- Department of Neurology, Faculty of Medicine, Oita University, Oita, Japan
Background: Social isolation is a significant public health concern in aging societies. The association between conversation time and social behavior remains unclear. This study examines whether objective conversation time is associated with social activity frequency in older adults.
Methods: This prospective cohort study enrolled 855 older adults (538 women; mean age, 73.8 years) aged 65 and older, who were followed from 2015 to 2019. All participants wore a wristband sensor to measure conversation time for at least 9 days and an average of 31.3 days per year. Social behaviors were assessed through interviews, and the frequency of engagement in community activities, outings, lessons, or classes and contact frequency were assessed using a self-report questionnaire. The association between conversation time and social behavior was evaluated using multi-linear regression analysis.
Results: Conversation time was significantly associated with the frequency of engagement in community activities and lessons or classes after adjusting for several covariates (β = 0.181, 95% confidence interval: 0.107–0.254, p < 0.001; β = 0.11, 95% confidence interval: 0.04–0.179, p = 0.002).
Conclusion: Objectively measured conversation time using a wearable sensor is associated with social behavior and may be a valuable parameter for social isolation in older adults.
Introduction
There is growing evidence that social isolation is implicated in an increased risk of poor health, all-cause mortality, depressive symptoms, cardiovascular disease, and cognitive decline (1–3). In recent years, social network sizes and contact with communities, neighborhoods, and families have decreased (4, 5). In particular, older adults frequently experience social isolation due to declining physical mobility, cognitive function, and economic resources or roles in society, as well as the death of their spouses. Globally, more than 25% of adults aged 65 and older experience social isolation (6), making it a significant public health concern in aging societies (7). Social isolation is a major modifiable risk factor for dementia, and social activity can increase cognitive reserve, improve motivation for healthy behaviors, and reduce stress and inflammation (8–10). Therefore, early identification and management of older adults at risk of social isolation is essential for public health. Two distinct terms refer to subjective experience and objective isolation from other social actors: loneliness refers to the perceived experience of being isolated, while social isolation denotes the actual absence of social contact. This study prefers the term “social isolation” over “loneliness.” Social isolation is characterized as a scarcity of social contact and communication with a broader community, neighborhood, and relatives. It is measured by the frequency of social engagement or social contact and social network size, including marital status or family structure, via questionnaires (4, 5, 11). However, the usefulness of subjective self-report questionnaires is limited with respect to consistency and reliability due to recall bias or misclassification among older adults (12, 13). Moreover, the social stigma of social isolation reduces the likelihood of reporting social isolation, resulting in decreased identification of isolated individuals. Developing an objective method to assess social isolation would help address it among older adults. Wristband sensors are cost-effective and non-invasive tools for objectively and continuously measuring lifestyle factors without recall bias in the community (14–17). We have developed a wristband sensor for quantifying conversation time and used it in a prospective cohort study on the association of lifestyle factors with cognitive function in older adults living in the community (16). The random forest regression analysis results show that objective conversation time is non-linearly associated with the global cognitive function. These results lead us to hypothesize that decreased conversation time may be related to cognitive impairment through social isolation in older adults. Several studies have examined the association of objectively measured conversation time using smartphones with loneliness in young adults or patients with schizophrenia spectrum disorders (18–20). To the best of our knowledge, few studies have measured conversation time using wearable sensors in older adults living in the community. Therefore, this study aims to determine whether objective conversation time is cross-sectionally associated with social behavior.
Methods
Study participants
This USUKI study is a prospective cohort study designed to explore the risk or protective lifestyle factors for dementia among community-dwelling older adults aged ≥65 years without dementia in Usuki, Oita Prefecture, Japan (16, 17). The criteria for participation included residents of Usuki, good physical and mental health, and independence in activities of daily living. All participants were asked to wear a wristband sensor (Silmee™ W20, TDK Corporation, Tokyo, Japan), except when bathing, for an average of 7–14 consecutive days every 3 months (i.e., four times per year (total study period = 56 days)) considering seasonal differences in lifestyle. We defined valid sensing data as at least 4 days per period and two periods per year, according to a previous study (14). Participants with at least 9 days of measured conversation time per year were included in this study. A total of 855 older individuals (538 women [62.9%]; mean age [standard deviation (SD)]: 73.8 [5.8] years; mean education duration [SD]: 11.8 [2.1] years) met the inclusion criteria and had valid sensing data for research between August 2015 and October 2017. The number of participants with valid conversation data declined during the follow-up period (770 [90.1%] in the second year and 664 [77.7%] in the third year). Moreover, conversation time or social behavior generally remain habitual without change over only a few years. Therefore, we focused on the cross-sectional association of objective conversation time with social behavior in this study. Trained medical staff collected information regarding demographic characteristics, including age, sex, years of education, engagement in community activity, outings, lesson or class frequency, and contact frequency. The valid data on engagement in community activity, outings, lesson or class frequency, and contact frequency were collected from 656, 785, 771, 764 individuals. Mini-Mental State Examination (MMSE) was used to assess the global cognitive function. This prospective study was conducted in accordance with the Declaration of Helsinki and approved by the Ethics Committee of Oita University Hospital (UMIN000017442). Written informed consent was obtained from all participants before participating in this study.
Wearable sensor data
A novel wristband sensor was developed to measure the duration of a conversation but not its content. The microphone on the sensor continuously captured the sound pressure generated by speech within a 2-m radius from the device at 1-min intervals. The level of sound pressure of the voice at this distance ranged from 55 to 75 dBA. Frequency bands comparable to speech were extracted as sound frames from the surrounding sounds. A conversation is defined as 1 min with four or more speech frames per minute. Although the sound data included speech from nearby people, participation in the conversation was considered necessary for social activity. Therefore, the amount of time spent in conversation was calculated by summing the daily sensing data and averaging them over the total measurement period. We verified the false detection of various sounds, such as noise while clothing, television, working in an office, or commuting, wind, passing vehicles, musical instruments, animals, and appliances. Moreover, the measurement accuracy was evaluated by comparing the sensor data with video observational data on the conversation time in healthy older adults aged 60–80 years. The conversation time measured by the wearable sensor was found to be significantly correlated with that measured via video observation (r = 0.8512; p < 0.0001, Pearson correlation). Detailed methods and results have been discussed in previous reports (16).
Questionnaire on social behavior
In this study, social behavior was constructed from the frequency of outings or contact and cognitive or social activity. Data were collected using self-report questionnaires. The frequency of engagement in community activities was measured as a continuous variable. The frequency of outings, lessons or classes, and contact with friends or relatives was measured on a four-or five-point frequency scale as follows: outing (none, 1–2 days a week, 3–4 days a week, and ≥ 5 days a week), lesson or class (the frequency of taking a lesson or class: none, 1–2 days a month, 1–2 days a week, 3–4 days a week, ≥ 5 days a week), and contact with friends or relatives (none, 1–2 days a month, 1–2 days a week, 3–4 days a week, and ≥ 5 days a week). The score of outings is on a 3-point scale from 0 to 3, whereas that of lessons or classes and contact with friends or relatives is on a 4-point scale from 0 to 4.
Statistical analysis
The association between conversation time and social or cognitive activity was examined as follows. First, the correlation between conversation time and the frequency of engagement in community activities and the score of outings, lessons or class, and contact with friends or relatives was analyzed using Spearman’s rank correlation. Second, a multi-regression analysis was conducted to examine the association between conversation time and the frequency of engagement in community activities and the score of outings, lesson or class frequency, and contact with friends or relatives after adjusting for covariates, including age, sex, and years of education. The IBM SPSS Statistics software package v. 29.0 was used for the statistical analyses. Statistical significance was set at p < 0.05.
Results
Demographic and clinical characteristics of the participants
Table 1 summarizes the demographic characteristics, conversation times, and social behaviors of the participants. The mean (SD) point of the MMSE score was 28.4 (1.9). The mean (SD) conversation time collection duration with the wristband sensor was 31.3 (7.1) days per year (7.8 days on average every 3 months).
Association between conversation time and social behavior
Spearman’s rank correlation results showed that conversation time was positively correlated with the frequency of engagement in community activities and lessons or classes (Table 2; Figure 1; r = 0.217, p < 0.001 and r = 0.168, p < 0.001, respectively). Additionally, conversation time remained significantly associated with the engagement in community activities and lessons or classes after adjusting for several covariates in the multi-regression analysis (Table 3; β = 0.181, 95% CI: 0.107–0.254, p < 0.001 and β = 0.11, 95% CI: 0.04–0.179, p = 0.002, respectively; β = 0.11, 95% CI: 0.04–0.179, p = 0.002 and β = 0.181, 95% CI: 0.107–0.254, p < 0.001, respectively).
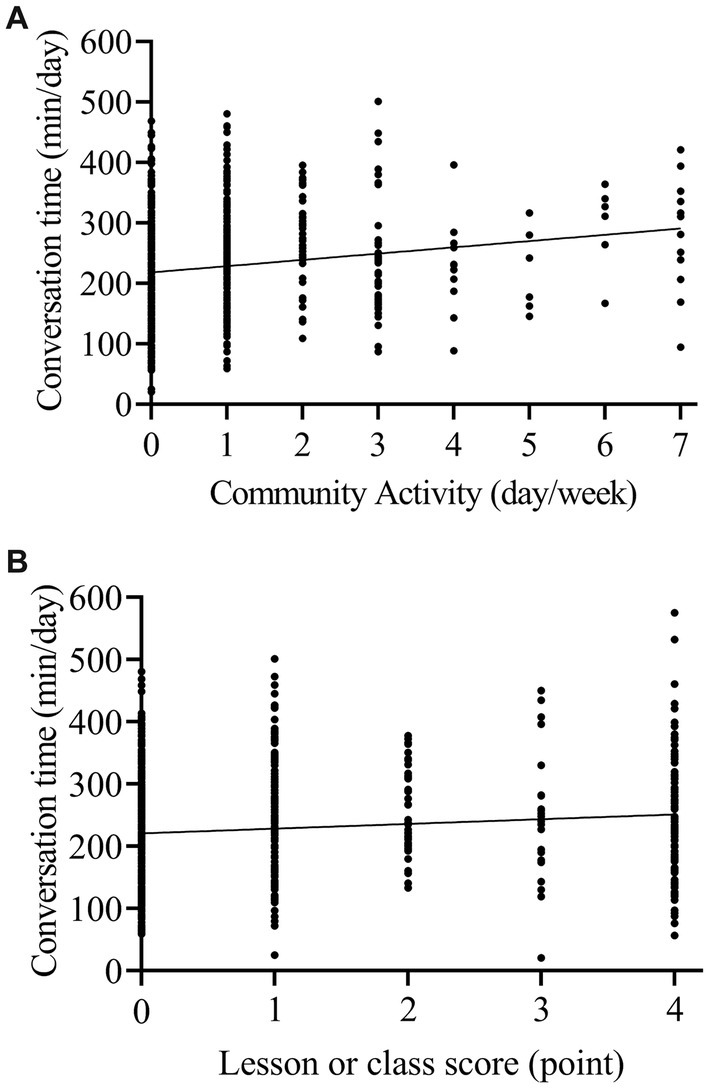
Figure 1. Relationship between objectively measured conversation time and social or cognitive activity. A significant correlation was found between conversation time and the frequency of engagement in community activities (A) and lessons or classes (B).
Discussion
We developed a novel wearable sensor that enables the quantification of conversation time. To the best of our knowledge, this is the first study to examine the association between objectively measured conversation time and self-reported social behavior in older adults living in the community. This study provides valuable insight into the relationship between daily living activities and social isolation. Our results show that decreased conversation time is associated with less frequency of engagement in community activities and lessons or classes. We suggest that objective conversation time may be a valuable marker of social isolation. Moreover, the main advantages of this study include the objective measurement of conversations and the large-scale inclusion of community-dwelling older adults.
A key finding of this study was that objective conversation time is positively associated with the frequency of engagement in community activities and lessons or classes. Furthermore, this association remained significant even after controlling for age, sex, and years of education. However, no significant correlation was found between conversation time and the frequency of outings or contact with friends or relatives. These results suggest that conversation time is associated with engagement in community activities or classes rather than simple outings or social contact. Social isolation is defined as a lack of social contact with family and friends or involvement in social, religious, or other outdoor activities beyond social contact. Social isolation is generally assessed by self-report questionnaires regarding social network size, frequency of social contacts, and engagement in social activities (4, 5, 11). However, there is little consensus on these assessment methods. In this study, we used self-report questionnaires to assess social behavior, such as the frequency of outings, contact, and cognitive or social activity. The increasing number of older adults who live alone and are housebound has led to a higher proportion of them experiencing social isolation. Therefore, quantifying social isolation is essential better to understand the relationship between social isolation and health. Additionally, developing simple methods to quantify the degree of social activity is an important yet challenging task for researchers. Since wearable sensors can remotely collect data on daily life and behavior in real time, they may be used for early detection of social issues, including social isolation (21, 22). Several studies have assessed loneliness or social isolation by analyzing data on household behavior, physical activity, or sleep from smartphones or wearable sensors (23–28). These results show that eating behavior, mobility, and sleep quality are associated with social isolation (23, 24). On the other hand, the association of objectively measured physical activity with social isolation showed inconsistent findings (25–27). Moreover, making and receiving phone calls, social engagement on the web, computer usage, social interaction, smartphone usage, physical activity, or sleep duration are associated with loneliness (28). Therefore, objectively measuring various lifestyles using sensing techniques may be a surrogate parameter for social isolation. Conversation is an essential lifestyle factor for older adults to develop social relationships and preserve brain function, mental health, and quality of life (29, 30). Only three studies have measured conversation time using smartphone data collected in the ambient environment as a conversation and examined the association between conversation time and loneliness (18–20). Two studies found no significant association between conversation time and loneliness in young adults or patients with schizophrenia spectrum disorders (18, 19). In contrast, the remaining research showed a substantial association of conversation time with depression in students (20). Our results showed a significant association of objective conversation time with social behavior. We suggest that objectively measured conversation time using a wearable sensor could be a simple and non-invasive method to assess the social behavior of older adults living in the community. Social isolation is associated with an increased risk of poor health and leads to increased personal and societal costs by increasing the demands for healthcare resources, including the management of acute, chronic, and consumptive illnesses, the use of emergency medical care, and institutionalization (31–33). Therefore, early detection and management of social isolation are crucial for the prevention of various diseases, including dementia, and decreased need for hospital visits, hospitalization, and institutionalization. Social implementation of conversation time measuring technology has the potential to facilitate screening for social isolation among community-dwelling older adults. Additionally, this tool may reduce the demands for human resources, resulting in decreased time or cost burden on individuals, medical staff, or researchers in research or clinical settings.
Several limitations should be noted in this study. First, since the aim of this study is to clarify the association of lifestyle factors with cognitive function, established self-report questionnaires of social isolation, such as the Lubben Social Network Scale (34) and Social Disconnectedness Scale (35), were not included in this study design. Second, we did not consider the effect of family structure on conversation time. Nonetheless, there was no significant association between conversation time and superficial contact with friends or relatives. Third, the accuracy of conversation time detection was verified by comparing the wristband sensor data with video observational data, but television viewing or radio-listening sounds could have been detected as conversation. Fourth, it is important to collect only conversations with good reciprocity and alternation between social actors. However, our sensor technology cannot distinguish between one-way and reciprocal conversations. In this study, we considered that participation in both one-way or reciprocal conversation was necessary for social activity. Further technical developments are required to identify only reciprocal conversations. Fifth, the number of valid data form self-report questionnaire was different for each item due to missing data.
Conclusion
To our knowledge, this is the first study to report the association between objective conversation time and the frequency of engagement in community activities and lessons or classes. Objectively measured conversation time using a wearable sensor could be a valuable parameter for determining social isolation in large cohort studies.
Data availability statement
The raw data used in this study contains sensitive and identifying information on individuals, including sex, age, and education level, that could compromise the privacy of research participants. However, the data that support the findings of this study are available upon ethical approval by the local ethics committee of the Oita University Hospital. Please contact the corresponding author. Email: cmlucmlrZW5reXVAb2l0YS11LmFjLmpw.
Ethics statement
The studies involving humans were approved by the Ethics Committee of Oita University Hospital (UMIN000017442). The studies were conducted in accordance with the local legislation and institutional requirements. The participants provided their written informed consent to participate in this study.
Author contributions
NK: Writing – original draft, Writing – review & editing. TM: Methodology, Writing – review & editing. TA: Formal Analysis, Methodology, Writing – review & editing. EM: Conceptualization, Funding acquisition, Writing – review & editing.
Funding
The author(s) declare that financial support was received for the research, authorship, and/or publication of this article. This research was supported by the Japan Agency for Medical Research and Development (Grant No. 18he140200) and Grant-in-Aid for Scientific Research (C) (Grant No. 22K07474).
Acknowledgments
We gratefully acknowledge Usuki city employees for their assistance in recruiting adults. We thank Suzuki Co., Ltd. for their help with data collection and Fusa Matsuzaki for database construction and data analysis. The authors are sincerely grateful to all the participants who enrolled in this study. The authors would like to thank Editage for the English language review.
Conflict of interest
NK received honoraria from Takeda Pharmaceuticals, Daiichi Sankyo, Eisai, Sumitomo Pharma, PDRadiopharma, and Otsuka Pharmaceutical outside the submitted work.
The remaining authors declare that the research was conducted in the absence of any commercial or financial relationships that could be construed as a potential conflict of interest.
Publisher’s note
All claims expressed in this article are solely those of the authors and do not necessarily represent those of their affiliated organizations, or those of the publisher, the editors and the reviewers. Any product that may be evaluated in this article, or claim that may be made by its manufacturer, is not guaranteed or endorsed by the publisher.
References
1. Courtin, E, and Knapp, M. Social isolation, loneliness and health in old age: a scoping review. Health Soc Care Community. (2017) 25:799–812. doi: 10.1111/hsc.12311
2. Fratiglioni, L, Paillard-Borg, S, and Winblad, B. An active and socially integrated lifestyle in late life might protect against dementia. Lancet Neurol. (2004) 3:343–53. doi: 10.1016/S1474-4422(04)00767-7
3. Cho, JH, Olmstead, R, Choi, H, Carrillo, C, Seeman, TE, and Irwin, MR. Associations of objective versus subjective social isolation with sleep disturbance, depression, and fatigue in community-dwelling older adults. Aging Ment Health. (2019) 23:1130–8. doi: 10.1080/13607863.2018.1481928
4. Gardiner, C, Geldenhuys, G, and Gott, M. Interventions to reduce social isolation and loneliness among older people: an integrative review. Health Soc Care Community. (2018) 26:147–57. doi: 10.1111/hsc.12367
5. Valtorta, N, and Hanratty, B. Loneliness, isolation and the health of older adults: do we need a new research agenda? J R Soc Med. (2012) 105:518–22. doi: 10.1258/jrsm.2012.120128
6. Blazer, D . Social isolation and loneliness in older adults-a mental health/public health challenge. JAMA Psychiatry. (2020) 77:990–1. doi: 10.1001/jamapsychiatry.2020.1054
7. Malcolm, M, Frost, H, and Cowie, J. Loneliness and social isolation causal association with health-related lifestyle risk in older adults: a systematic review and meta-analysis protocol. Syst Rev. (2019) 8:48. doi: 10.1186/s13643-019-0968-x
8. Livingston, G, Huntley, J, Liu, KY, Costafreda, SG, Selbæk, G, Alladi, S, et al. Dementia prevention, intervention, and care: 2024 report of the lancet standing commission. Lancet. (2024) 404:572–628. doi: 10.1016/S0140-6736(24)01296-0
9. Song, S, Stern, Y, and Gu, Y. Modifiable lifestyle factors and cognitive reserve: a systematic review of current evidence. Ageing Res Rev. (2022) 74:101551. doi: 10.1016/j.arr.2021.101551
10. Sommerlad, A, Kivimäki, M, Larson, EB, Röhr, S, Shirai, K, Singh-Manoux, A, et al. Social participation and risk of developing dementia. Nat Aging. (2023) 3:532–45. doi: 10.1038/s43587-023-00387-0
11. Kobayashi, LC, and Steptoe, A. Social isolation, loneliness, and health behaviors at older ages: longitudinal cohort study. Ann Behav Med. (2018) 52:582–93. doi: 10.1093/abm/kax033
12. Buchman, AS, Boyle, PA, Yu, L, Shah, RC, Wilson, RS, and Bennett, DA. Total daily physical activity and the risk of AD and cognitive decline in older adults. Neurology. (2012) 78:1323–9. doi: 10.1212/WNL.0b013e3182535d35
13. Falck, RS, Landry, GJ, Best, JR, Davis, JC, Chiu, BK, and Liu-Ambrose, T. Cross-sectional relationships of physical activity and sedentary behavior with cognitive function in older adults with probable mild cognitive impairment. Phys Ther. (2017) 97:975–84. doi: 10.1093/ptj/pzx074
14. Barnes, DE, Blackwell, T, Stone, KL, Goldman, SE, Hillier, T, Yaffe, K, et al. Cognition in older women: the importance of daytime movement. J Am Geriatr Soc. (2008) 56:1658–64. doi: 10.1111/j.1532-5415.2008.01841.x
15. Diem, SJ, Blackwel, TL, Stone, KL, Yaffe, K, Tranah, G, Cauley, JA, et al. Measures of sleep-wake patterns and risk of mild cognitive impairment or dementia in older women. Am J Geriatr Psychiatry. (2016) 24:248–58. doi: 10.1016/j.jagp.2015.12.002
16. Kimura, N, Aso, Y, Yabuuchi, K, Ishibashi, M, Hori, D, Sasaki, Y, et al. Modifiable lifestyle factors and cognitive function in older people: a cross-sectional observational study. Front Neurol. (2019) 10:401. doi: 10.3389/fneur.2019.00401
17. Kimura, N, Aso, Y, Yabuuchi, K, Ishibashi, M, Hori, D, Sasaki, Y, et al. Association of modifiable lifestyle factors with cortical amyloid burden and cerebral glucose metabolism in older adults with mild cognitive impairment. JAMA Netw Open. (2020) 3:e205719. doi: 10.1001/jamanetworkopen.2020.5719
18. Ben-Zeev, D, Scherer, EA, Wang, R, Xie, H, and Campbell, AT. Next-generation psychiatric assessment: using smartphone sensors to monitor behavior and mental health. Psychiatr Rehabil J. (2015) 38:218–26. doi: 10.1037/prj0000130
19. Fulford, D, Mote, J, Gonzalez, R, Abplanalp, S, Zhang, Y, Luckenbaugh, J, et al. Smartphone sensing of social interactions in people with and without schizophrenia. J Psychiatr Res. (2021) 137:613–20. doi: 10.1016/j.jpsychires.2020.11.002
20. Wang, R, Chen, F, Chen, Z, Li, T, Harari, G, Tignor, S, et al. StudentLife: assessing mental health, academic performance and behavioral trends of college students using smartphones. UbiComp '14: Proceedings of the 2014 ACM International Joint Conference on Pervasive and Ubiquitous Computing (2014), 3–14.
21. Austin, J, Dodge, HH, Riley, T, Jacobs, PG, Thielke, S, and Kaye, J. A smart-home system to unobtrusively and continuously assess loneliness in older adults. IEEE J Transl Eng Health Med. (2016) 4:2800311. doi: 10.1109/JTEHM.2016.2579638
22. Khan, SS, Gu, T, Spinelli, L, and Wang, RH. Sensor-based assessment of social isolation in community-dwelling older adults: a scoping review. Biomed Eng Online. (2023) 22:18. doi: 10.1186/s12938-023-01080-4
23. Benson, JA, McSorley, VE, Hawkley, LC, and Lauderdale, DS. Associations of loneliness and social isolation with actigraph and self-reported sleep quality in a national sample of older adults. Sleep. (2021) 44:zsaa140. doi: 10.1093/sleep/zsaa140
24. Bouaziz, G, Brulin, D, and Campo, E. Technological solutions for social isolation monitoring of the elderly: a survey of selected projects from academia and industry. Sensors. (2022) 22:8802. doi: 10.3390/s22228802
25. Schrempft, S, Jackowska, M, Hamer, M, and Steptoe, A. Associations between social isolation, loneliness, and objective physical activity in older men and women. BMC Public Health. (2019) 19:74. doi: 10.1186/s12889-019-6424-y
26. Herbolsheimer, F, Ungar, N, and Peter, R. Why is social isolation among older adults associated with depressive symptoms? The mediating role of out-of-home physical activity. Int J Behav Med. (2018) 25:649–57. doi: 10.1007/s12529-018-9752-x
27. de Koning, J, Richards, S, and Stathi, A. Loneliness, social isolation, and objectively measured physical activity in rural-living older adults. J Aging Phys Act. (2019) 28:467–77. doi: 10.1123/japa.2019-0027
28. Qirtas, MM, Zafeiridi, E, Pesch, D, and White, EB. Loneliness and social isolation detection using passive sensing techniques: scoping review. JMIR Mhealth Uhealth. (2022) 10:e34638. doi: 10.2196/34638
29. Fukaya, Y, Kawaguchi, M, and Kitamura, T. Does everyday conversation contribute to cognitive functioning? A comparison of brain activity during task-oriented and life-worldly communication using near-infrared spectroscopy. Gerontol Geriatr Med. (2020) 6:2333721420980309. doi: 10.1177/2333721420980309
30. Dodge, HH, Zhu, J, Mattek, N, Bowman, M, Ybarra, O, Wild, K, et al. Web-enabled conversational interactions as a means to improve cognitive functions: results of a 6-week randomized controlled trial. Alzheimers Dement. (2015) 1:1–12. doi: 10.1016/j.trci.2015.01.001
31. Longman, J, Passey, M, Singer, J, and Morgan, G. The role of social isolation in frequent and/or avoidable hospitalisation: rural community-based service providers' perspectives. Aust Health Rev. (2013) 37:223–31. doi: 10.1071/AH12152
32. Sirois, FM, and Owens, J. A meta-analysis of loneliness and use of primary health care. Health Psychol Rev. (2023) 17:193–210. doi: 10.1080/17437199.2021.1986417
33. Prabhu, D, Kholghi, M, Sandhu, M, Lu, W, Packer, K, Higgins, L, et al. Sensor-based assessment of social isolation and loneliness in older adults: a survey. Sensors. (2022) 22:9944. doi: 10.3390/s22249944
34. Lubben, JE . Assessing social networks among elderly populations. Family Community Health. (1988) 11:42–52. doi: 10.1097/00003727-198811000-00008
Keywords: cohort study, community-dwelling older adults, conversation time, social behavior, wearable sensor
Citation: Kimura N, Masuda T, Ataka T and Matsubara E (2024) Relationship between objectively measured conversation time and social behavior in community-dwelling older adults. Front. Neurol. 15:1479296. doi: 10.3389/fneur.2024.1479296
Edited by:
Paolo Taurisano, University of Bari Aldo Moro, ItalyReviewed by:
Maria Arioli, University of Bergamo, ItalyWaleska Berrios, Hospital Italiano de Buenos AIres, Argentina
Copyright © 2024 Kimura, Masuda, Ataka and Matsubara. This is an open-access article distributed under the terms of the Creative Commons Attribution License (CC BY). The use, distribution or reproduction in other forums is permitted, provided the original author(s) and the copyright owner(s) are credited and that the original publication in this journal is cited, in accordance with accepted academic practice. No use, distribution or reproduction is permitted which does not comply with these terms.
*Correspondence: Noriyuki Kimura, bm9yaXl1a2lAb2l0YS11LmFjLmpw