- 1Department of Neurology and Laboratory of Neuroscience, IRCCS Istituto Auxologico Italiano, Milano, Italy
- 2Neuroradiology Unit, Fondazione IRCCS Ca’ Granda Ospedale Maggiore Policlinico, Milano, Italy
- 3Department of Pathophysiology and Transplantation, "Dino Ferrari" Center, Università degli Studi di Milano, Milano, Italy
- 4Department of Electrical Engineering and Computer Sciences, University of California, Berkeley, Berkeley, CA, United States
- 5Department of Diagnostic Imaging, IRCCS Istituto Auxologico Italiano, Milano, Italy
- 6Department of Oncology and Hemato-Oncology, Università degli Studi di Milano, Milano, Italy
Background: This study aimed to assess whether quantitative susceptibility imaging (QSM)-based measures of iron accumulation in the cerebellum predict cognitive and behavioral features in non-demented amyotrophic lateral sclerosis (ALS) patients.
Methods: A total of ALS patients underwent 3-T MRI and a clinical assessment using the ALS Functional Rating Scale-Revised (ALSFRS-R) and the Edinburgh Cognitive and Behavioural ALS Screen (ECAS). Regression models were applied to each subscale of the cognitive section of the ECAS and the ECAS-Carer Interview to examine the effect of QSM-based measures in white and gray matter (WM; GM) of the cerebellum, separately for right, left, and bilateral cerebellar regions of interest (ROIs). These effects were compared to those of cerebellar volumetrics in WM/GM, right and left hemispheres while controlling for demographics, disease status, and total intracranial volume.
Results: Higher QSM measures of the cerebellar GM on the left, right, and bilateral sides significantly predicted (ps ≤ 0.003) a greater number of errors on the executive functioning (EF) subscale of the ECAS (ECAS-EF). Moreover, higher GM-related, QSM measures of the cerebellum were associated with an increased probability of a below-cut-off performance on the ECAS-EF (ps ≤ 0.024). No significant effects were observed for QSM measures of the cerebellar WM or for volumetric measures on the ECAS-EF. Other ECAS measures showed no significant effects. Bilateral QSM measures of the cerebellar GM also selectively predicted performance on backward digit span and social cognition tasks.
Discussion: Iron accumulation within the cerebellar GM, particularly in the cerebellar cortices, may be associated with executive functioning deficits in non-demented ALS patients. Therefore, QSM-based measures could be useful for identifying the neural correlates of extra-motor cognitive deficits in ALS patients.
1 Background
Among the non-pyramidal abnormalities observed in amyotrophic lateral sclerosis (ALS) (1), cerebellar involvement has recently been proposed as a key contributor to the phenotypic heterogeneity of the disease, possibly due to the cerebellum’s extensive connections with several encephalic and spinal sites (2). It has been suggested that an interplay between primary degenerative processes affecting the cerebellum and its compensatory role for brain/spinal cord dysfunctions may be the pathophysiological mechanism through which cerebellar involvement contributes to the clinical manifestations of ALS (2).
While the association between cerebellar involvement and motor manifestations in ALS has been sufficiently explored (3, 4), less is known on its role towards non-motor, neuropsychological features (2). Despite being poorly understood how cerebellar abnormalities contribute to cognition and behaviour in brain disorders (5), a number of reports would suggest they do account, at least to an extent, for both cognitive (6–8) and behavioural dysfunctions (6, 9, 10) in ALS – likely due to an altered connectivity between cerebellar nuclei and extra-motor networks (11).
While currently available studies on the topic mostly addressed morphological/functional neuroimaging techniques (2), none of them employed quantitative susceptibility mapping (QSM) algorithms (12) – although such an approach has proved promising in delivering a biomarker of subtle brain abnormalities underlying motor signs/symptoms in ALS (13–16). QSM algorithms indeed allow to derive an estimate of iron accumulation in brain tissues based on magnetic resonance imaging (MRI) scans, this being a feature common to several neurodegenerative diseases (17). Relatedly, it has been shown that QSM measures do relate to neuropsychological measures in such conditions (17), having thus been suggested as a promising marker for cognitive decline in several brain disorders (18).
A growing body of evidence has indicated that iron overload may play a causal role in neuronal death, leading to neurodegeneration (19, 20). Thus, QSM analyses might serve as a valuable tool for detecting subtle alterations in brain tissue iron content before regional brain atrophy occurs as an epiphenomenon of neurodegeneration (21), including in conditions such as ALS (16). This is particularly relevant for cerebellar involvement, in ALS, which is often subtle and may go undetected by standard volumetric measurements (8). Within such a framework, it would not be unreasonable to hypothesize that QSM algorithms might enhance the study of the relationships between cerebellar involvement and neuropsychological features in this population.
Given the above premises, the present study aimed to assess whether cerebellar QSM measures, compared to standard volumetric MRI measures, can predict cognitive and behavioral features in non-demented ALS patients.
2 Methods
2.1 Participants
Data from 61 consecutive ALS patients diagnosed according to the El Escorial criteria (22) and referred to IRCCS Istituto Auxologico Italiano between 2016 and 2021 were retrospectively retrieved. Patients with available MRI and neuropsychological data, specifically Edinburgh Cognitive and Behavioural ALS Screen (ECAS) scores (23), were selected for inclusion. No patient met the criteria for any frontotemporal degeneration phenotype (24, 25). The exclusion criteria included (1) the presence of other neurological/psychiatric disorders, (2) severe or uncompensated general medical conditions (i.e., internal/metabolic diseases or organ/system failures), or (3) uncorrected hearing/vision deficits. This study was approved by the Ethical Committee of IRCCS Istituto Auxologico Italiano (I.D.: 23C722_2017). Informed consent was obtained from all participants, and data were treated in accordance with current regulations.
2.2 Materials
2.2.1 Clinical assessment
The ALS Functional Rating Scale-Revised was employed to assess motor-functional outcomes (26). Cognition and behavior assessments were conducted using the cognitive section of the ECAS (23) and the ECAS-Carer Interview (ECAS-CI) (27), respectively. The cognitive section of the ECAS is a performance-based screening tool ranging from 0 to 136, comprising five subscales that assess both ALS-specific cognitive function, including language (ECAS-L; range = 0–28), verbal fluency (ECAS-F; range = 0–24), and executive functioning (ECAS-EF; range = 0–28), as well as ALS-nonspecific cognitive functions, such as memory (ECAS-M; range = 0–24) and visuospatial abilities (ECAS-VS; range = 0–12) (23). The ECAS-CI is a 13-item, Likert-scaled, caregiver-report questionnaire that covers the full range of frontotemporal and dysexecutive behavioral features typical of ALS; higher scores indicate a greater degree of behavioral involvement (27).
2.2.2 MRI acquisition
The patients underwent brain MRI scans using a 3-T SIGNA (General Electric, GE Healthcare Medical Systems, Chicago, Illinois, US) at the Istituto Auxologico Italiano, IRCCS, Milano, Italy. The MR protocol included the following sequences: a whole-brain sagittal three-dimensional FSPGR BRAVO T1-weighted sequence (TR = 8.7 ms, TE = 3.2 ms, inversion time = 450 ms, voxel size = 1 × 1 × 1 mm3, flip angle = 12°, acquisition matrix 256 × 256); a sagittal 3D fluid-attenuated inversion recovery (FLAIR) sequence (TR = 6,000 ms, TE = 108 ms, inversion time = 1,824 ms, voxel size = 1 × 1 × 1.4 mm3, flip angle = 90°, acquisition matrix 224 × 224); an axial T2-weighted fast spin-echo (FSE) sequence (TR = 3,000 ms, TE = 82 ms, pixel size = 0.234 × 0.234 mm2, slice thickness = 2 mm, slice gap = 0.2 mm, flip angle = 111°, acquisition matrix 320 × 320); and three-dimensional spoiled gradient-echo multi-echo (GRE) pulse sequences (TR = 39 ms, TE = 24 ms, delta TE = 3.3 ms, number of echoes = 7, voxel size = 0.468 × 0.468 × 1.4 mm3, flip angle = 20°, acquisition matrix 416 × 320).
Images were assessed by an expert neuroradiologist and were found to be free of motion and severe artifacts on T1-weighted or GRE images.
2.2.3 MRI processing
FreeSurfer was used to automatically segment T1-weighted images (28). The Left-Cerebellum-Cortex (coded “8”) and Right-Cerebellum-Cortex (coded “47”) regions were merged to create the CerebellumGM Region of Interest (ROI). Similarly, the Left-Cerebellum-White-Matter (coded “7”) and Right-Cerebellum-White-Matter (coded “46”) regions were merged to create the CerebellumWM ROI, as shown in Figure 1. The volumes of the CerebellumGM and CerebellumWM ROIs were measured in cubic millimeters (mm3).
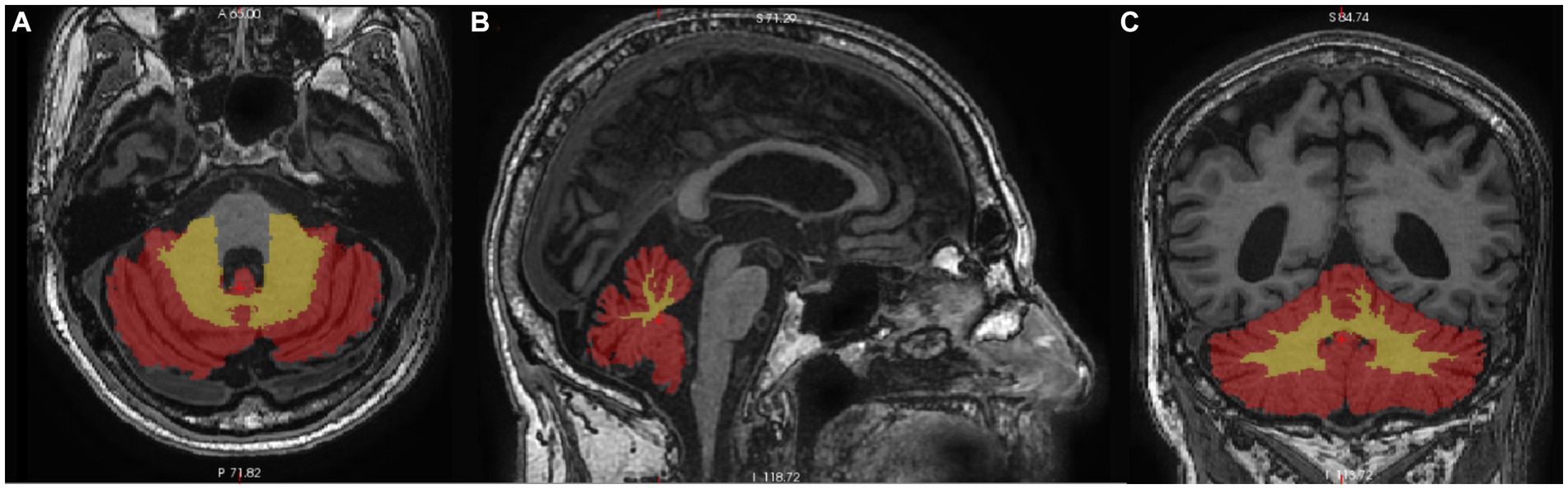
Figure 1. Axial (A), sagittal (B), and coronal (C) view of the cerebellum segmented on T1-weighted images. Cerebellar gray matter is colored red, and cerebellar white matter is yellow.
Whole-brain QSM (quantitative susceptibility mapping) was calculated using STI Suite, a MATLAB toolbox specifically developed at UC Berkeley for MRI phase and magnetic susceptibility mapping (29). First, a single-subject brain mask was generated from the echoes-averaged magnitude image using the FSL Brain Extraction Tool (30). Then, the VSHARP algorithm was applied to remove the background field from phase images using the brain mask, followed by the application of the Streaking Artifacts Reduction (STAR) algorithm (31) to the VSHARP filtered phase images, resulting in subject-level susceptibility maps (32).
The SPM12 MATLAB Toolbox was used to coregister the QSM map to the T1-weighted image. For each patient, a transformation matrix was calculated by aligning the magnitude image to the T1-weighted image, which was then applied to the QSM image. The CerebellumGM and CerebellumWM ROIs overlaid on the coregistered QSM map (Figure 2). MATLAB (The MathWorks, Natick, MA) R2018 was used to extract the mean of the QSM values for the CerebellumGM and CerebellumWM ROIs in the left and right hemispheres separately, as well as for the total bilateral ROIs.
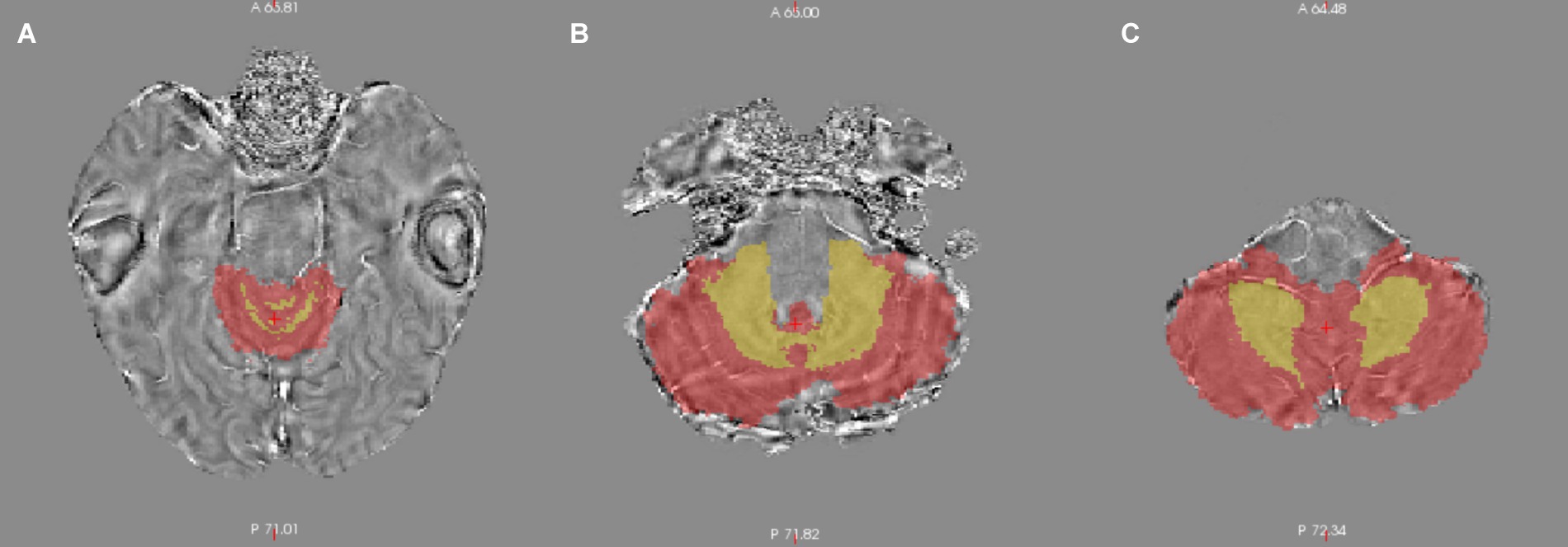
Figure 2. Superior (A), middle (B), and inferior (C) axial slices of the segmented cerebellum overlapped on the quantitative susceptibility map.
The neuroimaging pipeline was adapted from a previous QSM study focused on the precentral cortex (13).
2.3 Statistics
Both cognitive and behavioral measures did not meet the linear model assumptions (i.e., normality and homoscedasticity), as indexed by excessive skewness and values (i.e., ≥ |1| and |3|, respectively) (33), visual abnormalities in Q-Q plots and histograms, and significant Shapiro–Wilk’s tests (ps < 0.001). Specifically, ECAS-L, -F, -EF, -M, and-VS scores were left-skewed (i.e., showed ceiling effects), whereas ECAS-CI scores were right-skewed (i.e., showed floor effects). Additionally, both cognitive and behavioral measures showed overdispersion, meaning that they were highly variable between individuals. To address these issues, predictions of interests were tested using negative binomial (NB) regressions, which are suitable for modeling left-skewed and overdispersed count-like data (34) and have been shown to effectively model ALS patients’ cognitive/behavioral data (27, 35, 36).
For the ECAS-L/-F/-EF/-M/-VS subscales, NB regression was applied by focusing on the number of errors on each subscale as the outcome (37). This approach reversed the skewness of the empirical distribution without altering the original operationalization of the data (i.e., accuracy) (38). Moreover, the NB model was chosen over linear models with normalized and variance-stabilized transformed outcomes, as those transformations would have changed the original measurement units of the outcomes. In contrast, ECAS-CI scores already followed an NB distribution and, thus, did not require any transformation.
NB regressions were conducted for each subscale of the cognitive section of the ECAS and the ECAS-CI to test the effect of QSM-based white and gray matter (WM; GM) cerebellar measures, separately for right, left, and bilateral cerebellar ROIs (resulting in three models for each outcome). These were compared to WM/GM measures and right and left cerebellar volumetrics. Within these models, demographic variables (age, education, and sex), disease duration (in months), ALSFRS-R scores, presence of C9orf72 hexanucleotide repeat expansion, and total intracranial volume were included as covariates.
For those NB regressions that identified significant QSM predictors, a logistic regression (LR) was subsequently performed to assess above versus. Below-cut-off performance on the relevant ECAS measure of interest (23). Poletti et al.’s (23) cut-offs were derived using a 2 SD-based approach and are stratified by age and education level (i.e., ≤60 and > 60 years of age and < 14 and ≥ 14 years of education, respectively). Accordingly, given that ECAS cut-offs are already adjusted for age and education, these covariates were not included in the LR models. Collinearity within the LR models was diagnosed if the variance inflation factor (VFI) exceeded 10 or if the tolerance index was less than 0.1 (39).
Missing data were excluded on a pairwise basis. The significance level was set at α = 0.05, with Bonferroni-corrected applied to target predictors whenever adequate. Analyses were conducted using jamovi 2.5.1
3 Results
Table 1 summarizes the patients’ background, clinical, volumetric, and QSM-based measures.
As shown in Table 2, no significant effects were observed for either QSM-based or volumetric cerebellar measures on ECAS-L, ECAS-F, ECAS-M, ECAS-VS, or ECAS-CI scores. However, higher QSM measures of the cerebellar GM, on both the left and right sides, as well as the total mean QSM measures, were found to selectively predict a higher number of errors on the ECAS-EF subscale (Figure 3). In contrast, neither QSM-based measures of the cerebellar WM nor volumetric cerebellar measures significantly predicted ECAS-EF errors (Table 2). Supplementary Tables 1–6 provide the full results of the NB models that address bilateral QSM-based and volumetric measures.
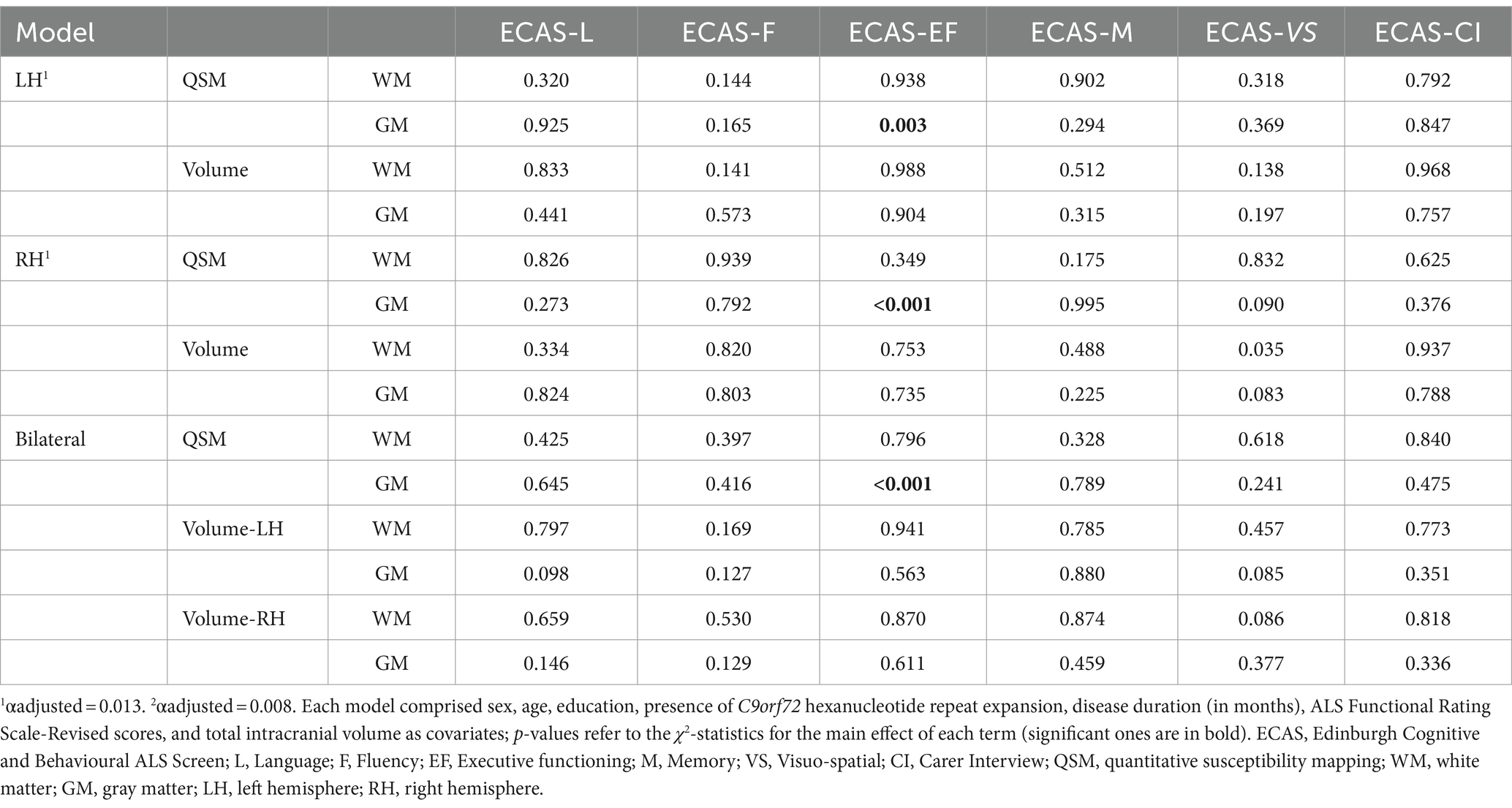
Table 2. The negative binomial regressions yielded the effects of QSM-based and volumetric measures on the number of errors on the cognitive subscales of the ECAS and the ECAS-CI.
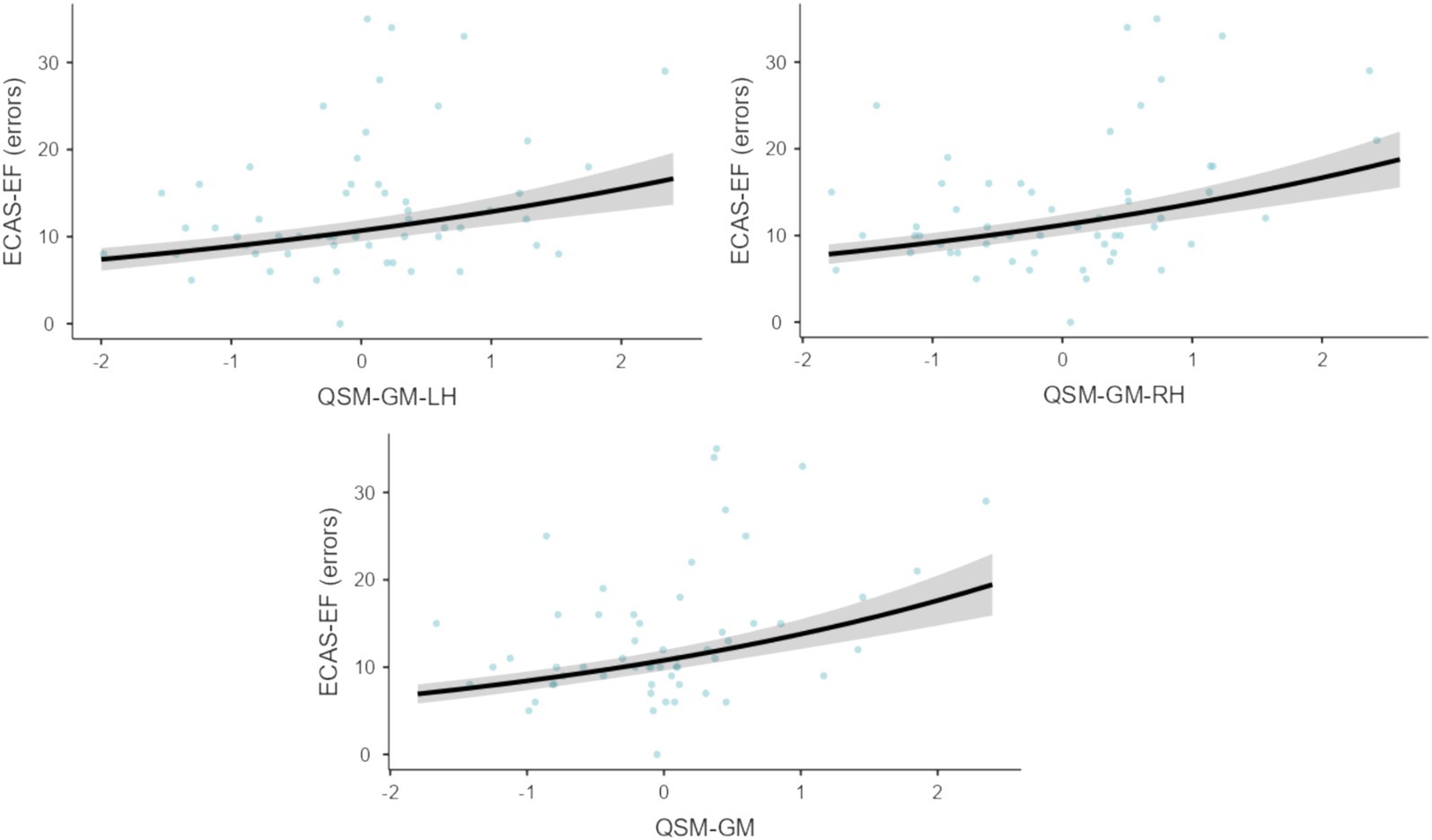
Figure 3. Predictions of left- (upper-left panel) and right-sided (upper-right panel), as well as bilateral (lower panel) QSM-based measures of the cerebellum towards the ECAS-EF. ECAS, Edinburgh Cognitive and Behavioural ALS Screen; EF, Executive functioning; QSM, quantitative susceptibility mapping; WM, white matter; GM, gray matter; LH, left hemisphere; RH, right hemisphere. Gray areas represent the SE of the estimate of the Negative Binomial regression (left-sided: z = 2.97; p = 0.003; right-sided: z = 3.75; p < 0.001; bilateral: z = 3.95; p < 0.001). The measurement unit on the x-axis is ppb; these predictors have been mean-centered for this graphical representation.
LRs conducted on above-vs. below-cut-off ECAS-EF performance confirmed that higher GM-related, QSM-based measures of the cerebellum were associated with a higher likelihood of a below-cut-off ECAS-EF performance (right-sided: b = 3.72, z = 2.41, p = 0.016; left-sided: b = 1.85, z = 2.36, p = 0.018; total: b = 4.36, z = 2.06, p = 0.034). In contrast, neither QSM-based measures of the cerebellar WM nor volumetric measures have any significant effects (ps ≥ 0.538 and ps ≥ 0.197, respectively). No collinearity issues were detected in the models addressing right-and left-sided measures; however, collinearity was observed between right-and left-sided GM and WM volumetric measures (VFI ≥ 14.8; tolerance index≤0.07) in the model addressing total mean QSM measures. Supplementary Table 7 shows the full results of the LR model addressing bilateral QSM-based and volumetric measures.
Finally, an explanatory, data-driven set of regression models was conducted to test which specific ECAS-EF tasks (i.e., backward digit span, alternation, sentence completion, and social cognition) could be predicted by QSM measures. To this end, each ECAS-EF task was regressed using the set of predictors and covariates used in the abovementioned NB models addressing bilateral cerebellar ROIs. However, models including only right or left cerebellar measures were not conducted, as no laterality effects were used in the analyses addressing the ECAS-EF as a whole. Specifically, linear regressions were used for backward digit span and sentence completion scores due to their normal distribution, while NB regressions were used for alternation and social cognition tasks, with the number of errors as the outcome variable.
Table 3 presents the results of these off-label analyses. Overall, only backward digit span (b = −0.74; z = −2.69; p = 0.010) and social cognition performances (b = 0.90, z = 2.89, p = 0.004) were significantly predicted by QSM measures of the cerebellar GM. No significant effects were found for QSM measures of the cerebellar WM or volumetric measures. Supplementary Tables 8–11 provide the full results of the NB models addressing bilateral QSM-based and volumetric measures.
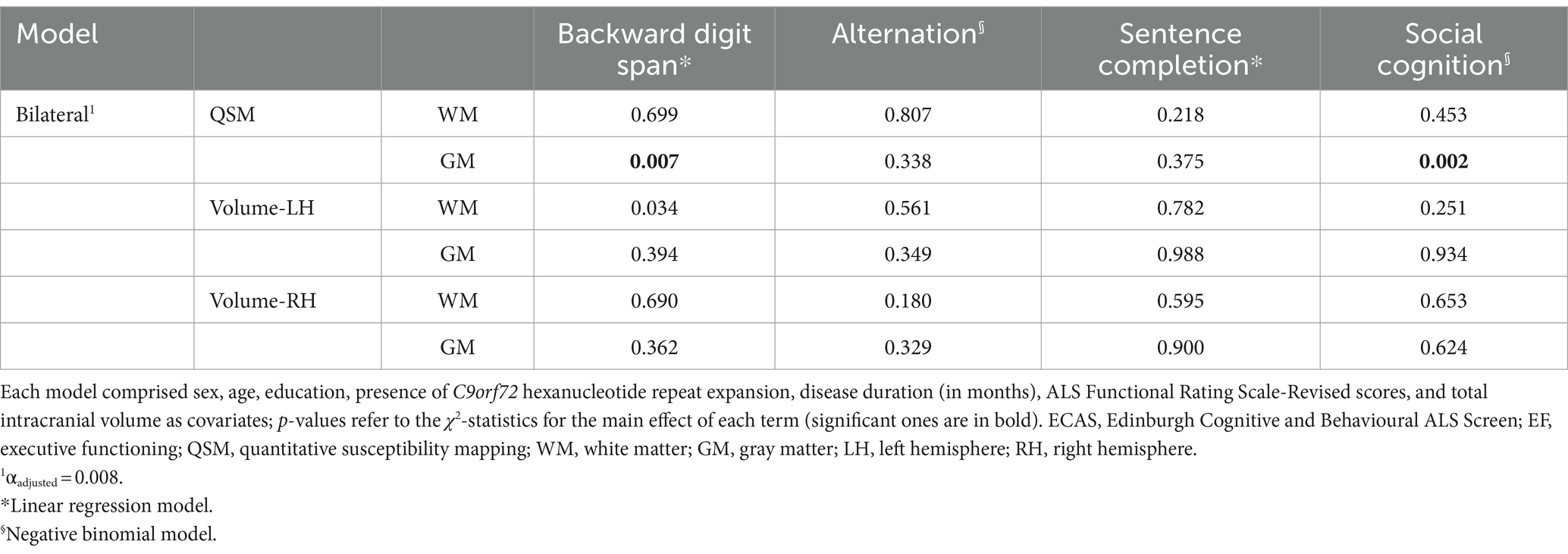
Table 3. The effects of QSM-based and volumetric measures on each task of the ECAS-EF were yielded by linear and negative binomial regressions.
4 Discussion
The present study provides, for the first time, promising evidence of the predictive capability of QSM-detected iron accumulation within the cerebellar GM in relation to executive functioning (EF) deficits in non-demented ALS patients.
Notably, this predictive capability (1) was not observed in QSM-based measures and (2) did not apply to common volumetrics of the cerebellum. Such selectivity of QSM-based measures, as opposed to macroscopic volumetric ones, aligns with the idea that tissue alteration, such as iron overload, may precede brain atrophy (19, 20).
However, it is somewhat surprising that these findings were significant for cerebellar GM regions of interest (ROIs) but not for WM ROIs, which, in this study using Freesurfer segmentation, included the cerebellar nuclei. This is unexpected, given that both cerebellar nuclei and cortical-cerebellar connections have frequently been shown to underlie the clinical phenotype of ALS (2), rather than the cerebellar cortex. This raises the possibility that, in non-demented ALS patients, the cerebellar cortices also play a role in their cognitive profiles. Given the novelty of these results, further research is needed to determine whether the cerebellar cortex is selectively involved in characterizing the cognitive features of non-demented ALS patients.
In the present study, QSM-based measures of cerebellar GM selectively predicted EF measures but did not predict other cognitive functions or behaviors. This finding aligns with the current knowledge of the cerebellum’s contributions to cognition in both healthy and brain-damaged individuals, where the cerebellum is known to be substantially involved in EF (40, 41). However, previous studies on neurodegenerative conditions other than ALS have not detected a link between cognitive features and QSM-detected iron accumulation in the cerebellum (17, 18). This unprecedented finding highlights the need for further research into the role of cerebellar iron accumulation in cognitive impairment across neurodegenerative disorders, including ALS.
In this respect, it is also worth mentioning that a set of off-label analyses revealed that QSM-based measures of the cerebellar GM selectively predicted performance on backward digit span tasks, which are related to phonological working memory and social cognition tasks. The first finding aligns with previous neuroimaging studies in both healthy (42, 43) and brain-damaged populations (44, 45), which have linked phonological working memory abilities to the integrity of cerebellar structures. Similarly, the current link between social-cognitive abilities and iron accumulation in the cerebellar GM is consistent with the widely accepted idea that the cerebellum supports such a set of high-order skills by storing “social scripts,” which help individuals predict social interactions (46).
The present study is not free of limitations. First, the current investigation merely focuses on the cerebellum, which limits our ability to determine whether iron accumulation in other structures, either supratentorial or supratentorial, might also be associated with EF in the present cohort. Future research should explore whether the association between iron accumulation and FTD-spectrum cognitive deficits in ALS patients is specific to the cerebellum or extends to other brain regions.
Second, the lack of a control group prevented us from assessing whether QSM-based measures are similarly associated with executive functioning in healthy individuals. Third, the sample was relatively limited in size, possibly undermining the generalizability of the present findings. Fourth, the patients included were not stratified according to Strong et al.’s (47) criteria, and there were no cases of ALS with comorbid frontotemporal degeneration. Future studies should aim to replicate these analyses with a larger, more representative, and well-characterized sample of ALS patients.
Fifth, the cerebellum was segmented based on broad dichotomies (i.e., GM vs. WM and left vs. right hemispheric lateralization). Cerebellar nuclei were included within the WM ROIs and were not individually analyzed, although, as previously stated, cerebellar nuclei have been frequently identified as relevant to the clinical characterization of ALS (2). Therefore, further studies are needed to focus on more detailed cerebellar ROIs. In this respect, it should also be noted that, instead of using FreeSurfer, other cerebellum-specific segmentation techniques, such as SUIT, could have been employed (48). While the current choice of FreeSurfer was motivated by its widespread use and acceptability among local collaborating Italian institutions, future studies should consider using more specialized segmentation approaches to replicate or challenge these findings. Finally, a statistical limitation must be highlighted: collinearity was identified among cerebellar volumetrics within the LR model addressing bilateral QSM-based measures. Although this collinearity was expected, it may have introduced some bias into the results of the abovementioned model.
In conclusion, iron accumulation within the cerebellar GM, i.e., in the cerebellar cortices, may be associated with EF deficits in non-demented ALS patients, thereby suggesting that cerebellar in this population might contribute, at least in part, to FTD-spectrum phenotypic features in this population. Additionally, this report is the first to demonstrate the potential usefulness of QSM-based measures in uncovering the neural correlates of extra-motor cognitive deficits in ALS.
Data availability statement
Datasets associated with the present study have been stored in an online repository (https://doi.org/10.5281/zenodo.13619839). These datasets cannot be made publicly available on ethical and legal grounds. Requests to access the datasets should be directed to the corresponding authors who will forward a request for a data transfer agreement to the relevant Ethical Committee.
Ethics statement
The studies involving humans were approved by Ethics Committe of IRCCS Istituto Auxologico Italiano (I.D.: 23C722_2017). The studies were conducted in accordance with the local legislation and institutional requirements. The participants provided their written informed consent to participate in this study.
Author contributions
EA: Conceptualization, Formal analysis, Methodology, Writing – original draft, Writing – review & editing. VC: Conceptualization, Data curation, Formal analysis, Investigation, Methodology, Project administration, Resources, Software, Supervision, Writing – original draft, Writing – review & editing. GC: Data curation, Investigation, Methodology, Resources, Supervision, Writing – original draft, Writing – review & editing. FS: Data curation, Formal analysis, Writing – original draft, Writing – review & editing. BC: Formal analysis, Writing – original draft, Writing – review & editing. AM: Data curation, Writing – review & editing. ST: Data curation, Investigation, Writing – review & editing. SC: Supervision, Writing – review & editing. AD: Data curation, Writing – review & editing. EC: Data curation, Writing – review & editing. FV: Data curation, Supervision, Writing – review & editing. VS: Funding acquisition, Resources, Writing – review & editing. CL: Writing – review & editing. CC: Investigation, Methodology, Resources, Supervision, Validation, Writing – review & editing. FT: Resources, Writing – review & editing. CM: Conceptualization, Data curation, Investigation, Methodology, Project administration, Resources, Supervision, Validation, Writing – original draft, Writing – review & editing. BP: Investigation, Resources, Supervision, Validation, Writing – original draft, Writing – review & editing. NT: Funding acquisition, Resources, Writing – review & editing.
Funding
The author(s) declare that financial support was received for the research, authorship, and/or publication of this article. This study was funded by the European Union—Next Generation EU—NRRP M6C2—Investment 2.1: Enhancement and strengthening of biomedical research in the NHS.
Acknowledgments
The authors are thankful to patients, their caregivers, and healthy participants. The authors also acknowledge the support of the Italian Ministry of Health, Ricerca Corrente, and of the Italian Ministry of Education and Research (“Dipartimenti di Eccellenza” Program 2023–2027, Department of Pathophysiology and Transplantation, “Dino Ferrari” Center, Università degli Studi di Milano).
Conflict of interest
VS received compensation for consulting services and/or speaking activities from AveXis, Cytokinetics, Italfarmaco, Liquidweb S.r.l., and Novartis Pharma AG, receives or has received research supports from the Italian Ministry of Health, AriSLA, and E-Rare Joint Transnational Call. He is in the Editorial Board of Amyotrophic Lateral Sclerosis and Frontotemporal Degeneration, European Neurology, American Journal of Neurodegenerative Diseases, Frontiers in Neurology. BP received compensation for consulting services and/or speaking activities from Liquidweb S.r.l. She is Associate Editor for Frontier in Neuroscience. NT received compensation for consulting services from Amylyx Pharmaceuticals and Zambon Biotech SA. He is Associate Editor for Frontiers in Aging Neuroscience. ENA serves as an Editorial Board Member for BMC Neurology. FV is Associated Editor for Journal of Alzheimer’s Disease.
The remaining authors declare that the research was conducted in the absence of any commercial or financial relationships that could be construed as a potential conflict of interest.
The author(s) declared that they were an editorial board member of Frontiers, at the time of submission. This had no impact on the peer review process and the final decision.
Publisher’s note
All claims expressed in this article are solely those of the authors and do not necessarily represent those of their affiliated organizations, or those of the publisher, the editors and the reviewers. Any product that may be evaluated in this article, or claim that may be made by its manufacturer, is not guaranteed or endorsed by the publisher.
Supplementary material
The Supplementary material for this article can be found online at: https://www.frontiersin.org/articles/10.3389/fneur.2024.1426841/full#supplementary-material
Footnotes
References
1. Swinnen, B, and Robberecht, W. The phenotypic variability of amyotrophic lateral sclerosis. Nat Rev Neurol. (2014) 10:661–70. doi: 10.1038/nrneurol.2014.184
2. Chipika, RH, Mulkerrin, G, Pradat, PF, Murad, A, Ango, F, Raoul, C, et al. Cerebellar pathology in motor neuron disease: neuroplasticity and neurodegeneration. Neural Regen Res. (2022) 17:2335. doi: 10.4103/1673-5374.336139
3. Qiu, T, Zhang, Y, Tang, X, Liu, X, Wang, Y, Zhou, C, et al. Precentral degeneration and cerebellar compensation in amyotrophic lateral sclerosis: a multimodal MRI analysis. Hum Brain Mapp. (2019) 40:3464–74. doi: 10.1002/hbm.24609
4. Abidi, M, De Marco, G, Couillandre, A, Feron, M, Mseddi, E, Termoz, N, et al. Adaptive functional reorganization in amyotrophic lateral sclerosis: coexisting degenerative and compensatory changes. Eur J Neurol. (2020) 27:121–8. doi: 10.1111/ene.14042
5. Devita, M, Alberti, F, Fagnani, M, Masina, F, Ara, E, Sergi, G, et al. Novel insights into the relationship between cerebellum and dementia: a narrative review as a toolkit for clinicians. Ageing Res Rev. (2021) 70:101389. doi: 10.1016/j.arr.2021.101389
6. Tan, RH, Devenney, E, Dobson-Stone, C, Kwok, JB, Hodges, JR, Kiernan, MC, et al. Cerebellar integrity in the amyotrophic lateral sclerosis-frontotemporal dementia continuum. PLoS One. (2014) 9:e105632. doi: 10.1371/journal.pone.0105632
7. Matías-Guiu, JA, Pytel, V, Cabrera-Martín, MN, Galán, L, Valles-Salgado, M, Guerrero, A, et al. Amyloid-and FDG-PET imaging in amyotrophic lateral sclerosis. Eur J Nucl Med Mol Imaging. (2016) 43:2050–60. doi: 10.1007/s00259-016-3434-1
8. Consonni, M, Dalla Bella, E, Nigri, A, Pinardi, C, Demichelis, G, Porcu, L, et al. Cognitive syndromes and C9orf72 mutation are not related to cerebellar degeneration in amyotrophic lateral sclerosis. Front Neurosci. (2019) 13:440. doi: 10.3389/fnins.2019.00440
9. Floeter, MK, Katipally, R, Kim, MP, Schanz, O, Stephen, M, Danielian, L, et al. Impaired corticopontocerebellar tracts underlie pseudobulbar affect in motor neuron disorders. Neurology. (2014) 83:620–7. doi: 10.1212/WNL.0000000000000693
10. Canosa, A, Vacchiano, V, D'Ovidio, F, Calvo, A, Moglia, C, Manera, U, et al. Brain metabolic correlates of apathy in amyotrophic lateral sclerosis: an 18F-FDG-positron emission tomography stud. Eur J Neurol. (2021) 28:745–53. doi: 10.1111/ene.14637
11. Bharti, K, Khan, M, Beaulieu, C, Graham, SJ, Briemberg, H, Frayne, R, et al. Involvement of the dentate nucleus in the pathophysiology of amyotrophic lateral sclerosis: a multi-center and multimodal neuroimaging study. NeuroImage Clin. (2020) 28:102385. doi: 10.1016/j.nicl.2020.102385
12. Wang, Y, and Liu, T. Quantitative susceptibility mapping (QSM): decoding MRI data for a tissue magnetic biomarker. Magn Reson Med. (2015) 73:82–101. doi: 10.1002/mrm.25358
13. Contarino, VE, Conte, G, Morelli, C, Trogu, F, Scola, E, Calloni, SF, et al. Toward a marker of upper motor neuron impairment in amyotrophic lateral sclerosis: a fully automatic investigation of the magnetic susceptibility in the precentral cortex. Eur J Radiol. (2020) 124:108815. doi: 10.1016/j.ejrad.2020.108815
14. Conte, G, Contarino, VE, Casale, S, Morelli, C, Sbaraini, S, Scola, E, et al. Amyotrophic lateral sclerosis phenotypes significantly differ in terms of magnetic susceptibility properties of the precentral cortex. Eur Radiol. (2021) 31:5272–80. doi: 10.1007/s00330-020-07547-5
15. Costagli, M, Donatelli, G, Cecchi, P, Bosco, P, Migaleddu, G, Siciliano, G, et al. Distribution indices of magnetic susceptibility values in the primary motor cortex enable classifying patients with amyotrophic lateral sclerosis. Brain Sci. (2022) 12:942. doi: 10.3390/brainsci12070942
16. Bhattarai, A, Egan, GF, Talman, P, Chua, P, and Chen, Z. Magnetic resonance iron imaging in amyotrophic lateral sclerosis. J Magn Reson Imaging. (2022) 55:1283–300. doi: 10.1002/jmri.27530
17. Ravanfar, P, Loi, SM, Syeda, WT, Van Rheenen, TE, Bush, AI, Desmond, P, et al. Systematic review: quantitative susceptibility mapping (QSM) of brain iron profile in neurodegenerative diseases. Front Neurosci. (2021) 15:618435. doi: 10.3389/fnins.2021.618435
18. Verma, S., Goel, T., and Tanveer, M (2022). Quantitative susceptibility mapping in cognitive decline: a review of technical aspects and applications. Cognitive Computation.
19. Ndayisaba, A, Kaindlstorfer, C, and Wenning, GK. Iron in neurodegeneration, cause or consequence? Front Neurosci. (2019) 13:180. doi: 10.3389/fnins.2019.00180
20. Zhang, N, Yu, X, Xie, J, and Xu, H. New insights into the role of ferritin in iron homeostasis and neurodegenerative diseases. Mol Neurobiol. (2021) 58:2812–23. doi: 10.1007/s12035-020-02277-7
21. Nikparast, F, Ganji, Z, Danesh Doust, M, Faraji, R, and Zare, H. Brain pathological changes during neurodegenerative diseases and their identification methods: how does QSM perform in detecting this process? Insights Imaging. (2022) 13:1–20. doi: 10.1186/s13244-022-01207-6
22. Brooks, BR, Miller, RG, Swash, M, and Munsat, TL. El Escorial revisited: revised criteria for the diagnosis of amyotrophic lateral sclerosis. Amyotroph Lateral Scler Other Motor Neuron Disord. (2000) 1:293–9. doi: 10.1080/146608200300079536
23. Poletti, B, Solca, F, Carelli, L, Madotto, F, Lafronza, A, Faini, A, et al. The validation of the Italian Edinburgh cognitive and behavioural ALS screen (ECAS). Amyot Lateral Sclerosis Frontot Degen. (2016) 17:489–98. doi: 10.1080/21678421.2016.1183679
24. Gorno-Tempini, ML, Hillis, AE, Weintraub, S, Kertesz, A, Mendez, M, Cappa, SF, et al. Classification of primary progressive aphasia and its variants. Neurology. (2011) 76:1006–14. doi: 10.1212/WNL.0b013e31821103e6
25. Rascovsky, K, Hodges, JR, Knopman, D, Mendez, MF, Kramer, JH, Neuhaus, J, et al. Sensitivity of revised diagnostic criteria for the behavioural variant of frontotemporal dementia. Brain. (2011) 134:2456–77. doi: 10.1093/brain/awr179
26. Cedarbaum, JM, Stambler, N, Malta, E, Fuller, C, Hilt, D, Thurmond, B, et al. The ALSFRS-R: a revised ALS functional rating scale that incorporates assessments of respiratory function. J Neurol Sci. (1999) 169:13–21. doi: 10.1016/S0022-510X(99)00210-5
27. Poletti, B, Aiello, EN, Solca, F, Torre, S, Carelli, L, Ferrucci, R, et al. Diagnostic properties of the Italian ECAS Carer interview (ECAS-CI). Neurol Sci. (2023) 44:941–6. doi: 10.1007/s10072-022-06505-x
29. Liu, C, Li, W, Tong, KA, Yeom, KW, and Kuzminski, S. Susceptibility-weighted imaging and quantitative susceptibility mapping in the brain. J Magn Reson Imaging. (2015) 42:23–41. doi: 10.1002/jmri.24768
30. Smith, SM. Fast robust automated brain extraction. Hum Brain Mapp. (2002) 17:143–55. doi: 10.1002/hbm.10062
31. Wei, H, Dibb, R, Zhou, Y, Sun, Y, Xu, J, Wang, N, et al. Streaking artifact reduction for quantitative susceptibility mapping of sources with large dynamic range. NMR Biomed. (2015) 28:1294–303. doi: 10.1002/nbm.3383
32. Li, W, Wang, N, Yu, F, Han, H, Cao, W, Romero, R, et al. A method for estimating and removing streaking artifacts in quantitative susceptibility mapping. NeuroImage. (2015) 108:111–22. doi: 10.1016/j.neuroimage.2014.12.043
33. Kim, HY. Statistical notes for clinical researchers: assessing normal distribution (2) using skewness and kurtosis. Restorative Dent Endod. (2013) 38:52–4. doi: 10.5395/rde.2013.38.1.52
34. Ver Hoef, JM, and Boveng, PL. Quasi-Poisson vs. negative binomial regression: how should we model overdispersed count data? Ecology. (2007) 88:2766–72. doi: 10.1890/07-0043.1
35. Iazzolino, B, Pain, D, Laura, P, Aiello, EN, Gallucci, M, Radici, A, et al. Italian adaptation of the Beaumont behavioral inventory (BBI): psychometric properties and clinical usability. Amyot Lateral Sclerosis Frontot Degen. (2022) 23:81–6. doi: 10.1080/21678421.2021.1946085
36. Aiello, EN, Pain, D, Radici, A, Aktipi, KM, Sideri, R, Appollonio, I, et al. Cognition and motor phenotypes in ALS: a retrospective study. Neurol Sci. (2022) 43:5397–402. doi: 10.1007/s10072-022-06157-x
37. Aiello, EN, Depaoli, EG, and Gallucci, M. Usability of the negative binomial model for analyzing ceiling and highly-inter-individually-variable cognitive data. Neurol Sci. (2020) 41:S273–4. doi: 10.1007/s10072-020-04753-3
38. Saffari, SE, Soo, SA, Mohammadi, R, Ng, KP, Greene, W, and Kandiah, N. Modelling the distribution of cognitive outcomes for early-stage neurocognitive disorders: a model comparison approach. Biomedicine. (2024) 12:393. doi: 10.3390/biomedicines12020393
39. Midi, H, Sarkar, SK, and Rana, S. Collinearity diagnostics of binary logistic regression model. J Interdis Math. (2010) 13:253–67. doi: 10.1080/09720502.2010.10700699
40. Clark, SV, Semmel, ES, Aleksonis, HA, Steinberg, SN, and King, TZ. Cerebellar-subcortical-cortical systems as modulators of cognitive functions. Neuropsychol Rev. (2021) 31:422–46. doi: 10.1007/s11065-020-09465-1
41. Bellebaum, C, and Daum, I. Cerebellar involvement in executive control. Cerebellum. (2007) 6:184–92. doi: 10.1080/14734220601169707
42. Gerton, BK, Brown, TT, Meyer-Lindenberg, A, Kohn, P, Holt, JL, Olsen, RK, et al. Shared and distinct neurophysiological components of the digits forward and backward tasks as revealed by functional neuroimaging. Neuropsychologia. (2004) 42:1781–7. doi: 10.1016/j.neuropsychologia.2004.04.023
43. Emch, M, Von Bastian, CC, and Koch, K. Neural correlates of verbal working memory: an fMRI meta-analysis. Front Hum Neurosci. (2019) 13:180. doi: 10.3389/fnhum.2019.00180
44. Ravizza, SM, McCormick, CA, Schlerf, JE, Justus, T, Ivry, RB, and Fiez, JA. Cerebellar damage produces selective deficits in verbal working memory. Brain. (2006) 129:306–20. doi: 10.1093/brain/awh685
45. Cooper, FE, Grube, M, Von Kriegstein, K, Kumar, S, English, P, Kelly, TP, et al. Distinct critical cerebellar subregions for components of verbal working memory. Neuropsychologia. (2012) 50:189–97. doi: 10.1016/j.neuropsychologia.2011.11.017
46. Van Overwalle, F, Manto, M, Cattaneo, Z, Clausi, S, Ferrari, C, Gabrieli, JD, et al. Consensus paper: cerebellum and social cognition. Cerebellum. (2020) 19:833–68. doi: 10.1007/s12311-020-01155-1
47. Strong, MJ, Abrahams, S, Goldstein, LH, Woolley, S, Mclaughlin, P, Snowden, J, et al. Amyotrophic lateral sclerosis-frontotemporal spectrum disorder (ALS-FTSD): revised diagnostic criteria. Amyot Lateral Sclerosis Frontot Degen. (2017) 18:153–74. doi: 10.1080/21678421.2016.1267768
Keywords: quantitative susceptibility imaging, amyotrophic lateral sclerosis, cerebellum, frontotemporal degeneration, executive functioning
Citation: Aiello EN, Contarino VE, Conte G, Solca F, Curti B, Maranzano A, Torre S, Casale S, Doretti A, Colombo E, Verde F, Silani V, Liu C, Cinnante C, Triulzi FM, Morelli C, Poletti B and Ticozzi N (2024) QSM-detected iron accumulation in the cerebellar gray matter is selectively associated with executive dysfunction in non-demented ALS patients. Front. Neurol. 15:1426841. doi: 10.3389/fneur.2024.1426841
Edited by:
Thomas Welton, National Neuroscience Institute (NNI), SingaporeReviewed by:
Andrea Calvo, University of Turin, ItalySilvia Basaia, Neuroimaging Research Unit, Division of Neuroscience, IRCCS San Raffaele Scientific Institute, Milan, Italy
Jun Li, School of Information Science and Technology, ShanghaiTech University, China
Copyright © 2024 Aiello, Contarino, Conte, Solca, Curti, Maranzano, Torre, Casale, Doretti, Colombo, Verde, Silani, Liu, Cinnante, Triulzi, Morelli, Poletti and Ticozzi. This is an open-access article distributed under the terms of the Creative Commons Attribution License (CC BY). The use, distribution or reproduction in other forums is permitted, provided the original author(s) and the copyright owner(s) are credited and that the original publication in this journal is cited, in accordance with accepted academic practice. No use, distribution or reproduction is permitted which does not comply with these terms.
*Correspondence: Valeria Elisa Contarino, valeria.contarino@policlinico.mi.it; Barbara Poletti, b.poletti@auxologico.it
†These authors have contributed equally to this work
‡ORCID: Valeria Elisa Contarino, orcid.org/00000-0002-8922-657X
Barbara Poletti, orcid.org/00000-0003-4398-2051