- 1Department of Rehabilitation Medicine, Ruijin Hospital, School of Medicine, Shanghai Jiao Tong University, Shanghai, China
- 2Department of Rehabilitation Medicine, Ruijin Rehabilitation Hospital, Shanghai, China
- 3School of Medicine, Shanghai Jiao Tong University, Shanghai, China
Objective: To compare the effects of robot-assisted therapy with conventional therapy for accelerating stratified intervention in poststroke patients with upper limb dysfunction.
Background: For stroke survivors, recovery of upper extremity function remains a major challenge in rehabilitation. Literature has suggested that the rate of recovery may improve if treatments can be individualized to their clinical profiles. However, there still lack clinical evidence on how to create treatment tailored to individual patients. Robot-assisted Therapy (RT) provides a straightforward approach to adjustment of the assistance-resistance continuum for individual patients. In early Brunnstrom stages of recovery, patients benefit from assistance training, whereas in later stages the training is favored with resistance. Therefore, RT may enhance Conventional Therapy (CT) but the use of RT in stratified intervention has not been investigated. This study evaluated the possible benefit of adopting RT following a protocol of upper-limb training, which was stratified with the Brunnstrom stage of each individual.
Methods: This study was a single-blinded randomized controlled trial. A total of 53 patients with stroke were recruited and randomized into 2 groups (CT, n = 27, 3 dropped out and RT, n = 26, 2 dropped out). Both groups were trained once per day, 5 days per week for 4 weeks. The CT group received 30 min of conventional therapy; the RT group received 30 min of upper limb robot-assisted training. Patients were assessed at the beginning, week-2, and week-4 of the treatment. The outcome measures included the Fugl-Meyer Assessment Upper-Extremity (FMA-UE) and the Modified Barthel Index (MBI).
Results: Across the 4-week intervention, participants in the RT group recovered 1.979 points of FMA-UE per week, compared to 1.198 points per week in the CT group (t94 = 3.333, p < 0.01); the recovery rate was 0.781 points/week higher in the RT group than in the CT group. Moreover, the recovery of FMA-UE was faster in proximal joints (t94 = 3.199, p < 0.01), and for patients in Brunnstrom Stage III (t34 = 2.526, p < 0.05). The improvements in MBI were not significantly different between RT and CT.
Conclusion: Robot-assisted therapy showed initial evidence for the acceleration of post-stroke recovery of motor function in the upper limb. Initial observations suggested that patients in Brunnstrom recovery stage III might benefit the most from the stratified intervention assisted by robotics.
Clinical trial registration: https://www.chictr.org.cn/showproj.html?proj=61834, Identifier [ChiCTR2000039010]. Registered 13 March 2020.
1 Introduction
Upper limb motor impairment is present in about 85% of stroke survivors (1). However, 30–60% of the cases may still show deficits in motor function after 6 months from the onset (2). Mounting evidence supports that motor recovery in the upper extremities is attainable using rehabilitation regimens, such as constraint-induced movement therapy, non-invasive brain stimulation, mental imagery, and bilateral arm training (3). However, one major challenge for upper-limb rehabilitation is that patients can be highly heterogeneous in the causes, locations, timing of stroke, etc. (4–6). As a result, clinical efficacy may be sub-optimal if the treatments fail to be tailored to the clinical profile of each patient (7).
One approach toward individualized treatment is stratified intervention, which subcategorizes patients into groups to apply group-specific treatments (8). A common indicator for stratification in stroke rehabilitation is the score of the Fugl-Meyer Assessment (9), which is a stroke-specific, performance-based impairment index. It is designed to assess motor functioning, balance, sensation, and joint functioning in patients with post-stroke hemiplegia (10). For example, in a study that gave 12-week training to patients with chronic stroke (11), those with moderate upper limb impairment (FMA-UE ≥ 26) gained 5.66 more points in wrist and hand compared with the other group (FMA-UE < 26). The advantage of using FMA as an indicator for stratification is that it contains standardized information about motor performance (10, 12). However, it is a challenge to associate a total score of FMA with a specific goal of post-stroke motor recovery (13). Alternatively, Brunnstrom Recovery Stages (BRS) provide a concise description of the key motor problems poststroke (14). Sum scores of the BRS could quickly provide an overall impression of a patient’s motor function as an alternative to inspecting the score of every joint. Moreover, sum scores could be an outcome indicator because any progress made on each item by a patient could be detected, which is useful for monitoring a patient’s overall change over time and determining the effects of intervention (15). Patients at Brunnstrom stages II, III, and IV (upper-limb) may all benefit from movement training (16–18), but in early stages, the movement needs substantial assistance (19), whereas in later stages the training is favored with resistance (20). For a heterogeneous collection of patients with stroke, therefore, their motor recovery may improve if the treatments can be stratified according to BRS (21).
Stratified intervention imposes new challenges on conventional therapies. On the one hand, differentiation in treatment plans must be rigorously followed across subgroups; on the other, within a subgroup, the treatment should be sufficiently consistent and repeatable. Robot-assisted therapy (RT) has the potential to facilitate stratified intervention, because of the high intensity, good repeatability, and task specificity provided by robotics (22–24). Given that the training may alter from assistive to resistive according to BRS, these requirements are straightforward to administer and regulate using programmable robots, which may enhance the eventual clinical outcome.
Previous studies have explored the key factors of robotic-assisted therapy for clinical efficacy, including the intensity (22), duration (23), and content of training (25). Data from several studies suggest that the recovery rate may improve if treatments can be individualized to their clinical profiles. A much-debated question is how to achieve functional progress according to individual heterogeneity, which may be the reason for the non-significant difference between groups in the RATULS (Robot-assisted training for the upper limb after stroke) study (26). Cases were limited, however, that incorporated robot-assisted therapy in stratified intervention. In a recent clinical study that stratified patients according to their FMA-UE, a combination of shoulder-elbow and wrist-hand robots was used to train chronic stroke patients, but the recovery was not significantly better in the robot-assisted group (11). In this study, we investigated whether upper-limb motor recovery could be accelerated by Robot-assisted Therapy, given that patients were subcategorized according to BRS. In the Robot-assisted Therapy (RT) group, participants performed upper-limb reaching movements using a robot with a group-specific setup of force; in the Conventional Therapy (CT) group, subjects accomplished comparable training under the guidance of an occupational therapist. We hypothesized that the recovery of motor functions in the upper extremities would be faster with RT. Results from this study may warrant larger-scale clinical trials for the evaluation of robot-assisted therapy for individualized treatments.
2 Methods
2.1 Participants
Patients were recruited between April 2020 and January 2021 from the in-patient rehabilitation center of Ruijin Hospital, School of Medicine, Shanghai Jiao Tong University, Shanghai, China. The data were also collected from the same in-patient rehabilitation center.
The inclusion criteria were:
1. Ischemic or hemorrhagic stroke confirmed by CT or MRI;
2. Age ranging from 18 to 80 years;
3. First onset of stroke within 1 ~ 12 months from recruitment;
4. Brunnstrom Recovery Stages (upper-limb) between 2 and 4;
5. Mini-Mental State Examination score > 15 and the ability to cooperate.
The exclusion criteria were:
1. Unstable medical condition;
2. Severe cognitive dysfunction;
3. Severe pain in the upper limb affecting the training;
4. Severe cardiac and pulmonary diseases;
5. Visual impairment;
6. Participation in other research involving robotic-assisted therapy.
All participants gave written informed consent before recruitment.
2.2 Study design and randomization
The study was approved by the Ethics Committee of Ruijin Hospital, School of Medicine, Shanghai Jiao Tong University. The trial was registered at the Chinese Clinical Trial Registry (ChiCTR2000039010, https://www.chictr.org.cn/showproj.html?proj=61834). The protocol was a single-blinded randomized controlled trial. We followed the CONSORT (Consolidated Standards of Reporting Trials) (27) statement and Figure 1 provides the flow diagram of patient screening, randomization, assessment, and intervention. All assessments were performed by an assessor who was blinded for group allocation. Participants were stratified by baseline Brunnstrom recovery stages (BRS II, III, or IV) and allocated to the RT group and CT group using block randomization with a size of 4, by a computer routine. Randomization was performed and documented by a researcher who was not involved in the intervention process. The allocation sequence was concealed from the researcher enrolling and assessing participants in sequentially numbered, opaque, sealed, and stapled envelopes. Therefore, the assessor was ignorant about the group to which the patient belonged. Each outcome measures were blinded to the assessor, therapists, and researchers until the final analysis. It took each participant around 4 weeks to accomplish the protocol (see Figure 2).
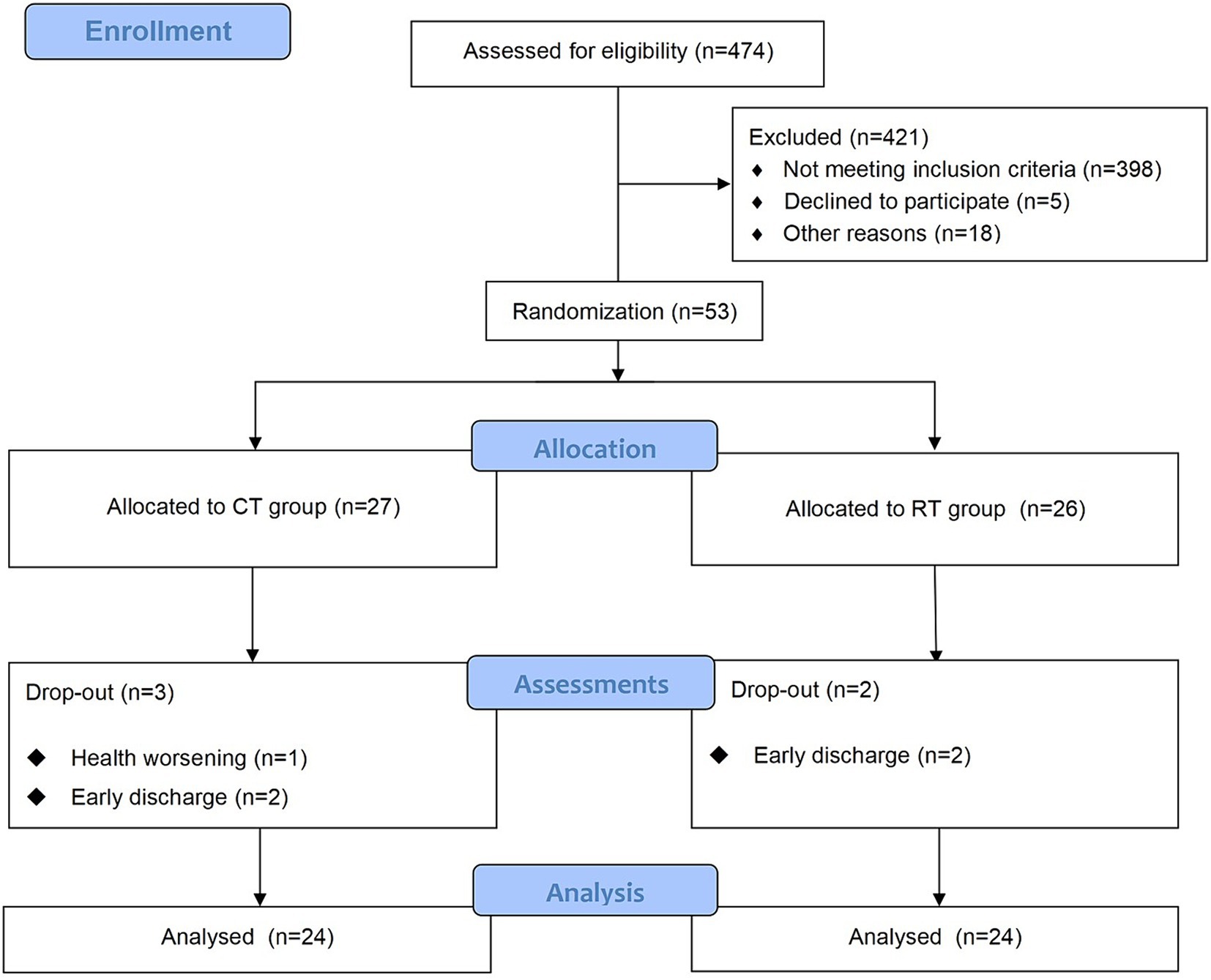
Figure 1. The CONSORT flow diagram of patient screening, randomization, assessment, and intervention.
2.3 Interventions
The CT group received 30 min of conventional occupational therapy focused on upper-limb motor function; the RT group received 30 min of robot-assisted training with details to follow. In addition to the CT/RT interventions involved in this study, all participants also received routine therapies during the 4 weeks (5 days per week). The routine programs focused on motor impairment and recovery to establish deconditioning and fitness after stroke (28). There were several exercises guided by a physiotherapist in the routine therapy: balance training (sitting or standing), walking practice, aerobic exercises, strengthening exercises, and ADL training tailored to individual needs. Patients were required to rest for at least 5 min between exercises. The routine therapies took approximately 150 min each day for all patients. There was a 0.5 to 1-h break between intervention and routine physical therapy, and physicians would ensure patients had regained stamina before each intervention.
2.3.1 Conventional therapy
The CT involved 30-min arm activities on a table. During the training, the arm of the patient was held by a certified occupational therapist (Figure 3, left), and this therapist provided support, assistance, or resistance to all patients as required by the protocol:
Passive-dominated:
1. Supporting activities involving shoulder joints;
2. Simple activities involving shoulder and elbow joints;
Assistive-dominated:
1. Independent muscle training of upper extremity;
2. Basic grip training and simple movements;
3. Progressive grip training and simple activities with both hands;
Resistive-dominated:
1. Fine grip and upper-limb coordination activities;
2. Small palm muscle training and finger separation training;
3. Complex upper limb activities.
Correspondence between the types of training and subgroups is shown in Table 1.
2.3.2 Robot-assisted therapy
The RT session lasted 30 min supervised by a therapist (Figure 3, right). A commercial robot (ArmMotus M2, Fourier Intelligence Co. Ltd., Shanghai, China) was used to administer Robot-assisted Therapy. The robot provided a handle as the end-effector for participants to interact with. Participants would experience assistance or resistance through the handle. Before each session, the range of motion and specified amount of force were tested for each participant. During the gamified training session, icons of fruits and vegetables (radius 2 cm) appeared at random positions on the screen, one at a time. Patients were instructed in a sitting position to reach and stop at each icon for 1 s to acquire it. As can be seen, the gamified training session was equivalent to a series of point-to-point reaching movements. Simulated forces were added during the session with 3 different modes:
1. Passive mode: The handle guided patients to perform point-to-point reaching movements with a pre-defined velocity profile. The residual force applied by the patient was counteracted by the handle. Whenever the residual force on the handle exceeded a safety threshold (80 N), the robot would stop moving. The velocity was set to 0.025–0.05 m/s, and the range of motion in each movement was approximately 22 cm*10 cm. Thus the estimated number of repetitions during each RT intervention was around 150 times.
2. Assistive mode: An assistive force was determined for each patient before every session. The patient sat in front of the robot with the affected hand holding the handle, and then the patient was requested to make a 30 cm center-forward movement with a pre-defined assistive force. The initial magnitude of assistive force was set to 32 N, which usually meant accomplishing the task without any voluntary movement. If the patient was able to accomplish the task, then in the next trial the assistive force was reduced by 1 N. Eventually, the suitable magnitude of assistive force was set to the level that the patient was barely able to accomplish the task. Notice that the minimal assistive force was 15 N due to the design of the robot. During the search for a suitable magnitude of assistive force, at least a 1-min break was given between adjacent trials. The number of movement repetitions was around 100–180.
3. Resistive mode: A resistive force was determined for each patient prior to every session. The patient sat in front of the robot with the affected hand holding the handle, and then the patient was also asked to make a 30 cm center-forward movement with a pre-defined resistive force. The initial magnitude of resistive force was set to 1 N. If the patient was able to accomplish the task, then in the next trial the resistive force was increased by 1 N. Eventually, the appropriate magnitude of resistive force was set to the level that the patient was barely able to accomplish the task. Notice that the maximum resistive force was 5 N due to the design of the robot. During the search for a suitable magnitude of resistive force, at least a 1-min break was given between adjacent trials. The number of movement repetitions was also around 100–180.
The ArmMotus M2 robot also required specification of movement velocity prior to each session. Five levels of velocity were available: 0.025, 0.05, 0.075, 0.1, and 0.125 m/s, which represented the enforced average velocity during the passive mode, and recommended average velocity during the assistive and resistive modes. The assignment of force modes and velocity levels for subgroups is shown in Table 1. The scope of movement was measured for each participant prior to each session. The measurement program required the patients to reach as many points as possible, which were evenly scattered over the working space of the ArmMotus M2 robot. By fitting a rectangle encompassing all points that had been reached, the scope of movement could therefore be chosen as either small (22 cm*10 cm), medium (31 cm*17 cm), or large (44ccm*28 cm).
2.4 Outcome measurements
2.4.1 Primary outcome measure
The primary outcome FMA-UE was evaluated every 2 weeks. FMA-UE was chosen due to its prevalence in clinical studies for stroke rehabilitation (29). The FMA-UE examines reflex activity and synergic voluntary movements. The evaluation includes 33 items and could be classified into four subscales: shoulder/elbow, wrist, hand, and coordination/speed. We were interested in both the total score (full points = 66) and the subscales for shoulder/elbow, wrist, and hand (30).
2.4.2 Secondary outcome measure
The secondary outcome Modified Barthel Index was also performed every 2 weeks. The full score of MBI is 100, which assesses 10 aspects of the activity of daily living. Parts of MBI are related to upper limb motor function, including grooming, bathing, feeding, and dressing, with a total score of 30 points (31).
2.5 Statistical analysis
Statistical analyses were performed using R (version 4.1.2). A power analysis was performed using ‘simr’ package in R (32), considering β = 0.2, and α = 0.05. An estimated effect size was set to 0.9 according to the previous studies (33, 34). It was suggested that 21 patients in each group would be sufficient to detect the desired change. Baseline comparisons between groups were conducted by the t-test or Chi-square test. Effects of intervention on outcome measures were fitted using linear mixed-effect models (R library lme4 v1.1–21) as follows:
where was treated as a continuous variable, which represented the number of weeks passed since the beginning of intervention; denoted whether the intervention was CT or RT; the term accounted for subject-specific intercepts due to repeated measures. The represented the interaction between and . p < 0.05 was considered statistically significant. Outcome measures include FMA-UE, subscales of FMA-UE, and MBI.
3 Results
We screened 474 patients and 53 eligible candidates agreed to participate. Using per-protocol analysis (35), a total of 48 participants (24 in the CT group, 24 in the RT group) finished the 4-week intervention, and 5 participants dropped out during the intervention due to health worsening, early discharge, etc. Consultations with a multi-disciplinary team confirmed that none of the dropouts were related to the intervention in this study. According to Table 2, there was no significant difference between BRS, FMA-UE, MBI, and MAS (p > 0.05) in the baseline assessment of the two groups. For more detailed demographic characteristics of each participant, see the Supplementary Material. No adverse events or unintended effects were reported.
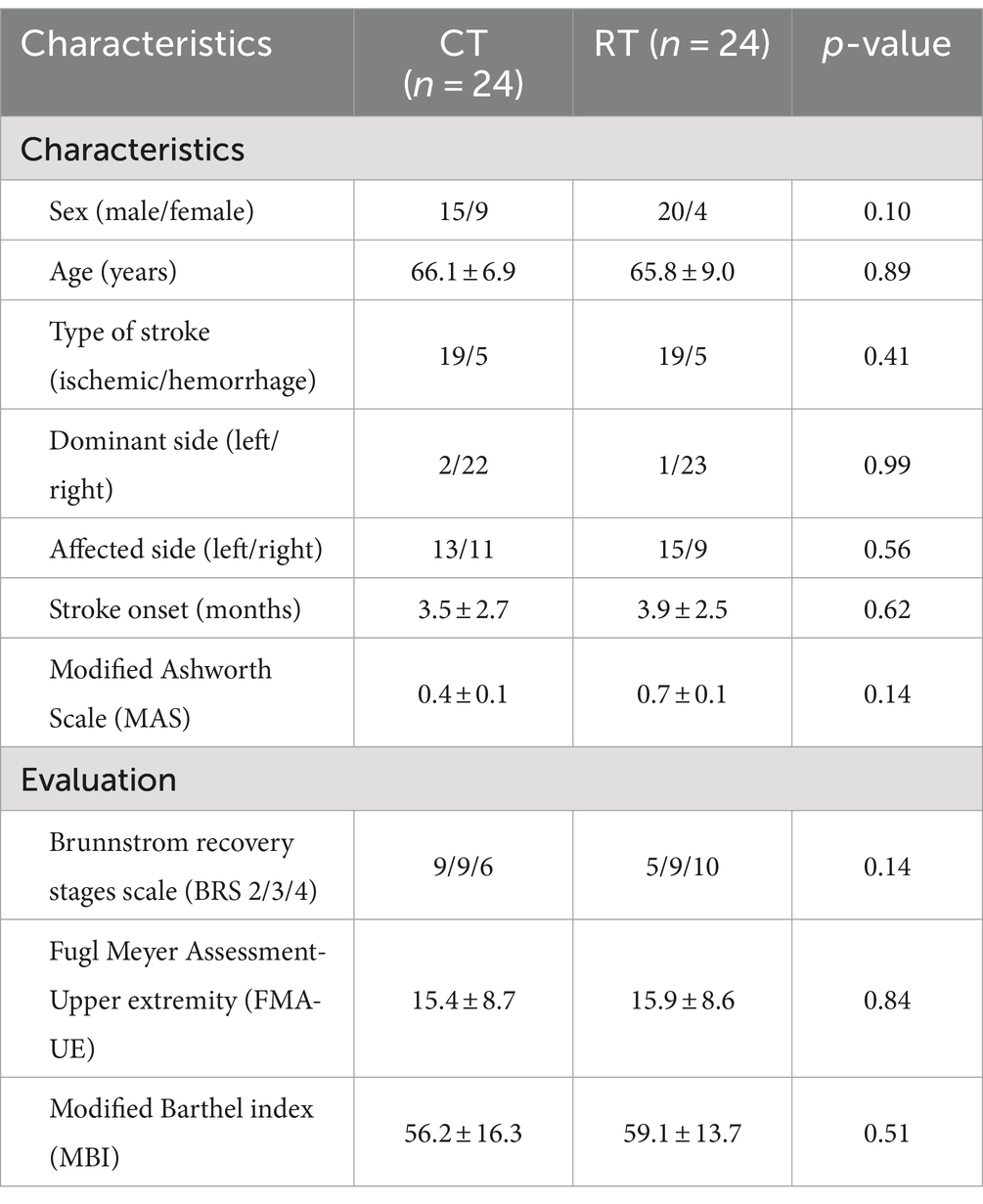
Table 2. Demographic and clinical characteristics at baseline of stroke participants in robot-assisted therapy (RT) and conventional therapy (CT) groups.
3.1 Primary outcome measures
The mixed-effect linear model showed that in the CT group, the FMA-UE score increased by 1.198/week (t94 = 7.228, p < 0.01). The interaction between time and group was also significant (t94 = 3.333, p < 0.01), meaning that in the RT group, the FMA-UE score increased by an additional 0.781 per week (total rate in RT = 1.979/week). Overall, the RT group recovered 65% faster than the CT group. Table 3 presents average scores at each evaluation time point. It is noteworthy that in the RT group, the mean increase in FMA-UE (7.9 ± 4.8, Mean ± SD) exceeded 5.25, the minimal-clinically-important-difference (MCID) as previously reported (36). In contrast, the mean increase in FMA-UE in the CT group (4.8 ± 3.2, Mean ± SD) did not exceed the MCID. A total of 15 out of 24 subjects in the RT group and 11 out of 24 in the CT group improved their FMA-UE over the MCID.
We also found that the RT group recovered faster than the CT group when the shoulder-elbow scores were extracted from the total FMA-UE. In shoulder-elbow subscales (full points = 36), the CT group increased by 0.979/week (t94 = 7.332, p < 0.01), whereas the RT group increased by a total of 1.583 per week (significant interaction, t94 = 3.199, p < 0.01), which was 62% faster than the CT group.
The hand subscale of FMA-UE increased by 0.219/week (t94 = 3.154, p < 0.01) in the CT group, whereas the RT group increased at a higher rate of 0.333/week, but the between-group difference was non-significant.
3.2 Secondary outcome measures
The activity of daily living measured in MBI increased by 2.115/week in the CT group (t94 = 5.436, p < 0.01), but the additional increase in the RT group was not significant. Similar results were found in the MBI upper-limb subscale (full points = 30), which increased by 0.875/week (t94 = 4.197, p < 0.01), and no significant difference was found between the CT and RT groups.
3.3 Subgroup analysis
We analyzed the effect of RT for each Brunnstrom subgroup. In subgroup A (BRS = 2), mixed-effect linear model found significant increase in FMA-UE total score (1.028/week, t26 = 6.144, p < 0.01, see Figure 4A), shoulder-elbow subscale (0.944/week, t26 = 5.543, p < 0.01, see Figure 5A), MBI (1.722/week, t26 = 2.918, p < 0.01) under CT, but no significant difference in between-group interaction was found in these outcomes, meaning that the RT did not incur faster motor recovery than did the CT for subgroup A.
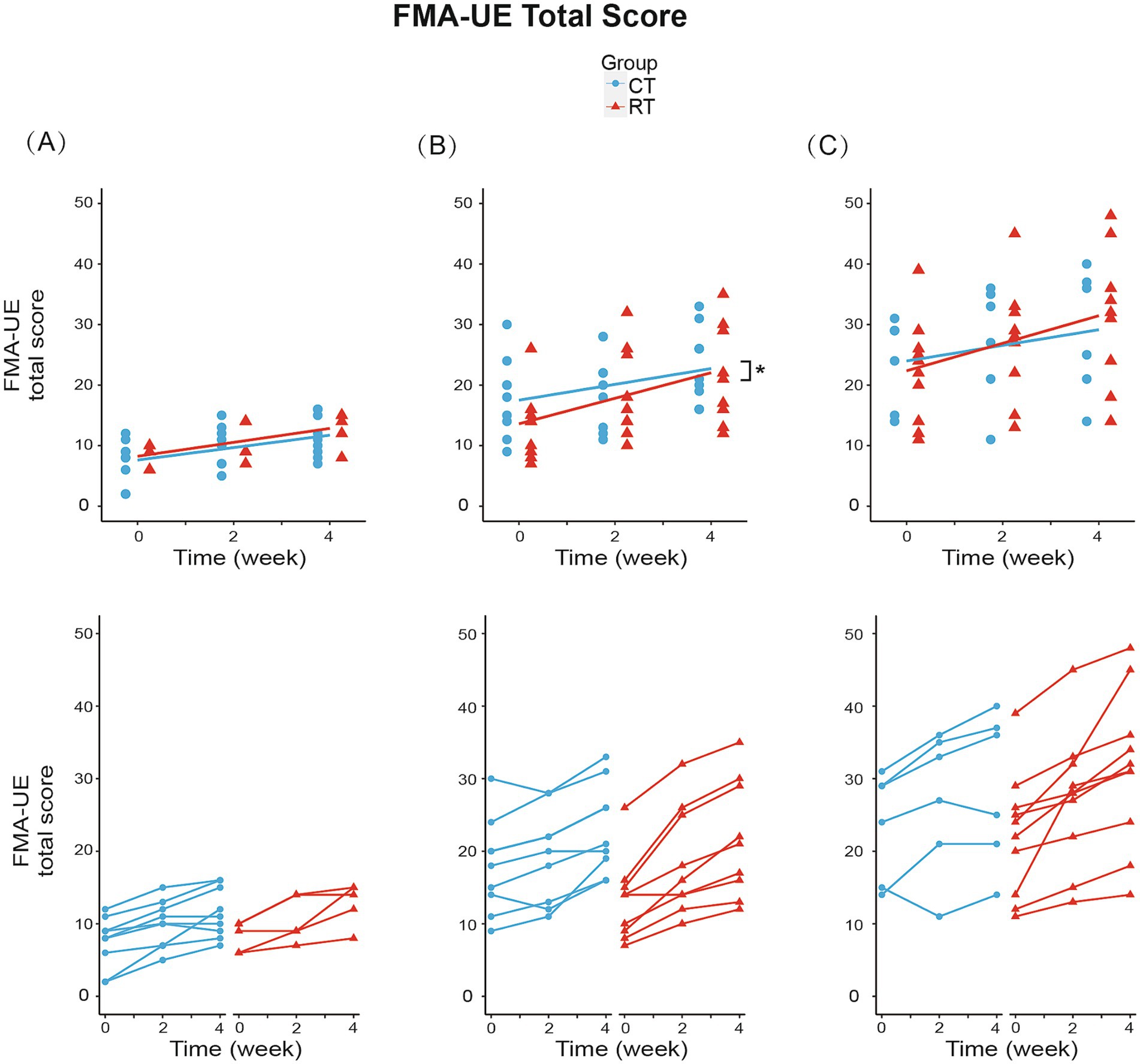
Figure 4. Change from baseline in FMA-UE total score in three subgroups. (A) In subgroup A (BRS = 2): RT group increased 1.150/week (p < 0.01), while CT group increased 1.028/week (p < 0.01). (B) In subgroup B (BRS = 3): RT group increased 2.111/week (p < 0.01), while CT group increased 1.306/week (p < 0.01). Between-group differences were significant (p < 0.05). (C) In subgroup C (BRS = 4): RT group increased 2.275/week (p < 0.01), while CT group increased 1.292/week (p < 0.01). The lines in the first row represent the arithmetic mean of the score. The lines in the second row represent the trend for the score of each patient in subgroups. *p < 0.05.
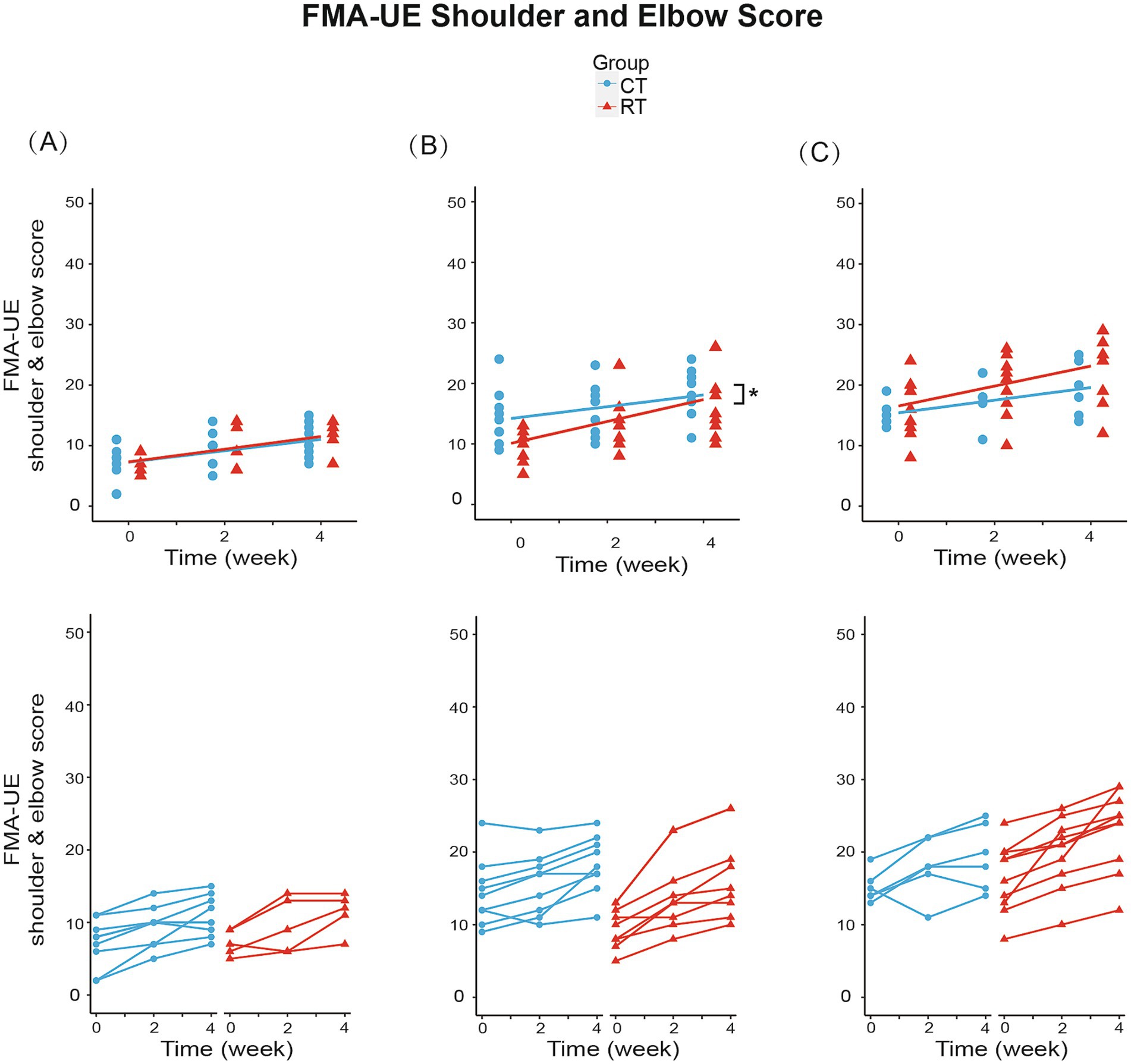
Figure 5. Change from baseline in FMA-UE shoulder and elbow score in three subgroups. (A) In subgroup A (BRS = 2): RT group increased 1.050/week (p < 0.01), while CT group increased 0.944/week (p < 0.01). (B) In subgroup B (BRS = 3): RT group increased 1.806/week (p < 0.01), while CT group increased 0.972/week (p < 0.01). Between-group differences were significant (p < 0.05). (C) In subgroup C (BRS = 4): RT group increased 1.650/week (p < 0.01), while CT group increased 1.042/week (p < 0.01). The lines in the first row represent the arithmetic mean of the score. The lines in the second row represent the trend for the score of each patient in subgroups. *p < 0.05.
In subgroup B (BRS = 3), the FMA-UE score increased by 1.306/week (t34 = 5.327, p < 0.01) under CT, compared to the 61% faster recovery of 2.111/week under RT (significant interaction, Figure 4B). Similarly, the shoulder/elbow FMA increased by 0.972/week (t34 = 4.168, p < 0.01) under CT, compared to an 86% faster recovery of 1.806/week under RT (t34 = 2.526, p < 0.05, see Figure 5B). The hand FMA and MBI scores showed no significant between-group interaction. Taken together, our results indicated that in subgroup B (BRS = 3), faster recovery of motor functions was observed under RT than CT.
In subgroup C (BRS = 4), FMA-UE total score (1.292 per week, t30 = 3.056, p < 0.01, see Figure 4C), shoulder-elbow subscale (1.042 per week, t30 = 3.627, p < 0.01, see Figure 5C), MBI (2.750 per week, t30 = 3.104, p < 0.01) were found significantly increased in the CT group, but the between-group interaction was not significant.
4 Discussion
Through this study, we found that both Robot-assisted Therapy and Conventional Therapy were likely to have a positive effect on post-stroke recovery of motor function. In comparison with conventional therapy, RT showed a higher rate of improvement in FMA-UE. Across all three subgroups, the added benefits of RT seemed the most prominent in patients with moderate motor impairment (BRS = 3). Taken together, our findings supported the hypothesis that even though both RT and CT groups received stratified intervention, the recovery of motor functions in the upper extremities would be faster with RT. Our results were consistent with these well-controlled RCTs, such as the RATULS trial, which demonstrated improvements in upper limb function within groups (26). However, our findings observed significant differences in the FMA scores between groups, which might be due to the stratified intervention or the different robot types. However, with a small sample size, caution must be applied, as our findings might not be extrapolated to all stroke patients.
A probable but noteworthy finding was that RT accelerated the motor recovery in the proximal joints of the upper limb (shoulder and elbow) but not distal ones (wrist and hand). Several possible explanations exist for this finding. Firstly, the participants moved the robot by contracting muscles around the shoulder and elbow, meanwhile, they kept the wrists and fingers strapped to the handle, therefore it could be the shoulder and elbow muscles that underwent the most training. It follows that the training ought to be extended to the wrist and hand, otherwise, it would leave a minimal chance for the wrist and hand to recover. Secondly, motor skills usually transfer from the proximal to the distal segments of the limb (37), thus the shoulder and elbow would lead the wrist and hand to show recovery. Thirdly, competition of adjacent joints in movement recovery suggests that proximal joints often recover better (38). In line with these findings, the end-effector robot employed in this study emphasized larger joint movements while fixing the hand at the terminal handle. In future studies, it might be possible to alter the type of robot to balance proximal and distal joints.
Another possible implication is that among the three subgroups, moderately impaired patients (BRS = 3, Subgroup B) recovered significantly faster when treated with RT compared to CT. In other two subgroups (BRS = 2 and 4) even though the between-group differences in FMA/week were not significant, the slopes were still steeper in RT, meaning that these two subgroups both contributed to the overall trend in pooled analysis. One reason why subgroup B (BRS = 3) outperformed the others was that patients in this stage might enjoy a higher capability of voluntary movement compared to subgroup A (BRS = 2). Therefore, the training may incur more afferent activity (i.e., the training signal) for motor re-learning (39). On the other hand, subgroups with better motor function (subgroup C, BRS = 4) might not be adequately challenged using the existing parameters, which inspires future studies on how much challenge is optimal for using robot-assisted therapy.
One limitation of this study was that it could not differentiate the contribution between assistive and resistive training, which required continuous monitoring of robot-applied force and the reaction from the participant. Note that the reaction might include a wide range of metrics, such as force, trajectory, electromyography, and brain activity. Another interesting but untested factor was whether the participants were compliant with the training, as has been shown critical for clinical outcome (40). Future robot-assistant studies could focus on finding more precise rehabilitation programs, such as the successful detection of voluntary muscle activity onset (41) and the utilization of brain-computer interfaces (BCI) system to achieve coordinated movements (42).
In clinic, our results support the application of robot-assisted therapy for the acceleration of post-stroke recovery in upper-extremity, especially with stratified intervention based on BRS. While this study was an initial, pilot study about the added benefits of robotics in stratified intervention for upper extremities poststroke. The clinical efficacy of our protocol was suggested by this pilot study, but it can only be asserted with larger-scale, multi-center trials.
5 Conclusion
Robot-assisted therapy showed promising clinical evidence in accelerating the poststroke recovery of upper-extremity motor performance following stratified intervention for 4 weeks. The improvements had been identified in general motor behaviors (Fugl-Meyer scores), especially in proximal parts of the upper limb. Among the tested (BRS II, III, and IV), individuals within Brunnstrom recovery stage III might benefit the most from robot-assisted training. However, with a small sample size, these findings cannot be extrapolated to all patients.
Data availability statement
The raw data supporting the conclusions of this article will be made available by the authors, without undue reservation.
Ethics statement
The studies involving humans were approved by the Ethics Committee of Ruijin Hospital, School of Medicine, Shanghai Jiao Tong University. The studies were conducted in accordance with the local legislation and institutional requirements. The participants provided their written informed consent to participate in this study. Written informed consent was obtained from the individual (s) for the publication of any potentially identifiable images or data included in this article.
Author contributions
YL: Data curation, Investigation, Writing – original draft, Writing – review & editing, Conceptualization, Methodology. LC: Formal analysis, Investigation, Project administration, Writing – original draft, Writing – review & editing. JW: Conceptualization, Formal analysis, Supervision, Validation, Writing – review & editing. ZX: Data curation, Formal analysis, Investigation, Project administration, Writing – review & editing. ZC: Data curation, Investigation, Methodology, Writing – review & editing. JY: Formal Analysis, Validation, Writing – review & editing. CN: Formal analysis, Funding acquisition, Project administration, Supervision, Writing – original draft, Writing – review & editing. QX: Funding acquisition, Project administration, Supervision, Validation, Writing – original draft, Writing – review & editing.
Funding
The author(s) declare that financial support was received for the research, authorship, and/or publication of this article. This work has been supported by grants from the National Key R&D Program of China (2022YFC3602704), the National Natural Science Foundation of China (81971722), the Key disciplines construction project of Shanghai municipal (shslczdzk02701), Shanghai Municipal Health Commission (2019SY004), Shanghai Science and Technology Commission Project (19511105600), and Shanghai Jiao Tong University School of Medicine Yuan Shen Rehabilitation Research Institute Center Project (yskf3-23-1107-1).
Acknowledgments
The authors thank Jiaxi Tang for the technical support of Fourier ArmMotus M2 Robot, thank Zeyu Wang for the help in writing the manuscript, and thank Jie Zhang, Yunming Zhang, and Siyang Yu for the help during conventional therapy.
Conflict of interest
The authors declare that the research was conducted in the absence of any commercial or financial relationships that could be construed as a potential conflict of interest.
Publisher’s note
All claims expressed in this article are solely those of the authors and do not necessarily represent those of their affiliated organizations, or those of the publisher, the editors and the reviewers. Any product that may be evaluated in this article, or claim that may be made by its manufacturer, is not guaranteed or endorsed by the publisher.
Supplementary material
The Supplementary material for this article can be found online at: https://www.frontiersin.org/articles/10.3389/fneur.2024.1453508/full#supplementary-material
SUPPLEMENTARY DATA SHEET 1 | The detailed demographic characteristics of participants.
Abbreviations
RT, Robot-assisted therapy; CT, Conventional therapy; FMA-UE, The upper extremity part of Fugl-Meyer assessment; FMA-UE, The upper extremity part of Fugl-Meyer assessment; FMA-UE, The upper extremity part of Fugl-Meyer assessment; MBI, Modified Barthel Index; OT, Occupational therapy; BRS, Brunnstrom Recovery Stage.
References
1. Buma, F, Kwakkel, G, and Ramsey, N. Understanding upper limb recovery after stroke. Restor Neurol Neurosci. (2013) 31:707–22. doi: 10.3233/rnn-130332
2. Langhorne, P, Coupar, F, and Pollock, A. Motor recovery after stroke: a systematic review. Lancet Neurol. (2009) 8:741–54. doi: 10.1016/S1474-4422(09)70150-4
3. Saikaley, M, Pauli, G, Sun, H, Serra, JR, Iruthayarajah, J, and Teasell, R. Network Meta-analysis of non-conventional therapies for improving upper limb motor impairment Poststroke. Stroke. (2022) 53:3717–27. doi: 10.1161/STROKEAHA.122.040687
4. Katsanos, AH, Kamel, H, Healey, JS, and Hart, RG. Stroke prevention in atrial fibrillation: looking forward. Circulation. (2020) 142:2371–88. doi: 10.1161/CIRCULATIONAHA.120.049768
5. Lindsay, MP, Norrving, B, Sacco, RL, Brainin, M, Hacke, W, Martins, S, et al. World stroke organization (WSO): global stroke fact sheet 2019. Int J Stroke. (2019) 14:806–17. doi: 10.1177/1747493019881353
6. Alawieh, A, Zhao, J, and Feng, W. Factors affecting post-stroke motor recovery: implications on neurotherapy after brain injury. Behav Brain Res. (2018) 340:94–101. doi: 10.1016/j.bbr.2016.08.029
7. Boyd, LA, Hayward, KS, Ward, NS, Stinear, CM, Rosso, C, Fisher, RJ, et al. Biomarkers of stroke recovery: consensus-based core recommendations from the stroke recovery and rehabilitation roundtable. Int J Stroke. (2017) 12:480–93. doi: 10.1177/1747493017714176
8. Hara, T, Niimi, M, Yamada, N, Shimamoto, Y, Masuda, G, Hara, H, et al. Prognosis prediction of the effect of botulinum toxin therapy and intensive rehabilitation on the upper arm function in post-stroke patients using hierarchical cluster analysis. Disabil Rehabil. (2021) 44:6815–23. doi: 10.1080/09638288.2021.1977394
9. Rech, KD, Salazar, AP, Marchese, RR, Schifino, G, Cimolin, V, and Pagnussat, AS. Fugl-Meyer assessment scores are related with kinematic measures in people with chronic hemiparesis after stroke. J Stroke Cerebrovasc Dis. (2020) 29:104463. doi: 10.1016/j.jstrokecerebrovasdis.2019.104463
10. Fugl-Meyer, AR, Jääskö, L, Leyman, I, Olsson, S, and Steglind, S. The post-stroke hemiplegic patient. 1. A method for evaluation of physical performance. Scand J Rehabil Med. (1975) 7:13–31.
11. Conroy, SS, Wittenberg, GF, Krebs, HI, Zhan, M, Bever, CT, and Whitall, J. Robot-assisted arm training in chronic stroke: addition of transition-to-task practice. Neurorehabil Neural Repair. (2019) 33:751–61. doi: 10.1177/1545968319862558
12. Gladstone, DJ, Danells, CJ, and Black, SE. The Fugl-Meyer assessment of motor recovery after stroke: a critical review of its measurement properties. Neurorehabil Neural Repair. (2002) 16:232–40. doi: 10.1177/154596802401105171
13. Stinear, CM, Lang, CE, Zeiler, S, and Byblow, WD. Advances and challenges in stroke rehabilitation. Lancet Neurol. (2020) 19:348–60. doi: 10.1016/S1474-4422(19)30415-6
14. Naghdi, S, Ansari, NN, Mansouri, K, and Hasson, S. A neurophysiological and clinical study of Brunnstrom recovery stages in the upper limb following stroke. Brain Inj. (2010) 24:1372–8. doi: 10.3109/02699052.2010.506860
15. Huang, C-Y, Lin, G-H, Huang, Y-J, Song, C-Y, Lee, Y-C, How, M-J, et al. Improving the utility of the Brunnstrom recovery stages in patients with stroke: validation and quantification. Medicine. (2016) 95:e4508. doi: 10.1097/MD.0000000000004508
16. Nishimoto, A, Kawakami, M, Fujiwara, T, Hiramoto, M, Honaga, K, Abe, K, et al. Feasibility of task-specific brain-machine interface training for upper-extremity paralysis in patients with chronic hemiparetic stroke. J Rehabil Med. (2018) 50:52–8. doi: 10.2340/16501977-2275
17. Narayan Arya, K, Verma, R, Garg, RK, Sharma, VP, Agarwal, M, and Aggarwal, GG. Meaningful task-specific training (MTST) for stroke rehabilitation: a randomized controlled trial. Top Stroke Rehabil. (2012) 19:193–211. doi: 10.1310/tsr1903-193
18. Yavuzer, G, Selles, R, Sezer, N, Sütbeyaz, S, Bussmann, JB, Köseoğlu, F, et al. Mirror therapy improves hand function in subacute stroke: a randomized controlled trial. Arch Phys Med Rehabil. (2008) 89:393–8. doi: 10.1016/j.apmr.2007.08.162
19. McLaren, R, Signal, N, Lord, S, Taylor, S, Henderson, J, and Taylor, D. The volume and timing of upper limb movement in acute stroke rehabilitation: still room for improvement. Disabil Rehabil. (2020) 42:3237–42. doi: 10.1080/09638288.2019.1590471
20. Harris, JE, and Eng, JJ. Strength training improves upper-limb function in individuals with stroke: a Meta-analysis. Stroke. (2010) 41:136–40. doi: 10.1161/STROKEAHA.109.567438
21. Saita, K, Morishita, T, Hyakutake, K, Ogata, T, Fukuda, H, Kamada, S, et al. Feasibility of robot-assisted rehabilitation in Poststroke recovery of upper limb function depending on the severity. Neurol Med Chir (Tokyo). (2020) 60:217–22. doi: 10.2176/nmc.oa.2019-0268
22. Kwakkel, G, Kollen, BJ, and Krebs, HI. Effects of robot-assisted therapy on upper limb recovery after stroke: a systematic review. Neurorehabil Neural Repair. (2007) 22:111–21. doi: 10.1177/1545968307305457
23. Bertani, R, Melegari, C, De Cola, MC, Bramanti, A, Bramanti, P, and Calabrò, RS. Effects of robot-assisted upper limb rehabilitation in stroke patients: a systematic review with meta-analysis. Neurol Sci. (2017) 38:1561–9. doi: 10.1007/s10072-017-2995-5
24. Mehrholz, J, Pohl, M, Platz, T, Kugler, J, and Elsner, B. Electromechanical and robot-assisted arm training for improving activities of daily living, arm function, and arm muscle strength after stroke. Cochrane Database Syst Rev. (2018) 9:CD006876. doi: 10.1002/14651858.CD006876.pub5
25. Chien, W, Chong, Y, Tse, M, Chien, C, and Cheng, H. Robot-assisted therapy for upper-limb rehabilitation in subacute stroke patients: a systematic review and meta-analysis. Brain Behav. (2020) 10:e01742. doi: 10.1002/brb3.1742
26. Rodgers, H, Bosomworth, H, Krebs, HI, van Wijck, F, Howel, D, Wilson, N, et al. Robot assisted training for the upper limb after stroke (RATULS): a multicentre randomised controlled trial. Lancet. (2019) 394:51–62. doi: 10.1016/S0140-6736(19)31055-4
27. Boutron, I, Altman, DG, Moher, D, Schulz, KF, and Ravaud, Pfor the CONSORT NPT Group. CONSORT statement for randomized trials of nonpharmacologic treatments: a 2017 update and a CONSORT extension for nonpharmacologic trial abstracts. Ann Intern Med. (2017) 167:40. doi: 10.7326/M17-0046
28. Winstein, CJ, Stein, J, Arena, R, Bates, B, Cherney, LR, Cramer, SC, et al. Guidelines for adult stroke rehabilitation and recovery. Stroke. (2016) 47:98–169. doi: 10.1161/str.0000000000000098
29. Chen, Z, Wang, C, Fan, W, Gu, M, Yasin, G, Xiao, S, et al. Robot-assisted arm training versus therapist-mediated training after stroke: a systematic review and Meta-analysis. J Healthcare Eng. (2020) 2020:1–10. doi: 10.1155/2020/8810867
30. Hernández, E, Galeano, C, Barbosa, N, and Forero, S. Intra-and inter-rater reliability of Fugl-Meyer assessment of upper extremity in stroke. J Rehabil Med. (2019) 51:652–9. doi: 10.2340/16501977-2590
31. Ohura, T, Hase, K, Nakajima, Y, and Nakayama, T. Validity and reliability of a performance evaluation tool based on the modified Barthel index for stroke patients. BMC Med Res Methodol. (2017) 17:131. doi: 10.1186/s12874-017-0409-2
32. Green, P, and Mac Leod, CJ. SIMR: an R package for power analysis of generalized linear mixed models by simulation. Methods Ecol Evol. (2016) 7:493–8. doi: 10.1111/2041-210X.12504
33. Chinembiri, B, Ming, Z, Kai, S, Xiu Fang, Z, and Wei, C. The fourier M2 robotic machine combined with occupational therapy on post-stroke upper limb function and independence-related quality of life: a randomized clinical trial. Top Stroke Rehabil. (2020) 28:1–18. doi: 10.1080/10749357.2020.1755815
34. Lin, C-H, Chou, L-W, Luo, H-J, Tsai, P-Y, Lieu, F-K, Chiang, S-L, et al. Effects of computer-aided Interlimb force coupling training on paretic hand and arm motor control following chronic stroke: a randomized controlled trial. PLoS One. (2015) 10:e0131048. doi: 10.1371/journal.pone.0131048
35. Hernán, MA, and Robins, JM. Per-protocol analyses of pragmatic trials. N Engl J Med. (2017) 377:1391–8. doi: 10.1056/NEJMsm1605385
36. Page, SJ, Fulk, GD, and Boyne, P. Clinically important differences for the upper-extremity Fugl-Meyer scale in people with minimal to moderate impairment due to chronic stroke. Phys Ther. (2012) 92:791–8. doi: 10.2522/ptj.20110009
38. Hu, X-L, Tong, RK, Ho, NSK, Xue, J, Rong, W, and Li, LSW. Wrist rehabilitation assisted by an electromyography-driven neuromuscular electrical stimulation robot after stroke. Neurorehabil Neural Repair. (2015) 29:767–76. doi: 10.1177/1545968314565510
39. van Dijk, L, van der Sluis, C, and Bongers, RM. Reductive and emergent views on motor learning in rehabilitation practice. J Mot Behav. (2016) 49:244–54. doi: 10.1080/00222895.2016.1191418
40. Mastos, M, Miller, K, Eliasson, AC, and Imms, C. Goal-directed training: linking theories of treatment to clinical practice for improved functional activities in daily life. Clin Rehabil. (2007) 21:47–55. doi: 10.1177/0269215506073494
41. Li, L, Wang, X, Yao, B, Zhang, X, and Zhou, P. Sample entropy-based surface Electromyographic examination with a linear electrode Array in survivors with spinal cord injury. IEEE Trans Neural Syst Rehabil Eng. (2023) 31:2944–52. doi: 10.1109/TNSRE.2023.3290607
Keywords: stroke, robotics, upper limb, motor recovery, stratified intervention
Citation: Liu Y, Cui L, Wang J, Xiao Z, Chen Z, Yan J, Niu CM and Xie Q (2024) Robot-assisted therapy in stratified intervention: a randomized controlled trial on poststroke motor recovery. Front. Neurol. 15:1453508. doi: 10.3389/fneur.2024.1453508
Edited by:
Longbin Zhang, Nanyang Technological University, SingaporeReviewed by:
Le Li, Northwestern Polytechnical University, ChinaFayaz Khan, King Abdulaziz University, Saudi Arabia
Gauthier Everard, Laval University, Canada
Copyright © 2024 Liu, Cui, Wang, Xiao, Chen, Yan, Niu and Xie. This is an open-access article distributed under the terms of the Creative Commons Attribution License (CC BY). The use, distribution or reproduction in other forums is permitted, provided the original author(s) and the copyright owner(s) are credited and that the original publication in this journal is cited, in accordance with accepted academic practice. No use, distribution or reproduction is permitted which does not comply with these terms.
*Correspondence: Qing Xie, xq11231@rjh.com.cn; Chuanxin M. Niu, minos.niu@sjtu.edu.cn
†These authors have contributed equally to this work and share first authorship