life Sci. 13, 57–78.
Kello, C. T., Beltz, B. C., Holden, J. G., and van Orden, G. (2007). The emergent coordination of cognitive function. J. Exp. Psychol. Gen. 136, 551–568.
Kelso, J. A. S. (1984). Phase transitions and critical behavior in human bimanual coordination. Am. J. Physiol. 246, R1000–R1004.
Kelso, J. A. S. (2002). The complementary nature of coordination dynamics: self-organization and agency. Nonlinear Phenom. Complex Sys. 5, 364–371.
Kelso, J. A. S. (2009). “Synergies: atoms of brain and behavior,” in Progress in Motor Control, ed D. Sternad (New York, NY: Springer), 83–91.
Kelso, J. A. S. (2012). Multistability and metastability: understanding dynamics coordination in the brain. Philos. Trans. R. Soc. Lond. B Biol. Sci. 367, 906–918.
Kelso, J. A. S., Delcolle, S., and Schöner, G. (1990). “Action-perception as a pattern formation process,” in Attention and Performance XIII: Motor Representations and Control, ed M. Jeannerod (New Jersey, NJ: Hillsdale), 139–169.
Kilner, J. M., Friston, K. J., and Frith, C. D. (2007a). Predictive coding: an account of the mirror neuron system. Cogn. Process. 8, 159–166.
Kilner, J. M., Hamilton, A. F., and Blakemore, S. J. (2007b). Interference effect of observed human movement on action is due to velocity profile of biological motion. Soc. Neurosci. 2, 158–166.
Kilner, J. M., Paulignan, Y., and Blakemore, S. J. (2003). An interference effect of observed biological movement on action. Curr. Biol. 13, 522–525.
Kloos, H., and van Orden, G. (2010). Voluntary behavior in cognitive and motor tasks. Mind Matter 8, 19–43.
Knoblich, G., and Sebanz, N. (2006). The social nature of perception and action. Curr. Dir. Psychol. Sci. 15, 99–104.
Kugler, P. N., and Turvey, M. T. (1987). Information, Natural Law, and the Self-assembly of Rhythmic Movement. Hillsdale: Erlbaum.
Kugler, P. N., Kelso, J. A. S., and Turvey, M. T. (1980). “Coordinative structures as dissipative structure I. Theoretical lines of convergence,” in Tutorials in Motor Behavior, eds G. E. Stelmach and J. Requin (Amsterdam: North Holland), 5–47.
Lagarde, J., and Kelso, J. A. S. (2006). Binding of movement, sound and touch: multimodal coordination dynamics. Exp. Brain Res. 173, 673–688.
Latash, M. L., Scholz, J. P., and Schöner, G. (2002). Motor control stragies revealed in the structure of motor variability. Exerc. Sport Sci. Rev. 30, 26–31.
Lindenberger, U., Li, S., Gruber, W., and Muller, V. (2009). Brains swinging in concert: cortical phase synchronization while playing guitar. BMC Neurosci. 10. doi: 10.1186/1471-2202-10-22
Lopresti-Goodman, S. M., Richardson, M. J., Silva, P., and Schmidt, R. C. (2008). Period basin of entrainment for unintentional visual coordination. J. Mot. Behav. 40, 3–10.
Marsh, K. L., Richardson, M. J., Baron, R. M., and Schmidt, R. C. (2006). Contrasting approaches to perceiving and acting with others. Ecol. Psychol. 18, 1–38.
Marsh, K. L., Richardson, M. J., and Schmidt, R. C. (2009). Social connection through joint action and interpersonal coordination. Top. Cogn. Sci. 1, 320–339.
Marsh, K. L., Johnston, L., Richardson, M. J., and Schmidt, R. C. (2009a). Toward a radically embodied, embedded social psychology. Eur. J. Soc. Psychol. 39, 1217–1225.
Miles, L. K., Nind, L. K., and Macrae, C. N. (2009). The rhythm of rapport: interpersonal synchrony and social perception. J. Exp. Soc. Psychol. 45, 585–589.
Miles, L. K., Lumsden, J., Richardson, M. J., and Macrae, C. N. (2011). Do birds of a feather move together? Group membership and behavioral synchrony. Exp. Brain Res. 211, 495–503.
Miles, L. K., Griffiths, J. L., Richardson, M. J., and Macrae, C. N. (2010a). Too late to coordinate: contextual influences on behavioral synchrony. Eur. J. Soc. Psychol. 40, 52–60.
Mottet, D., Guiard, Y., Ferrand, T., and Bootsma, R. J. (2001). Two-handed performance of a rhythmic Fitts task by individuals and dyads. J. Exp. Psychol. Hum. Percept. Perform. 27, 1275–1286.
Newman-Norland, R. D., Bosga, J., Meulenbroek, R. G. J., and Bekkering, H. (2008). Anatomical substrates of cooperative joint-action in a continuous motor task: virtual lifting and balancing. Neuroimage 41, 169–177.
Newman-Norland, R. D., Noordzij, M. L., Meulenbroek, R. G., and Bekkering, H. (2007). Exploring the brain basis of joint action: co-ordination of actions, goals, and intentions. Soc. Neurosci. 2, 48–65.
Oullier, O., de Guzman, G. C., Jantzen, K. J., Lagarde, J., and Kelso, J. A. S. (2008). Social coordination dynamics: measuring human bonding. Soc. Neurosci. 3, 178–192.
Pattee, H. H. (1978). The complementarity principle in biological and social structures. J. Soc. Biol. Struct. 1, 191–200.
Pattee, H. H. (1982). “The need for complementarity in models of cognitive behavior: a response to Fowler and Turvey,” in Cognition and the Symbolic Process, eds W. B. Weimer and D. S. Palermo (Hillsdale, NJ: Erlbaum), 21–34.
Paladino, M. P., Muzzurega, M., Pavani, F., and Schubert, T. W. (2010). Synchronous multisensory stimulation blurs self-other boundaries. Psychol. Sci. 21, 1202–1207.
Pikovsky, A., Rosenblum, M., and Kurths, J. (2001). Synchronization: A Universal Concept in Nonlinear Sciences. New York, NY: Cambridge University Press.
Press, C., Bird, G., Flach, R., and Heyes, C. (2005). Robotic movement elicits automatic imitation. Brain Res. Cogn. Brain Res. 25, 632–640.
Prinz, W. (1990). “A common coding approach to perception and action,” in Cognition and Motor Processes, eds O. Neumann and W. Prinz (Berlin: Springer), 167–201.
Puce, A., and Perrett, D. (2003). Electrophysiology and brain imaging of biological motion. Philos. Trans. R. Soc. Lond. B Biol. Sci. 358, 435–445.
Repp, B. H., and Penel, A. (2004). Rhythmic movement is attracted more stronlgly to auditory than visual rhythms. Psychol. Res. 68, 252–270.
Richardson, M. J., Harrison, S. J., May, R., Kallen, R. W., and Schmidt, R. C. (2011). Self-organized complementary coordination: dynamics of an interpersonal collision-avoidance task. BIO Web Conf. doi: 10.1051/bioconf/20110100075. [Epub ahead of print].
Richardson, M. J., Marsh, K. L., and Schmidt, R. C. (2005). Effects of visual and verbal interaction on unintentional interpersonal coordination. J. Exp. Psychol. Hum. Percept. Perform. 31, 62–79.
Richardson, M. J., Marsh, K. L., Isenhower, R. W., Goodman, J. R. L., and Schmidt, R. C. (2007). Rocking together: dynamics of intentional and unintentional interpersonal coordination. Hum. Mov. Sci. 26, 867–891.
Richardson, M. J., Lopresti-Goodman, S., Mancini, M., Kay, B., and Schmidt, R. C. (2008). Comparing the attractor strength of intra- and interpersonal interlimb coordination using cross-recurrence analysis. Neurosci. Lett. 438, 340–345.
Richardson, M. J., Marsh, K. L., and Schmidt, R. C. (2010). “Challenging the egocentric view of coordinated perceiving, acting, and knowing,” in The Mind in Context, eds B. Mesquita, L. F. Barrett, and E. R. Smith (New York, NY: The Guilford Press), 307–333.
Richardson, M. J., Campbell, W. L., and Schmidt, R. C. (2009). Movement interference during action observation as emergent coordination. Neurosci. Lett. 449, 117–122.
Riley, M. A., Richardson, M. J., Shockley, K., and Ramenzoni, V. C. (2011). Interpersonal synergies. Front. Psychol. 2, 1–7. doi: 10.3389/fpsyg.2011.00038
Rizzolatti, G., and Craighero, L. (2004). The mirror-neuron system. Annu. Rev. Neurosci. 27, 169–192.
Rizzolatti, G., Fadiga, L., Gallese, V., and Fogassi, L. (1996a). Premotor cortex and the recognition of motor actions. Brain Res. Cogn. Brain Res. 3, 131–141.
Rizzolatti, G., Fadiga, L., Matelli, M., Bettinardi, V., Paulesu, E., Perani, D., and Fazio, F. (1996b). Localization of grasp representation in humans by PET: observation versus execution. Exp. Brain Res. 111, 246–252.
Rizzolatti, G., and Sinigaglia, C. (2010). The functional role of the parieto-frontal mirror circuit: interpretations and misinterpretations. Nat. Rev. Neurosci. 11, 264–274.
Saltzman, E. L., and Kelso, J. A. S. (1987). Skilled actions: a task-dynamics approach. Psychol. Rev. 94, 84–106.
Saltzman, E. L., and Munhall, K. G. (1992). Skill acquisition and development: the roles of state-, parameter-, and graph-dynamics. J. Mot. Behav. 24, 49–57.
Sandamirskaya, Y., and Schöner, G. (2006). “Dynamic field theory and embodied communication,” in Lecture Notes in Artificial Intelligence: Modeling Communication with Robots and Virtual Humans, eds I. Wachsmuth and G. Knoblich (Berlin: Springer), 260–278.
Schmidt, R. C., and O'Brien, B. (1997). Evaluating the dynamics of unintended interpersonal coordination. Ecol. Psychol. 9, 189–206.
Schmidt, R. C., and Richardson, M. J. (2008). “Dynamics of interpersonal coordination,” in Coordination: Neural, Behavioral, and Social Dynamics, eds A. Fuchs and V. K. Jirsa (Heidelberg: Springer), 281–308.
Schmidt, R. C., and Turvey, M. T. (1994). Phase entrainment dynamics of visually coupled rhythmic movements. Biol. Cybern. 70, 369–376.
Schmidt, R. C., Bienvenu, M., Fitzpatrick, P. A., and Amazeen, P. G. (1998). A comparison of intra- and interpersonal interlimb coordination: coordination breakdowns and coupling strength. J. Exp. Psychol. Hum. Percept. Perform. 24, 884–900.
Schmidt, R. C., Carello, C., and Turvey, M. T. (1990). Phase transitions and critical fluctuations in the visual coordination of rhythmic movements between people. J. Exp. Psychol. Hum. Percept. Perform. 16, 227–247.
Schmidt, R. C., Richardson, M. J., Arsenault, C., and Galantucci, B. (2007). Visual tracking and entrainment to an environmental rhythm. J. Exp. Psychol. Hum. Percept. Perform. 33, 860–870.
Schmidt, R. C., Fitzpatrick, P., Caron, R., and Mergeche, J. (2011). Understanding social motor coordination. Hum. Mov. Sci. 30, 834–845.
Schöner, G., and Kelso, J. A. S. (1988). Dynamic pattern generation in behavioral and neural systems. Science. 239, 1513–1520.
Schultz, J., Imamizu, H., Kawato, M., and Frith, C. (2004). Activation of the human superior temporal gyrus during observation of goal attribution by intentional objects. J. Cogn. Neurosci. 16, 1695–1705.
Schultz, J., Friston, K. J., O'Doherty, J., Wolpert, D. M., and Frith, C. (2005). Activation in posterior superior temporal sulcus parallels parameter inducing the percept of animacy. Neuron 45, 625–635.
Sebanz, N., and Knoblich, G. (2009). Prediction in joint action: what, when, and where. Top. Cogn. Sci. 1, 353–367.
Sebanz, N., Knoblich, G., and Prinz, W. (2003). Representing others' actions: just like one's own? Cognition 88, B11–B21.
Sebanz, N., Knoblich, G., and Prinz, W. (2005). How two share a task: corpresenting stimulus-response mappings. J. Exp. Psychol. Hum. Percept. Perform. 31, 1234–1246.
Semjen, A., and Ivry, R. B. (2001). The coupled oscillator model of between-hand coordination in alternate-hand tapping: a reappraisal. J. Exp. Psychol. Hum. Percept. Perform. 25, 251–265.
Shockley, K., Santana, M., and Fowler, C. A. (2003). Mutual interpersonal postural constraints are involved in cooperative conversation. J. Exp. Psychol. Hum. Percept. Perform. 29, 326–332.
Stanley, J., Gowen, E., and Miall, R. C. (2007). Effects of agency on movement interference during observation of a moving dot stimulus. J. Exp. Psychol. Hum. Percept. Perform. 33, 915–926.
Streeck, J., and Jordan, J. S. (2009). Projection and anticipation: the forward-looking nature of embodied communication. Discourse Process. 46, 93–102.
Temprado, J. J., and Laurent, M. (2004). Attentional load associated with performing and stabilizing a between-persons coordination of rhythmic limb movements. Acta Psychol. (Amst.) 115, 1–16.
Thelen, E., and Smith, L. B. (1994). A Dynamics Systems Approach to the Development of Perception and Action. Cambridge, MA: MIT Press.
Tognoli, E., Lagarde, J., de Guzman, G. C., and Kelso, J. A. S. (2007). The phi complex as a neuromaker of human social coordination. Proc. Natl. Acad. Sci. U.S.A. 104, 8190–8195.
Turvey, M. T., and Shaw, R. E. (1979). “The primacy of perceiving: an ecological reformulation of perception for understanding memory,” in Perspcectives on Memory Research: Essays in Honor of Uppsala University's 500th Aniversary, ed L. G. Nillson (Hillsdale: Erlbaum), 167–222.
Turvey, M. T., Shaw, R. E., and Mace, W. M. (1978). “Issues in the theory of action: degrees of freedom, coordinative structures, and coalitions,” in Attention and Performance VII, ed J. Requin (Hillsdale: Erlbaum), 557–595.
Turvey, M. T., Shaw, R. E., and Mace, W. M. (1981). Ecological laws of perceiving and acting: in reply to Fodor and Pylyshn. Cognition 9, 237–304.
van der Wel, R. P. D., Knoblich, G., and Sebanz, N. (2011). Let the force be with us: dyads exploit haptic coupling for coordination. J. Exp. Psychol. Hum. Percept. Perform. 37, 1420–1431.
van Dijk, J., Kerkhofs, R., van Rooij, I., and Haselager, P. (2008). Can these be such a thing as embodied embedded cognitive neuroscience? Theory Psychol. 18, 297–316.
van Orden, G., Holden, J. G., and Turvey, M. T. (2003). Self-organization of cognitive performance. J. Exp. Psychol. Gen. 132, 331–350.
Varlet, M., Marin, L., Raffard, S., Schmidt, R. C., Capdevielle, D., Boulenger, P., Del_Monte, J., and Bardy, B. G. (2012). Impairments of interpersonal coordination in schizophrenia. PLoS ONE, 7:e29772. doi: 10.1371/journal.pone.0029772
Varlet, M., Coey, C. A., Schmidt, R. C., and Richardson, M. J. (in press). Influence of stimulus amplitude on unintended visuomotor entrainment. Hum. Mov. Sci.
Vogt, S., Taylor, P., and Hopkins, B. (2003). Visuomotor priming by picture of hand postures: perspective matters. Neuropsychologia 41, 941–951.
von Holst, E. (1937/1973). “On the nature and order of the central nervous system,” in The Collected Papers of Erich von Holst Vol. 1, The Behavioral Physiology of Animal and Man, ed R. Martin (Coral Gables: University of Miami Press), 133–155.
Walker, T. J. (1969). Acoustic synchrony: two mechanisms in the snowy tree cricket. Science 166, 891–894.
Keywords: neuroscience, joint action, dynamics, embodiment, rhythmic coordination
Citation: Coey CA, Varlet M and Richardson MJ (2012) Coordination dynamics in a socially situated nervous system. Front. Hum. Neurosci. 6:164. doi: 10.3389/fnhum.2012.00164
Received: 02 February 2012; Accepted: 22 May 2012;
Published online: 07 June 2012.
Edited by:
Chris Frith, Wellcome Trust Centre for Neuroimaging at University College London, UKReviewed by:
Harold Bekkering, University of Nijmegen, NetherlandsScott Kelso, Florida Atlantic University, USA
Wolfgang Prinz, Max Planck Institute for Cognitive and Brain Sciences, Germany
Copyright: © 2012 Coey, Varlet and Richardson. This is an open-access article distributed under the terms of the Creative Commons Attribution Non Commercial License, which permits non-commercial use, distribution, and reproduction in other forums, provided the original authors and source are credited.
*Correspondence: Charles A. Coey, Department of Psychology, Perceptual-Motor Dynamics Laboratory, CAP Center for Cognition, Action and Perception, University of Cincinnati, ML 0376, 4150-B Edwards C1, Cincinnati, OH 45221-0376, USA. e-mail: coeyca@mail.uc.edu