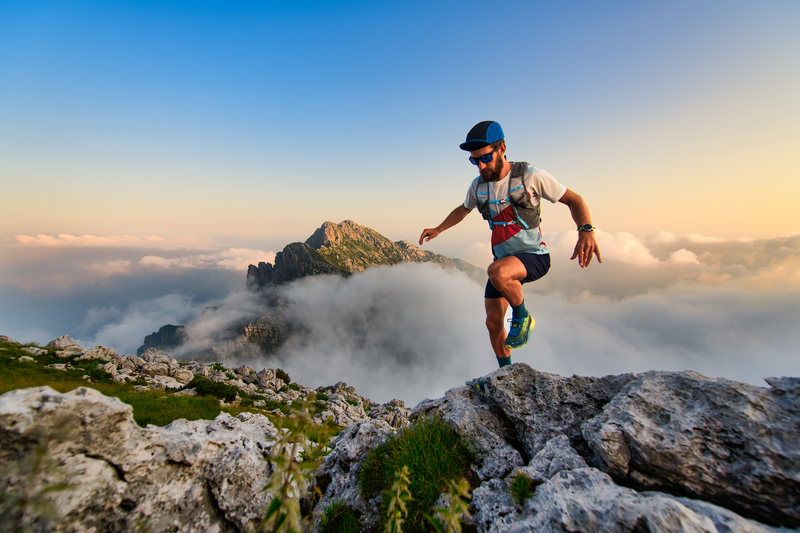
95% of researchers rate our articles as excellent or good
Learn more about the work of our research integrity team to safeguard the quality of each article we publish.
Find out more
ORIGINAL RESEARCH article
Front. Hum. Neurosci. , 07 June 2012
Sec. Brain Imaging and Stimulation
Volume 6 - 2012 | https://doi.org/10.3389/fnhum.2012.00152
This article is part of the Research Topic Cortico-cortical connectivity and memory: function and dysfunction View all 11 articles
In recent years, several theories have been proposed in attempts to identify the neural mechanisms underlying successful cognitive aging. Old subjects show increased neural activity during the performance of tasks, mainly in prefrontal areas, which is interpreted as a compensatory mechanism linked to functional brain efficiency. Moreover, resting-state studies have concluded that elders show disconnection or disruption of large-scale functional networks. We used functional MRI during resting-state and a verbal n-back task with different levels of memory load in a cohort of young and old healthy adults to identify patterns of networks associated with working memory and brain default mode. We found that the disruption of resting-state networks in the elderly coexists with task-related overactivations of certain brain areas and with reorganizations within these functional networks. Moreover, elders who were able to activate additional areas and to recruit a more bilateral frontal pattern within the task-related network achieved successful performance on the task. We concluded that the balanced and plastic reorganization of brain networks underlies successful cognitive aging. This observation allows the integration of several theories that have been proposed to date regarding the aging brain.
Cognitive aging affects a wide range of functions including working memory, processing speed, and inhibitory function (Park et al., 2002; Reuter-Lorenz and Park, 2010). Despite the gradual decline described in aging, some seniors are able to keep their cognitive functions with minimal differences in performance compared to healthy young subjects. Several theories have been proposed in attempts to identify the neural correlates of what is known as “successful cognitive aging” (Cabeza et al., 2002a; Park and Reuter-Lorenz, 2009).
Functional imaging is well suited to the study of changes in brain functionality in advanced age (for a review, see Eyler et al., 2011). Across various cognitive domains, age-related functional reorganizations have been described as changes in brain responsivity in several brain regions when subjects are scanned during the performance of cognitively demanding tasks (see Spreng et al., 2010; Turner and Spreng, 2012 for recent meta-analyses). Hence, both reductions and increases in activity have been described in different brain regions. Reductions in activity are commonly located in the left prefrontal cortex (PFC) and temporo-occipital areas and are normally associated with less efficient processing in aging (Cabeza et al., 1997), but the interpretation of increases is less straightforward. However, when associated with better or preserved performance they have been commonly interpreted as evidence of functional compensatory mechanisms (Grady, 2000; Cabeza et al., 2002b; Grady et al., 2006; Mattay et al., 2006; Berlingeri et al., 2010).
Moreover, recent advances in neuroimaging techniques have made possible the study of functional brain networks that can be observed even during resting-state periods, revealing an intrinsic network-based organization of the brain (Smith et al., 2009). In this context, cognitive aging has been associated with disruptions/reorganizations within certain brain functional networks (Grady et al., 2010; Littow et al., 2010; Tomasi and Volkow, 2012). Particularly, areas forming part of the default-mode network (DMN), including the posterior cingulate cortex and middle frontal gyrus, are characterized by patterns of age-related decreases in functional connectivity (Damoiseaux et al., 2008; Hafkemeijer et al., 2012). These functional correlation reductions have been associated with cognitive decline across multiple domains in healthy old individuals (Andrews-Hanna et al., 2007).
Despite this evidence of changes in both brain activity and brain connectivity, very few studies have simultaneously investigated the DMN and a task-related brain network in aging in terms of their BOLD responsivity and functional connectivity, and during both resting and cognitive performance. Hence, the objective of our study was to investigate the brain connectivity/activity characteristics of the DMN and a working memory network in a sample of healthy elders (HE) and young adults (YA). In the present report, we placed special emphasis on investigating how these patterns differ between elders who are able to keep their working memory abilities at a level comparable to young subjects during the most demanding conditions, and those who show a decline in this function.
Twenty-nine HE (mean age: 62.55, SD: 9.43, 20 women) and 16 YA (mean age: 21.31, SD: 2.41, 9 women) were included in the study. Old subjects underwent neuropsychological testing, including memory, language, attention, and visuoperceptive/visuospatial functions. The neuropsychological battery was similar to the one used recently in other reports by our group (e.g., Arenaza-Urquijo et al., 2011). All reported scores were within normal range on the domains tested, and all subjects had scores on the mini-mental state examination ≥ 24 (mean: 28.83, SD: 1.64). All participants in the study were scanned using functional MRI (fMRI) in the resting-state and during the performance of a working memory task, an n-back task including different levels of working memory load (n = 0, 1, 2, and 3 letters to be retained: see Braver et al., 1997; Sala-Llonch et al., 2011). Basically, during the task, blocks of 0-, 1-, 2-, 3-back conditions lasting 26 s were presented four times each in a pseudo-randomized order with inter-block fixation periods (white cross on a black screen) of 13 s. Before any n-back block was presented, an instruction screen appeared to inform the subject about the task. Within each block, a sequence of 12 letters was presented in white in the center of the screen; each letter remained visible for 500 ms, with an inter-stimulus interval of 1500 ms. The subject was asked to press a button when the stimulus on the screen was the same as the one showed n items before. For the 0-back task, subjects were asked to press the button when the letter “X” appeared. All subjects underwent a training session before entering the scanner in order to ensure that they understood the task instructions. All achieved a task accuracy of at least 80%.
Subjects’ responses were collected and the performance of each n-back condition was calculated using the d′ measure (Z hit rate − Z false alarm rate), with higher d′ scores indicating higher performance. Mean reaction time (RT) was also collected for each subject within each load condition.
The HE group was further subdivided into low performers (low-HE) and high-performers (high-HE) according to the score obtained during the performance of the 3-back task (percentile 50 of the distribution). Between-group differences in d′ measures and RT measures were assessed with one-way ANOVA implemented in PASW vs. 17 (Statistical Package for Social Sciences, Chicago, IL, USA).
Functional MRI images were acquired on a 3T MRI scanner (Magnetom Trio Tim, Siemens Medical Systems, Germany), using a 32-channel coil. During both the resting-fMRI and task-fMRI conditions, a set of T2*-weighted volumes (150 and 336 volumes for resting and task fMRI, respectively) were acquired (TR = 2000 ms, TE = 29 ms, 36 slices per volume, slice thickness = 3 mm, distance factor = 25%, FOV = 240 mm, matrix size = 128 × 128). A high-resolution 3D structural dataset (T1-weighted MPRAGE, TR = 2300 ms, TE = 2.98 ms, 240 slices, FOV = 256 mm; matrix size = 256 × 256; slice thickness = 1 mm) was also acquired in the same scanning session for registration purposes.
Resting-state fMRI images were analyzed with independent component analysis (ICA) and a dual-regression approach. Image preprocessing was carried out in FSL1 and AFNI2 softwares. This step included the removal of the first five scans, motion correction, skull stripping, spatial smoothing using a Gaussian kernel of FWHM = 6 mm, grand mean scaling, temporal filtering (low-pass and high-pass filters). Functional scans were then registered to their corresponding individual MPRAGE structural scans using linear registration with 6 df (Jenkinson and Smith, 2001) and further registered to the standard MNI template by concatenation of both registration matrices. Resampling resolution was set to 3 mm.
We used ICA, as implemented in MELODIC (Beckmann et al., 2005) from FSL, in order to decompose resting-state data into 25 independent components (ICs) which described common spatio-temporal independent patterns of correlated brain activity across the whole group of subjects in the study. Within the 25 ICs obtained, we identified the common resting-state functional networks (Damoiseaux et al., 2008; Smith et al., 2009; van den Heuvel and Hulshoff Pol, 2010), and selected the DMN, and two components corresponding to the right-lateralized and the left-lateralized fronto-parietal networks (right-FPN, and left-FPN). The selection procedure was performed by visual inspection together with template matching with online available data (Smith et al., 2009; Biswal et al., 2010) and with average task-related activation maps obtained from the data-driven analysis (see Figure 1 for a summary of the methods used in the study).
Then, we used the spatial patterns of the three selected networks in a dual-regression approach (as described in Filippini et al., 2009; Leech et al., 2011) in order to explore between-group differences. In the dual-regression analysis, we first regressed each subject’s resting-state functional data against the spatial IC maps and obtained individual time-series associated to each network (DMN, right-FPN, and left-FPN). These time-courses were then used to regress again the individual preprocessed fMRI data and to obtain individual spatial maps that were also specific for networks. Spatial maps were finally tested for voxel-wise differences between groups using non-parametric testing with 5000 random permutations.
Task-fMRI images were analyzed with a model-driven protocol to explore ROI-based BOLD signal change across task conditions. We also used a dual-regression analysis of task-fMRI data to investigate differences in network integration.
First, data preprocessing was performed in FSL and AFNI. It included motion correction, skull stripping, spatial smoothing using a Gaussian kernel of FWHM = 6 mm, grand mean scaling, temporal filtering (high-pass filter of sigma = 80 s), and registration to individual anatomical scans and to MNI standard space (Jenkinson and Smith, 2001). As in resting-fMRI, resampling resolution was set to 3 mm.
Task-fMRI data were analyzed using standard random-effects general linear model. We used the procedure as implemented in FMRI Analysis Tool (FEAT, Woolrich et al., 2001) from FSL. Five regressors were used to model the different blocks (0, 1-, 2-, 3-back, and fixation), and five additional regressors, modeling their first derivatives were introduced as nuisance variables. Contrast images were computed from the preprocessed functional data as follows: for the different levels of cognitive load, each condition was evaluated against the 0-back (1-, 2-, and 3-back >0-back), and for the fixation blocks, the signal was compared against the average of the other blocks. Average maps were created including all the subjects in the study. To investigate the load-dependent differences in brain activity especially within the networks of interest, we used peak coordinates of the selected IC spatial maps in order to create a set of spherical ROIs of 6 mm radius and extracted the percentage signal change for each contrast.
Between-group differences on all the quantitative measures of percentage signal change were assessed using one-way ANOVA implemented in PASW. The significance level was set at p < 0.05 (two-tailed).
As with resting-fMRI data, a dual-regression approach was applied to the preprocessed task-fMRI data. The spatial maps of the DMN and the right- and left-frontoparietal networks were used to regress task-fMRI data and to obtain individual patterns of these networks during task-performance. These maps were introduced in a voxel-wise group comparison with 5000 permutations.
Low-HE and high-HE groups differed in task-performance, but there were no significant differences in the performance of the 3-back task between YA and high-HE subjects. Mean RT was higher in elders than in young subjects for all the conditions, but there were no differences between high-HE and low-HE. Across the two groups of elders, age, gender, and MMSE were comparable, but high-HE had significantly higher education levels (Table 1; Figure 2).
Figure 2. Results of the task-performance during the different working memory loads. (A) Mean and SD values of d prime index, and (B) Mean and SD values of average response time (RT), in seconds. *Indicates p < 0.05 in the ANOVA post hoc analysis of between-group differences. 0B, 0-back; 1B, 1-back, 2B, 2-back, 3B, 3-back, YA, young adults; high-HE, high-performing healthy elders; low-HE, low-performing healthy elders.
The main findings, which are reported in the following sections, are summarized in Table 2.
Spatial maps derived from the whole-sample ICA decomposition of resting-state fMRI data corresponding to the DMN, the right-FPN, and the left-FPN are shown in Figure 3. The component identified as the DMN (Figure 3A) comprised areas in the frontal pole, middle frontal gyrus, and paracingulate gyrus (BA9, 10), the precuneus and posterior cingulate gyrus (BA7, 18, 23, 30, 31), and bilaterally in the superior occipital and posterior parietal cortices (BA19, 39). The right- and left-lateralized FPN (Figures 3B,C) involved areas in the middle and inferior frontal cortices (BA8, 9, 10, and 46), the paracingulate and anterior cingulate (BA6, BA8, BA32) and right and left parietal lobes, including the supramarginal and angular gyri. Although the lateralized pattern differed between right- and left-FPN, there was a broad overlap between these two networks.
Figure 3. Identification of the functional networks of interest from the ICA analysis of resting-state fMRI. Spatial maps of the three selected networks. (A) DMN network, (B) right-FPN, and (C) left-FPN.
With the dual-regression approach, we found differences in these three networks during the resting-state. As regards the DMN, low-HE exhibited decreased connectivity during the resting-state in frontal areas compared with YA and high-HE groups (Figure 4A). In the right-FPN (Figure 4B), the high-HE group had lower resting-state connectivity than both YA and low-HE groups. Finally, in the left-FPN (Figure 4C), low-HE, and high-HE had decreased resting-state connectivity in frontal areas. In the low-HE group, this decreased connectivity was observed in the left inferior and middle frontal gyri, left pars opercularis and left frontal pole (BA10, 44, 45, and 46). High-HE subjects showed the same pattern of decreased connectivity, but it was bilateral and extended to the anterior part of the superior temporal gyri, also including the anterior cingulate (BA6) and the insular cortex.
Figure 4. Results of the dual-regression analysis of resting-state fMRI. Maps show voxel-wise group-comparisons thresholded at a FWE corrected significance level of p < 0.05. (A) Group differences in the DMN, (B) Group differences in the right-FPN, and (C) group differences in the left-FPN.
We focused the analysis of task-fMRI data on a set of spherical ROIs that were selected from the networks identified in the resting-fMRI analysis (Figure 5). Three ROIs were created from the right-FPN: one in the right inferior frontal gyrus: right-IFG ROI (MNI coordinates: x = 42, y = 54, z = −4), one in the right middle frontal gyrus: right-MFG ROI (MNI coordinates: x = 46, y = 34, z = 32) and one in the right superior parietal gyrus: right-PAR ROI (MNI coordinates: x = 42, y = −58, z = 52). Three were created from the left-FPN: one in the left inferior frontal gyrus: left-IFG ROI (MNI coordinates: x = −46, y = 50, z = 0), one in the left middle frontal gyrus: left-MFG ROI (MNI coordinates: x = −46, y = 34, z = 20), and one in the left superior parietal gyrus: left-PAR ROI (x = −46, y = −50, z = 31). We also selected a region in the anterior cingulate cortex that was common for the right-FPN and the left-FPN: ACC ROI (MNI coordinates: x = −2, y = 26, z = 44). As regards the DMN, we defined four ROIs, one in the precuneus and posterior cingulate cortex: PCC ROI (MNI coordinates: x = 2, y = −66, z = 40), two in the left and right lateral occipital cortices: LLO ROI (MNI coordinates: x = −38, y = −82, z = 32), and RLO ROI (MNI coordinates: x = 42, y = −74, z = 36) and one in the middle frontal cortex: MFC ROI (MNI coordinates: x = 2, y = 58, z = −8).
Figure 5. Results of the ROI-based analysis of brain activity during the performance of the n-back task. Within each defined ROI, percent signal change are plotted for each group and condition. *Indicates that differences have a significance value of p < 0.05.
As shown in Figure 5, ROIs inside the task-positive networks showed, in general, a positive percentage of signal change during cognitively demanding blocks and negative values during fixation blocks, and ROIs within the DMN had the opposite behavior.
BOLD responsivity scores for the high-HE group were significantly higher (p < 0.05) than those for the YA group in the ACC, left-IFG, and right-IFG ROIs, for the 1-back and the 2-back conditions, and only in the right-IFG for the 3-back condition. No differences were observed in these regions between the low-HE and the YA groups. Moreover, in the right-IFG ROI, responsivity was also greater in the high-HE group than in the low-HE during 2-back blocks. During fixation, the high-HE group also showed greater deactivation of the right-IFG compared to YA.
No significant group-effects were found in task-related BOLD response in left-MFG, right-MFG, left-PAR, and right-PAR ROIs, during any of the cognitive blocks. During fixation, both high-HE and low-HE groups showed increased deactivation of the right-PAR ROI compared to YA.
Within the MFC ROI, only the YA group showed a clear pattern of deactivation (negative percentage signal change) during cognitive blocks that was not observable in the other two groups. High-HE had significant differences in BOLD response of this region with respect to YA in 2-back condition. Overall, in the MFC ROI we observed positive percentage signal changes associated with cognitive demands in high-HE whereas these values were always negative for the YA group.
In the PCC ROI, high-HE also showed increased task-related activation during cognitive blocks than YA. Although the described effect could be seen at all the load levels of the task, this difference was statistically significant only in 1-back blocks. In Figure 4, we see that the PCC was moderately activated in the YA group when performing the levels of the task with the highest demand.
Finally, no group differences were found regarding BOLD response in left and right lateral occipital ROIs within the DMN.
We used the dual-regression approach to explore differences in the selected networks during task-fMRI. High-HE showed decreased connectivity of the DMN with respect to YA (Figure 6A). However, in the right-FPN the same subjects had increased connectivity with respect to YA in several regions (Figure 6B), including the frontal pole, precentral gyrus, supplementary motor areas, anterior cingulate and paracingulate, insular cortex, and frontal orbital areas (BA6, 9, and 10). Finally, we found no differences in the connectivity of the left-FPN during task-fMRI.
Figure 6. Results of the dual-regression analysis of task-related fMRI. Maps show voxel-wise group-comparisons thresholded at a FWE corrected significance level of p < 0.05. (A) Group differences in the DMN, and (B) Group differences in the right-FPN.
Using ICA we identified intrinsic functional connectivity networks that are in operation during resting-state fMRI and during task fMRI, supporting the idea that brain connectivity has a network-based functional substrate that is not limited to the fact that the brain is functionally active (Smith et al., 2009). Although the ICA decomposition allowed the identification of other common resting-state brain networks, we only studied the DMN and the networks in the frontoparietal system due to their involvement in the working memory task that we used (similar to the approach considered in Leech et al., 2011). The three networks were identified in both resting- and task-fMRI, and functional reorganizations were found in both conditions, but in different directions. During the resting-state, elders with lower task-performance showed the largest differences with respect to YA within DMN connectivity, and those with higher task-performance showed reduced connectivity of the frontoparietal system. However, during task-fMRI, high-HE showed decreased connectivity of the DMN and increased connectivity of the FPN, but no differences were found between low-HE and young individuals. The analysis of task-related brain activity helped the interpretation of the results obtained by the ICA and the dual-regression approach, revealing task-related overactivations in frontal areas of the FPN, and the involvement of some DMN areas during task-performance in high-HE. Overall, our data show an age-by-performance modulation of brain networks that depends on external task demands.
Our data suggest evidence of reorganizations in the DMN connectivity in elders when compared to the group of YA. The pattern of functional reorganizations varied according to whether subjects are performing a cognitively demanding task. We found decreases in DMN connectivity that are in agreement with previously published work reporting age-related reduced DMN connectivity at rest (Damoiseaux et al., 2008; Littow et al., 2010; Wu et al., 2011; Tomasi and Volkow, 2012), and during task-fMRI (Sambataro et al., 2010, see Hafkemeijer et al., 2012 for a review of DMN and aging). Interestingly, we observed that disruptions in DMN connectivity, when studied at rest, were related with poor cognitive performance in the working memory domain. Studying the DMN connectivity in a group of aged subjects, Andrews-Hanna et al. (2007) also found a relationship between anterior-posterior connectivity and cognitive performance in several cognitive domains. Moreover, during task-fMRI, only the high-HE group showed a reduction in DMN connectivity compared to YA. This latter result suggests dynamic modulations/interactions within networks during the performance of a task: old adults who performed the task successfully needed to recruit/engage additional brain resources which are typically not related to the WM task. Other studies using task-fMRI (Grady et al., 2006, 2010; Filippini et al., 2012) reported age-related increases in activity within DMN regions, such as the mPFC, which are not traditionally implicated in task-performance. They also found that these activity increases were accompanied with decreases in task-related functional connectivity in the same areas (Grady et al., 2010).
The results we obtained with ICA and dual-regression were supported and extended by those of brain responsivity in DMN regions. We found differences in brain activity (measured as percent signal change) in two core regions of the DMN, the PCC, and the MFC. High-HE subjects recruited these areas during task-performance, but YA and low-HE groups did not. The recruitment of the anterior/frontal node of the DMN was specific for the high-HE group (Figure 5), a finding that supports the utilization of non-task-related resources as a compensation mechanism in the aged brain (Cabeza et al., 1997; Mattay et al., 2006). The fact that this area is located in the frontal node may support the notion of the Posterior-anterior shift in aging (PASA model, see Davis et al., 2008). As regards the recruitment of the PCC node, this effect was also observable in the YA cohort at high levels of working memory load, in agreement with other studies showing involvement of the precuneus in cognitive control (Leech et al., 2011). High-performing elders recruited this area at the lowest memory load. This result can be interpreted in the light of the compensation-related utilization of neural circuits hypothesis (CRUNCH) which posits that older adults need to recruit additional neural resources at lower loads than younger adults (Reuter-Lorenz and Cappell, 2008; Schneider-Garces et al., 2009).
In summary, age-related DMN disruptions have been discussed either as a compensatory mechanism (Grady et al., 2010; Filippini et al., 2012) or as a functional marker of deficits in cognitive control that lead to poorer performance in elder subjects (Persson et al., 2007; Sambataro et al., 2010; Hedden et al., 2012). Our results clearly support the first idea. However it is also plausible that both mechanisms of compensation and dysfunction associated with cognition coexist in the aging brain. Finally, as our elder groups differed in educational attainment, the present results may also be interpreted within the context of neural compensation, as posited by Stern (2009).
Focusing on the brain networks responsible for the working memory system, we found functional reorganizations in terms of altered connectivity and greater BOLD response in the high-performing elders. During the resting-state, high-HE showed decreased connectivity of the frontoparietal system. To date, few studies have reported age-related changes in resting-state networks other than the DMN. Filippini et al. (2012) found age-related increases in the executive network at rest but they did not consider the cognitive performance of the subjects. However, Littow et al. (2010) found age-related decreases in some resting-state networks related to executive control.
During task-fMRI, the ROI-based analysis of responsivity showed increased activity in frontal regions, mainly in the inferior frontal gyrus bilaterally and in the anterior cingulate cortex. The same behavior was observed in the left middle frontal gyrus, but did not reach the level of significance established. Although the greater effects were observed in areas of the right-FPN, we interpreted this result as a reduction of asymmetry in task-related networks. The results of the dual-regression on task-fMRI also showed that the spatial pattern of the right-FPN becomes almost bilateral in high-HE subjects when compared to YA. Grady et al. (2010) also found that a greater expression of a network comprising right dorsolateral prefrontal areas predicted better performance in old adults. In some studies, the contralateral PFC activation was interpreted as a result of the difficulty of recruiting specialized neural mechanisms (the dedifferentiation hypothesis, see Persson et al., 2006; Eyler et al., 2011); however, our results add evidence for the compensation hypothesis, and more specifically for the hemispheric asymmetry reduction in older adults (HAROLD, Cabeza et al., 2002a) pattern, since this effect was specific to the elders who performed well on the task. In addition, studies with other techniques such as TMS have also supported the HAROLD model and its relationship with successful aging in episodic memory performance (Solé-Padullés et al., 2006; Manenti et al., 2011).
Compensation mechanisms in terms of increased task-related BOLD activity have also been described in the pathologic brain in Alzheimer’s disease and mild cognitive impairment (Bokde et al., 2010). However, these patterns usually reflect activation of additional brain areas due to inefficient functioning of a network that might be compromised by disease; what is more, they have been studied in the context of keeping the same performance level as their age-matched controls, rather than in the context of increased performance as we found in healthy adults.
The interpretation of our results as the specialization of a task-related network also provides an insight into the study of intervention methods that include cognitive training in healthy aging. Although the neural mechanisms of training effects are still unknown, it has been commonly reported that higher BOLD activity is associated with better performance or higher improvement in task-performance (for a review see Klingberg, 2010). Thus, the study of inter-individual differences in the relationship between brain activity and behavioral outcome may be a key point in the design of effective training programs for patients whose limited cognitive capacities are restricting their daily lives. Moreover, further studies should determine whether these brain-behavior associations are limited to the cognitive task that is being performed in the scanner or whether they can be extrapolated to other cognitive domains. The latter question can be partially answered by examining our results of the resting-state analysis, since we already found brain reorganizations in the resting-state networks in high-performing old subjects.
Using analysis of functional data during resting-state and during the performance of an n-back task, we provide evidence that the precepts of the principal neurocognitive theories of aging can be accommodated. First, functional compensation mechanisms were found: older people with successful working memory performance utilize different brain regions during cognitive activity than young people, and some of these regions are recruited from the DMN, a brain network that is typically deactivated during working memory performance. The recruitment of the frontal DMN regions was specific to elders with high-performance levels; however, recruitment of the precuneus was also observed in young subjects at high levels of working memory load (supporting the CRUNCH hypothesis, Reuter-Lorenz and Cappell, 2008). Moreover, within task-related networks, high-performing elders showed both increased connectivity and increased BOLD response bilaterally in frontal regions, supporting the HAROLD model (Cabeza et al., 2002a) as well as the PASA model (Davis et al., 2008). Moreover, these dynamic network reorganizations were different at rest, when high-performing elders had less disruption of the DMN but greater disruption within the frontoparietal system than low-performing elders. We therefore suggest that successful aging is characterized by a level of brain plasticity that may mediate the efficient recruitment of functional resources in task-relevant areas when the subject is exposed to a task with a high cognitive demand even though this recruitment is not observable at rest. It has been proposed that there is an optimal level of brain plasticity during the age span that varies across subjects and allows this adaptation to a changing environment. Thus, both hypo- and hyperplastic mechanisms may set the stage for dementia or age-related declines in cognitive abilities (Pascual-Leone et al., 2011).
The authors declare that the research was conducted in the absence of any commercial or financial relationships that could be construed as a potential conflict of interest.
Andrews-Hanna, J. R., Snyder, A. Z., Vincent, J. L., Lustig, C., Head, D., Raichle, M. E., and Buckner, R. L. (2007). Disruption of large-scale brain systems in advanced aging. Neuron 56, 924–935.
Arenaza-Urquijo, E. M., Bosch, B., Sala-Llonch, R., Solé-Padullés, C., Junqué, C., Fernández-Espejo, D., Bargalló, N., Rami, L., Molinuevo, J. L., and Bartrés-Faz, D. (2011). Specific anatomic associations between White matter integrity and cognitive reserve in normal and cognitively impaired elders. Am. J. Geriatr. Psychiatry 19, 33–42.
Beckmann, C. F., De Luca, M., Devlin, J. T., and Smith, S. M. (2005). Investigations into resting-state connectivity using independent component analysis. Philos. Trans. R. Soc. Lond. B Biol. Sci. 360, 1001–1013.
Berlingeri, M., Bottini, G., Danelli, L., Ferri, F., Traficante, D., Sacheli, L., Colombo, N., Sberna, M., Sterzi, R., Scialfa, G., and Paulesu, E. (2010). With time on our side? Task-dependent compensatory processes in graceful aging. Exp. Brain Res. 205, 307–324.
Biswal, B. B., Mennes, M., Zuo, X. N., Gohel, S., Kelly, C., Smith, S. M., Beckmann, C. F., Adelstein, J. S., Buckner, R. L., Colcombe, S., Dogonowski, A. M., Ernst, M., Fair, D., Hampson, M., Hoptman, M. J., Hyde, J. S., Kiviniemi, V. J., Kötter, R., Li, S. J., Lin, C. P., Lowe, M. J., Mackay, C., Madden, D. J., Madsen, K. H., Margulies, D. S., Mayberg, H. S., McMahon, K., Monk, C. S., Mostofsky, S. H., Nagel, B. J., Pekar, J. J., Peltier, S. J., Petersen, S. E., Riedl, V., Rombouts, S. A., Rypma, B., Schlaggar, B. L., Schmidt, S., Seidler, R. D., Siegle, G. J., Sorg, C., Teng, G. J., Veijola, J., Villringer, A., Walter, M., Wang, L., Weng, X. C., Whitfield-Gabrieli, S., Williamson, P., Windischberger, C., Zang, Y. F., Zhang, H. Y., Castellanos, F. X., and Milham, M. P. (2010). Toward discovery science of human brain function. Proc. Natl. Acad. Sci. U.S.A. 107, 4734–4739.
Bokde, A. L. W., Karmann, M., Born, C., Teipel, S. J., Omerovic, M., Ewers, M., Frodl, T., Meisenzahl, E., Reiser, M., Möller, H. J., and Hampel, H. (2010). Altered brain activation during a verbal working memory task in subjects with amnestic mild cognitive impairment. J. Alzheimers Dis. 21, 103–118.
Braver, T. S., Cohen, J. D., Nystrom, L. E., Jonides, J., Smith, E. E., and Noll, D. C. (1997). A parametric study of prefrontal cortex involvement in human working memory. Neuroimage 5, 49–62.
Cabeza, R., Anderson, N. D., Locantore, J. K., and McIntosh, R. (2002a). Aging gracefully: compensatory brain activity in high-performing older adults. Neuroimage 17, 1394–1402.
Cabeza, R., Dolcos, F., Graham, R., and Nyberg, L. (2002b) , Similarities and differences in the neural correlates of episodic memory retrieval and working memory. Neuroimage 16, 317–330.
Cabeza, R., Grady, C. L., Nyberg, L., McIntosh, A. R., Tulving, E., Kapur, S., Jennings, J. M., Houle, S., and Craik, F. I. M. (1997). Age-related differences in neural activity during memory and retrieval: a positron emission tomography study. J. Neurosci. 17, 391–400.
Damoiseaux, S., Beckmann, C. F., Arigita, E. J., Barkhof, F., Scheltens, P., Stam, C. J., Smith, S. M., and Rombouts, S. A. (2008). Reduced resting-state brain activity in the “default network” in normal aging. Cereb. Cortex 18, 1856–1864.
Davis, S. W., Dennis, N. A., Daselaar, S. M., Fleck, M. S., and Cabeza, R. (2008). Que PASA? The posterior-anterior shift in aging. Cereb. Cortex 18, 1201–1209.
Eyler, L. T., Sherzai, A., Kaup, A. R., and Jeste, D. V. (2011). A review of functional brain imaging correlates of successful cognitive aging. Biol. Psychiatry 70, 115–122.
Filippini, N., MacIntosh, B. J., Hough, M. G., Goodwin, G. M., Frisoni, G. B., Smith, S. M., Matthews, P. M., Beckmann, C. F., and Mackay, C. E. (2009). Distinct patterns of brain activity in young carriers of the APOE-epsilon4 allele. Proc. Natl. Acad. Sci. U.S.A. 29, 1021–1026.
Filippini, N., Nickerson, L. D., Beckmann, C. F., Ebmeier, K. P., Frisoni, G. B., Mathews, P. M., Smith, S. M., and Mackay, C. E. (2012). Age-related adaptations of brain function during a memory task are also present at rest. Neuroimage 59, 565–572.
Grady, C. L. (2000). Functional brain imaging and age-related changes in cognition. Biol. Psychol. 54, 259–281.
Grady, C. L., Protzner, A. B., Kovacevic, N., Strother, S. C., Afshin-Pour, B., Wojtowicz, M., Anderson, J. A. E., Churchill, N., and McIntosh, A. R. (2010). A multivariate analysis of age-related differences in default mode and task-positive networks across multiple cognitive domains. Cereb. Cortex 20, 1432–1447.
Grady, C. L., Springer, M. V., Hongwanishkul, D., McIntosh, A. R., and Winocur, G. (2006). Age-related changes in brain activity across the adult lifespan. J. Cogn. Neurosci. 18, 227–241.
Hafkemeijer, A., Van der Grond, J., and Rombouts, S. A. (2012). Imaging the default mode network in aging and dementia. Biochim. Biophys. Acta. 1822, 431–441.
Hedden, T., Van Dijk, K. R., Shire, E. R., Sperling, R. A., Johnosn, K. A., and Buckner, R. L. (2012). Failure to modulate attentional control in white matter pathology. Cereb. Cortex. 22, 1038–1051.
Jenkinson, M., and Smith, S. M. (2001). A global optimization method for robust affine registration of brain images. Med. Image Anal. 5, 143–156.
Klingberg, T. (2010). Training and plasticity of working memory. Trends Cogn. Sci. (Regul. Ed.) 14, 317–324.
Leech, R., Kamouriech, S., Beckmann, C. F., and Sharp, D. J. (2011). Fractionating the default mode network: distinct contributions of the ventral and dorsal posterior cingulate cortex to cognitive control. J. Neurosci. 31, 3217–3224.
Littow, H., Elseoud, A. A., Haapea, M., Isohanni, M., Moilanen, I., Mankinen, K., Nikkinen, J., Rahko, J., Rantala, H., Remes, J., Starck, T., Tervonen, O., Veijola, J., Beckmann, C., and Kiviniemi, V. J. (2010). Age-related differences in functional nodes of the brain cortex – a high model order group ICA study. Front. Syst. Neurosci. 4:32. doi:10.3389/fnsys.2010.00032
Manenti, R., Cotelli, M., and Miniussi, C. (2011). Successful physiological aging and episodic memory: a brain stimulation study. Behav. Brain Res. 216, 153–158.
Mattay, V. S., Fera, F., Tessitore, A., Hariri, A. R., Berman, K. F., Das, S., Meyer-Lindenberg, A., Goldberg, T. E., Callicott, J. H., and Weinberger, D. R. (2006). Neurophysiological correlates of age-related changes in working memory capacity. Neurosci. Lett. 392, 32–37.
Park, D. C., Lautenschlager, G., Hedden, T., Davidson, N. S., Smith, A. D., and Smith, P. K. (2002). Models of visuospatial and verbal memory across the adult life span. Psychol. Aging 17, 229–320.
Park, D. C., and Reuter-Lorenz, P. (2009). The adaptive brain: aging and neurocognitive scaffolding. Annu. Rev. Psychol. 60, 173–196.
Pascual-Leone, A., Freitas, C., Oberman, L., Horvath, J. C., Halko, M., Eldaief, M., Bashir, S., Vernet, M., Shafi, M., Westover, B., Vahabzadeh-Hagh, A. M., and Rotenberg, A. (2011). Characterizing brain cortical plasticity and network dynamics across the age-span in health and disease with TMS-EEG and TMS-fMRI. Brain Topogr. 24, 302–315.
Persson, J., Lustig, C., Nelson, J. K., and Reuter-Lorenz, P. A. (2007). Age differences in deactivation: a link to cognitive control? J. Cogn. Neurosci. 19, 1021–1032.
Persson, J., Nyberg, L., Lind, J., Larsson, A., Nilsson, L. G., Ingvar, M., and Buckner, R. L. (2006). Structure-function correlates of cognitive decline in aging. Cereb. Cortex 16, 907–915.
Reuter-Lorenz, P. A., and Cappell, K. A. (2008). Neurocognitive aging and the compensation hypothesis. Curr. Dir. Psychol. Sci. 17, 177–182.
Reuter-Lorenz, P. A., and Park, D. C. (2010). Human neuroscience and the aging mind: a new look at old problems. J. Gerontol. B Psychol. Sci. Soc. Sci. 65B, 405–415.
Sala-Llonch, R., Peña-Gómez, C., Arenaza-Urquijo, E. M., Vidal-Piñeiro, D., Bargalló, N., Junqué, C., and Bartrés-Faz, D. (2011). Brain connectivity during resting state and subsequent working memory task predicts behavioural performance. Cortex. (in press).
Sambataro, F., Murty, V. P., Callicott, J. H., Tan, H. Y., Das, S., Weinberger, D. R., and Mattay, V. S. (2010). Age-related alterations in default mode network: impact on working memory performance. Neurobiol. Aging 31, 839–852.
Schneider-Garces, N. J., Gordon, B. A., Brumback-Peltz, C. R., Shin, E., Lee, Y., Sutton, B. P., Maclin, E. L., Gratton, G., and Fabiani, M. (2009). Span, CRUNCH, and beyond: working memory capacity and the aging brain. J. Cogn. Neurosci. 22, 655–669.
Smith, S. M., Fox, P. T., Miller, K. L., Glahn, D. C., Fox, P. M., Mackay, C. E., Filippini, N., Watkins, K. E., Toro, R., Laird, A. R., and Beckmann, C. F. (2009). Correspondence of the brain’s functional architecture during activation and rest. Proc. Natl. Acad. Sci. U.S.A. 106, 13040–13045.
Solé-Padullés, C., Bartrés-Faz, D., Junqué, C., Clemente, I. C., Molinuevo, J. L., Bargalló, N., Sánchez-Aldeguer, J., Bosch, B., Falcón, C., and Valls-Solé, J. (2006). Repetitive transcranial magnetic stimulation effects on brain function and cognition among elders with memory dysfunction. A randomized sham-controlled study. Cereb. Cortex 16, 1487–1493.
Spreng, R. N., Wojtowiicz, M., and Grady, C. L. (2010). Reliable differences in brain activity between young and old adults: a quantitative meta-analysis across multiple domains. Neurosci. Biobehav. Rev. 34, 1178–1194.
Tomasi, D., and Volkow, N. D. (2012). Aging and functional brain networks. Mol. Psychiatry. 17, 549–558.
Turner, G. R., and Spreng, R. N. (2012). Executive functions and neurocognitive aging: dissociable patterns of brain activity. Neurobiol. Aging 33, 826.e1–826.e13.
van den Heuvel, M. P., and Hulshoff Pol, H. E. (2010). Exploring the brain network: a review on resting-state fMRI functional connectivity. Eur. Neuropsychopharmacol. 20, 519–534.
Woolrich, M. W., Riple, B. D., Brady, J. M., and Smith, S. M. (2001). Temporal autocorrelation in univariate linear modelling of FMRI data. Neuroimage 14, 1370–1386.
Keywords: fMRI, aging, working memory, compensation, plasticity, frontal cortex, default-mode network
Citation: Sala-Llonch R, Arenaza-Urquijo EM, Valls-Pedret C, Vidal-Piñeiro D, Bargalló N, Junqué C and Bartrés-Faz D (2012) Dynamic functional reorganizations and relationship with working memory performance in healthy aging. Front. Hum. Neurosci. 6:152. doi: 10.3389/fnhum.2012.00152
Received: 15 January 2012; Accepted: 15 May 2012;
Published online: 07 June 2012.
Edited by:
Simone Rossi, Azienda Ospedalira Universitaria Senese, ItalyReviewed by:
Stefano F. Cappa, Vita-Salute San Raffaele University, ItalyCopyright: © 2012 Sala-Llonch, Arenaza-Urquijo, Valls-Pedret, Vidal-Piñeiro, Bargalló, Junqué and Bartrés-Faz. This is an open-access article distributed under the terms of the Creative Commons Attribution Non Commercial License, which permits non-commercial use, distribution, and reproduction in other forums, provided the original authors and source are credited.
*Correspondence: David Bartrés-Faz, Departament de Psiquiatria i Psicobiologia Clínica, Facultat de Medicina, Universitat de Barcelona, Casanova 143, 08036 Barcelona, Spain. e-mail:ZGJhcnRyZXNAdWIuZWR1
Disclaimer: All claims expressed in this article are solely those of the authors and do not necessarily represent those of their affiliated organizations, or those of the publisher, the editors and the reviewers. Any product that may be evaluated in this article or claim that may be made by its manufacturer is not guaranteed or endorsed by the publisher.
Research integrity at Frontiers
Learn more about the work of our research integrity team to safeguard the quality of each article we publish.