- Experimental Neuropsychology Unit, Saarland University, Saarbrücken, Germany
In the present experiment, we used event-related potentials (ERP) to investigate the role of immediate and delayed feedback in an artificial grammar learning (AGL) task. Two groups of participants were engaged in classifying non-word strings according to an underlying rule system, not known to the participants. Visual feedback was provided after each classification either immediately or with a short delay of 1 s. Both groups were able to learn the artificial grammar system as indicated by an increase in classification performance. However, the gain in performance was significantly larger for the group receiving immediate feedback as compared to the group receiving delayed feedback. Learning was accompanied by an increase in P300 activity in the ERP for delayed as compared to immediate feedback. Irrespective of feedback delay, both groups exhibited learning related decreases in the feedback-related positivity (FRP) elicited by positive feedback only. The feedback-related negativity (FRN), however, remained constant over the course of learning. These results suggest, first, that delayed feedback is less effective for AGL as task requirements are very demanding, and second, that the FRP elicited by positive prediction errors decreases with learning while the FRN to negative prediction errors is elicited in an all-or-none fashion by negative feedback throughout the entire experiment.
1. Introduction
As our society becomes increasingly multilingual and learning a second language (L2) becomes more and more important, the neural processes by which humans learn a language have gained considerable interest over the past years (Hauser et al., 2002). Often, the achievements of late L2-learners seem very poor, at least in the core computational component of language, that is, grammar. Such difficulties have been linked to the age of acquisition, declining brain plasticity with age (Lenneberg, 1967; Johnson and Newport, 1988), or general cognitive and linguistic factors (Elman et al., 1996; Clahsen and Felser, 2006). How these different factors affecting proficiency in L2 may influence the neural correlates of L2 learning is still widely unexplored. In order to test and further specify models of L2 processing at the neurophysiological level, artificial grammar learning (AGL) has been recently used to study language acquisition in adults (e.g., Hoen and Dominey, 2000; Friederici et al., 2002; Opitz and Friederici, 2004; Petersson et al., 2004). As event-related potential (ERP) patterns observable in AGL studies were highly similar to those observed for syntactic processing in natural language (Friederici et al., 2006; Mueller et al., 2009) and both share some neural substrates as revealed by neuroimaging studies (Opitz and Friederici, 2003, 2004; Forkstam et al., 2006), AGL seems to be a valid tool for investigating natural language acquisition.
Artificial grammar learning typically involves the learning of symbol strings specified by an artificial grammar, which is a set of rules used to generate a set of structured sequences (Reber, 1967; Pothos and Bailey, 2000). Early studies demonstrated that subjects exposed to such grammar systems learn to categorize strings as grammatical (i.e., conforming to the underlying rules) or non-grammatical and after some time of exposure perform this categorization task with an accuracy greater than chance (Reber, 1967). The explanation of these learning effects in this and later work (Reber, 1989) was that participants acquired an abstract representation of the underlying rules (see also Opitz and Friederici, 2004, for a similar argument). A recently proposed theoretical account of category learning (Ashby and O’Brien, 2005) suggests that such rule sets are developed via a feedback-dependent, trial-and-error learning process. Indeed, increasing experimental evidence suggests that the development of an implicit rule set depends on reinforcement learning (RL): If people are not provided with explicit feedback, they do not learn the rules (e.g., Ashby et al., 1999). Furthermore, a recent study on L2 learning (Mueller et al., 2009) is also consistent with this proposal. In this study, the ERPs to auditorily presented correct and incorrect Italian sentences were compared in native and non-native speakers after brief exposure to correct Italian sentences of a similar structure without any feedback. Native speakers in this experiment exhibited an N400 followed by a P600 component in the ERP to incorrect sentences, indicating grammatical rule use (Hahne and Friederici, 1999; Kaan and Swaab, 2003; Hagoort, 2008). In contrast, non-native speakers did only show an N400. As no P600 to grammatical violations was observed, it can be inferred that non-native speakers did not acquire an abstract representation of the underlying syntactic rules after mere exposure to simple Italian sentences. From this it can be assumed that feedback is necessary for the acquisition of a grammatical rule set.
Interestingly, some of the brain structures implicated by Ashby’s theory 2005 in feedback-based rule learning, e.g., the basal ganglia (BG) and the anterior cingulate cortex (ACC), are also thought to play an important role in RL (Montague et al., 1996; Holroyd et al., 2004; Kok et al., 2006; Ullsperger and von Cramon, 2006, for review, see Schultz, 2002). According to this previous research the BG evaluate ongoing events and predict the hedonistic value of future events, i.e., whether the event will be better or worse that expected. When the BG revise their predictions for the better or for the worse, they induce a phasic increase or decrease in the activity of midbrain dopamine neurons, respectively. These phasic changes in dopamine activity indicate whether the outcome of an action deviates from a prediction and are used by the motor-related areas in the ACC to evaluate whether behavioral adjustments are necessary in order to improve performance on the task at hand according to principles of RL (Holroyd and Coles, 2002).
One component in the ERP that has been associated with RL is the feedback-related negativity (FRN, Miltner et al., 1997; Nieuwenhuis et al., 2004). It can be observed between 200 and 300 ms after subjects received negative feedback regarding the accuracy of their performance. It has a fronto-central scalp distribution and source localization studies revealed generators in the ACC (Miltner et al., 1997). More recently, Holroyd and Coles (2002) argued that the FRN reflects the activity of a RL-system that continually evaluates ongoing events against expected outcomes. By this, the amplitude of the FRN is assumed to be modulated by the dopaminergic input from the BG to the ACC, with phasic decreases in dopamine activity (indicating that ongoing events are worse than expected) being associated with large FRNs (Holroyd and Coles, 2002). To test this model participants were asked to learn stimulus–response mappings by trial-and-error based on feedback information in a probabilistic learning task. The results of this study showed an FRN decrease with learning due to the decreasing information value of the feedback stimulus.
So far the RL-model of the FRN was examined in gambling tasks where the feedback indicated a loss (Holroyd et al., 2003; Yeung et al., 2005) or in tasks in which the feedback indicated an incorrect response (Miltner et al., 1997) but was not yet applied to AGL tasks. It seems reasonable to suggest that the RL-system also plays a key role in AGL, by facilitating feedback-dependent learning of a rule set that underlies an artificial grammar. Thus, in the present experiment, we used ERPs to investigate the role of the medial–frontal RL-system in the AGL task. Our approach relied on examining learning related changes in the FRN. More precisely, if feedback is necessary for successful AGL we expect the FRN to decrease as learning proceeds.
A second important issue concerning the understanding of the mechanisms underlying AGL is how the timing of feedback enables learners to extract relevant information from the feedback. One idea originating from behaviorist theories of RL, is that feedback must be given immediately in order to reinforce correct responses (e.g., Skinner, 1954). This position holds that with feedback delay the predictions of the potential outcome are less specific. This should result in a smaller prediction error and, consequently, in a reduced amplitude of the FRN. Indirect support for this view is provided by a recent study employing a motor learning task requiring subjects to move a cursor across a computer screen using a mouse (Lieberman et al., 2008). There were hidden target locations on the screen and points were awarded in proportion to how close participants came to the center of these targets. Crucially, the performance of one group of participants that received immediate feedback was superior to the performance of a group that was given feedback after a short delay of 6 s. This result emphasizes that feedback delay plays a critical role when participants have little a priori information about what response, i.e., cursor position is correct. It has been argued, that the diminished learning under delayed feedback conditions is caused by the interference of alternative response options held in working memory.
This situation bears some similarity with AGL. Like in the case of hidden target locations learners of an artificial grammar do not know which particular rule of the entire rule set applies to a grammatical string or renders this string ungrammatical. For instance the string ADC can be ungrammatical at the second position following the rule “alphabetical order” or at the first position according to the rule “reversed alphabetical order.” The learner would receive the same negative feedback in both cases, and thus, simply does not know which rule was violated. This uncertainty imposed by the alternative rules would increase if more time is available to consider alternative rules in working memory as in a delayed feedback condition. As the feedback will equally apply to all of the alternatives held in working memory, the information value of the feedback for each of these alternatives will decrease. Consequently, the choice of the correct rule will be hindered and learning the artificial grammar will be impaired. As can be inferred from previous research (Lieberman et al., 2008) the effect of a feedback delay depends on how many alternative rules are held in working memory. When feedback is provided immediately, alternative rules are largely ignored while delayed feedback will increase the likelihood of considering alternative rules. As a consequence, delaying the feedback should lead to abated AGL. Due to the decreased information value of the delayed feedback stimulus a reduced FRN is expected.
2. Materials and Methods
2.1. Participants
The participants were 48 students (24 in each experiment) from Saarland University, Saarbrücken. They all signed informed consent before the experiment and were paid 8 Euros per hour or received course credits. They all were monolingual native speakers of German. Three subjects (two in experiment 1 and one in experiment 2) were excluded from all analyses because of excessive eye blink artifacts. The remaining 45 participants (20 male) were 20–34 years old (experiment 1: M = 22.3 years, experiment 2: M = 23.5 years). They all had normal or corrected-to-normal vision and were without history of neurological or psychiatric disorder.
2.2. Stimuli
The stimulus sentences of both experiments were based on a subject–verb–[object] structure (Figure 1), according to the artificial language BROCANTO (Friederici et al., 2002; Opitz and Friederici, 2003). BROCANTO is based on the universal principles of natural languages, i.e., it consists of different syntactic word categories and defined phrase structure rules. The subject and the object of a sentence were a noun phrase (NP) composed of a determiner (D, d), an adjective (M), and a noun (N). The verb phrase (VP) consisted of a verb (v) and an optional adverb (m). A total of 200 sentences were formulated according to these rules. Another 200 sentences contained a severe syntactic violation: either an agreement violation, a word category repetition, or a phrase structure violation (Opitz and Friederici, 2003).
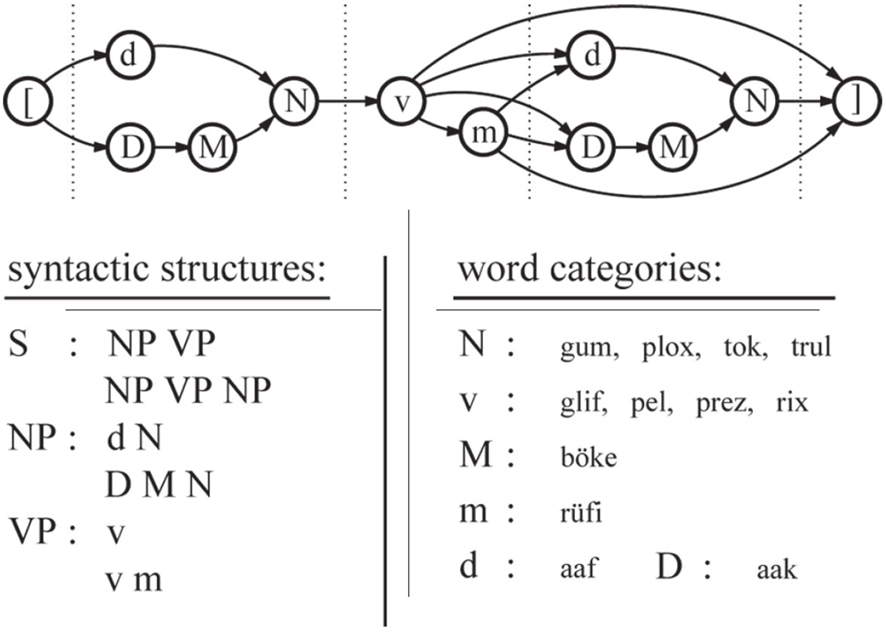
Figure 1. Schematic representation of the artificial grammar of BROCANTO. Nodes in the upper panel specify word classes (nouns, verbs, etc.), while arrows denote valid transitions between nodes. A correct sentence is formed by a transition from beginning ([) to end (]). The lower panel depicts the rules according to which valid phrases are formed. Thus, a sentence (S) consists of a noun phrase (NP) and a verb phrase (VP). An NP in turn is either the sequence dN or DMN, where N is one of the possible noun choices gum, plox, tok, and trul. Word classes: N = noun; v = verb; M = adjective; m = adverb; d, D = determiner. Examples of correct and incorrect sentences (words marked with an asterisk violate the grammatical rules):
correct aaf gum pel rüfi aak böke trul.
agreement violation aaf gum pel rüfi aaf *böke trul.
word category repetition aaf gum pel *prez aak böke trul.
phrase structure violation aaf gum *aak böke trul pel rüfi.
2.3. Procedure
Acquisition phase
The acquisition phase of the present experiment was similar to the ones used in previous studies examining artificial grammar systems (Kinder and Assmann, 2000; Opitz and Friederici, 2007). It comprised 15 learning-test cycles with a fixed order of a learning block (140 s) and a test block (140 s). A brief instruction started each cycle. During learning, participants viewed 20 correct sentences for 7 s each on a computer monitor and were instructed to learn the underlying grammatical rules. During test blocks, participants were presented with 20 entirely new sentences (7 s each) that were either grammatical (half of the sentences) or non-grammatical. The participants task was to give a grammaticality judgment on a 6-point confidence scale (ranging from 1, surely non-grammatical, to 6, surely grammatical) for each presented sentence. For the purpose of the present analysis three confidence responses were collapsed to represent non-grammatical responses (i.e., a 1, 2, or 3-confidence rating) or grammatical responses (i.e., a 4, 5, or 6-confidence rating), respectively. Visual feedback in terms of the written words “richtig” (“correct,” written in green) or “falsch” (“incorrect,” written in red) was given for 500 ms after each response either immediately (experiment 1) or with a delay (experiment 2). As previous results indicated that rather long delay periods (larger than 2500 ms) did not further increase the effect of feedback delay (Maddox et al., 2003), a delay interval of 1000 ms was chosen.
Transfer test
After the acquisition phase, a transfer test was performed in which 200 new sentences were presented, half followed the same grammatical rules as during the initial acquisition (correct sentences) and half were new non-grammatical sentences (incorrect sentences). The task for the participants was the same as during the acquisition phase. However, no feedback was provided in order to prevent further learning.
2.4. EEG Recordings and Data Analysis
Subjects were comfortably seated in a dimly lit, electrically shielded, and sound-attenuated chamber. Electroencephalograms (EEG) were continuously recorded from 59 Ag/AgCl scalp electrodes embedded in an elastic cap (EASYCAP GmbH) according to the extended 10/20 system (Sharbrough et al., 1991). The EEG from all sites was recorded with reference to the left mastoid electrode. An additional channel recorded EEG from the right mastoid and was used for off-line re-referencing the scalp recordings to linked mastoids. Vertical and horizontal electrooculograms were recorded with additional electrodes located above and below the right eye and outside the outer canthi of both eyes. Inter electrode impedances were kept below 5 kΩ. All channels were amplified with a band-pass from DC to 70 Hz and A/D converted with 16 bit resolution at a rate of 500 Hz. Off-line data processing included a digital high-pass filter set to 0.1 Hz (−3 dB cutoff) to eliminate low frequency signal drifts. An automatic rejection criterion (voltage variation of more than 30 μV within a 200-ms sliding time interval) was applied to the EOG channels to mark segments contaminated by eye movement artifacts. These recording epochs were corrected using a linear regression approach (Gratton et al., 1983). Furthermore, all channels were scanned manually for additional disturbances. This resulted in 28, 25, and 22 artifact-free epochs for negative feedback and 58, 60, 61 epochs for positive feedback for each of the three learning phases, respectively.
These artifact-free epochs ranging from −100 to 600 ms with respect to the onset of the feedback were averaged separately for each participant and feedback type (i.e., positive and negative feedback), with the 100-ms prior to feedback onset serving as the baseline. To examine the learning related development of ERP components, we divided the test blocks of both experiments into three phases of five test blocks (i.e., 100 trials) each. Following previous studies (Yeung et al., 2005) ERPs were quantified as the mean amplitude relative to baseline at midline electrodes (Fz, FCz, Cz, CPz, Pz) in an early time interval from 240 to 340 ms post-stimulus onset. Previous studies demonstrated that the early feedback-related ERP effects are typically superimposed on a subsequent P300 component (e.g., Yeung et al., 2005; Holroyd et al., 2008). Thus, the P300 was also analyzed at the same electrodes in a late time interval from 340 to 440 ms. The feedback-locked ERP components were analyzed with a repeated-measures ANOVA (alpha level = 0.05) with the between-subject factor delay (immediate vs delayed) and the within-subject factors feedback type (positive vs negative feedback), time interval (early vs late), learning phase (first, second, and last phase), and electrode (five levels). In case of significant interactions involving the factor feedback type, separate analyses for positive and negative feedback were conducted. The Greenhouse–Geisser adjustment for non-sphericity was used whenever appropriate and the corrected p values are reported together with the uncorrected degrees of freedom.
3. Results
All participants were able to acquire the artificial grammar of BROCANTO as reflected in a reliable increase in the proportion of correct responses from the first to the last phase in both experiments (see Table 1). This was confirmed by a main effect of learning phase (F2,86 = 55.02, p < 0.001), that was qualified by a significant linear trend (F1,43 = 78.75, p < 0.001). As expected, the group receiving immediate feedback performed better than the group receiving delayed feedback (main effect delay, F1,43 = 7.62, p < 0.01) throughout the entire experiment as indicated by a non-significant learning phase × delay interaction (F2,86 = 0.44, p > 0.6). The results of the transfer test confirmed these findings in that classification performance after immediate feedback was superior to the performance after delayed feedback (F1,43 = 20.31, p < 0.001).
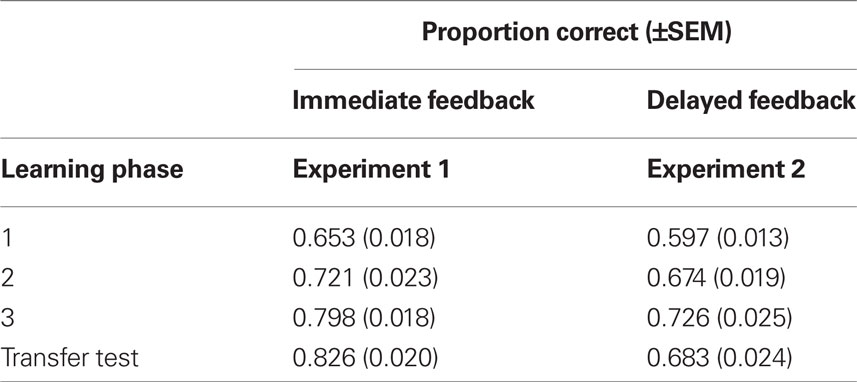
Table 1. Proportion correct responses for the three learning phases and the transfer test in both experiments.
Grand average ERP waveforms elicited by positive and negative feedback in the two delay conditions at midline electrode sites are depicted in Figure 2. At first glance there is a striking similarity between the ERP components elicited by feedback stimuli in both feedback delays. As apparent from the figure, negative feedback elicited a negative deflection irrespective of delay beginning approximately 200 ms after feedback onset with a maximum at central electrodes which was not evident for positive feedback. Importantly, the scalp distribution and the temporal characteristics of this early negative deflection correspond well with the FRN. The ERP deflection elicited by positive feedback seems to differ from the FRN in its morphology and its sensitivity to experimental manipulations (see Figure 3). To indicate the more positive-going waveform this deflection elicited by positive feedback is labeled feedback-related positivity (FRP) throughout the manuscript.
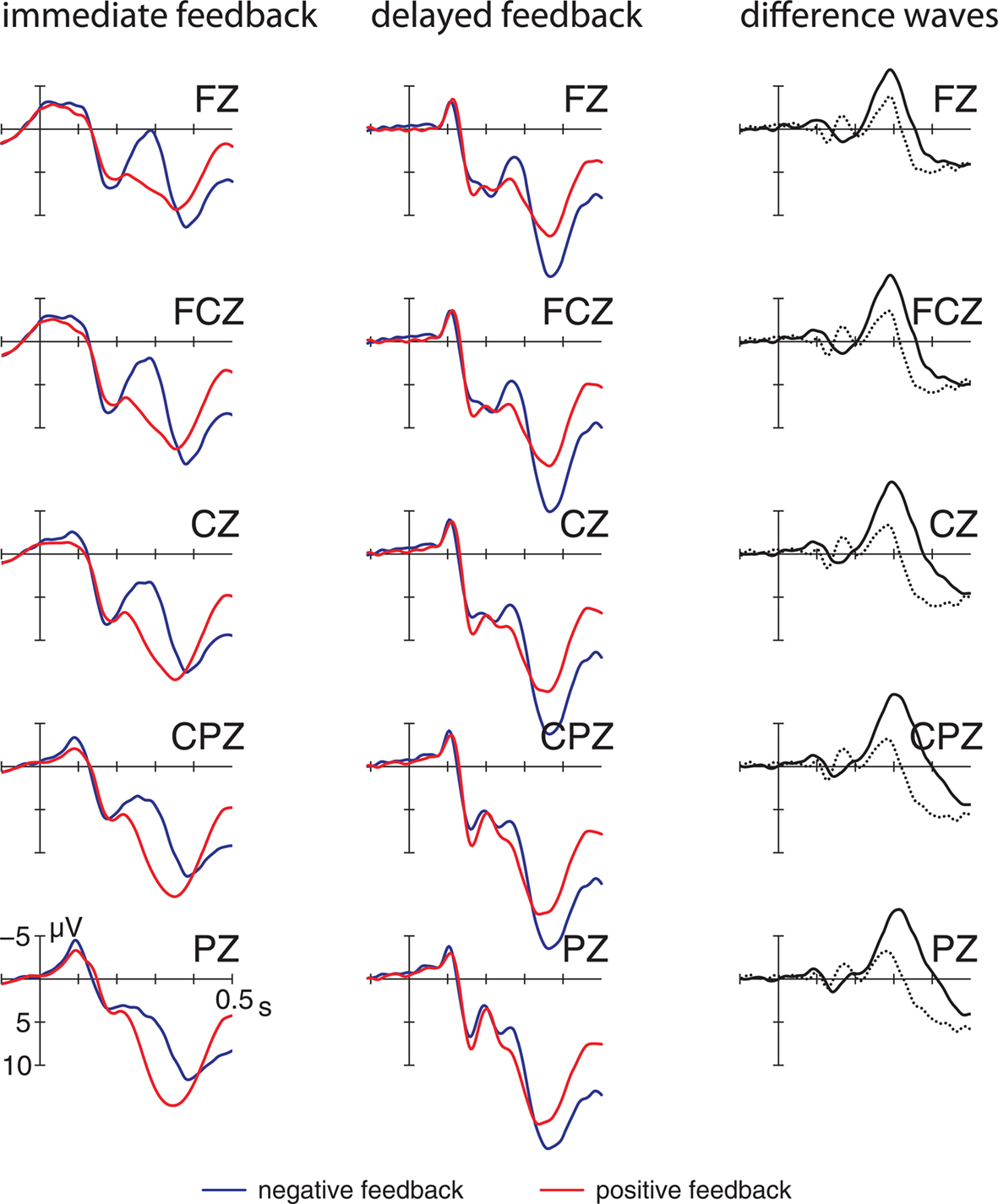
Figure 2. Event-related potentials at midline electrodes elicited by positive and negative feedback when feedback was provided immediately (left column) or with a delay (middle column). The right column displays the difference waveforms (negative–positive) for both feedback conditions. solid line – immediate feedback, dotted line – delayed feedback.
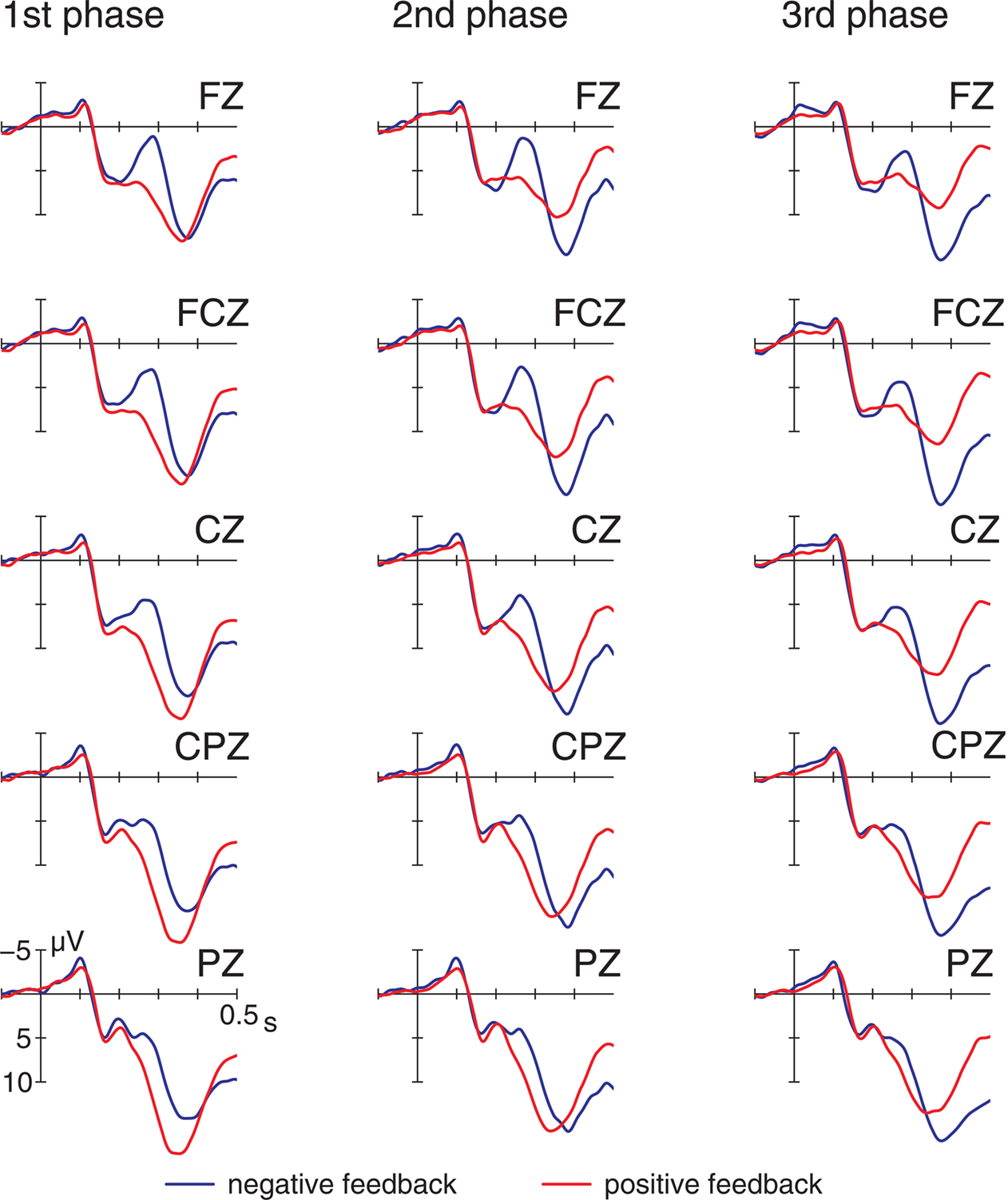
Figure 3. Event-related potentials at midline electrodes elicited by positive and negative feedback for all three learning phases.
These early components were followed by a long lasting positivity (P300), that was elicited by both positive and negative feedback. The P300 was maximal between 340 and 440 ms and exhibited a central scalp distribution. Learning related effects, i.e., changes across learning phases seem to be most evident in an increase of the ERP for positive feedback. In contrast, the FRN elicited by negative feedback seems to remain stable over the course of learning (see Figure 3).
Effects of Immediate and Delayed Feedback
A first analysis focused on identifying the effects of immediate vs delayed feedback on the ERPs during the acquisition of an artificial grammar. Thus, in an overall ANOVA with the between-subject factor delay (immediate vs delayed) and repeated-measured factors feedback type (positive vs negative feedback), learning phase (first, second, and last five test blocks), time interval (early vs late), and electrode (Fz, FCz, Cz, CPz, Pz) we were especially interested in interactions involving the delay factor. This analysis revealed a main effect of feedback type (F1,43 = 10.53, p < 0.005), a feedback type by delay interaction (F1,43 = 18.13, p < 0.001), and a feedback type by time interval interaction (F1,43 = 45.50, p < 0.001). The triple interaction feedback type by time interval by electrode (F4,172 = 45.51, p < 0.001) and the feedback type by time interval by electrode by delay interaction (F4,172 = 15.92, p < 0.001) both reached significance suggesting that the early FRN/FRP and the late P300 were differentially affected by the delay manipulation. Subsequent analyses for each time interval separately revealed a significant main effect of feedback type in the early time interval only (F1,43 = 37.84, p < 0.001, ) and a feedback type by delay interaction in both time intervals (early: F1,43 = 14.25, p < 0.001,
; late: F1,43 = 16.85, p < 0.001,
).
To further investigate the feedback type by delay interaction the ERPs were examined in a repeated-measure ANOVA separately for both feedback types. This analysis suggests that the delay manipulation affected only the ERPs elicited by negative feedback. A reduced FRN (F1,43 = 10.08, p < 0.005) and an enhanced P300 (F1,43 = 13.23, p < 0.001) elicited by negative feedback were observed when feedback was delayed as compared to when it was provided immediately. Measures of effect size indicated that there was a larger effect of delayed vs immediate feedback on the P300 enhancement () as compared to FRN attenuation (
). In contrast, for the FRP and the P300 elicited by positive feedback no effects involving the delay factor were obtained (all p > 0.3). Taken together, these findings indicate that only the ERP responses to negative feedback (FRN and P300) were modulated by the delay factor.
Learning Related Effects
A second set of analyses aimed at disentangling learning related effects on feedback processing, i.e., on interactions involving the factor learning phase. As none of the significant interactions of the first analysis involved the two factors learning phase and delay at the same time, the delay manipulation had no differential effects on learning related changes in the ERP data. As a consequence, for all analyses focusing on learning related changes ERP data were collapsed across both delay conditions (see Figure 3). The overall ANOVA including both feedback types revealed a feedback type by learning phase interaction (F2,88 = 20.57, p < 0.001), and a significant triple interaction, feedback type by time interval by learning phase (F2,88 = 12.41, p < 0.001). To further explore this interaction ANOVAs were performed for each time interval separately. This analysis exhibited a significant feedback type by phase interaction in both time intervals (early: F2,88 = 12.12, p < 0.001, ; late: F2,88 = 25.96, p < 0.001,
).
In order to investigate the relative contribution of positive and negative feedback the ERPs were again examined separately for the two feedback types. This analysis revealed that learning related changes in both time intervals were limited to positive feedback, showing less positive-going waveforms when learning proceeds (main effect learning phase, FRP: F2,88 = 12.95, p < 0.001, P300: F2,88 = 24.96, p < 0.001,
). There was a significant linear trend in both time intervals (early: F2,88 = 16.83, p < 0.001,
; late: F2,88 = 32.24, p < 0.001,
), indicating a reduction of the FRP and the P300 with learning. In contrast, for the ERPs elicited by negative feedback no learning related changes were observed (all p > 0.1). In sum, this analysis indicated that the effects of feedback processing on learning were associated with ERP changes following positive feedback rather than ERP modulations on negative feedback.
To provide further support for this suggestion, a complementary analysis examined whether the potential ERP indices of feedback processing were predictive of individual differences in learning. For this analysis, learning was operationally defined as the performance increase from the first to the last learning phase as a dependent variable. The difference wave (i.e., the difference in the ERPs between positive and negative feedback) in the initial learning phase as well as learning related changes of the FRN, the FRP, and the P300 elicited by positive and negative feedback (quantified as the difference of the waveforms elicited in the last minus the first learning phase for positive and negative feedback, separately) served as regressors in this analysis. These regressors were subjected to a stepwise regression analysis, using as stepping criteria p < 0.05 for inclusion and p > 0.10 for exclusion. In the resulting model only the predictors “learning related changes in the FRP” and “difference wave in the initial learning phase” were associated with a significant regression weight (F1,43 = 8.32, p < 0.01, R2 = 0.422; β = −0.403) and (F1,42 = 4.34, p < 0.05, R2 = 0.08; β = −0.281), respectively. Accordingly, the larger the decrease of the FRP to positive feedback, the higher the level of observed increase in performance from the first to the last learning phase. Furthermore, these results suggest, that the amplitude difference between positive and negative feedback in the initial learning phase made an independent contribution on the increase of classification performance. This is consistent with the idea that a larger FRN in the initial learning phase predicts larger performance increases during learning.
As the FRP and the P300 elicited by positive feedback may temporally overlap learning related changes of the FRP may be confounded by corresponding changes in the P300. In order to examine whether the FRP and the P300 elicited by positive feedback are functionally distinct a detailed topographic analysis of the ERPs elicited by positive feedback was performed (see Figure 4). In contrast to all previous analyses additional lateral electrode sites (F3, FC3, C3, CP3, P3, PO3, F4, FC4, C4, CP4, P4, PO4) were included to provide a better estimate of the scalp distribution. The resulting 18 electrode sites were organized into a topographic factor representing the anterior/posterior dimension (six levels) and another factor accounting for laterality effects (three levels). The ANOVA with the repeated-measure factors learning phase (three levels), time interval (early vs late), and both topographic factors (anterior/posterior and laterality) revealed a significant learning phase by anterior/posterior interaction (F10,440 = 5.38, p < 0.005), and a time interval by learning phase by anterior/posterior interaction (F10,440 = 8.67, p < 0.001). This indicates that in each learning phase the FRP and the P300 showed a differential scalp distribution.
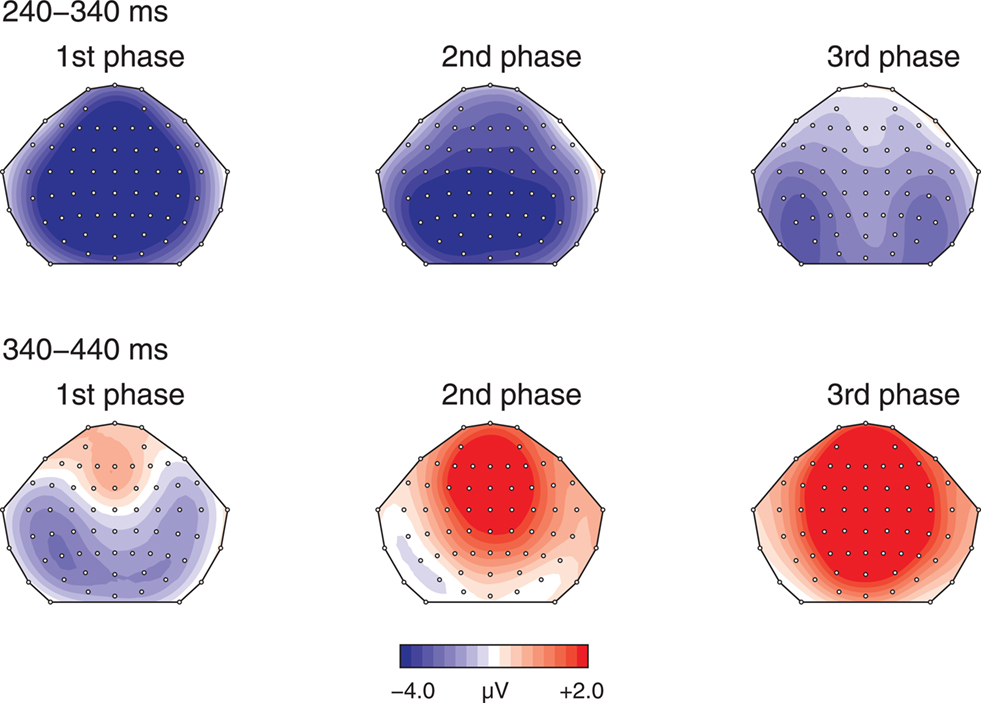
Figure 4. Topographic maps illustrating learning related changes in scalp distribution of the difference waves (negative–positive) in the FRN/FRP time range (240–340 ms) and the subsequent P300 time range (340–440 ms) across the three learning phases.
Taken together, our results indicate that delayed feedback led to a reduction of the FRN and an increase of the P300 for negative feedback but did not influence the ERPs elicited by positive feedback. In contrast, learning had an effect on the ERPs elicited by positive feedback. A decrease of the FRP and a reduction of the P300 elicited by positive feedback in the last as compared to the initial learning phase was observed. Crucially, based on different scalp topographies we conclude that functionally distinct processes underlie the FRP and P300 effects.
4. Discussion
In the present study we aimed at investigating the role of feedback processing during AGL. We focused on the questions whether the ERP-correlates of feedback processing reflect learning related changes and how they are modulated by feedback delay. The behavioral results suggest that delayed feedback had a detrimental effect on AGL, indicated by a substantially reduced proportion of correct answers as compared to immediate feedback. Interestingly, the present results suggest that the feedback delay manipulation mainly affects the processing of negative feedback, leading to an increase of the P300 amplitude and an attenuation of the FRN. In contrast, changes related to the successful acquisition of the artificial grammar were prominent in the ERPs elicited by positive feedback. Here a clear decrease of the FRP and a reduction of P300 to positive feedback was observed. Moreover, as indicated by a topographic analysis these effects were functionally independent from each other.
The present results on the influence of delayed feedback are largely consistent with recent studies demonstrating reduced learning when feedback was delayed by a few seconds (Maddox et al., 2003; Lieberman et al., 2008). Crucially, this delay manipulation was most effective when task demands (Lieberman et al., 2008) or stimulus complexity (Maddox et al., 2003) were very high. The latter study investigated categorization of Gabor patches that varied either in spatial frequency or in orientation (unidimensional rule) or in both features simultaneously (multidimensional rule). Learning unidimensional rules was successful irrespective of whether immediate or delayed feedback was provided. However, learning multidimensional rules was impaired when feedback was delayed by as little as 2.5 s. It has been argued, that the diminished learning under delayed feedback conditions is caused by interference in working memory imposed by the stimulus and/or task complexity (Lieberman et al., 2008).
In light of these findings one could propose that in the present study a relatively large number of applicable rules along with one’s own response had to be retained in working memory. As working memory representations of the alternative rules decrease with longer retention intervals, especially when the load is high (cf., Glidden and Scott, 1975; Chen et al., 2003), delaying the feedback in the present AGL task will lead to diminished memory for each of the alternative rules. This is reflected in the overall poorer performance in the delay condition. As a consequence assigning the feedback stimulus to the correct alternative is harder when feedback is delayed as when it is provided immediately. In other words, the feedback carries less information with respect to the correct rule when feedback is delayed, due to the diminished representation of the applicable rules. From this it follows, that the participant’s predictions about the potential outcome are less specific resulting in a smaller prediction error, indexed by a smaller FRN. As previous studies observed an FRN reduction caused by a decreasing feedback information value (cf., Holroyd and Coles, 2002), the reduction of the FRN elicited by negative feedback observed in the present experiment is supportive for the view that delayed feedback increases the interference of alternative rules held in working memory during the delay. However, the overall impaired performance in the delay condition did not interact with learning related changes in accuracy or ERPs. A possible explanation for this unexpected finding might be that the differences between both feedback conditions in working memory demands imposed by alternative rules remained constant over the course of learning. That is, although the working memory demands decrease with learning in both conditions, as the number of potentially applicable rules is reduced, they remain larger when feedback was delayed. This would be in line with the finding of an increased P300 amplitude elicited by delayed as compared to immediate negative feedback. An increase in P300 amplitude as a function of increasing stimulus and/or task complexity has been previously demonstrated (Johnson, 1986; Holroyd et al., 2008). In both studies P300 amplitude was measured from the same subjects during the performance of two different tasks. Crucially, the same stimuli served either as targets in an oddball paradigm (they had to be counted) or signified correct performance in a feedback task. Although task complexity was not directly assessed it is plausible to suggest that the amplitude of the P300 increased with increasing task demands, being largest for feedback stimuli. It has been argued that these increasing task demands require more extensive processing of a feedback stimulus in order to extract its full informational content. Thus, it is conceivable, that due to the increased working memory demands when feedback was delayed, the information conveyed by the feedback was harder to extract resulting in larger P300 amplitude. Furthermore, this increase in P300 amplitude was restricted to negative feedback. This highly specific effect on one particular type of feedback might indicate that especially negative feedback is evaluated by the learners to increase their performance. These results are also in agreement with the proposal that unfavorable events elicit larger P300s than favorable events because of a “negativity bias,” i.e. that negative information tends to influence evaluations more strongly than comparable positive information (Ito et al., 1998).
Another interesting finding is the learning related change in the feedback-locked ERPs. According to the RL theory (Holroyd and Coles, 2002), a decreasing FRN with progress in learning was expected, because as a function of learning participants should rely less on the external feedback. The present analysis partly confirmed this prediction by demonstrating that the amplitude of the difference wave between positive and negative feedback decreased with learning. However, in a separate analysis for the two feedback types, we found learning related changes only for positive but not for negative feedback. Thus, our data suggest that the more participants learn, the more negative the waveform elicited by positive feedback becomes. This finding is supported by the outcome of the regression analysis demonstrating that the reduction in the FRP amplitude elicited by positive feedback is predictive of the individual increase in classification performance from the initial to the later learning phases. These results are consistent with a growing body of evidence suggesting greater modulation of feedback-related ERP effects by positive feedback than by negative feedback (Potts et al., 2006; Eppinger et al., 2008; Holroyd et al., 2008). Importantly, as indicated by the topographic analysis, this reduction of the FRP elicited by correct feedback did not result from a temporal overlap of the FRP with the P300 (see Holroyd et al., 2008, for a discussion of this issue). To date, the most widely accepted interpretation of the learning related decreases in the FRP would be the assumption that this positive-going component reflects a positive prediction error that decreases the more participants are able to internally represent the correctness of the response (Eppinger et al., 2008). In other words, during learning participants develop a mental representation of the underlying grammatical rules and, as a consequence, become able to correctly predict the upcoming feedback and the expectation of positive feedback increases. Further support for this notion is provided by a recent experiment demonstrating that the probability of reward modulated the FRN to wins, but not to losses in a probabilistic RL task (Cohen et al., 2007). Thus, as the expectation of reward or positive feedback increases and, consequently, the positive prediction error decreases during learning, the FRP to positive feedback becomes less positive.
It has been previously suggested that prediction errors might signal the need to adjust behavior (Ridderinkhof et al., 2004). Consequently, the FRN/FRP should not only reflect whether the current feedback is positive or negative but also how behavior has to be adjusted in the future. In case of negative feedback that indicates that some action fails to meet the predicted result, the action representation needs cognitive control for additional processing. Consistent with this view, the RL theory on feedback processing suggests that the midbrain dopamine system modulates activity of the ACC to cognitively control motor behavior (Holroyd and Coles, 2002). Further evidence for the notion that the FRN reflects the need of behavioral adjustments is provided by a recent study where subjects played a strategic economic game against a computer opponent allowing for flexible adaptation of decision strategies based on recent outcomes (Cohen and Ranganath, 2007). In line with predictions of the RL theory, it was found that the magnitude of the FRN after losing to the computer opponent predicted whether subjects would change decision behavior on the subsequent trial. Applying this reasoning to the present results, one could argue that negative feedback in an AGL task provides information for behavioral adjustments that remains constant throughout learning. Consequently, the amplitude of the FRN elicited by this negative feedback is not modulated by learning. Positive feedback, even though reflecting a positive prediction error, also indicates that no adjustment in overt behavior is required. However, some covert action might still be required to adjust the action value of the alternative rules. That means, that in the initial learning phase the information value of positive feedback is relatively low with respect to which of the alternative rules could be applied for the present outcome. During the course of learning the search space of potentially applicable rules is narrowed down, possibly increasing the information value of the positive feedback. As a consequence, the FRP elicited by positive feedback will become more negative with learning. Tentative support for this view can be derived from the study by Cohen and Ranganath (2007). Similar to changes in decision behavior following increased FRN amplitudes after negative feedback, the ERP elicited by positive feedback in this study was more negative when the decision was changed in the upcoming trial. Although this effect was not significant it might suggest that a phasic negative deflection elicited by the feedback stimulus might reflect a decision conflict and, consequently, the need for cognitive control to adjust future overt or covert behavior. However, further studies are required directly testing this suggestion.
Beside learning related changes in the FRP a reduction of the P300 elicited by positive feedback was observed. These effects of learning on the P300 amplitude might be related to subjective expectations regarding the frequency of positive and negative feedback. For instance, subjects may notice that positive feedback is more likely than negative feedback at the end of learning. As the P300 amplitude is inversely related to stimulus probability (Johnson, 1986) the reduction of the P300 in the present experiment might reflect the fact that positive feedback is perceived more frequently than negative feedback at the end of learning.
Taken together, our data suggest that one of the reasons why delayed feedback is less effective for AGL is interference arising from conflicting information of alternative rule sets held in working memory during the delay. This underscores the importance of timely feedback, especially when stimulus complexity and/or task requirements are very demanding, as for instance in AGL. Moreover, the specificity of the delay effect for negative feedback might indicate that especially negative feedback provides more information for learners to increase their performance and is thus more susceptible to interference. In accordance with this view, the need for an adjustment in future behavior, as indicated by a negative feedback, remains constant throughout the entire experiment, and the FRN to negative feedback is elicited in an all-or-none fashion. In addition, the present data support the view that the learning related variance in the feedback-related ERPs is to a large extent caused by positive feedback processing. This view is consistent with several studies showing that learning is driven by an increase in dopaminergic activity when the outcome of an action is better than expected. That is, the positive prediction error, and, as a consequence, the FRP elicited by positive feedback, is reduced with learning, indicating that as a function of learning positive feedback is to a lesser extend considered as better than expected, presumably reflecting that the prediction is confirmed by the feedback stimulus.
Conflict of Interest Statement
The authors declare that the research was conducted in the absence of any commercial or financial relationships that could be construed as a potential conflict of interest.
References
Ashby, F. G., and O’Brien, J. B. (2005). Category learning and multiple memory systems. Trends Cogn. Sci. 9, 83–89.
Ashby, F. G., Queller, S., and Berretty, P. M. (1999). On the dominance of unidimensional rules in unsupervised categorization. Percept. Psychophys. 61, 1178–1199.
Chen, J., Hale, S., and Myerson, J. (2003). Effects of domain, retention interval, and information load on young and older adults? Visuospatial working memory. Neuropsychol. Dev. Cogn. B Aging Neuropsychol. Cogn. 10, 122–133.
Clahsen, H., and Felser, C. (2006). How native-like is non-native language processing? Trends Cogn. Sci. 10, 564–570.
Cohen, M. X., Elger, C. E., and Ranganath, C. (2007). Reward expectation modulates feedback-related negativity and EEG spectra. Neuroimage 35, 968–978.
Cohen, M. X., and Ranganath, C. (2007). Reinforcement learning signals predict future decisions. J. Neurosci. 27, 371–378.
Elman, J. L., Bates, E. A., Johnson, M. H., Karmiloff-Smith, A., Parisi, D., and Plunkett, K. (1996). Rethinking Innateness. Cambridge, MA: MIT Press.
Eppinger, B., Kray, J., Mock, B., and Mecklinger, A. (2008). Better or worse than expected? Aging, learning, and the ERN. Neuropsychologia 46, 521–539.
Forkstam, C., Hagoort, P., Fernandez, G., Ingvar, M., and Petersson, K. M. (2006). Neural correlates of artificial syntactic structure classification. Neuroimage 32, 956–967.
Friederici, A. D., Bahlmann, J., Heim, S., Schubotz, R. I., and Anwander, A. (2006). The brain differentiates human and non-human grammars: functional localization and structural connectivity. Proc. Natl. Acad. Sci. U.S.A. 103, 2458–2463.
Friederici, A. D., Steinhauer, K., and Pfeifer, E. (2002). Brain signatures of artificial language processing: evidence challenging the critical period hypothesis. Proc. Natl. Acad. Sci. U.S.A. 99, 529–534.
Glidden, L. M., and Scott, K. G. (1975). Recognition memory retention interval and information load. J. Exp. Child. Psychol. 19, 195–208.
Gratton, G., Coles, M. G., and Donchin, E. (1983). A new method for off-line removal of ocular artifact. Electroencephalogr. Clin. Neurophysiol. 55, 468–484.
Hagoort, P. (2008). The fractionation of spoken language understanding by measuring electrical and magnetic brain signals. Philos. Trans. R. Soc. Lond., B, Biol. Sci. 363, 1055–1069.
Hahne, A., and Friederici, A. D. (1999). Electrophysiological evidence for two steps in syntactic analysis: early automatic and late controlled processes. J. Cogn. Neurosci. 11, 194–205.
Hauser, M. D., Chomsky, N., and Fitch, W. T. (2002). The faculty of language: what is it, who has it, and how did it evolve? Science 298, 1569–1579Â.
Hoen, M., and Dominey, P. F. (2000). ERP analysis of cognitive sequencing: a left anterior negativity related to structural transformation processing. Neuroreport 11, 3187–3191.
Holroyd, C. B., and Coles, M. G. H. (2002). The neural basis of human error processing: reinforcement learning, dopamine, and the error-related negativity. Psychol. Rev. 109, 679–709.
Holroyd, C. B., Nieuwenhuis, S., Yeung, N., and Cohen, J. D. (2003). Errors in reward prediction are reflected in the event-related brain potential. Neuroreport 14, 2481–2484.
Holroyd, C. B., Nieuwenhuis, S., Yeung, N., Nystrom, L., Mars, R. B., Coles, M. G. H., and Cohen, J. D. (2004). Dorsal anterior cingulate cortex shows fMRI response to internal and external error signals. Nat. Neurosci. 7, 497–498.
Holroyd, C. B., Pakzad-Vaezi, K. L., and Krigolson, O. E. (2008). The feedback correct-related positivity: sensitivity of the event-related brain potential to unexpected positive feedback. Psychophysiology 45, 688–697.
Ito, T. A., Larsen, J. T., Smith, N. K., and Cacioppo, J. T. (1998). Negative information weighs more heavily on the brain: the negativity bias in evaluative categorizations. J. Pers. Soc. Psychol. 75, 887–900.
Johnson, J. S., and Newport, E. L. (1988). Critical period effects in second language learning: the influence of maturational state on the acquisition of English as a second language. Cogn. Psychol. 21, 60–99.
Kaan, E., and Swaab, T. Y. (2003). Repair, revision, and complexity in syntactic analysis: an electrophysiological differentiation. J. Cogn. Neurosci. 15, 98–110.
Kinder, A., and Assmann, A. (2000). Learning artificial grammars: no evidence for the acquisition of rules. Mem. Cogn. 28, 1321–1332.
Kok, A., Ridderinkhof, K. R., and Ullsperger, M. (2006). The control of attention and actions: current research and future developments. Brain Res. 1105, 1–6.
Lieberman, D. A., Vogel, A. C. M., and Nisbet, J. (2008). Why do the effects of delaying reinforcement in animals and delaying feedback in humans differ? A working-memory analysis. Q. J. Exp. Psychol. (Colchester) 61, 194–202.
Maddox, W. T., Ashby, F. G., and Bohil, C. J. (2003). Delayed feedback effects on rule-based and information–integration category learning. J. Exp. Psychol. Learn. Mem. Cogn. 29, 650–662.
Miltner, W. H. R., Braun, C. H., and Coles, M. G. H. (1997). Event-related brain potentials following incorrect feedback in a time-estimation task: evidence for a “generic” neural system for error detection. J. Cogn. Neurosci. 9, 788–798.
Montague, P. R., Dayan, P., and Sejnowski, T. J. (1996). A framework for mesencephalic dopamine systems based on predictive Hebbian learning. J. Neurosci. 16, 1936–1947.
Mueller, J. L., Oberecker, R., and Friederici, A. D. (2009). Syntactic learning by mere exposure–an ERP study in adult learners. BMC Neurosci. 10, 89. doi: 10.1186/1471-2202-10-89
Nieuwenhuis, S., Yeung, N., Holroyd, C. B., Schurger, A., and Cohen, J. D. (2004). Sensitivity of electrophysiological activity from medial frontal cortex to utilitarian and performance feedback. Cereb. Cortex 14, 741–747.
Opitz, B., and Friederici, A. D. (2003). Interactions of the hippocampal system and the prefrontal cortex in learning language-like rules. Neuroimage 19, 1730–1737.
Opitz, B., and Friederici, A. D. (2004). Brain correlates of language learning: the neuronal dissociation of rule-based versus similarity-based learning. J. Cogn. Neurosci. 24, 8436–8440.
Opitz, B., and Friederici, A. D. (2007). The neural basis of processing sequential and hierarchical syntactic structures. Hum. Brain Mapp. 28, 585–592.
Petersson, K. M., Forkstam, C., and Ingvar, M. (2004). Artificial syntactic violations activate Broca’s region. Cogn. Neurosci. 28, 383–407.
Pothos, E. M., and Bailey, T. M. (2000). The role of similarity in artificial grammar learning. J. Exp. Psychol. Learn. Mem. Cogn. 26, 847–862.
Potts, G. F., Martin, L. E., Burton, P., and Montague, P. R. (2006). When things are better or worse than expected: the medial frontal cortex and the allocation of processing resources. J. Cogn. Neurosci. 18, 1112–1119.
Reber, A. S. (1967). Implicit learning of artificial grammars. J. Verbal Learn. Verbal Behav. 6, 855–863.
Ridderinkhof, K. R., van Den Wildenberg, W. P. M., Segalowitz, S. J., and Carter, C. S. (2004). Neurocognitive mechanisms of cognitive control: the role of prefrontal cortex in action selection, response inhibition, performance monitoring, and reward-based learning. Brain Cogn. 56, 129–140.
Sharbrough, F., Chatrian, G.-E., Lesser, R. P., Lüders, H., Nuwer, M., and Picton, T. W. (1991). American Electroencephalographic Society guidelines for standard electrode position nomenclature. J. Clin. Neurophysiol. 8, 200–202.
Ullsperger, M., and von Cramon, D. Y. (2006). The role of intact frontostriatal circuits in error processing. J. Cogn. Neurosci. 18, 651–664.
Keywords: artificial grammar learning, ERN, feedback positivity, delayed feedback
Citation: Opitz B, Ferdinand NK and Mecklinger A (2011) Timing matters: the impact of immediate and delayed feedback on artificial language learning. Front. Hum. Neurosci. 5:8. doi: 10.3389/fnhum.2011.00008
Received: 20 January 2010;
Paper pending published: 02 February 2010;
Accepted: 11 January 2011;
Published online: 01 February 2011.
Edited by:
Hans-Jochen Heinze, University of Magdeburg, GermanyReviewed by:
Markus Ullsperger, Max Planck Institute for Neurological Research, GermanyThomas F. Münte, University of Magdeburg, Germany
Copyright: © 2011 Opitz, Ferdinand and Mecklinger. This is an open-access article subject to an exclusive license agreement between the authors and Frontiers Media SA, which permits unrestricted use, distribution, and reproduction in any medium, provided the original authors and source are credited.
*Correspondence: Bertram Opitz, Experimental Neuropsychology Unit, Saarland University, Campus A2.4, 66123 Saarbrücken, Germany. e-mail: b.opitz@mx.uni-saarland.de