- 1 Department of Otolaryngology – Head and Neck Surgery, University of California San Francisco, San Francisco, CA, USA
- 2 Department of Radiology and Biomedical Imaging, University of California San Francisco, San Francisco, CA, USA
Spoken language exists because of a remarkable neural process. Inside a speaker’s brain, an intended message gives rise to neural signals activating the muscles of the vocal tract. The process is remarkable because these muscles are activated in just the right way that the vocal tract produces sounds a listener understands as the intended message. What is the best approach to understanding the neural substrate of this crucial motor control process? One of the key recent modeling developments in neuroscience has been the use of state feedback control (SFC) theory to explain the role of the CNS in motor control. SFC postulates that the CNS controls motor output by (1) estimating the current dynamic state of the thing (e.g., arm) being controlled, and (2) generating controls based on this estimated state. SFC has successfully predicted a great range of non-speech motor phenomena, but as yet has not received attention in the speech motor control community. Here, we review some of the key characteristics of speech motor control and what they say about the role of the CNS in the process. We then discuss prior efforts to model the role of CNS in speech motor control, and argue that these models have inherent limitations – limitations that are overcome by an SFC model of speech motor control which we describe. We conclude by discussing a plausible neural substrate of our model.
Introduction
Speech motor control is unique among motor behaviors in that it is a crucial part of the language system. It is the final neural processing step in speaking, where intended messages drive articulator movements that create sounds conveying those messages to a listener (Levelt, 1989). Many questions arise concerning this neural process we call speech motor control. What is its neural substrate? Is it qualitatively different from other motor control processes? Recently, research into other areas of motor control has benefited from a vigorous interplay between people who study the psychophysics and neurophysiology of motor control and engineers that develop mathematical approaches to the abstract problem of control. One of the key results of these collaborations has been the application of state feedback control (SFC) theory to modeling the role of the higher central nervous system (i.e., cortex, the cerebellum, thalamus, and basal ganglia – hereafter referred to as “the CNS”) in motor control (Arbib, 1981; Todorov and Jordan, 2002; Todorov, 2004; Guigon et al., 2008; Shadmehr and Krakauer, 2008). SFC postulates that the CNS controls motor output by (1) estimating the current state of the thing (e.g., arm) being controlled, and (2) generating controls based on this estimated state. SFC has successfully predicted a great range of the phenomena seen in non-speech motor control, but as yet has not received attention in the speech motor control community. Here we review some of the key characteristics of how sensory feedback appears to be used during speaking and what this says about the role of the CNS in the speech motor control process. Along the way, we discuss prior efforts to model this role, but ultimately we argue that such models can be seen as approximating characteristics best modeled by SFC. We conclude by presenting an SFC model of the role of the CNS in speech motor control and discuss its neural plausibility.
The Role of the CNS in Processing Sensory Feedback during Speaking
It is not controversial that the CNS plays a role in speech motor output: cortex appears to be a main source of motor commands in speaking. In humans, the speech-relevant areas of motor cortex (M1) make direct connections with the motor neurons of the lips, tongue, and other speech articulators (Jürgens et al., 1982; Jürgens, 2002; Ludlow, 2004). Damage to these M1 areas causes mutism and dysarthria (Jürgens, 2002; Duffy, 2005). On the other hand, it is much less clear what the role of the CNS is in processing the sensory feedback from speaking. Sensory feedback, and especially auditory feedback, is critically important for children learning to speak (Smith, 1975; Ross and Giolas, 1978; Levitt et al., 1980; Osberger and McGarr, 1982; Oller and Eilers, 1988; Borden et al., 1994). However, once learned, the control of speech has the characteristics of being both responsive to, yet not dependent on sensory feedback. In the absence of sensory feedback, speaking is only selectively disrupted. Somatosensory nerve block impacts only certain aspects of speech (e.g., lip rounding, fricative constrictions), and even for these, the impact is not sufficient to prevent intelligible speech (Scott and Ringel, 1971). In post-lingually deafened speakers, the control of pitch and loudness degrades rapidly after hearing loss, yet their speech will remain intelligible for decades (Cowie and Douglas-Cowie, 1992; Lane et al., 1997). Normal speakers also produce intelligible speech with their hearing temporarily blocked by loud masking noise (Lombard, 1911; Lane and Tranel, 1971).
But this does not mean speaking is largely a feedforward control process that is unaffected by feedback. Delaying auditory feedback (DAF) by roughly a syllable’s production time (100–200 ms) is very effective at disrupting speech (Lee, 1950; Fairbanks, 1954; Yates, 1963). Masking noise feedback causes increases in speech loudness (Lombard, 1911; Lane and Tranel, 1971), while amplifying feedback causes compensatory decreases in speech loudness (Chang-Yit et al., 1975). Speakers compensate for mechanical perturbations of their articulators (Abbs and Gracco, 1984; Saltzman et al., 1998; Shaiman and Gracco, 2002), and compensatory changes in speech production are seen when auditory feedback is altered in its pitch (Elman, 1981; Jones and Munhall, 2000a), formant frequencies (Houde and Jordan, 1998, 2002; Purcell and Munhall, 2006), or, in the case of fricative production, when the center of spectral energy is shifted (Shiller et al., 2007).
Taken together, such phenomena reveal a complex role for feedback in the control of speaking – a role not easily modeled as simple feedback control. Beyond this, however, there are also more basic difficulties with modeling the control of speech as being based on sensory feedback. In biological systems, sensory feedback is noisy, due to environment noise and the stochastic firing properties of neurons (Kandel et al., 2000). Furthermore, when considering the role of the CNS in particular, an even more significant problem is that sensory feedback is delayed. There are several obvious reasons why sensory feedback to the CNS is delayed [e.g., by axon transmission times and synaptic delays (Kandel et al., 2000)], but a less obvious reason involves the time needed to process raw sensory feedback into features useful in controlling speech. For example, in the auditory domain, there are several key features of the acoustic speech waveform that are important for discriminating between speech utterances. For some of these features, like pitch, spectral envelope, and formant frequencies, signal processing theory dictates that the accuracy in which the features are estimated from the speech waveform depends on the duration of the time window used to calculate them (Parsons, 1987). In practice, this means such features are estimated from the acoustic waveform using sliding time windows with lengths on the order of 30–100 ms in duration. Such integration-window-based feature estimation methods are slow to respond to changes in the speech waveform, and thus effectively will introduce additional delays in the detection of such changes. Consistent with this theoretical account, studies show that response latencies of auditory areas to changes in higher-level auditory features can range from 30 ms to over 100 ms (Heil, 2003; Cheung et al., 2005; Godey et al., 2005). A particularly relevant example is the long (∼100 ms) response latency of neurons in a recently discovered area of pitch-sensitive neurons in auditory cortex (Bendor and Wang, 2005). As a result, while auditory responses can be seen within 10–15 ms of a sound at the ear (Heil and Irvine, 1996; Lakatos et al., 2005), there are important reasons to suppose that the features needed for controlling speech are not available to the CNS until a significant time (∼30–100 ms) after they are peripherally present. This is a problem for feedback control models, because direct feedback control based on delayed feedback is inherently unstable, particularly for fast movements (Franklin et al., 1991).
The CNS as a Feedforward Source of Speech Motor Commands
Given these problems with controlling speech via sensory feedback control, it is not surprising that, in some models of speech motor control, the role of the CNS has been relegated to being a pure feedforward source, outputting desired trajectories for the lower motor system to follow (Ostry et al., 1991, 1992; Perrier et al., 1996; Payan and Perrier, 1997; Sanguineti et al., 1997, 1998). In these models, it is the lower motor system (e.g., brainstem and spinal cord) which implements feedback control and responds to feedback perturbations. The inspiration for these models comes from consideration of biomechanics and neurophysiology. A muscle has mechanical spring-like properties that naturally resist perturbations (Hill, 1925; Zajac, 1989), and these spring-like properties are further enhanced by somatosensory feedback to the motor neurons in the brainstem and spinal cord that control the muscle [e.g., for the jaw: (Pearce et al., 2003); see also the stretch reflex (Matthews, 1931; Merton, 1951; Hulliger, 1984)]. This local feedback control of the muscle makes it look, to a first approximation, like a spring with an adjustable rest-length that can be set by control descending from the higher levels of the CNS (Asatryan and Feldman, 1965). The muscles affecting an articulator’s position (e.g., the muscles controlling the position of the tongue tip) always come in opposing pairs – agonists and antagonists – whose contractions have opposite effects on articulator position. Thus, for any given set of muscle activations, an articulator will always come to rest at an equilibrium point where the muscle forces are balanced. In response to perturbations from its current equilibrium point, the articulator will naturally generate forces that return it to the equilibrium point, without any higher-level intervention. This characteristic was the inspiration for models of motor control based on equilibrium point control (EPC; Polit and Bizzi, 1979; Bizzi et al., 1982; Feldman, 1986). EPC models postulate that to control an articulator’s movement, the higher-level CNS need only provide the lower motor system with a sequence of desired equilibrium point to specify the trajectory of that articulator. The lower motor system handles responses to perturbations.
In speech, EPC models can explain the phenomenon of “undershoot,” or “carryover,” coarticulation (Lindblom, 1963). This can be seen when a speaker produces a vowel in a CVC context: as the duration of the vowel segment is made shorter, the formants of the vowel do not reach (i.e., they undershoot) their normal steady-state values. This undershoot is easily explained by supposing that successive equilibrium points are generated faster than they can be achieved. In the case of a rapidly produced CVC syllable, undershoot of vowel formants would happen if, while it was still moving toward the equilibrium point for the vowel, the tongue was retargeted to the equilibrium point of the following consonant.
There are, however, several problems with the EPC account of the lower motor system being solely responsible for feedback control. First, although both somatosensory (Kandel et al., 2000; Jürgens, 2002) and auditory (Burnett et al., 1998; Jürgens, 2002) pathways make subcortical connections with descending motor pathways, the latencies of responses to somatosensory and auditory feedback perturbations (on the order of 50–150 ms) are longer than would be expected for subcortical feedback loops (Abbs and Gracco, 1983). Instead, such response delays appear sufficiently long enough for neural signals to go to and come from cortex (Kandel et al., 2000). By themselves, such timing estimates do not prove involvement of cortex, but a study by Ito and Gomi using transcranial magnetic stimulation (TMS) gives further evidence (Ito et al., 2005). The authors examined the facilitatory effect of applying a subthreshold TMS pulse to mouth motor cortex on two oral reflexes: the compensatory response by the upper lip to a jaw-lowering perturbation during the production of /ph/ (a soft version of /f/ in Japanese made only with the lips), and a response to upper lip stimulation know to be subcortically mediated called the perioral reflex. The TMS pulse was applied approximately 10 ms before the time of the reflex response – i.e., at the time motor cortex would be activated if it governed the response. The authors found motor TMS only facilitated the response to jaw perturbation during /ph/, implicating cortex involvement specifically in only the task-dependent perturbation response during speaking.
Perhaps a larger problem with ascribing feedback control to only subcortical levels is that responses to sensory feedback perturbations in speaking often look task specific. For example, perturbation of the upper lip will induce compensatory movement of the lower lip, but only in the production of bilabials: the upper lip is not involved in the production of /f/ and perturbation of the upper lip before /f/ in /afa/ induces no lower lip response. On the other hand, the upper lip is involved in the production of /p/ and here, perturbation of the upper lip before /p/ in /apa/ does induce compensatory movement of the lower lip (Shaiman and Gracco, 2002). Task-dependence is also seen in responses to auditory feedback. The production of vowels in stressed syllables appears to be more sensitive to immediate auditory feedback than vowels in unstressed syllables (Kalveram and Jancke, 1989; Natke and Kalveram, 2001; Natke et al., 2001), responses to pitch perturbations are modulated by how fast the subject is changing pitch (Larson et al., 2000), and responses to loudness perturbations appear to be modulated by syllable emphasis (Liu et al., 2007). Such task-dependent perturbation responses cannot be simply explained with pure feedback control by setting stiffness levels, i.e., muscle impedance, for individual articulators (e.g., upper lip or lower lip), and suggest instead that depending on the task (i.e., the particular speech target being produced), the higher-level CNS uses sensory feedback to couple the behavior of different articulators in ways that accomplish a higher-level goal (e.g., closing of the lip opening; Bernstein, 1967; Kelso et al., 1984; Saltzman and Munhall, 1989).
Taken together, these several lines of evidence suggest that, rather than simply instructing the lower motor system on what its goals are, the CNS instead likely plays an active role in responding to sensory information about deviations from task goals.
Adding Feedback Control to a Feedforward Model of the CNS’S Role in Speech Motor Control
One approach to remedying the EPC model of the CNS in speaking is to simply add a feedback control system to it. This is the approach has long been considered in non-speech motor control research (Arbib, 1981), most formally by Kawato et al. with the feedback error learning architecture (Kawato et al., 1987; Kawato and Gomi, 1992). This feedback error learning architecture has been adapted to modeling speech motor control in the well-known DIVA (directions into velocities of articulators) model (Guenther, 1995; Guenther et al., 1998, 2006; Ghosh, 2004). A discrete-time version of the DIVA model is shown in Figure 1, which shows the anatomical locations of model components, as postulated by Guenther et al. (2006). DIVA has a feedforward control subsystem operating in parallel with a feedback control subsystem, with ut being the sum of the two subsystems, weighted by αff and αfb, respectively. For well-learned speech sounds, the feedforward control subsystem is entirely responsible for generating output controls ut; the feedback control subsystem (red pathway in figure) only generates corrections if disturbances (random control variations or external feedback perturbations) cause sensory feedback yt−N to stray outside the bounds specified by speech category target .
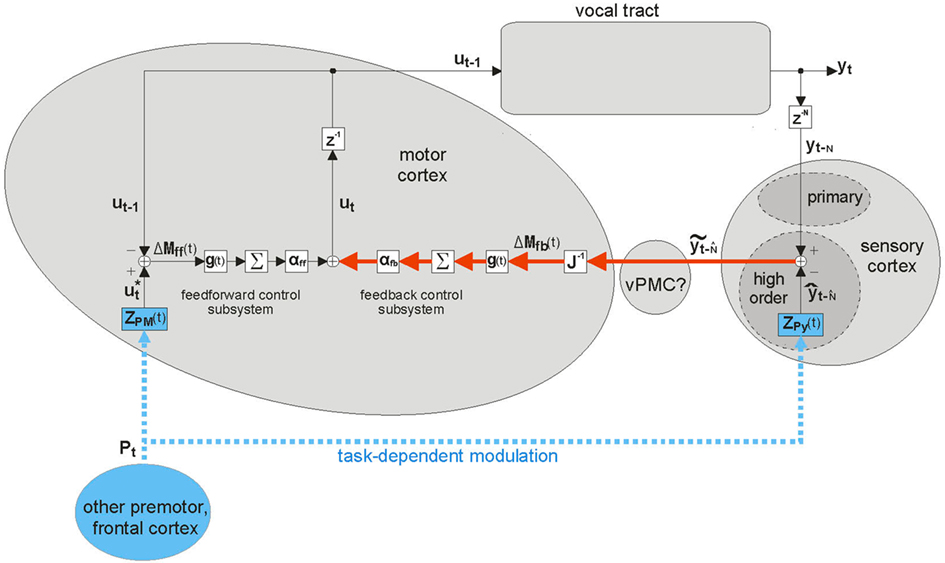
Figure 1. Schematic of DIVA. This diagram differs from published diagrams of the model that show both the auditory and somatosensory feedback control subsystems (Guenther et al., 2006); here for simplicity, we show only one generic feedback control subsystem and sensory cortex that represents two similar subsystems (auditory and somatosensory) with different but analogous anatomical substrates. In addition, here we focus on the operation of the feedback control subsystem (red). This discrete-time depiction shows the model at time t, when desired articulatory position ut−1 has previously been applied to the vocal tract, causing it to produce sensory feedback yt. This sensory feedback is seen N msec later in the sensory cortices as yt−N, where it is compared with the target representation . If yt−N strays outside the bounds of
, a non-zero sensory feedback error
is generated in the feedback control subsystem (red), which is converted to an articulatory position error ΔMfb(t) by the learned inverse Jacobian J−1(ut−1), and added in to the feedback control subsystem’s contribution (weighted by αfb) to the next desired articulatory position ut to be applied to the vocal tract. Task-dependent modulation of the control system (blue) is provided by speech sound units Pt in frontal cortex making time-varying synapses ZPM(t) and ZPy(t) onto units in the motor and high order sensory cortices, respectively. [Note: Until recently, the DIVA model had the sensory cortices linked directly to motor cortex, where all operations of the feedback control subsystem occurred. However, recent neuroimaging work (Tourville et al., 2008) has shown that right ventral premotor cortex (vPMC) appears to be in at least the auditory part of this subsystem (Guenther, 2008).]
Like the EPC models, DIVA models the CNS as generating desired trajectories for the lower motor system to follow. However, the DIVA model represents a big departure from pure EPC models in that it assumes the higher CNS actively processes feedback during ongoing speaking, and uses that feedback to make ongoing adjustments to the articulatory trajectory being generated. The model is not dependent on sensory feedback being present to produce speech since the feedforward subsystem can generate articulatory controls by itself. Yet, via the feedback control system, it can respond to alterations from expected feedback. Furthermore, by representing speech targets not as points but instead as acceptable ranges of features, the DIVA model can account for more coarticulatory effects than just the undershoot phenomena accounted for by EPC models. In instances of lookahead coarticulation, speakers appear to anticipate the future need of currently non-critical articulators by moving them in advance to their ultimately needed positions (Henke, 1966; Kent and Minifie, 1977; Hardcastle and Hewlett, 2006). For example, in the production of /ba/, the tongue is already moved to the position for /a/ during the production of /b/. This is accommodated in the DIVA model by having permissive bounds on non-critical features of a given speech sound target. In DIVA, the bounds on features relating to tongue position in the target representation of /b/ would be wide enough to allow the tongue to be put in position for the upcoming /a/ target (which has more stringent bounds on tongue position), without straying outside the target bounds for /b/.
In this way, the DIVA model embodies a hypothesis that the CNS processes sensory feedback for control of speech production in a categorical manner similar to that seen in speech perception. The target/category feature bounds allow for the variability in articulation seen in coarticulation, but this comes at a cost: for each feature range, the upper limit on tolerance for variability is also the lower limit on sensitivity to unexpected perturbations: in DIVA, only perturbations that stray outside the permitted feature range are detected and corrected. Yet there are reasons to suppose speakers would benefit from being sensitive to changes in feedback at a more fine-grained, sub-categorical level. Although the sound inventory of a language partly reflects constraints averaged over the history of its speakers (MacNeilage, 1998; MacNeilage and Davis, 2001; Blevins, 2004; Hayes and Steriade, 2004), a particular speaker’s vocal apparatus will not be perfectly matched to the language’s sounds. Yet, as much as possible, he cannot allow the particular characteristics of his own vocal apparatus to prevent him from producing sounds within the allowable variations of his language’s sound categories (Lindblom, 1990). This is not just a concern when a speaker learns to speak, but is also a concern in the maintenance of the ability to speak. This is because the response characteristics of any motor execution system (e.g., an arm, leg, or vocal tract) can vary from day to day and even, to some extent, from hour to hour (Kording et al., 2007; Shadmehr and Krakauer, 2008). For example, the latency and vigor to which a muscle responds to neural stimulation vary (e.g., it may have been just frequently used, and is now somewhat more fatigued than usual). In general, such variations in a speaker’s vocal production system would have acoustic consequences, and thus it would be advantageous for a speaker to use sensory feedback (both auditory and somatosensory) to detect these variations and correct them before they have categorical consequences (i.e., before a deviation strays outside a category boundary) that could confuse the listener and impede communication.
Yet when we look at how speakers actually do correct for feedback deviations, we instead find evidence suggesting a lack of sensitivity to sensory feedback: speakers’ compensation for feedback alterations are usually far from complete, often compensating for no more that 10 or 20% of the audio alteration. This could be interpreted as imprecision in the speech production process, but it does not necessarily follow that incompleteness equals imprecision. Firstly, at the onset of any feedback perturbation, compensation cannot be large because of stability considerations. As discussed above, there are significant delays inherent in the conveying and processing of sensory feedback from the periphery to the CNS, and any immediate fully compensating responses to this “out-of-date” sensory information will likely result in an unstable motor control system. But even in experiments where the feedback alterations are sustained, and the CNS has time to stably and slowly adapt, there are reasons why the CNS would not fully compensate. In the audio feedback alteration experiments, the CNS is receiving two types of feedback: auditory and somatosensory. If the CNS does not compensate at all for the altered audio feedback, its somatosensory feedback reports that production is fully on target, while its auditory feedback reports that production is off target. On the other hand, if the CNS compensates fully, the two feedback sources report the opposite production situation. Thus, no matter how precise the speech production system may be, there is no amount of compensation the CNS could produce that would resolve this situation; either audition or somatosensation (or both) will always report some degree of target mismatch. To compensate at all, the CNS is forced to decide how much it is willing to tolerate mismatch in each of the senses. An interesting prediction, however, from considering this situation is that if the CNS has a fixed tolerance for somatosensory mismatch but is always striving for minimal mismatch (i.e., maximal precision) in auditory feedback, then compensation should be more complete for smaller audio feedback alterations that do not require the compensatory articulations to deviate much from the somatosensory target. We explore this possibility in a recently published paper, and find that this is indeed the case: in an experiment where F1 was altered between 50 and 250 Hz, mean percent compensation across subjects increased from roughly 50% for a 250-Hz F1 shift to essentially 100% for a 50-Hz F1 shift (Katseff et al., 2011). Other recent studies have also found this pattern of more compensation for smaller formant shifts (MacDonald et al., 2010), and analogous results have been found in studies of responses to pitch feedback perturbations, where complete compensation was found for small (25 cent) pitch perturbations (Burnett et al., 1998).
The results suggest that speakers do not reduce their sensitivity to feedback deviations as those deviations get smaller. However, this poses a problem if, as described above, we put categorical limits on feedback sensitivity in order to tolerate those feedback variations arising from coarticulatory variation. How can this variability be tolerated while maintaining sensitivity to feedback deviations? As we will discuss, the answer to this question is related to another problem common to both EPC models and DIVA: neither model type takes into account the dynamical properties of the articulators (e.g., their current velocity or their momentum) when formulating commands to move them. Both types of models implicitly assume that the dynamical properties of the articulators are controlled by the lower motor system in such a way that desired articulatory trajectories are faithfully executed. EPC models have no recourse if this is not the case, while DIVA is able to detect and correct deviations from the desired trajectory (assuming they exceed the current target feature bounds), but even DIVA is not able to anticipate dynamical responses when outputting these corrective controls. This missing capacity in these models is at variance with behavior seen in actual movements, especially fast movements where articulator dynamics matter most. In controlling fast movements, the CNS behaves as if it does anticipate that the articulators will have dynamical responses to its motor commands. For example, arm movement studies have shown that fast movements are characterized by a “three-phase” muscle activation sequence, where (1) an initial burst of activation of the agonist muscle accelerates the articulator quickly toward its target, followed at about mid-movement by (2) a “breaking” burst of antagonist muscle activation that decelerates the articulator, causing it to come to rest near the target and followed in turn by (3) a weaker agonist burst to further correct the articulator’s position (Wachholder and Altenburger, 1926; Hallett et al., 1975; Shadmehr and Wise, 2005). Such activation patterns appear to take advantage of the momentum of the arm. When equilibrium points are determined for such muscle activations, they appear to follow complex trajectories, initially racing far ahead of the target position before finally converging back to it (Gomi and Kawato, 1996). Yet, in such cases, the actual trajectory of the arm is always a smooth path to the target that greatly differs from the complex equilibrium point trajectory. This mismatch suggests that even if the CNS were outputting “desired” articulatory trajectories to the lower motor system, it does so by taking into account dynamical responses to these trajectory requests, such that a fast smooth motion is achieved.
This ability of the CNS to take articulator dynamics into account can also be seen in speech production: A series of experiments have shown that speakers will learn to compensate for perturbations of jaw protrusion that are dependent on jaw velocity (Tremblay et al., 2003, 2008; Nasir and Ostry, 2008, 2009). In learning to compensate for such altered articulator dynamics, speakers show that they are formulating articulator movement commands that anticipate and cancel out the effects of those altered dynamics. Thus, the ability to anticipate articulator dynamics is not only a theoretically desirable property of a model of speech motor control, but it is actually a property required to account for real experimental results.
The Concept of Dynamical State
It turns out that having a control structure that endows the CNS with the ability to learn and anticipate dynamical responses of the articulators also endows the CNS with the ability to predict sensory feedback at a sub-categorical level. In order to make such fine-grained sensory predictions, the CNS would have to base them not simply on what the current articulatory target was, but instead on the actual articulatory commands currently being sent to the articulators – i.e., true efference copy of the descending motor commands output to the motor units of the articulators. However, without a model of how these motor commands affect articulator dynamics, accurate feedback predictions cannot be made, since it is only through their effects on the dynamics of the articulators that motor commands affect articulator positions and velocities, and thus acoustic output and somatosensory feedback from the vocal tract.
But how can we model the effects of motor commands on articulator dynamics? To say that vocal tract articulators have “dynamics” is another way of saying that how they will move in the future, and how they will react to applied controls, is dependent on their immediate past history (e.g., the direction they were last moving in). The past can only affect the future via the present, and in engineering terms, the description of the present sufficient to predict how a system’s past affects its future is called the dynamical state of the system. It is this concept of dynamical state that is basis for engineering models of systems and how they respond to applied controls.
Based on these ideas, Figure 2 illustrates how the problem of controlling speaking can be phrased in terms of the control of vocal tract state. This discrete-time description represents a snapshot of the speech motor control process at time t, where the controls ut−1 formulated at the previous timestep (t − 1) have now been applied to the muscles of the vocal tract, changing its dynamic state to xt which in turn results in the vocal tract outputting yt. In this process, xt represents an instantaneous dynamical description of the vocal tract (e.g., positions and velocities of various parts of the tongue, lips, or jaw) sufficient to predict its future behavior and vtdyn(ut−1,xt−1) expresses the physical processes (e.g., inertia) that dictate what next state xt will result from controls ut−1 being applied to prior state xt−1. The next state xt is also party determined by random disturbances wt−1 (called state noise). A key part of this formulation is that xt is not directly observable from sensory feedback. Instead, output function vtout(xt) represents all the physical and biophysical processes causing xt to generate sensory consequences yt. yt is also corrupted by noise vt and delayed by , where N is a vector of time delays representing the time taken to neurally transmit each element of yt to the higher CNS, and process it into a control-useable form (e.g., into pitch, formant frequencies, tongue height). Furthermore, certain elements of yt can be intermittently unavailable, as when auditory feedback is blocked by noise. From this description, therefore, the control of vocal tract state can be summarized as follows: How can the higher CNS correctly formulate the next controls ut to be applied to the vocal tract, given access only to previously applied controls ut−1 and noisy, delayed, and possibly intermittent feedback yt−N?
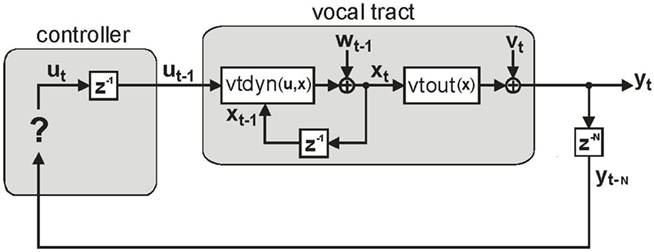
Figure 2. The control problem in speech motor control. The figure shows a snapshot at time t, when the vocal tract has produced output yt in response to the previously applied control ut−1.
A Model of Speech Motor Control Based on State Feedback
An approach to this problem is based on the following idealization shown in Figure 3: If the state xt of the vocal tract was available to the CNS via immediate feedback, then the CNS could control vocal tract state directly via feedback control. For this reason, this control approach is referred to as state feedback control (SFC). However, as discussed above, because xt is not directly observable from any type of sensory feedback, and because the sensory feedback that comes to the higher CNS is both noisy and delayed, the scheme as shown is unrealizable. As a result, a fundamental principle of SFC is that control must instead be based on a running internal estimate of the state xt (Jacobs, 1993). The first step toward getting this estimate is another idealization. Suppose, as shown in Figure 4, the higher CNS had an internal model of the vocal tract, , which had accurate forward models of the dynamics
and output function
(i.e., its acoustics, auditory, and somatosensory transformations) of the actual vocal tract. Such an internal model could mimic the response of the real vocal tract to applied controls and provide an estimate
of the actual vocal tract state. In this situation, the controller could permanently ignore the feedback yt−N of the actual vocal tract and perform ideal state feedback control
based only on
. The controls ut thus generated would correctly control both
and the actual vocal tract.
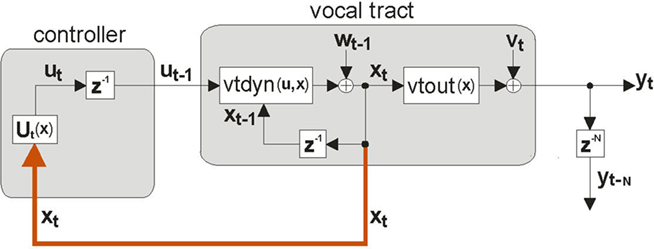
Figure 3. Ideal state feedback control. If the controller in the CNS had access to the full internal state xt of the vocal tract system (red path), it could ignore feedback yt−N and formulate a state feedback control law Ut(xt) that would optimally guide the vocal tract articulators to produce the desired speech output yt. However, as discussed in the text, the internal vocal tract state xt is, by definition, not directly available.
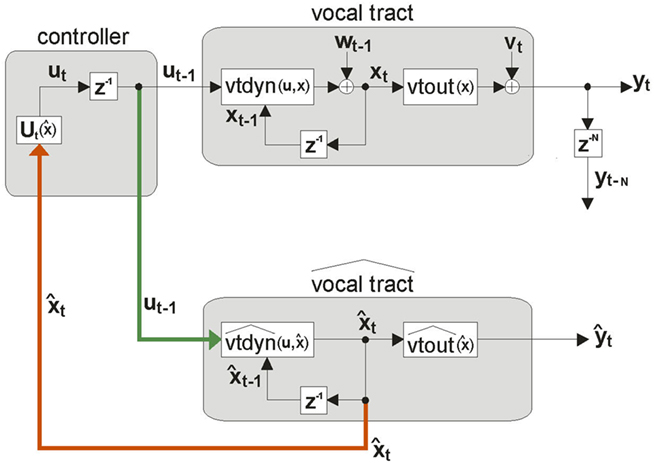
Figure 4. A more realizable model of state feedback control based on an estimate of the true internal vocal tract state xt. If the CNS had had an internal model of the vocal tract,
(comprised of dynamics model
and sensory feedback model
), it could send efference copy (green path) of vocal tract controls ut−1 to the internal model, whose state
is accessible and could be used as in place of xt in the controller’s feedback control law
(red path). However, this scheme only works if
always closely tracks xt, which is not a realistic assumption.
But this situation is still idealized: the vocal tract state xt is subject to disturbances wt−N, and the forward models and
could never be assumed to be perfectly accurate. Furthermore,
could not be assumed to start out in the same state as the actual vocal tract. Thus, without corrective help,
will not in general track xt. Unfortunately, only noisy and delayed sensory feedback yt−N is available to the controller, and yt−N is not tightly correlated with the current vocal tract state xt. Nevertheless, because yt−N is not completely uncorrelated with xt, it carries some information about xt that can be used to correct
. Figure 5 shows how this can be done by augmenting the idealization shown in Figure 4 to include the following prediction/correction process: First, in the prediction (green) direction, efference copy of the previous vocal tract control ut−1 is input to forward dynamics model
to generate a prediction
of the next vocal tract state.
is then delayed by
where
is a learned estimate of the actual sensory delays N. The resulting delayed state estimate
is input to forward output model
to generate a prediction
of the expected sensory feedback yt−N. The resulting sensory feedback prediction error
is a measure of how well
is currently tracking xt (note, for example, if
was perfectly tracking xt,
would be approximately zero). Next, in the correction (red) direction, feedback prediction error
is converted into state estimate correction
by the function
Finally,
is added to the original next state prediction
to derive the corrected state estimate
. By this process, therefore, an accurate estimate of the true vocal tract state xt can be derived in a feasible way and used by the state feedback control law
to determine the next controls ut output to the vocal tract.
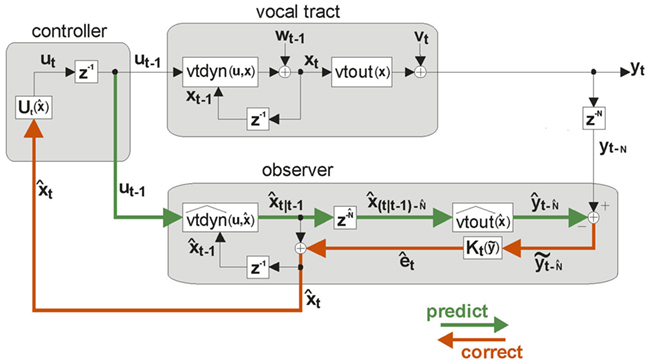
Figure 5. State feedback control (SFC) model of speech motor control. The model is similar to that depicted in Figure 4 (i.e., the forward models and
constitute the internal model of the vocal tract
shown in Figure 4), but here sensory feedback is used to keep the state estimate
tracking the true vocal tract state xt. This is accomplished with a prediction/correction process in which, in the prediction (green) direction, efference copy of vocal motor commands ut−1 are passed through dynamics model
to generate next state prediction
, which is delayed by
.
outputs the next state prediction
from
seconds ago, in order to match the sensory transduction delay of N seconds.
is passed through sensory feedback model
to generate feedback prediction
. Then, in the correction (red) direction, incoming sensory feedback yt−N is compared with prediction
, resulting in sensory feedback prediction error
.
is converted by Kalman gain function
into state correction
, which is added to
to make corrected state estimate
. Finally, as in Figure 4,
is used by state feedback control law
in the controller to generate the controls ut that will be applied at the next timestep to the vocal tract.
As Figure 5 indicates, the combination of plus this feedback-based correction process is called an observer (Jacobs, 1993; Stengel, 1994; Wolpert, 1997; Tin and Poon, 2005), which in this case, because it includes allowances for feedback delays, is also a variant of a Smith Predictor (Smith, 1959; Miall et al., 1993; Mehta and Schaal, 2002). Within the observer,
converts changes in feedback to changes in state. When it is optimally determined,
is a feedback gain proportional to how correlated the feedback prediction error
is with the state prediction error
Thus, if
is highly uncorrelated with
– as happens with large feedback delays or feedback being blocked –
largely attenuates the influence of feedback prediction errors on correcting the current state estimate. When
is so optimally determined, it is referred to as the Kalman gain function and the observer is referred to as a Kalman filter (Kalman, 1960; Jacobs, 1993; Stengel, 1994; Todorov, 2006). We will also refer to
as the Kalman gain function because we assume the speech motor control system would seek an optimal value for this function.
State feedback control (SFC), therefore, is the combination of a control law acting on a state estimate provided by an observer. This is a relatively new way to model speech motor control, but SFC models are well-known in other areas of motor control research. Interest in SFC models of motor control has a long history that can trace its roots all the way back to Nikolai Bernstein, who suggested that the CNS would need to take into account the current state of the body (both the nervous system and articulatory biomechanics) in order to know the sensory outcomes of motor commands it issued (Bernstein, 1967; Whiting, 1984). Since then, the problem of motor control has been formulated in state-space terms like those discussed above (Arbib, 1981), and observer-based SFC models of reaching motor control have been advanced to explain how people optimize their movements (Todorov and Jordan, 2002; Todorov, 2004; Guigon et al., 2008; Shadmehr and Krakauer, 2008). More generally, the SFC model can also be viewed as a type of linear Gaussian model which has deep connections with statistical learning theory. In this framework, the state estimation process of the Kalman filter described above has been shown to be a type of Bayesian inference (Roweis and Ghahramani, 1999) that can be accomplished using variational free-energy optimization principles (Friston, 2010), which appears to be a ubiquitous computational approach that applies to many computational problems in neuroscience.
Is SFC Neurally Plausible?
For speech, the SFC model suggests that not only is auditory processing used by the CNS for comprehension during listening, but that the CNS also uses auditory information in a distinctly different way during speech production: it is compared with a prediction derived from efference copy of motor output, with the resulting prediction error used to keep an internal model tracking the state of the vocal tract. There are a number of lines of evidence supporting the neural plausibility of this second, production-specific mode of sensory processing. First, even in other primates, there appear to be at least two distinct pathways, or streams, of auditory processing. The concept of multiple sensory processing streams was first advanced for the visual system, with a dorsal “where” stream leading to parietal cortex that is concerned with object location, and a ventral “what” stream leading to the temporal pole concerned with object recognition (Mishkin et al., 1983). Subsequently, studies of the auditory system found a match to this visual system organization. Neurons responding to auditory source location were found in a dorsal pathway leading up to parietal cortex, and neurons responding to auditory source type were found in a ventral pathway leading down toward the temporal pole (Rauschecker and Tian, 2000). More recent evidence, however, has refined the view of the dorsal stream’s task to be one of sensorimotor integration. The dorsal visual stream was found to be closely linked with motor control systems (e.g., reaching, head, and eye movement control; Andersen, 1997; Rizzolatti et al., 1997), while, in humans, the dorsal auditory stream was found to be closely linked with the vocal motor control system. In particular, a variety of studies have implicated the posterior superior temporal gyrus (STG; Zheng et al., 2009) and the superior parietal temporal area (Spt; Buchsbaum et al., 2001; Hickok et al., 2003) as serving feedback processing specifically related to speech production. Consistent with this, studies of stroke victims have shown a double dissociation between ability to perform discreet production-related perceptual judgments and ability to understand continuous speech that depends on lesion location (dorsal and ventral stream lesions, respectively; Miceli et al., 1980; Baker et al., 1981). This has led to refined looped and “dual stream” models of speech processing (Hickok and Poeppel, 2007; Rauschecker and Scott, 2009; Hickok et al., 2011) with a ventral stream serving speech comprehension and a dorsal stream serving feedback processing related to speaking.
When this production-oriented auditory processing of the dorsal stream is disrupted, a number of speech sensorimotor disorders appear to result (Hickok et al., 2011). Conduction aphasia is a neurological condition resulting from stroke in which production and comprehension of speech is preserved but the ability to repeat speech sound sequences just heard is impaired (Geschwind, 1965). Conduction aphasia appears to result from damage to area spt in the dorsal auditory processing stream (Buchsbaum et al., 2011). Consistent with this, the impairment is particularly apparent in the task of repeating nonsense speech sounds, because when the sound sequences do not form meaningful words, the intact speech comprehension system (the ventral stream) cannot aid in remembering what was heard. More speculatively, stuttering may also result from impairments in auditory feedback processing in the dorsal stream. It is well-known that altering auditory feedback (e.g., altering pitch (Howell et al., 1987), masking feedback with noise (Maraist and Hutton, 1957), and delaying auditory feedback (DAF) (Soderberg, 1968)) can make many persons who stutter speak fluently. Evidence for dorsal stream involvement in these fluency enhancements comes from a study relating DAF-induced fluency to structural MRIs of the brains of persons who stutter (Foundas et al., 2004). The planum temporale (PT) is an area of temporal cortex encompassing dorsal stream areas like spt, and the study found that right PT was aberrantly larger than left PT in those stutterers whose fluency was enhanced by DAF. Several other anatomical studies have also implicated dorsal stream dysfunction in stuttering, including studies showing impaired white matter connectivity in this region (Cykowski et al., 2010), as well as aberrant gyrification patterns (Foundas et al., 2001).
There are a number of studies that have found evidence that production-specific feedback processing involves comparison of incoming feedback with a feedback prediction derived from motor efference copy. Non-speech evidence for this is seen when a robot creates delay between the tickle action subjects produce and when they feel it on their own hand (Blakemore et al., 1998, 1999, 2000). With increasing delay, subjects report a more ticklish sensation, as expected if the delay created mismatch between a sensory prediction derived from the tickle action and the actual somatosensory feedback. By using different neuroimaging techniques, an analogous effect can be seen in speech production: the response of a subject’s auditory cortices to his/her own self-produced speech is significantly smaller than their response to similar, but externally produced speech (e.g., tape playback of the subject’s previous self-productions). This effect, which we call speaking-induced suppression (SIS), has been seen using positron emission tomography (PET; Hirano et al., 1996, 1997a,b), electroencephalography (EEG; Ford et al., 2001; Ford and Mathalon, 2004), and magnetoencephalography (MEG) (Numminen and Curio, 1999; Numminen et al., 1999; Curio et al., 2000; Houde et al., 2002; Heinks-Maldonado et al., 2006; Ventura et al., 2009). An analog of the SIS effect has also been seen in non-human primates (Eliades and Wang, 2003, 2005, 2008). Our own MEG experiments have shown that the SIS effect is only minimally explained by a general suppression of auditory cortex during speaking and that this suppression is not happening in the more peripheral parts of the CNS (Houde et al., 2002). We have also shown that the observed suppression goes away if the subject’s feedback is altered to mismatch his/her expectations (Houde et al., 2002; Heinks-Maldonado et al., 2006), as is consistent with some of the PET study findings. Finally, if SIS depends on a precise match between feedback and prediction, then precise time alignment of prediction with feedback would be critical for complex rapidly changing productions (e.g., rapidly speaking “ah-ah-ah”), and less critical for slow or static productions (e.g., speaking “ah”). Assuming a given level of time alignment inaccuracy, the prediction/feedback match should therefore be better (and SIS stronger) for slower, less dynamic productions, which is what we found in a recent study (Ventura et al., 2009).
By itself, evidence of feedback being compared with a prediction derived from efference copy implies the existence of predictive forward models within the CNS, but another line of evidence for forward models comes from sensorimotor adaptation experiments (Wolpert et al., 1995; Ghahramani et al., 1996; Wolpert and Ghahramani, 2000). Such experiments have been conducted with speech production, where subjects are shown to alter and then retain compensatory production changes in response to extended exposure to artificially altered audio feedback (Houde and Jordan, 1997, 1998, 2002; Jones et al., 1998; Jones and Munhall, 2000a,b, 2002, 2003, 2005; Purcell and Munhall, 2006; Villacorta et al., 2007; Shiller et al., 2009) or altered somatosensory feedback (Tremblay et al., 2003, 2008; Nasir and Ostry, 2006, 2009, 2008). For example, in the original speech sensorimotor adaptation experiment, subjects produced the vowel /ε/ (as in “head”), first hearing normal audio feedback and then hearing their formants shifted toward /i/ (as in “heed”). Over repeated productions while hearing the altered feedback, subjects gradually shifted their productions of /ε/ in the opposite direction; i.e., they shifted their produced formants toward /a/ (as in “hot”). This had the effect of making the altered feedback sound more like /ε/ again. These changes in the production of /ε/ were retained even when feedback was subsequently blocked by noise (Houde and Jordan, 1997, 1998, 2002). The retained production changes are consistent with the existence of a forward model making feedback predictions that are modified by experience. In addition to providing evidence for forward models, such adaptation experiments also allow investigation of the organization of forward models in the speech production system. By examining how compensation trained in the production of one phonetic task (e.g., the production of /ε/) generalizes to another untrained phonetic task (e.g., the production of /a/), such experiments can determine if there are shared representations like forward models used in the control of both tasks. Some of these experiments have found generalization of adaptation across speech tasks (Houde and Jordan, 1997, 1998; Jones and Munhall, 2005), but other experiments have not found such generalization (Pile et al., 2007; Tremblay et al., 2008), suggesting that, in many cases, forward models used in the control of different speech tasks are perhaps not shared across tasks.
Based partly on these study results, Figure 6 suggests a putative neural substrate for the SFC model, while Figure 7 shows the anatomical locations of the suggested substrate. Basic neuroanatomical facts dictate the neural substrates on both ends of the SFC prediction/correction processing loop. On one end of the loop, motor cortex (M1) is the likely area where the feedback control law generates neuromuscular controls applied to the vocal tract. Motor cortex is the main source of motor fibers of the pyramidal tract, which synapse directly with motor neurons in the brainstem and spinal cord and enable fine motor movements (Kandel et al., 2000). As mentioned above, damage to the vocal tract areas of motor cortex often results in mutism (Jürgens, 2002; Duffy, 2005). On the other end of the loop, auditory and somatosensory information first reaches the higher CNS in the primary auditory (A1) and somatosensory (S1) cortices, respectively (Kandel et al., 2000). Based on our SIS studies (see above), we hypothesize this end of the loop is where the operation comparing the feedback prediction with incoming feedback occurs. Between these endpoints, the model also predicts the need for an additional area that mediates the prediction (green) and correction (red) processes running between motor and the sensory cortices. The premotor cortices are ideally placed for such an intermediary role: premotor cortex is both bidirectionally well connected to motor cortex (Kandel et al., 2000), and, via the arcuate and longitudinal fasciculi (Schmahmann et al., 2007; Glasser and Rilling, 2008; Upadhyay et al., 2008), bidirectionally connected to the higher order auditory (Spt/PT) and somatosensory (S2/PV) cortices, respectively. In this way, the key parts of the SFC model are a good fit for a known network of sensorimotor areas that are, in turn, well placed to receive task-dependent, modulatory connections (blue dashed arrows in Figure 6) from other frontal areas.
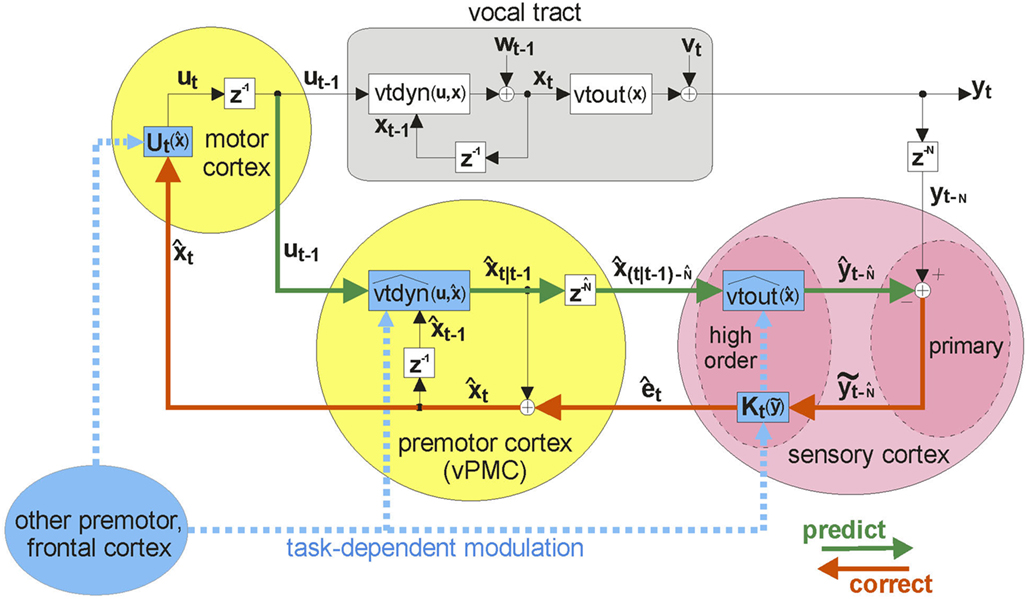
Figure 6. State feedback control (SFC) model of speech motor control with putative neural substrate. The figure depicts the same operations as those shown in Figure 5, but with suggested cortical locations of the operations (motor areas are in yellow, while sensory areas are in pink). The current model is largely agnostic regarding hemispheric specialization for these operations. Also, for diagrammatic simplicity, the operations in the auditory and somatosensory cortices are depicted in the single area marked “sensory cortex,” with the understanding that it represents analogous operations occurring in both of these sensory cortices: i.e., the delayed state estimate is sent to both high order somatosensory and auditory cortex, each with separate feedback prediction modules (
for predicting auditory feedback in high order auditory cortex and
for predicting somatosensory feedback in high order somatosensory cortex. The feedback prediction errors
generated in auditory and somatosensory cortex are converted into separate state corrections
based on auditory and somatosensory feedback by auditory and somatosensory Kalman gain functions
in high the order auditory and somatosensory cortices, respectively. The auditory- and somatosensory-based state corrections are then added to
in premotor cortex to make next state estimate
. Finally, the key operations depicted in blue are all postulated to be modulated by the current speech task goals (e.g., what speech sound is currently meant to be produced) that are expressed in other areas of frontal cortex.
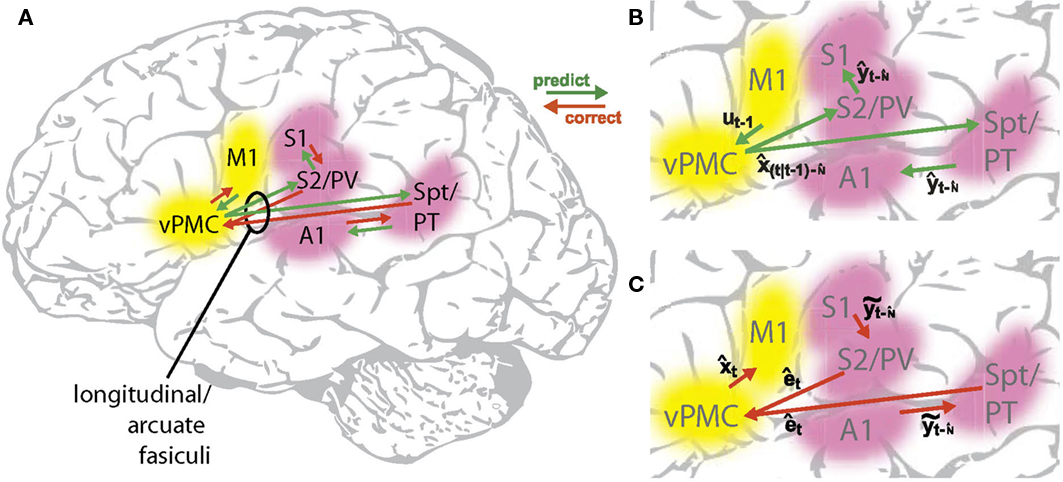
Figure 7. Cortical substrate of SFC model. (A) Anatomical locations of candidate cortical areas and white matter tracts comprising network of the core SFC model. The same color scheme used in Figure 6 is used here: motor areas are in yellow, while sensory areas are in pink; connections conveying predictive information are in green, while those conveying corrective information are in red. Here, however, the single depiction of sensory cortex made up of primary and higher-level areas shown in Figure 6 is shown here in more detail as a parallel organization of primary (A1, S1) and higher-level (Spt/PT, S2/PV) auditory and somatosensory cortices. The main white matter tracts that bidirectionally connect premotor cortex with the higher auditory and somatosensory cortices are hypothesized to be the arcuate and longitudinal fasiculi, respectively. Note that although, for simplicity, only the neural substrate in the left hemisphere is shown here, we would expect the full network of the neural substrate to include analogous areas in the right hemisphere as well. At this point, the SFC model is agnostic regarding hemispheric dominance in the proposed neural substrate. (B) Cortical connections in the prediction (green) direction: Efference copy of the neuromuscular controls ut−1 generated in motor cortex (M1) and sent to the vocal tract motor neurons are also sent to premotor cortex (vPMC), which uses this to generate state prediction that it sends to both the high level auditory (Spt/PT) and somatosensory (S2/PV) cortices. These higher-level sensory areas in turn use
to generate feedback predictions
, which they send to their associated primary sensory areas (A1,S1), where these predictions are compared with incoming feedback. (C) Cortical connections in the correction (red) direction: By comparing feedback predictions with incoming feedback, the primary sensory areas (A1,S1) compute feedback prediction errors
that are sent back from the to the higher-level sensory areas (Spt/PT, S2/PV), where they are converted into state estimate corrections
that are sent back to premotor cortex (vPMC). Finally, in premotor cortex these corrections are added to the state prediction, making the corrected state estimate
sent back to motor cortex (M1), which uses
along with current task goals to generate further neuromuscular commands sent to the vocal tract motor neurons.
What evidence is there for premotor cortex playing such an intermediary role in speech production? First, reciprocal connections with sensory areas suggest the possibility that premotor cortex could also be active during passive listening to speech, and indeed this appears to be the case. Wilson et al. (2004) found the superior ventral premotor area (svPMC), bilaterally was activated by both listening to and speaking meaningless syllables, but not listening to non-speech sounds. In a follow-up study, Wilson et al. (2004) found that this area, bilaterally, showed greater activation when subjects heard speech sounds they rated as un-producible than when they heard sounds they rated as producible. In this same study, auditory areas were also activated more for speech sounds rated least producible, and that svPMC was functionally connected to these auditory areas during listening (Wilson and Iacoboni, 2006). This activation of premotor cortex when speech is heard has also been seen in other functional imaging studies (Skipper et al., 2005) and studies based on TMS (Watkins and Paus, 2004).
Second, altering sensory feedback during speech production should create feedback prediction errors in sensory cortices, increasing activations in these areas, and the resulting state estimate corrections should be passed back to premotor cortex, increasing its activation as well. A study that tested this prediction was carried out by Tourville et al. (2008), where they used fMRI to examine how cortical activations changed when subjects spoke with their auditory feedback altered. In the study, subjects spoke simple CVC words with the frequency of first formant occasionally altered in their audio feedback of some of their productions. When they looked for areas more active in altered feedback versus non-altered trials, Tourville et al. (2008) found auditory areas (pSTG in both hemispheres), and they also found areas in the right frontal cortex: a motor area (vMC), a premotor area (vPMC), and an area (IFt) in the inferior frontal gyrus, pars triangularis (Broca’s) region. When they looked at the functional connectivity of these right frontal areas, they found that the presence of the altered feedback significantly increased the functional connectivity only of the left and right auditory areas, as well as the functional connectivity of these auditory areas with vPMC and IFt. The result suggests that the auditory feedback correction information from higher auditory areas has a bigger effect on premotor/pars triangularis regions than motor cortex regions, which is consistent with our SFC model if we expand the neural substrate of our state estimation process beyond premotor cortex to also include Broca’s area. The results of Tourville et al. (2008) are partly confirmed by another fMRI study. Toyomura et al. (2007) had subjects continuously phonate a vowel, and on some trials, the pitch of the subjects’ audio feedback was briefly perturbed higher or lower by two semitones. In examining the contrast between perturbed and unperturbed trials, Toyomura et al. (2007) found premotor activation in the left hemisphere, and a number of activations in the right hemisphere, including auditory cortex (STG) and frontal area BA9, which is nearby the IFt activation found by Tourville et al. (2008).
In the discussion above, we have limited our consideration to the very lowest levels of the speech production process – i.e., where muscle commands are generated to produce a chosen speech sound or syllable (e.g., like /ba/). However, other researchers have considered the anatomical substrate of the speech production process at higher levels – i.e., where speech sound sequences are generated and words are chosen to produce. For example, a careful study and literature review by Eickhoff et al. (2009) showed that, in the word production process, premotor cortex is not only a functional intermediary between sensory and motor cortex, but is also a key intermediary between higher-level speech areas (e.g., Broca’s area) and motor cortex. The study also showed that during speaking, premotor cortex is functionally connected with a larger complex of structures including the insula, basal ganglia, and cerebellum – all of which also have the capability to integrate sensory feedback with motor output (Huang et al., 1991; Yeterian and Pandya, 1998; Ackermann and Riecker, 2004). Thus, it is quite possible that the feedback processing role we have hypothesized for premotor cortex alone is actually supported by a larger network of areas. It is also plausible that other areas may process feedback in a manner similar to premotor cortex, but at hierarchically higher levels of the speech production process. These may include other premotor areas, like the supplementary motor area (SMA), which are thought to play a role in the sequencing of syllables, or their sub-syllabic components (Riecker et al., 2008).
Conclusion
In this review, the applicability of SFC to modeling speech motor control has been explored. The phenomena related to the CNS’s role in speech production, especially its role in processing sensory feedback, are complex, and suggest that speech motor control is not an example of pure feedback control or feedforward control. The task-specificity of responses to feedback perturbations in speech further argues that feedback control is not only a function of the lower motor system, but that the CNS plays an active role in the online processing of sensory feedback during speaking. Considering the probable uses of this processing (i.e., responding to changes in vocal tract characteristics, compensating for perturbations), it is likely that the CNS processes feedback not only in the categorical manner used in speech recognition, but also at a sub-categorical level where deviations from expected feedback could be detected in ongoing speaking.
All of these characteristics put constraints on models of the role of the CNS in the speech motor control process. Existing models account for some of these characteristics, but all have two important and related limitations: (1) the precise sensory consequences of motor commands to the vocal articulators cannot be predicted and (2) the dynamics of the articulators cannot be taken into account in formulating vocal tract controls. The key missing concept that allows these limitations to be overcome (which evidence suggests that the CNS is able to do) is the concept of dynamical state: dynamical state relates applied controls to their sensory consequences, and by keeping track of the dynamical state of the vocal articulators, their dynamics can be taken into account in formulating controls. The feasible way of incorporating this concept in a model of motor control is the SFC model, which is advanced as the most appropriate and neurally plausible model of how the CNS processes feedback and controls the vocal tract. It is concluded, therefore, that modeling speech motor control as an SFC process is not only possible but also sufficiently plausible that future experiments in speech motor control should be designed to test it.
Conflict of Interest Statement
The authors declare that the research was conducted in the absence of any commercial or financial relationships that could be construed as a potential conflict of interest.
Acknowledgments
We thank Greg Hickok and Keith Johnson for helpful comments. This work was funded by NIH grants RO1 DC006435, R01 DC010145, and by NSF grant BCS-0926196.
References
Abbs, J. H., and Gracco, V. L. (1983). Sensorimotor actions in the control of multi-movement speech gestures. Trends Neurosci. 6, 391.
Abbs, J. H., and Gracco, V. L. (1984). Control of complex motor gestures: orofacial muscle responses to load perturbations of lip during speech. J. Neurophysiol. 51, 705–723.
Ackermann, H., and Riecker, A. (2004). The contribution of the insula to motor aspects of speech production: a review and a hypothesis. Brain Lang. 89, 320–328.
Andersen, R. A. (1997). Multimodal integration for the representation of space in the posterior parietal cortex. Philos. Trans. R. Soc. Lond. B Biol. Sci., 352, 1421–1428.
Arbib, M. A. (1981). “Perceptual structures and distributed motor control,” in Handbook of Physiology, Section 1: The Nervous System, Volume 2: Motor Control, Part 2, eds J. M. Brookhart, V. B. Mountcastle, and V. B. Brooks (Bethesda, MD: American Phsyiological Society), 1449–1480.
Asatryan, D. G., and Feldman, A. G. (1965). Biophysics of complex systems and mathematical models. Functional tuning of nervous system with control of movement or maintenance of a steady posture. I. Mechanographic analysis of the work of the joint on execution of a postural task. Biophysics 10, 925–935.
Baker, E., Blumstein, S. E., and Goodglass, H. (1981). Interaction between phonological and semantic factors in auditory comprehension. Neuropsychologia 19, 1–15.
Bendor, D., and Wang, X. (2005). The neuronal representation of pitch in primate auditory cortex. Nature 436, 1161–1165.
Bizzi, E., Accornero, N., Chapple, W., and Hogan, N. (1982). Arm trajectory formation in monkeys. Exp. Brain Res. 46, 139–143.
Blakemore, S. J., Wolpert, D. M., and Frith, C. D. (1998). Central cancellation of self-produced tickle sensation. Nat. Neurosci. 1, 635–640.
Blakemore, S. J., Wolpert, D. M., and Frith, C. D. (1999). The cerebellum contributes to somatosensory cortical activity during self-produced tactile stimulation. Neuroimage 10, 448–459.
Blakemore, S. J., Wolpert, D. M., and Frith, C. D. (2000). Why can’t you tickle yourself? Neuroreport 11, R11–R16.
Blevins, J. (2004). Evolutionary Phonology: The Emergence of Sound Patterns. Cambridge, UK: Cambridge University Press.
Borden, G. J., Harris, K. S., and Raphael, L. J. (1994). Speech Science Primer: Physiology, Acoustics, and Perception of Speech, 3rd Edn. Baltimore: Williams & Wilkins.
Buchsbaum, B. R., Baldo, J., Okada, K., Berman, K. F., Dronkers, N., D’Esposito, M., and Hickok, G. (2011). Conduction aphasia, sensory-motor integration, and phonological short-term memory – an aggregate analysis of lesion and fMRI data. Brain Lang. doi: 10.1016/j.bandl.2010.12.001. [Epub ahead of print].
Buchsbaum, B. R., Hickok, G., and Humphries, C. (2001). Role of left posterior superior temporal gyrus in phonological processing for speech perception and production. Cogn. Sci. 25, 663–678.
Burnett, T. A., Freedland, M. B., Larson, C. R., and Hain, T. C. (1998). Voice F0 responses to manipulations in pitch feedback. J. Acoust. Soc. Am. 103, 3153–3161.
Chang-Yit, R., Pick, J., Herbert, L., and Siegel, G. M. (1975). Reliability of sidetone amplification effect in vocal intensity. J. Commun. Disord. 8, 317–324.
Cheung, S. W., Nagarajan, S. S., Schreiner, C. E., Bedenbaugh, P. H., and Wong, A. (2005). Plasticity in primary auditory cortex of monkeys with altered vocal production. J. Neurosci. 25, 2490–2503.
Cowie, R., and Douglas-Cowie, E. (1992). Postlingually Acquired Deafness: Speech Deterioration and the Wider Consequences. Hawthorne, NY: Mouton de Gruyter.
Curio, G., Neuloh, G., Numminen, J., Jousmaki, V., and Hari, R. (2000). Speaking modifies voice-evoked activity in the human auditory cortex. Hum. Brain Mapp. 9, 183–191.
Cykowski, M. D., Fox, P. T., Ingham, R. J., Ingham, J. C., and Robin, D. A. (2010). A study of the reproducibility and etiology of diffusion anisotropy differences in developmental stuttering: a potential role for impaired myelination. Neuroimage 52, 1495–1504.
Duffy, J. R. (2005). Motor Speech Disorders: Substrates, Differential Diagnosis, and Management, 2nd Edn. Saint Louis, MO: Elsevier Mosby.
Eickhoff, S. B., Heim, S., Zilles, K., and Amunts, K. (2009). A systems perspective on the effective connectivity of overt speech production. Philos. Trans. R. Soc. A Math. Phys. Eng. Sci. 367, 2399–2421.
Eliades, S. J., and Wang, X. (2003). Sensory-motor interaction in the primate auditory cortex during self-initiated vocalizations. J. Neurophysiol. 89, 2194–2207.
Eliades, S. J., and Wang, X. (2005). Dynamics of auditory-vocal interaction in monkey auditory cortex. Cereb. Cortex 15, 1510–1523.
Eliades, S. J., and Wang, X. (2008). Neural substrates of vocalization feedback monitoring in primate auditory cortex. Nature 453, 1102–1106.
Elman, J. L. (1981). Effects of frequency-shifted feedback on the pitch of vocal productions. J. Acoust. Soc. Am. 70, 45–50.
Fairbanks, G. (1954). Systematic research in experimental phonetics: 1. A theory of the speech mechanism as a servosystem. J. Speech Hear. Disord. 19, 133–139.
Feldman, A. G. (1986). Once more on the equilibrium-point hypothesis (lambda model) for motor control. J. Mot. Behav. 18, 17–54.
Ford, J. M., and Mathalon, D. H. (2004). Electrophysiological evidence of corollary discharge dysfunction in schizophrenia during talking and thinking. J. Psychiatr. Res. 38, 37–46.
Ford, J. M., Mathalon, D. H., Heinks, T., Kalba, S., Faustman, W. O., and Roth, W. T. (2001). Neurophysiological evidence of corollary discharge dysfunction in schizophrenia. Am. J. Psychiatry 158, 2069–2071.
Foundas, A. L., Bollich, A. M., Corey, D. M., Hurley, M., and Heilman, K. M. (2001). Anomalous anatomy of speech-language areas in adults with persistent developmental stuttering. Neurology 57, 207–215.
Foundas, A. L., Bollich, A. M., Feldman, J., Corey, D. M., Hurley, M., Lemen, L. C., and Heilman, K. M. (2004). Aberrant auditory processing and atypical planum temporale in developmental stuttering. Neurology 63, 1640–1646.
Franklin, G. F., Powell, J. D., and Emami-Naeini, A. (1991). Feedback Control of Dynamic Systems, 2nd Edn. Reading, MA: Addison-Wesley.
Friston, K. (2010). The free-energy principle: a unified brain theory? Nat. Rev. Neurosci., 11, 127–138.
Ghahramani, Z., Wolpert, D. M., and Jordan, M. I. (1996). Generalization to local remappings of the visuomotor coordinate transformation. J. Neurosci. 16, 7085–7096.
Ghosh, S. S. (2004). Understanding Cortical and Cerebellar Contributions to Speech Production Through Modeling and Functional Imaging. Ph. D. dissertation, Boston University, Boston, MA.
Glasser, M. F., and Rilling, J. K. (2008). DTI tractography of the human brain’s language pathways. Cereb. Cortex 18, 2471–2482.
Godey, B., Atencio, C. A., Bonham, B. H., Schreiner, C. E., and Cheung, S. W. (2005). Functional organization of squirrel monkey primary auditory cortex: responses to frequency-modulation sweeps. J. Neurophysiol. 94, 1299–1311.
Gomi, H., and Kawato, M. (1996). Equilibrium-point control hypothesis examined by measured arm stiffness during multijoint movement. Science 272, 117–120.
Guenther, F. H. (1995). Speech sound acquisition, coarticulation, and rate effects in a neural network model of speech production. Psychol. Rev. 102, 594–621.
Guenther, F. H. (2008). Involvement of auditory cortex in speech production. Paper presented at the 155th Meeting of the Acoustical Society of America, and 9th Congrès Français d’Acoustique, Paris.
Guenther, F. H., Ghosh, S. S., and Tourville, J. A. (2006). Neural modeling and imaging of the cortical interactions underlying syllable production. Brain Lang. 96, 280–301.
Guenther, F. H., Hampson, M., and Johnson, D. (1998). A theoretical investigation of reference frames for the planning of speech movements. Psychol. Rev. 105, 611–633.
Guigon, E., Baraduc, P., and Desmurget, M. (2008). Optimality, stochasticity, and variability in motor behavior. J. Comput. Neurosci. 24, 57–68.
Hallett, M., Shahani, B. T., and Young, R. R. (1975). EMG analysis of stereotyped voluntary movements in man. J. Neurol. Neurosurg. Psychiatry 38, 1154–1162.
Hardcastle, W. J., and Hewlett, N. (2006). Coarticulation: Theory, Data and Techniques. Cambridge: Cambridge University Press.
Hayes, B., and Steriade, D. (2004). “Introduction: the phonetic bases of phonological Markedness,” in Phonetically based phonology, eds B. Hayes, R. M. Kirchner, and D. Steriade (Cambridge, NY: Cambridge University Press), 1–33.
Heil, P. (2003). Coding of temporal onset envelope in the auditory system. Speech Commun. 41, 123–134.
Heil, P., and Irvine, D. R. (1996). On determinants of first-spike latency in auditory cortex. Neuroreport 7, 3073–3076.
Heinks-Maldonado, T. H., Nagarajan, S. S., and Houde, J. F. (2006). Magnetoencephalographic evidence for a precise forward model in speech production. Neuroreport 17, 1375–1379.
Henke, W. L. (1966). Dynamic Articulatory Model of Speech Production Using Computer Simulation. Ph.D. thesis, MIT, Cambridge, MA.
Hickok, G., Buchsbaum, B., Humphries, C., and Muftuler, T. (2003). Auditory-motor interaction revealed by fMRI: speech, music, and working memory in area Spt. J. Cogn. Neurosci. 15, 673–682.
Hickok, G., Houde, J. F., and Rong, F. (2011). Sensorimotor integration in speech processing: computational basis and neural organization. Neuron 69, 407–422.
Hickok, G., and Poeppel, D. (2007). The cortical organization of speech processing. Nat. Rev. Neurosci. 8, 393–402.
Hill, A. V. (1925). Length of muscle, and the heat and tension developed in an isometric contraction. J. Physiol. (Lond.) 60, 237–263.
Hirano, S., Kojima, H., Naito, Y., Honjo, I., Kamoto, Y., Okazawa, H., and Konishi, J. (1996). Cortical speech processing mechanisms while vocalizing visually presented languages. Neuroreport 8, 363–367.
Hirano, S., Kojima, H., Naito, Y., Honjo, I., Kamoto, Y., Okazawa, H., and Konishi, J. (1997a). Cortical processing mechanism for vocalization with auditory verbal feedback. Neuroreport 8, 2379–2382.
Hirano, S., Naito, Y., Okazawa, H., Kojima, H., Honjo, I., Ishizu, K., and Konishi, J. (1997b). Cortical activation by monaural speech sound stimulation demonstrated by positron emission tomography. Exp. Brain Res. 113, 75–80.
Houde, J. F., and Jordan, M. I. (1997). “Adaptation in speech motor control,” in Advances in Neural Information Processing Systems, Vol. 10, eds M. I. Jordan, M. J. Kearns, and S. A. Solla (Cambridge, MA: MIT Press), 38–44.
Houde, J. F., and Jordan, M. I. (1998). Sensorimotor adaptation in speech production. Science 279, 1213–1216.
Houde, J. F., and Jordan, M. I. (2002). Sensorimotor adaptation of speech I: compensation and adaptation. J. Speech Lang. Hear. Res. 45, 295–310.
Houde, J. F., Nagarajan, S. S., Sekihara, K., and Merzenich, M. M. (2002). Modulation of auditory cortex during speech: an MEG study. J. Cogn. Neurosci. 14, 1125–1138.
Howell, P., El-Yaniv, N., and Powell, D. J. (1987). “Factors affecting fluency in stutterers when speaking under altered auditory feedback” in Speech Motor Dynamics in Stuttering, eds H. F. Peters, and W. Hulstijn (New York, NY: Springer Press), 361–369.
Huang, C. M., Liu, G. L., Yang, B. Y., Mu, H., and Hsiao, C. F. (1991). Auditory receptive area in the cerebellar hemisphere is surrounded by somatosensory areas. Brain Res. 541, 252–256.
Hulliger, M. (1984). The mammalian muscle spindle and its central control. Rev. Physiol. Biochem. Pharmacol. 101, 1–110.
Ito, T., Kimura, T., and Gomi, H. (2005). The motor cortex is involved in reflexive compensatory adjustment of speech articulation. Neuroreport 16, 1791–1794.
Jacobs, O. L. R. (1993). Introduction to Control Theory, 2nd Edn. Oxford, UK: Oxford University Press.
Jones, J. A., and Munhall, K. G. (2000a). Perceptual calibration of F0 production: evidence from feedback perturbation. J. Acoust. Soc. Am. 108(3 Pt 1), 1246–1251.
Jones, J. A., and Munhall, K. G. (2000b). Perceptual contributions to fundamental frequency production. Paper presented at the 5th Seminar on Speech Production: Models and Data, Kloster Seeon.
Jones, J. A., and Munhall, K. G. (2002). The role of auditory feedback during phonation: studies of Mandarin tone production. J. Phon. 30, 303–320.
Jones, J. A., and Munhall, K. G. (2003). Learning to produce speech with an altered vocal tract: the role of auditory feedback. J. Acoust. Soc. Am. 113, 532–543.
Jones, J. A., and Munhall, K. G. (2005). Remapping auditory-motor representations in voice production. Curr. Biol. 15, 1768–1772.
Jones, J. A., Munhall, K. G., and Vatikiotis-Bateson, E. (1998). Adaptation to altered feedback in speech. Paper presented at the The 136th Meeting of the Acoustical Society of America, Norfolk, VA.
Jürgens, U., Kirzinger, A., and von Cramon, D. (1982). The effects of deep-reaching lesions in the cortical face area on phonation. A combined case report and experimental monkey study. Cortex 18, 125–139.
Kalman, R. E. (1960). A new approach to linear filtering and prediction problems. Trans. ASME J. Basic Eng. 82, 35–45.
Kalveram, K. T., and Jancke, L. (1989). Vowel duration and voice onset time for stressed and nonstressed syllables in stutterers under delayed auditory feedback condition. Folia Phoniatr. (Basel) 41, 30–42.
Kandel, E. R., Schwartz, J. H., and Jessell, T. M. (2000). Principles of Neural Science, 4th Edn. New York: McGraw-Hill.
Katseff, S., Houde, J. F., and Johnson, K. (2011). Partial compensation for altered auditory feedback: a tradeoff with somatosensory feedback? Lang. Speech (in press).
Kawato, M., Furukawa, K., and Suzuki, R. (1987). A hierarchical neural-network model for control and learning of voluntary movement. Biol. Cybern. 57, 169–185.
Kawato, M., and Gomi, H. (1992). A computational model of four regions of the cerebellum based on feedback-error learning. Biol. Cybern. 68, 95–103.
Kelso, J. S., Tuller, B., Vatikiotis-Bateson, E., and Fowler, C. A. (1984). Functionally specific articulatory cooperation following jaw perturbations during speech: evidence for coordinative structures. J. Exp. Psychol. Hum. Percept. Perform. 10, 812–832.
Kent, R. D., and Minifie, F. D. (1977). Coarticulation in recent speech production models. J. Phon. 5, 115–133.
Kording, K. P., Tenenbaum, J. B., and Shadmehr, R. (2007). The dynamics of memory as a consequence of optimal adaptation to a changing body. Nat. Neurosci. 10, 779–786.
Lakatos, P., Pincze, Z., Fu, K. M., Javitt, D. C., Karmos, G., and Schroeder, C. E. (2005). Timing of pure tone and noise-evoked responses in macaque auditory cortex. Neuroreport 16, 933–937.
Lane, H., and Tranel, B. (1971). The Lombard sign and the role of hearing in speech. J. Speech Hear. Res. 14, 677–709.
Lane, H., Wozniak, J., Matthies, M., Svirsky, M., Perkell, J., O’Connell, M., and Manzella, J. (1997). Changes in sound pressure and fundamental frequency contours following changes in hearing status. J. Acoust. Soc. Am. 101, 2244–2252.
Larson, C. R., Burnett, T. A., Kiran, S., and Hain, T. C. (2000). Effects of pitch-shift velocity on voice Fo responses. J. Acoust. Soc. Am. 107, 559–564.
Levitt, H., Stromberg, H., Smith, C., and Gold, T. (1980). The structure of segmental errors in the speech of deaf children. J. Commun. Disord. 13, 419–441.
Lindblom, B. (1990). “Explaining phonetic variation: a sketch of the H&H theory,” in Speech Production and Speech Modelling, Vol. 55, eds W. J. Hardcastle, and A. Marchal (Dordrecht: Kluwer Academic Publishers), 403–439.
Liu, H., Zhang, Q., Xu, Y., and Larson, C. R. (2007). Compensatory responses to loudness-shifted voice feedback during production of Mandarin speech. J. Acoust. Soc. Am. 122, 2405–2412.
Lombard, E. (1911). Le signe de l’elevation de la voix. Ann. maladies oreille larynx nez pharynx 37, 101–119.
Ludlow, C. L. (2004). Recent advances in laryngeal sensorimotor control for voice, speech and swallowing. Curr. Opin. Otolaryngol. Head Neck Surg. 12, 160–165.
MacDonald, E. N., Goldberg, R., and Munhall, K. G. (2010). Compensations in response to real-time formant perturbations of different magnitudes. J. Acoust. Soc. Am. 127, 1059–1068.
MacNeilage, P. F. (1998). The frame/content theory of evolution of speech production. Behav. Brain Sci. 21, 499–511; discussion 511–446.
MacNeilage, P. F., and Davis, B. L. (2001). Motor mechanisms in speech ontogeny: phylogenetic, neurobiological and linguistic implications. Curr. Opin. Neurobiol. 11, 696–700.
Maraist, J. A., and Hutton, C. (1957). Effects of auditory masking upon the speech of stutterers. J. Speech Hear. Disord. 22, 385–389.
Mehta, B., and Schaal, S. (2002). Forward models in visuomotor control. J. Neurophysiol. 88, 942–953.
Miall, R. C., Weir, D. J., Wolpert, D. M., and Stein, J. F. (1993). Is the cerebellum a smith predictor? J. Motor Behav. 25, 203–216.
Miceli, G., Gainotti, G., Caltagirone, C., and Masullo, C. (1980). Some aspects of phonological impairment in aphasia. Brain Lang. 11, 159–169.
Mishkin, M., Ungerleider, L. G., and Macko, K. A. (1983). Object vision and spatial vision: two cortical pathways. Trends Neurosci. 6, 414–417.
Nasir, S. M., and Ostry, D. J. (2006). Somatosensory precision in speech production. Curr. Biol. 16, 1918–1923.
Nasir, S. M., and Ostry, D. J. (2008). Speech motor learning in profoundly deaf adults. Nat. Neurosci. 11, 1217–1222.
Nasir, S. M., and Ostry, D. J. (2009). Auditory plasticity and speech motor learning. Proc. Natl. Acad. Sci. U.S.A. 106, 20470–20475.
Natke, U., Grosser, J., and Kalveram, K. T. (2001). Fluency, fundamental frequency, and speech rate under frequency-shifted auditory feedback in stuttering and nonstuttering persons. J. Fluency Disord. 26, 227–241.
Natke, U., and Kalveram, K. T. (2001). Effects of frequency-shifted auditory feedback on fundamental frequency of long stressed and unstressed syllables. J. Speech Lang. Hear. Res. 44, 577–584.
Numminen, J., and Curio, G. (1999). Differential effects of overt, covert and replayed speech on vowel- evoked responses of the human auditory cortex. Neurosci. Lett. 272, 29–32.
Numminen, J., Salmelin, R., and Hari, R. (1999). Subject’s own speech reduces reactivity of the human auditory cortex. Neurosci. Lett. 265, 119–122.
Oller, D. K., and Eilers, R. E. (1988). The role of audition in infant babbling. Child Dev. 59, 441–449.
Osberger, M. J., and McGarr, N. S. (1982). “Speech production characteristics of the hearing-impaired,” in Speech and Language: Advances in Basic Research and Practice, ed. N. J. Lass (New York: Academic Press), 221–284.
Ostry, D. J., Flanagan, J. R., Feldman, A. G., and Munhall, K. G. (1991). “Human jaw motion control in mastication and speech,” in Tutorials in Motor Neuroscience. NATO ASI Series; Series D: Behavioral and Social Sciences, Vol. 62, eds J. Requin, and G. E. Stelmach (New York, NY: Kluwer Academic/Plenum Publishers), 535–543.
Ostry, D. J., Flanagan, J. R., Feldman, A. G., and Munhall, K. G. (1992). “Human jaw movement kinematics and control,” in Tutorials in Motor Behavior, 2. Advances in Psychology, Vol. 87, eds G. E. Stelmach, and J. Requin (Oxford: North-Holland), 647–660.
Payan, Y., and Perrier, P. (1997). Synthesis of V-V sequences with a 2D biomechanical tongue model controlled by the equilibrium point hypothesis. Speech Commun. 22, 185–205.
Pearce, S. L., Miles, T. S., Thompson, P. D., and Nordstrom, M. A. (2003). Is the long-latency stretch reflex in human masseter transcortical? Exp. Brain Res. 150, 465–472.
Perrier, P., Ostry, D. J., and Laboissiere, R. (1996). The equilibrium point hypothesis and its application to speech motor control. J. Speech Hear. Res. 39, 365–378.
Pile, E. J. S., Dajani, H. R., Purcell, D. W., and Munhall, K. G. (2007). Talking under conditions of altered auditory feedback: does adaptation of one vowel generalize to other vowels? Paper presented at the International Congress of Phonetic Sciences, Saarland University, Saarbrücken.
Polit, A., and Bizzi, E. (1979). Characteristics of motor programs underlying arm movements in monkeys. J. Neurophysiol. 42(1 Pt 1), 183–194.
Purcell, D. W., and Munhall, K. G. (2006). Adaptive control of vowel formant frequency: evidence from real-time formant manipulation. J. Acoust. Soc. Am. 120, 966–977.
Rauschecker, J. P., and Scott, S. K. (2009). Maps and streams in the auditory cortex: nonhuman primates illuminate human speech processing. Nat. Neurosci. 12, 718–724.
Rauschecker, J. P., and Tian, B. (2000). Mechanisms and streams for processing of “what” and “where” in auditory cortex. Proc. Natl. Acad. Sci. U.S.A. 97, 11800–11806.
Riecker, A., Brendel, B., Ziegler, W., Erb, M., and Ackermann, H. (2008). The influence of syllable onset complexity and syllable frequency on speech motor control. Brain Lang. 107, 102–113.
Rizzolatti, G., Fogassi, L., and Gallese, V. (1997). Parietal cortex: from sight to action. Curr. Opin. Neurobiol. 7, 562–567.
Ross, M., and Giolas, T. G. (1978). Auditory Management of Hearing-Impaired Children: Principles and Prerequisites for Intervention. Baltimore: University Park Press.
Roweis, S., and Ghahramani, Z. (1999). A unifying review of linear gaussian models. Neural. Comput. 11, 305–345.
Saltzman, E. L., Lofqvist, A., Kay, B., Kinsella-Shaw, J., and Rubin, P. (1998). Dynamics of intergestural timing: a perturbation study of lip-larynx coordination. Exp. Brain Res. 123, 412–424.
Saltzman, E. L., and Munhall, K. G. (1989). A dynamical approach to gestural patterning in speech production. Ecol. Psychol. 1, 333–382.
Sanguineti, V., Laboissiere, R., and Ostry, D. J. (1998). A dynamic biomechanical model for neural control of speech production. J. Acoust. Soc. Am. 103, 1615–1627.
Sanguineti, V., Laboissiere, R., and Payan, Y. (1997). A control model of human tongue movements in speech. Biol. Cybern. 77, 11–22.
Schmahmann, J. D., Pandya, D. N., Wang, R., Dai, G., D’rceuil, H. E., de Crespigny, A. J., and Wedeen, V. J. (2007). Association fibre pathways of the brain: parallel observations from diffusion spectrum imaging and autoradiography. Brain 130(Pt 3), 630–653.
Scott, C. M., and Ringel, R. L. (1971). Articulation without oral sensory control. J. Speech Hear. Res. 14, 804–818.
Shadmehr, R., and Krakauer, J. W. (2008). A computational neuroanatomy for motor control. Exp. Brain Res. 185, 359–381.
Shadmehr, R., and Wise, S. P. (2005). The Computational Neurobiology of Reaching and Pointing: A Foundation for Motor Learning. Cambridge, MA: MIT Press.
Shaiman, S., and Gracco, V. L. (2002). Task-specific sensorimotor interactions in speech production. Exp. Brain Res. 146, 411–418.
Shiller, D. M., Sato, M., Gracco, V. L., and Baum, S. R. (2007). Motor and sensory adaptation following auditory perturbation of/s/production. Paper presented at the 154th Meeting of the Acoustical Society of America, New Orleans, LA.
Shiller, D. M., Sato, M., Gracco, V. L., and Baum, S. R. (2009). Perceptual recalibration of speech sounds following speech motor learning. J. Acoust. Soc. Am. 125, 1103–1113.
Skipper, J. I., Nusbaum, H. C., and Small, S. L. (2005). Listening to talking faces: motor cortical activation during speech perception. Neuroimage 25, 76–89.
Smith, C. R. (1975). Residual hearing and speech production in deaf children. J. Speech Hear. Res. 18, 795–811.
Soderberg, G. A. (1968). Delayed auditory feedback and stuttering. J. Speech Hear. Disord. 33, 260–267.
Tin, C., and Poon, C.-S. (2005). Internal models in sensorimotor integration: perspectives from adaptive control theory. J. Neural Eng. 2, S147–S.
Todorov, E. (2006). “Optimal control theory,” in Bayesian Brain: Probabilistic Approaches to Neural Coding, eds K. Doya, S. Ishii, A. Pouget, and R. P. N. Rao (Cambridge, MA: MIT Press), 269–298.
Todorov, E., and Jordan, M. I. (2002). Optimal feedback control as a theory of motor coordination. Nat. Neurosci. 5, 1226–1235.
Tourville, J. A., Reilly, K. J., and Guenther, F. H. (2008). Neural mechanisms underlying auditory feedback control of speech. Neuroimage 39, 1429–1443.
Toyomura, A., Koyama, S., Miyamaoto, T., Terao, A., Omori, T., Murohashi, H., and Kuriki, S. (2007). Neural correlates of auditory feedback control in human. Neuroscience 146, 499–503.
Tremblay, S., Houle, G., and Ostry, D. J. (2008). Specificity of speech motor learning. J. Neurosci. 28, 2426–2434.
Tremblay, S., Shiller, D. M., and Ostry, D. J. (2003). Somatosensory basis of speech production. Nature 423, 866–869.
Upadhyay, J., Hallock, K., Ducros, M., Kim, D.-S., and Ronen, I. (2008). Diffusion tensor spectroscopy and imaging of the arcuate fasciculus. Neuroimage 39, 1–9.
Ventura, M. I., Nagarajan, S. S., and Houde, J. F. (2009). Speech target modulates speaking induced suppression in auditory cortex. BMC Neurosci. 10, 58. doi: 10.1186/1471-2202-10-58
Villacorta, V. M., Perkell, J. S., and Guenther, F. H. (2007). Sensorimotor adaptation to feedback perturbations of vowel acoustics and its relation to perception. J. Acoust. Soc. Am. 122, 2306–2319.
Wachholder, K., and Altenburger, H. (1926). Beiträge zur physiologie der willkürlichen bewegung X. Mitteilung. Einzelbewegungen. Pflugers Arch. Gesamte Physiol. Menschen Tiere 214, 642–661.
Watkins, K., and Paus, T. (2004). Modulation of motor excitability during speech perception: the role of Broca’s area. J. Cogn. Neurosci. 16, 978–987.
Whiting, H. T. A. (ed.). (1984). Human Motor Actions: Bernstein Reassessed. Amsterdam, NL: North-Holland.
Wilson, S. M., and Iacoboni, M. (2006). Neural responses to non-native phonemes varying in producibility: evidence for the sensorimotor nature of speech perception. Neuroimage 33, 316–325.
Wilson, S. M., Saygin, A. P., Sereno, M. I., and Iacoboni, M. (2004). Listening to speech activates motor areas involved in speech production. Nat. Neurosci. 7, 701–702.
Wolpert, D. M. (1997). Computational approaches to motor control. Trends Cogn. Sci. (Regul. Ed.) 1, 209.
Wolpert, D. M., and Ghahramani, Z. (2000). Computational principles of movement neuroscience. Nat. Neurosci. 3(Suppl.), 1212–1217.
Wolpert, D. M., Ghahramani, Z., and Jordan, M. I. (1995). An internal model for sensorimotor integration. Science 269, 1880–1882.
Yeterian, E. H., and Pandya, D. N. (1998). Corticostriatal connections of the superior temporal region in rhesus monkeys. J. Comp. Neurol. 399, 384–402.
Zajac, F. E. (1989). Muscle and tendon: properties, models, scaling, and application to biomechanics and motor control. Crit. Rev. Biomed. Eng. 17, 359–411.
Keywords: speech neurophysiology, speech motor control, models of speech production, models of neural processes, sensory feedback
Citation: Houde JF and Nagarajan SS (2011) Speech production as state feedback control. Front. Hum. Neurosci. 5:82. doi: 10.3389/fnhum.2011.00082
Received: 09 May 2011; Paper pending published: 08 June 2011;
Accepted: 27 July 2011; Published online: 25 October 2011.
Edited by:
Kenneth Hugdahl, University of Bergen, NorwayReviewed by:
Frederic Dick, University of California San Diego, USAKarsten Specht, University of Bergen, Norway
Joanne Arciuli, University of Sydney, Australia
Copyright: © 2011 Houde and Nagarajan. This is an open-access article subject to a non-exclusive license between the authors and Frontiers Media SA, which permits use, distribution and reproduction in other forums, provided the original authors and source are credited and other Frontiers conditions are complied with.
*Correspondence: John F. Houde, Department of Otolaryngology – Head and Neck Surgery, University of California San Francisco, 513 Parnassus Avenue, HSE800, San Francisco, CA 94143, USA. e-mail:aG91ZGVAcGh5LnVjc2YuZWR1