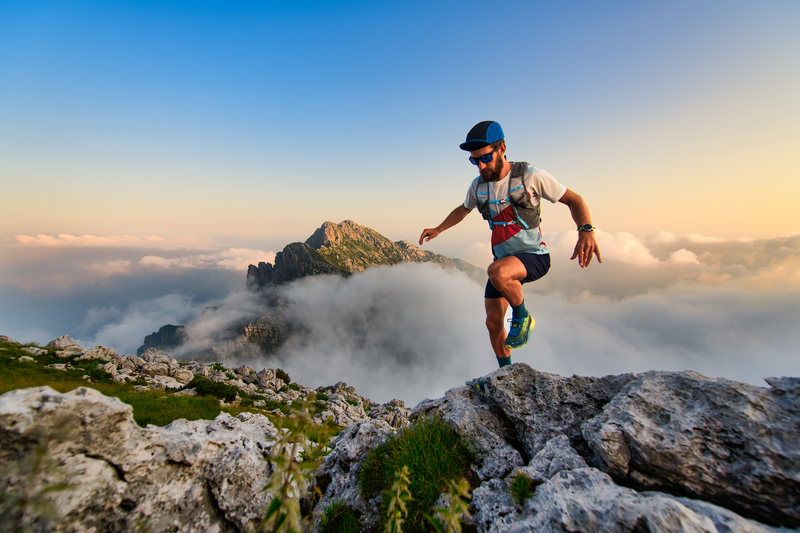
95% of researchers rate our articles as excellent or good
Learn more about the work of our research integrity team to safeguard the quality of each article we publish.
Find out more
ORIGINAL RESEARCH article
Front. Neurol. , 03 March 2025
Sec. Stroke
Volume 16 - 2025 | https://doi.org/10.3389/fneur.2025.1434740
This article is part of the Research Topic Advances and controversies in ischemic stroke management: from prevention to diagnosis and acute treatment View all 95 articles
Objective: This study aims to elucidate the potential impact of basal metabolic rate on ischemic stroke at the genetic prediction level through a two-sample Mendelian randomization analysis.
Methods: Using summary data from genome-wide association studies, we obtained information on basal metabolic rate and ischemic stroke from a large-scale genome-wide association study. MR analysis used inverse variance weighting, weighted median, MR-Egger, simple mode, and weighted estimation. Sensitivity analyses, including the MR-Egger method, MR-PRESSO, Cochran’s Q-test, and leave-one-out assessment, were performed to assess the reliability of the results.
Results: Genetic susceptibility to basal metabolic rate was significantly associated with ischemic stroke in multiple models, including the inverse variance weighting model (OR, 1.108 [95% CI: 1.005–1.221]; p = 0.0392), the weighted median method (OR, 1.179 [95% CI: 1.020–1.363]; p = 0.0263), and MR-Egger (OR, 1.291 [95% CI: 1.002–1.663]; p = 0.0491). These results indicate a positive causal relationship between basal metabolic rate and ischemic stroke. The MR-Egger intercept and Cochran’s Q-test indicated the absence of heterogeneity and horizontal pleiotropy in the analyses of basal metabolic rate and ischemic stroke.
Conclusion: The MR analysis suggests a positive correlation between basal metabolic rate and ischemic stroke.
Ischemic stroke (IS) accounts for the majority of stroke cases, representing 87% of all occurrences (1). The narrow therapeutic window, the risk of complications, and limited treatment efficacy impose significant constraints, resulting in only a small proportion of patients receiving treatment (2). Therefore, it is particularly important to focus on preventing potential risk factors for IS and reducing its incidence in the early stages.
Basal Metabolic Rate (BMR) is a critical indicator of the minimum metabolic activity necessary to sustain life, and also a significant component of total energy expenditure. An individual’s BMR is primarily influenced by factors such as age, genetic makeup, body weight, environmental temperature, and overall health status (3). High blood pressure is a well-established risk factor for IS, and recent studies have demonstrated that an increase in BMR can lead to elevated blood pressure (4). Furthermore, IS is closely linked to brain energy metabolism (5). Disruption of the brain’s energy metabolism process can exacerbate the condition. Current Mendelian randomization (MR) studies on BMR primarily focus on its causal relationship with cardiovascular diseases and its association with inflammatory markers. Furthermore, a cross-sectional study (6) on stroke patients indicated that individuals with IS had higher BMR levels, though it did not elucidate the causal relationship. Therefore, based on the aforementioned evidence, we raise the question: Is there a causal relationship between BMR and IS?
Understanding the interaction between BMR and IS is essential for revealing the underlying mechanisms. This study not only provides a new perspective for predicting the occurrence of IS but may also offer scientific evidence to reduce its incidence and improve disease management. However, clarifying the causal relationship is challenging due to the intricate interactions among physiological factors. Although randomized controlled trials (RCTs) are considered the gold standard for causal inference, they are complex in design and resource-intensive. Traditional observational studies (7), however, are susceptible to reverse causality, confounding factors, and sample size limitations. In contrast, MR effectively addresses these limitations by leveraging data from genome-wide association studies (GWAS) and using single nucleotide polymorphisms (SNPs) as instrumental variables, offering a more reliable approach to uncovering causal relationships between exposures and clinical outcomes (8).
Mendelian randomization (MR) studies must satisfy three core assumptions: (1) the instrumental variables (IVs) must be strongly associated with the exposure. (2) The IVs must be independent of any confounding factors. (3) The IVs must affect the outcome only through the exposure, with no direct effect on the outcome beyond the exposure (9). This study uses BMR as the exposure variable and IS as the outcome variable. We perform a two-sample MR analysis to investigate the causal relationship between BMR and IS. The specific research methodology is shown in Figure 1.
The data for BMR and IS were obtained from the GWAS database.1 The GWAS dataset for BMR (GWAS ID: ebi-a-GCST90029025) was obtained from the UK Biobank by Po-Ru Loh and colleagues using the BOLT-LMM Bayesian mixed model association method, which resulted in a cohort analysis of 534,045 participants and identified 11,973,469 SNPs (10). Similarly, the GWAS dataset for IS (GWAS ID: ebi-a-GCST90018864) included 484,121 participants (11,929 cases and 472,192 controls) and 9,587,836 SNPs (11). Both datasets focused on European populations to minimize biases from racial differences and are publicly available in the IEU GWAS database. Therefore, no additional ethical approvals are required. Further details on these data can be found in Supplementary Table 1.
To satisfy assumption 2, we selected instrumental variables for BMR based on genome-wide significance (p < 5e−8). Using the clump function from the TwoSampleMR package in R (version 4.3.3), we performed linkage disequilibrium analysis (r2 < 0.001, window size >10,000 kb) to ensure the independence of the instrumental variables. Subsequently, we excluded SNPs with intermediate allele frequencies, palindromic SNPs, and incompatible SNPs from the selection.
To eliminate potential confounding SNPs associated with the outcome variable, we used the PhenoScanner database.2 Previous studies have identified risk factors causally associated with IS, including blood lipids (12), blood pressure (13), and type 2 diabetes (14). In this study, these risk factors are regarded as potential confounders. Therefore, we excluded SNPs associated with blood lipid levels, blood pressure (including both diastolic and systolic pressure), and type 2 diabetes. Finally, to ensure the robustness of our analysis, we applied a stringent threshold for statistical strength, setting F > 10 as the criterion for strong associations to minimize the potential for weak instrument bias. If F < 10, the corresponding SNP should be excluded (15). The formula for calculating the F-statistic is presented below:
In this context, R2 represents the proportion of variance in BMR explained by the SNP, indicating the extent to which the instrumental variable accounts for the exposure (16). N represents the sample size of the GWAS, and K denotes the number of instrumental variables.
The selection of IVs in this study adhered to a rigorous step-by-step process based on the outlined methodology. Initially, we used the “TwoSampleMR” package in R to select 598 SNPs for the analysis of IS. Subsequently, 28 palindromic SNPs were excluded from the analysis. Additionally, 48 SNPs were excluded due to their association with potential confounding factors related to IS. Following this, we applied the MR-PRESSO method, which excluded 234 outlier SNPs (Supplementary Table 2). The remaining 288 SNPs were then subjected to F-statistic calculation. The F-statistics for these 288 SNPs ranged from 11.408 to 290.562, with all values exceeding 10, indicating that the potential for weak instrument bias was minimized. The combined R2 value for these 288 SNPs was 2.03%, demonstrating their robustness. Consequently, these 288 SNPs were ultimately included in our study (Supplementary Table 3).
In this study, we aim to establish the causal relationship between BMR and IS using several methodologies, including the Inverse-Variance Weighted (IVW) method, the Weighted Median (WME) method, the MR-Egger method, the weighted method, and the simple model. The core concept of the IVW method is to combine the effects of multiple genetic instrumental variables on exposure and outcome, with weights inversely proportional to the estimated variance (17). This weighting approach aims to provide more accurate and reliable results by giving greater weight to instrumental variables with lower standard errors. This method enhances the robustness of the estimation process by reducing the impact of random errors. Pleiotropy occurs when a genetic variant affects multiple phenotypes or biological pathways (18).
In MR studies, pleiotropy in IVs can lead to biased estimates of the causal relationship between exposure and outcome. The MR-Egger regression method is designed to address pleiotropy in instrumental variables, offering a method to detect and correct for bias caused by directional pleiotropy. When assumption 3 of Mendelian randomization is violated, MR-Egger regression is necessary to produce a robust estimate of the causal relationship between exposure and outcome (19). Heterogeneity refers to significant variation in effect estimates among different instrumental variables, which may indicate pleiotropy or other underlying issues. To detect such heterogeneity, we applied the IVW method and MR-Egger regression to identify SNPs with heterogeneity, followed by Cochran’s Q statistic to quantify the heterogeneity (20). Subsequently, we performed sensitivity analyses, such as leave-one-out analysis, to determine whether any individual SNPs significantly influence the primary causal relationship (21). Additionally, when horizontal pleiotropy is below 50%, the MR-PRESSO method is recommended, incorporating the MR-PRESSO global test to detect horizontal pleiotropy. The MR-PRESSO outlier test excludes outlier SNPs and estimates corrected results (18).
The “Two Sample MR” R package was used for all two-sample MR analyses and associated sensitivity tests. The MR-PRESSO analysis was performed using the R package “MR-PRESSO.” Statistical analyses were conducted in R (version 4.3.3), with a significance threshold set at p < 0.05 to determine statistical significance.
We used the IVW method to analyze the relationship between BMR and IS, revealing a potential positive genetic correlation between BMR and the risk of IS (odds ratio [OR], 1.090 [95% CI: 1.004–1.182]; p = 0.0396). The WME yielded similar results (OR, 1.151 [95% CI: 1.018–1.301]; p = 0.0244). Nonetheless, upon employing the MR-Egger method for analysis, we did not observe a statistically significant association between BMR and IS (OR, 1.139 [95% CI: 0.915–1.418]; p = 0.2454) (Supplementary Table 4; Figure 2). After removing 234 outlier SNPs, the MR-Egger intercept showed no evidence of directional pleiotropy (Egger intercept = −0.002, p = 0.2010). The MR-PRESSO global test rigorously scrutinized horizontal pleiotropy (p < 0.05), providing evidence of no statistically significant directional horizontal pleiotropy in our analysis (Supplementary Table 5). We conducted Cochran’s Q test both before and after removing outliers. Before outlier removal, the results indicated significant heterogeneity (MR-Egger: Q value = 598.632, p = 0.009; IVW: Q value = 598.842, p = 0.010) (Supplementary Table 6). Although we observed some heterogeneity in our analysis, the impact on our results was minimal due to the use of a random effects model. After the removal of outliers, our analysis showed no substantial heterogeneity (MR-Egger: Q value = 311.501, p = 0.144; IVW: Q value = 313.293, p = 0.1370) (Supplementary Table 6). Finally, we conducted IVW analysis (OR, 1.108 [95% CI: 1.005–1.221]; p = 0.0392), WME (OR, 1.179 [95% CI: 1.020–1.363]; p = 0.0263), and MR-Egger (OR, 1.291 [95% CI: 1.002–1.663]; p = 0.0491) on the final set of 288 SNPs (Supplementary Table 4; Figure 2). All results were statistically significant. The findings from the MR-Egger and weighted median sensitivity analyses supported the conclusions drawn from the IVW analyses, further reinforcing the consistency of the results. Scatter plots and funnel plots are provided in the supplementary figures (Supplementary Figures 1, 2). Additionally, our thorough examination using the leave-one-out analysis (Supplementary Figure 3) failed to detect any specific SNPs that significantly altered the overall relationship between BMR and IS. Thus, based on this comprehensive assessment, we confidently assert that BMR exerts a positive causal influence on IS.
Figure 2. Forest plots of Mendelian randomization analyses of the causal effects of BMR on IS before and after MR-PRESSO. (A) Forest plots of Mendelian randomization analyses of the causal effects of BMR on IS at before MR-PRESSO. (B) Forest plots of Mendelian randomization analyses of the causal effects of BMR on IS after MR-PRESSO.
In this study, we also conducted a reverse MR analysis to assess the causal impact of IS on BMR. The data sources for this analysis were consistent with those mentioned earlier. We initially selected instrumental variables associated with IS, resulting in 14 SNPs (Supplementary Table 7). After performing the MR analysis, we found no significant causal effect of IS on BMR in IVW analysis (OR, 1.004 [95% CI: 0.9708–1.0380]; p = 0.8224) (Supplementary Table 8).
We conducted MR analysis, and the final results indicated a positive effect of BMR on IS. We also performed various sensitivity analyses to ensure the robustness of our findings. This study found a significant association between BMR and the risk of IS, consistent with previous findings, and further established the causal relationship between BMR and IS. Previous studies have mainly focused on specific populations or short-term analyses, whereas this study strengthens the evidence by validating the robustness of this relationship using large samples and long-term data. However, these results may be influenced by certain confounding factors.
The first potential factor is that an increase in BMR indicates a higher energy demand from the body, including respiratory metabolism, organ function, neural activity, and muscle activity (22, 23). Energy production is closely related to mitochondria, the organelles primarily responsible for adenosine triphosphate (ATP) production. When BMR increases, energy demands rise, leading to a significant increase in mitochondrial workload, which may elevate the risk of mitochondrial dysfunction (24, 25). Furthermore, research has indicated a positive correlation between resting metabolic rate (RMR) and various aspects of mitochondrial function, including small molecule transport across mitochondrial membranes, as well as inner and outer membrane transport and mitochondrial transfer (26). RMR and BMR are closely related, as both describe energy expenditure during rest. Although they differ, they often have similar values and are generally considered equivalent in the study (27, 28). This correlation between BMR and mitochondrial transport suggests that an excessively high BMR may lead to an increase in mitochondrial transport rates, potentially causing decompensation. This decompensation may result in an imbalance in the internal mitochondrial environment, such as disturbances in cellular calcium ion flux, which may disrupt mitochondrial function (29, 30), indirectly indicating that a high BMR may lead to mitochondrial dysfunction.
Mitochondrial dysfunction can lead to several consequences, including energy metabolism disorders, redox imbalance, increased apoptosis, cellular calcium ion imbalance, and disrupted mitochondrial autophagy (31). Recent studies on the cellular and molecular mechanisms associated with IS have suggested that redox imbalance, cellular calcium ion dysregulation, and disruptions in mitochondrial autophagy may be linked to IS (32–34). Interestingly, an MR analysis examining the relationship between BMR and vascular disease risk, based on mitochondrial aging theory, also concluded that increased BMR can lead to a surge in reactive oxygen species (ROS) (35). These findings are consistent with our results.
An increase in ROS primarily results from redox imbalance, leading to enhanced oxidative stress. Calcium ion dysregulation is associated with mitochondrial transport; when mitochondrial transport increases, cellular calcium ion concentrations tend to rise, potentially increasing the risk of IS. Mitochondrial autophagy is a crucial physiological process, and its disruption can trigger a cascade of adverse effects (36). A potential mechanism underlying these effects could be that the pathogenesis of IS involves oxidative stress and inflammatory responses. Disruption of mitochondrial autophagy could exacerbate oxidative stress, leading to excess ROS production and increased release of inflammatory cytokines (34, 37). These pieces of evidence suggest that the causal relationship between BMR and IS may be driven by underlying mitochondrial dysfunction, warranting further exploration of the biological mechanisms involved in future studies.
Thyroid-stimulating hormone (TSH) levels may play a crucial role in the observed association between BMR and IS. Research indicates that thyroid hormone secretion is correlated with BMR levels and plays a critical role in regulating basal metabolic rate (38). As BMR increases, the hypothalamus reduces the release of thyrotropin-releasing hormone (TRH), which subsequently lowers TSH release, leading to a decrease in thyroid hormone production (39). This physiological process is regulated by the hypothalamic–pituitary-thyroid (HPT) axis. A reduction in TSH levels can increase the likelihood of hyperthyroidism (39, 40). An MR study investigating the impact of thyroid function on stroke risk through atrial fibrillation, along with another study examining stroke risk within the normal thyroid function range, found a significant association between higher TSH levels within the normal range and lower stroke risk, potentially mediated by atrial fibrillation (41, 42). Elevated BMR may increase the risk of IS due to its effect on TSH levels.
Furthermore, thrombosis is a direct pathological cause of IS (43). An MR study suggested that higher BMR may increase the risk of venous thrombosis in the lower limbs, potentially through BMR-induced endothelial dysfunction, which creates a pro-inflammatory and pro-coagulant environment in the vascular system (44). Although IS is caused by arterial thrombosis in the brain, the underlying mechanisms in the vascular system are similar. Therefore, we hypothesize that the causal relationship between BMR and IS may be mediated by thrombosis, and further investigation is needed to confirm the link between BMR and cerebral blood vessels.
The results of this study suggest a potential causal relationship between high BMR levels and IS, indicating that elevated BMR could serve as an early risk marker for IS. Monitoring individual BMR levels, especially in populations with metabolic abnormalities, could help identify high-risk individuals early, enabling timely intervention. This provides a new perspective for clinical practice and stroke prevention strategies. Furthermore, future studies could investigate whether lifestyle changes or pharmacological treatments can help individuals with elevated BMR maintain it within the normal range, potentially aiding in the prevention of IS. If successful, these approaches could expand clinical strategies for stroke prevention and open new avenues for reducing stroke risk in individuals with elevated BMR.
This study presents an MR analysis of the relationship between BMR and IS, providing new insights into early stroke prevention for individuals with elevated BMR. While numerous studies have explored BMR and cardiovascular health, research specifically investigating BMR and IS is still lacking, making this study innovative in its focus. Furthermore, the use of MR analysis reduces the impact of confounding factors and biases, enabling more robust causal inferences.
Despite these strengths, our study has several limitations. First, the GWAS data used in this analysis were derived from European populations, raising uncertainty about the applicability of the results to non-European populations. Second, although IS was used as the outcome measure, we did not conduct subgroup analyses by gender, age group, or geographic region due to the limitations of the GWAS database, which limits our ability to assess the stability of outcomes across different subgroups. Third, due to the inability to extract data on hemorrhagic stroke, our analysis was restricted to IS.
In conclusion, our research findings suggest that a higher BMR may increase the risk of IS. Intervention measures aimed at improving BMR may be beneficial in reducing the incidence of IS.
The datasets presented in this study can be found in online repositories. The names of the repository/repositories and accession number(s) can be found in the article/Supplementary material.
Ethical review and approval was not required for the study on human participants in accordance with the local legislation and institutional requirements. Written informed consent from the patients/participants or patients/participants’ legal guardian/next of kin was not required to participate in this study in accordance with the national legislation and the institutional requirements.
YC: Writing – original draft, Writing – review & editing, Conceptualization, Formal analysis, Investigation, Methodology, Software, Visualization. XZ: Writing – review & editing. ML: Writing – review & editing. YZ: Writing – review & editing. SL: Writing – review & editing. LZ: Writing – review & editing. XY: Writing – review & editing. XC: Writing – review & editing. MY: Writing – review & editing. QQ: Writing – review & editing. YQ: Writing – review & editing. JS: Writing – review & editing.
The author(s) declare that financial support was received for the research, authorship, and/or publication of this article. The study was supported by the National Natural Science Foundation of China (No.82060901), the Fundamental Research Key Project of Yunnan Science and Technology Department (202101AZ070001-165), and the Special Project for Famous Doctors of “Xingdian Talent Support Program” in Yunnan Province.
We thank all of the investigators for sharing summary level data on GWAS for basal metabolic rate and ischemic stroke.
The authors declare that the research was conducted in the absence of any commercial or financial relationships that could be construed as a potential conflict of interest.
All claims expressed in this article are solely those of the authors and do not necessarily represent those of their affiliated organizations, or those of the publisher, the editors and the reviewers. Any product that may be evaluated in this article, or claim that may be made by its manufacturer, is not guaranteed or endorsed by the publisher.
The Supplementary material for this article can be found online at: https://www.frontiersin.org/articles/10.3389/fneur.2025.1434740/full#supplementary-material
BMR, basal metabolic rate; IS, ischemic stroke; MR, Mendelian randomization; GWAS, genome-wide association studies; SNPs, single nucleotide polymorphisms; IVs, instrumental variables; RCTs, randomized controlled trials; IVW, inverse variance weighted; WME, Weighted Median Estimation; ATP, adenosine triphosphate; RMR, resting metabolic rate; ROS, reactive oxygen species; TSH, Thyroid-stimulating hormone; TRH, thyrotropin-releasing hormone; HPT, hypothalamic pituitary thyroid.
2. Powers, WJ, Rabinstein, AA, and Ackerson, T. Guidelines for the early Management of Patients with Acute Ischemic Stroke: 2019 update to the 2018 guidelines for the early Management of Acute Ischemic Stroke: a guideline for healthcare professionals from the American Heart Association/American Stroke Association. Stroke. (2019) 50:e344–418. doi: 10.1161/STR.0000000000000211
3. Bi, X, Forde, CG, and Goh, AT. Basal metabolic rate and body composition predict habitual food and macronutrient intakes: gender differences. Nutrients. (2019) 11:2653. doi: 10.3390/nu11112653
4. Ali, N, Mahmood, S, and Manirujjaman, M. Hypertension prevalence and influence of basal metabolic rate on blood pressure among adult students in Bangladesh. BMC Public Health. (2017) 18:58. doi: 10.1186/s12889-017-4617-9
5. Sifat, AE, Nozohouri, S, and Archie, SR. Brain energy metabolism in ischemic stroke: effects of smoking and diabetes. Int J Mol Sci. (2022) 23:8512. doi: 10.3390/ijms23158512
6. Wilczyński, J, Mierzwa-Molenda, M, and Habik-Tatarowska, N. Differences in body composition among Patientsafter hemorrhagic and ischemic stroke. Int J Environ Res Public Health. (2020) 17:4170. doi: 10.3390/ijerph17114170
7. Smith, GD, and Ebrahim, S. Mendelian randomization: prospects, potentials, and limitations. Int J Epidemiol. (2004) 33:30–42. doi: 10.1093/ije/dyh132
8. Bowden, J, and Holmes, MV. Meta-analysis and Mendelian randomization: a review. Res Synth Methods. (2019) 10:486–96. doi: 10.1002/jrsm.1346
9. Richmond, RC, and Davey, SG. Mendelian randomization: concepts and scope. Cold Spring Harb Perspect Med. (2022) 12:a040501. doi: 10.1101/cshperspect.a040501
10. Loh, PR, Kichaev, G, and Gazal, S. Mixed-model association for biobank-scale datasets. Nat Genet. (2018) 50:906–8. doi: 10.1038/s41588-018-0144-6
11. Sakaue, S, Kanai, M, and Tanigawa, Y. A cross-population atlas of genetic associations for 220 human phenotypes. Nat Genet. (2021) 53:1415–24. doi: 10.1038/s41588-021-00931-x
12. Bétrisey, S, Haller, ML, and Efthimiou, O. Lipid-lowering therapy and risk of hemorrhagic stroke: a systematic review and Meta-analysis of randomized controlled trials. J Am Heart Assoc. (2024) 13:e030714. doi: 10.1161/JAHA.123.030714
13. Buonacera, A, Stancanelli, B, and Malatino, L. Stroke and hypertension: an appraisal from pathophysiology to clinical practice. Curr Vasc Pharmacol. (2019) 17:72–84. doi: 10.2174/1570161115666171116151051
14. van Sloten, TT, Sedaghat, S, and Carnethon, MR. Cerebral microvascular complications of type 2 diabetes: stroke, cognitive dysfunction, and depression. Lancet Diabetes Endocrinol. (2020) 8:325–36. doi: 10.1016/S2213-8587(19)30405-X
15. Burgess, S, and Thompson, SG CRP CHD Genetics Collaboration. Avoiding bias from weak instruments in Mendelian randomization studies. Int J Epidemiol. (2011) 40:755–64. doi: 10.1093/ije/dyr036
16. Pierce, BL, and Burgess, S. Efficient design for Mendelian randomization studies: subsample and 2-sample instrumental variable estimators. Am J Epidemiol. (2013) 178:1177–84. doi: 10.1093/aje/kwt084
17. Brion, MJ, Shakhbazov, K, and Visscher, PM. Calculating statistical power in Mendelian randomization studies. Int J Epidemiol. (2013) 42:1497–501. doi: 10.1093/ije/dyt179
18. Verbanck, M, Chen, CY, and Neale, B. Detection of widespread horizontal pleiotropy in causal relationships inferred from Mendelian randomization between complex traits and diseases. Nat Genet. (2018) 50:693–8. doi: 10.1038/s41588-018-0099-7
19. Burgess, S, and Thompson, SG. Interpreting findings from Mendelian randomization using the MR-egger method. Eur J Epidemiol. (2017) 32:377–89. doi: 10.1007/s10654-017-0255-x
20. Sanderson, E, Smith, GD, and Windmeijer, F. Corrigendum to: an examination of multivariable Mendelian randomization in the single-sample and two-sample summary data settings. Int J Epidemiol. (2020) 49:1057. doi: 10.1093/ije/dyaa101
21. Nolte, IM. Metasubtract: an R-package to analytically produce leave-one-out meta-analysis GWAS summary statistics. Bioinformatics (Oxford, England). (2020) 36:4521–2. doi: 10.1093/bioinformatics/btaa570
22. Genoud, M, Isler, K, and Martin, RD. Comparative analyses of basal rate of metabolism in mammals: data selection does matter. Biol Rev Camb Philos Soc. (2018) 93:404–38. doi: 10.1111/brv.12350
23. Dahmen, N, Tonn, P, and Messroghli, L. Basal metabolic rate in narcoleptic patients. Sleep. (2009) 32:962–4. doi: 10.1093/sleep/32.7.962
24. Zong, WX, Rabinowitz, JD, and White, E. Mitochondria and Cancer. Mol Cell. (2016) 61:667–76. doi: 10.1016/j.molcel.2016.02.011
25. Chen, W, Zhao, H, and Li, Y. Mitochondrial dynamics in health and disease: mechanisms and potential targets. Signal Transduct Target Ther. (2023) 8:333. doi: 10.1038/s41392-023-01547-9
26. Dubé, JJ, Collyer, ML, and Trant, S. Decreased mitochondrial dynamics is associated with insulin resistance, metabolic rate, and fitness in African Americans. J Clin Endocrinol Metab. (2020) 105:1210–20. doi: 10.1210/clinem/dgz272
27. Alazzam, AM, Alrubaye, MW, and Goldsmith, JA. Trends in measuring BMR and RMR after spinal cord injury: a comprehensive review. Br J Nutr. (2023) 130:1720–31. doi: 10.1017/S0007114523000831
28. Jéquier, E, Acheson, K, and Schutz, Y. Assessment of energy expenditure and fuel utilization in man. Annu Rev Nutr. (1987) 7:187–208. doi: 10.1146/annurev.nu.07.070187.001155
29. Nakanishi, T, Kawasaki, Y, and Nakamura, Y. An implication of the mitochondrial carrier SLC25A3 as an oxidative stress modulator in NAFLD. Exp Cell Res. (2023) 431:113740. doi: 10.1016/j.yexcr.2023.113740
30. Bertero, E, and Maack, C. Calcium signaling and reactive oxygen species in mitochondria. Circ Res. (2018) 122:1460–78. doi: 10.1161/CIRCRESAHA.118.310082
31. Sorrentino, V, Menzies, KJ, and Auwerx, J. Repairing mitochondrial dysfunction in disease. Annu Rev Pharmacol Toxicol. (2018) 58:353–89. doi: 10.1146/annurev-pharmtox-010716-104908
32. Orellana-Urzúa, S, Rojas, I, and Líbano, L. Pathophysiology of ischemic stroke: role of oxidative stress. Curr Pharm Des. (2020) 26:4246–60. doi: 10.2174/1381612826666200708133912
33. Ludhiadch, A, Sharma, R, and Muriki, A. Role of calcium homeostasis in ischemic stroke: a review. CNS Neurol Disord Drug Targets. (2022) 21:52–61. doi: 10.2174/1871527320666210212141232
34. Cai, Y, Yang, E, and Yao, X. FUNDC1-dependent mitophagy induced by tPA protects neurons against cerebral ischemia-reperfusion injury. Redox Biol. (2021) 38:101792. doi: 10.1016/j.redox.2020.101792
35. Ning, L, He, C, and Lu, C. Association between basal metabolic rate and cardio-metabolic risk factors: evidence from a Mendelian randomization study. Heliyon. (2024) 10:e28154. doi: 10.1016/j.heliyon.2024.e28154
36. Goodall, EA, Kraus, F, and Harper, JW. Mechanisms underlying ubiquitin-driven selective mitochondrial and bacterial autophagy. Mol Cell. (2022) 82:1501–13. doi: 10.1016/j.molcel.2022.03.012
37. Tan, Z, Dong, F, and Wu, L. Transcutaneous electrical nerve stimulation (TENS) alleviates brain ischemic injury by regulating neuronal oxidative stress, Pyroptosis, and Mitophagy. Mediat Inflamm. (2023) 2023:1–13. doi: 10.1155/2023/5677865
38. Kim, B. Thyroid hormone as a determinant of energy expenditure and the basal metabolic rate. Thyroid. (2008) 18:141–4. doi: 10.1089/thy.2007.0266
39. Mullur, R, Liu, YY, and Brent, GA. Thyroid hormone regulation of metabolism. Physiol Rev. (2014) 94:355–82. doi: 10.1152/physrev.00030.2013
40. Silva, JE. Thyroid hormone control of thermogenesis and energy balance. Thyroid. (1995) 5:481–92. doi: 10.1089/thy.1995.5.481
41. Marouli, E, Kus, A, and Del Greco, MF. Thyroid function affects the risk of stroke via atrial fibrillation: a Mendelian randomization study. J Clin Endocrinol Metab. (2020) 105:2634–41. doi: 10.1210/clinem/dgaa239
42. Chaker, L, Baumgartner, C, and den Elzen, WPJ. Thyroid function within the reference range and the risk of stroke: an individual participant data analysis. J Clin Endocrinol Metab. (2016) 101:4270–82. doi: 10.1210/jc.2016-2255
43. Hankey, GJ. Secondary stroke prevention. Lancet Neurol. (2014) 13:178–94. doi: 10.1016/S1474-4422(13)70255-2
Keywords: basal metabolic rate, ischemic stroke, causal relationship, Mendelian randomization, MR analysis
Citation: Chen Y, Zhang X, Liu M, Zhang Y, Li S, Zhou L, Yang X, Chen X, Yue M, Qu Q, Qiu Y and Shi J (2025) The association between basal metabolic rate and ischemic stroke: a Mendelian randomization study. Front. Neurol. 16:1434740. doi: 10.3389/fneur.2025.1434740
Received: 18 May 2024; Accepted: 16 January 2025;
Published: 03 March 2025.
Edited by:
Giovanni Merlino, Udine University Hospital, ItalyReviewed by:
Jian Guo, Peking Union Medical College Hospital (CAMS), ChinaCopyright © 2025 Chen, Zhang, Liu, Zhang, Li, Zhou, Yang, Chen, Yue, Qu, Qiu and Shi. This is an open-access article distributed under the terms of the Creative Commons Attribution License (CC BY). The use, distribution or reproduction in other forums is permitted, provided the original author(s) and the copyright owner(s) are credited and that the original publication in this journal is cited, in accordance with accepted academic practice. No use, distribution or reproduction is permitted which does not comply with these terms.
*Correspondence: Yong Qiu, MjIyNTg1MTY3NEBxcS5jb20=; Jing Shi, MjY2MjgzMTI5MUBxcS5jb20=
†These authors have contributed equally to this work
Disclaimer: All claims expressed in this article are solely those of the authors and do not necessarily represent those of their affiliated organizations, or those of the publisher, the editors and the reviewers. Any product that may be evaluated in this article or claim that may be made by its manufacturer is not guaranteed or endorsed by the publisher.
Research integrity at Frontiers
Learn more about the work of our research integrity team to safeguard the quality of each article we publish.