- Department of Neurology, General Hospital of Northern Theater Command, Shenyang, China
Objective: This study aimed to develop a nomogram tool to predict cerebral white matter lesions (WMLs) in elderly men.
Methods: Based on a retrospective cohort from January 2017 to December 2019, a multivariate logistic analysis was performed to construct a nomogram for predicting WMLs. The nomogram was further validated using a follow-up cohort between January 2020 and December 2022. The calibration curve, receiver operating characteristics (ROC) curves, and the decision curves analysis (DCA) were used to evaluate discrimination and calibration of this nomogram.
Result: A total of 436 male patients were enrolled in this study, and all 436 patients were used as the training cohort and 163 follow-up patients as the validation cohort. A multivariate logistic analysis showed that age, cystatin C, uric acid, total cholesterol, platelet, and the use of antiplatelet drugs were independently associated with WMLs. Based on these variables, a nomogram was developed. The nomogram displayed excellent predictive power with the area under the ROC curve of 0.951 [95% confidence interval (CI), 0.929–0.972] in the training cohort and 0.915 (95% CI, 0.864–0.966) in the validation cohort. The calibration of the nomogram was also good, as indicated by the Hosmer–Lemeshow test with p-value of 0.594 in the training cohort and 0.178 in the validation cohort. The DCA showed that the nomogram holds good clinical application value.
Conclusion: We have developed and validated a novel nomogram tool for identifying elderly men at high risk of WMLs, which exhibits excellent predictive power, discrimination, and calibration.
Introduction
Cerebral white matter lesions (WMLs) are not only an important part of cerebral small vessel disease (SCVD), but also one of the most common causes of cognitive impairment in the elderly, and considered to be an early sign of brain damage (1, 2). The incidence of WMLs in the elderly has been reported to range from 11% to 21%, with rates reaching as high as 94% in advanced-aged individuals (3). Given the increasing global aging population, research on WMLs has gained significant attention (4). Growing evidence showed that heavier WMLs burden was closely associated with more severe cognitive impairment (5, 6). Some studies have shown that the location of WMLs was closely related to the prognosis of patients with acute ischemic stroke (7), and the severity of WMLs may affect the functional prognosis of ischemic stroke (8). Other studies suggest that WMLs can increase the risk of dementia and death in stroke patients (3). Furthermore, a prospective study revealed that severe WMLs can predict composite endpoints of death, pneumonia, and falls (9).
Some studies have identified the correlations of age, hypertension, female gender, high density lipoprotein (HDL) levels, and the use of antihypertensive medications with occurrence of WMLs (10, 11). However, accurately predicting the occurrence of WMLs remains a challenge. Only one recent study reported a prediction model focused on the elderly (10). However, constructing models that incorporate a wider range of clinically relevant variables could help enhance the accuracy of WMLs prediction, which is crucial for identifying the appropriate population for early intervention.
In this context, the current study aims to develop a predictive nomogram based on comprehensive baseline characteristics of elderly men.
Methods
Patients and study design
We retrospectively enrolled consecutive patients who underwent comprehensive medical examination including brain magnetic resonance imaging (MRI) between January 2017 and December 2019. Eligible patients were used as a training cohort to develop a nomogram for predicting WMLs. After this time, all these patients were retrospectively followed up for 3 years (between January 2020 and December 2022) as the validation cohort. Patients fulfilling the following eligibility criteria were included for analysis: (a) age ≥ 60 years; (b) complete brain MRI including T2-weighted images and fluid-attenuated inversion recovery (FLAIR); (c) complete medical examination. The exclusion criteria: (a) incomplete clinical data; (b) large area cerebral infarction, cerebral hemorrhage, subarachnoid hemorrhage and craniocerebral trauma; (c) severe liver dysfunction, severe cardiac dysfunction, hypothyroidism, gout, autoimmune system diseases, severe systemic infection; (d) taking immunosuppressant recently; (e) taking B vitamins and folic acid recently; (f) taking uric acid-lowering drugs recently; (g) other conditions known to cause WMLs, such as carbon monoxide poisoning, hypoxic encephalopathy, various types of hydrocephalus, immune white matter demyelination and so on; (h) diseases that may interfere with the analysis of WMLs, such as brain tumors, cysts and history of craniocerebral surgery, etc. This study was approved by the Ethics Committee of General Hospital of Northern Theater Command, Shenyang and waived the need for informed consent from all subjects.
Data collection
The following demographic and clinical characteristics were recorded: age, weight, smoking and drinking history, admission systolic blood pressure (SBP) and admission diastolic blood pressure (DBP), medical comorbidity [hypertension, diabetes, coronary heart disease (CHD), atrial fibrillation (AF), left heart failure (LHF) and tumor]; the use of statins, antiplatelet drugs and anticoagulants. Laboratory test values including serum total cholesterol (TC), triglyceride (TG), high density lipoprotein (HDL), low density lipoprotein (LDL), lipoprotein-A (LP-A), albumin (ALB), total protein (TP), high-sensitivity C-reactive protein (h-CRP), platelets (PLT), glycosylated hemoglobin (HbA1C), total bilirubin (TBIL), direct bilirubin (DBIL), indirect bilirubin (IBIL), alanine aminotransferase (ALT), gamma glutamyl transpeptidase (GGT), homocysteine (Hcy), cystatin C (Cys C), uric acid (UA), creatinine (CREA), urea nitrogen (UREA) and brain natriuretic peptide (BNP).
WMLs assessment
The WMLs were evaluated utilizing the Fazekas scale on MRI (12), and were defined as any occurrence of WMLs (namely a Fazekas score of at least 1 point). In the scale, periventricular and deep WMLs were rated separately, and periventricular WMLs were graded according to the following patterns: 0 = absent; 1 = caps or pencil-thin lining; 2 = smooth halo; and 3 = irregular periventricular WMLs extending into a deep WMLs. Deep WMLs were graded according to the following patterns: 0 = absent; 1 = punctate foci; 2 = beginning confluence of foci; and 3 = large fused areas. A total Fazekas score, ranging from 0 to 6, was acquired by summing the periventricular and deep WMLs scores. Based on the Fazekas scale, WMLs did not include old lacunar infarctions and/or white matter lesions at some other locations such as basal ganglia, deep gray matter and cortical/subcortical areas. The neuroimaging data were evaluated independently by two experienced neurologists who were blinded to the clinical data. Any disagreement between the two assessors was resolved by discussion until a consensus was achieved.
Statistical analysis
The patient data in the training cohort were used to develop the prediction model, and the patient data in the validation cohort were used to validate the model. Gaussian distributed data were expressed as means ± standard deviation (SD) and compared by student’s t-test, while non-Gaussian distributed data were expressed as medians (interquartile range, Q2–Q3) and compared by the Mann–Whitney U test. Categorical data are expressed using frequencies and ratios (%) and Fisher’s exact tests or the χ2 tests were used for categorical variables. SPSS 26.0 was used for statistical analyses. All variables with a probability value < 0.10 in the univariate analysis entered into a multivariate logistic regression analysis using a backwards stepwise method. Stata software (version 17.0) was used to build the nomogram prediction model. The nomogram converts each independent risk factor included in the model into an assessment point system. The total points obtained determine the final risk assessment value. The odds ratio (OR) and 95% confidence interval (CI) of each significant risk factor in the final logistic regression model were calculated. Performances of the nomogram were assessed in the validation cohort. Discrimination of the nomogram was assessed using the area under the ROC curve (AUC). An AUC of 0.51–0.7 indicates low accuracy, 0.71–0.9 indicates moderate accuracy, and 0.91–1.0 indicates high accuracy to discriminate between patients with WMLs and without WMLs. The calibration of the nomogram was evaluated by the Hosmer-Lemeshow test (p > 0.05 indicates good calibration). In addition, a decision curve analysis (DCA) of the model was developed to quantify the net benefit rate at different threshold probabilities to assess the clinical validity of the model. All statistical tests were 2-tailed. We deemed statistical significance at p = 0.05.
Results
Demographic and clinical features
As shown in Figure 1, 1,276 patients were screened for eligibility and 436 patients were included in the training cohort after excluding 840 individuals. The patients in the training cohort were followed for 3 years. However, due to incomplete follow-up information, 273 patients were subsequently excluded. As a result, a total of 163 follow-up patients were included in the validation cohort. The demographics and clinical characteristics of the patients in the two cohorts were shown in Table 1. There was a good balance in the baseline characteristics between the training and validation cohort except for tumor history, statins history, uric acid and serum total protein. The average age of patients in the training cohort and validation cohort were 73.93 ± 14.15 and 75.22 ± 11.84 years old, respectively. The prevalence rate of WMLs in the training cohort was 65.6%, whereas in the validation cohort was as high as 82.6%.
Predictors for WMLs and nomogram construction
In the training cohort, a univariate analysis was conducted to investigate the association of several variables with WMLs. These variables include age, medical comorbidities (hypertension, CHD, tumor), SBP, use of statins, use of antiplatelet drugs, laboratory tests (Hcy, Cys C, UA, CREA, UREA, h-CRP, TC, TG, HDL, LDL, TP, ALB, BNP, HbA1C, PLT; Table 2). Finally, six variables remained significant in the multivariate logistic model, including age, cystatin C, total cholesterol, platelet, uric acid and use of antiplatelet drugs (Table 2). The predictive nomogram for WMLs that integrated these six variables was constructed and depicted in Figure 2. Based on this nomogram, the probability of predicting WMLs in patients can be calculated as follows: PWMLs = 1/(1 + ex), x = −(−15.29 + 0.099 × age − 0.174 × antiplatelet drugs (yes) + 0 × antiplatelet drugs (no) + 6.455 × cystatin C − 0.611 × total cholesterol +0.023 × platelet +0.006 × uric acid).
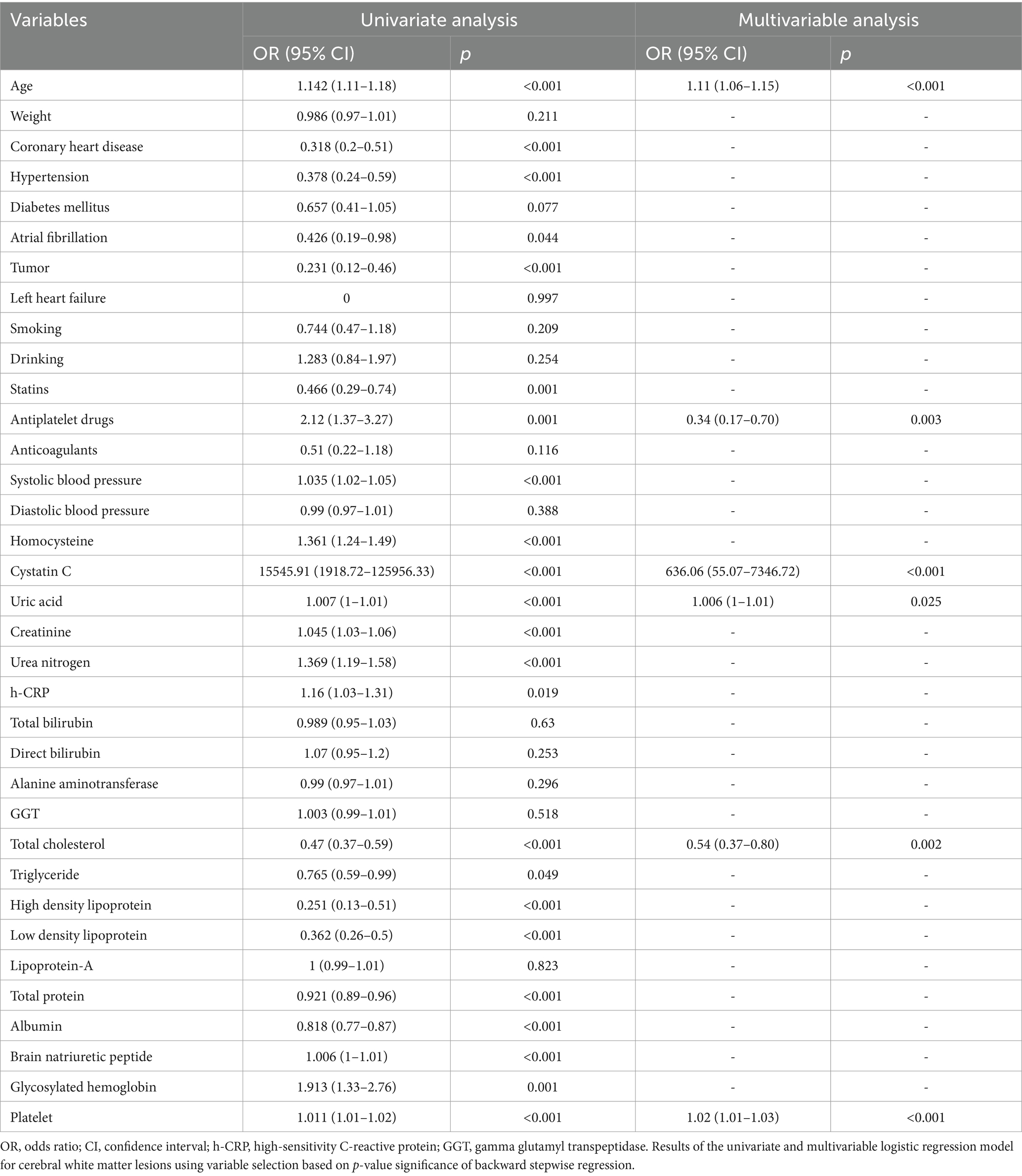
Table 2. Univariate and multivariable logistic regression analysis of the variables associated with white matter lesions in the training cohort.
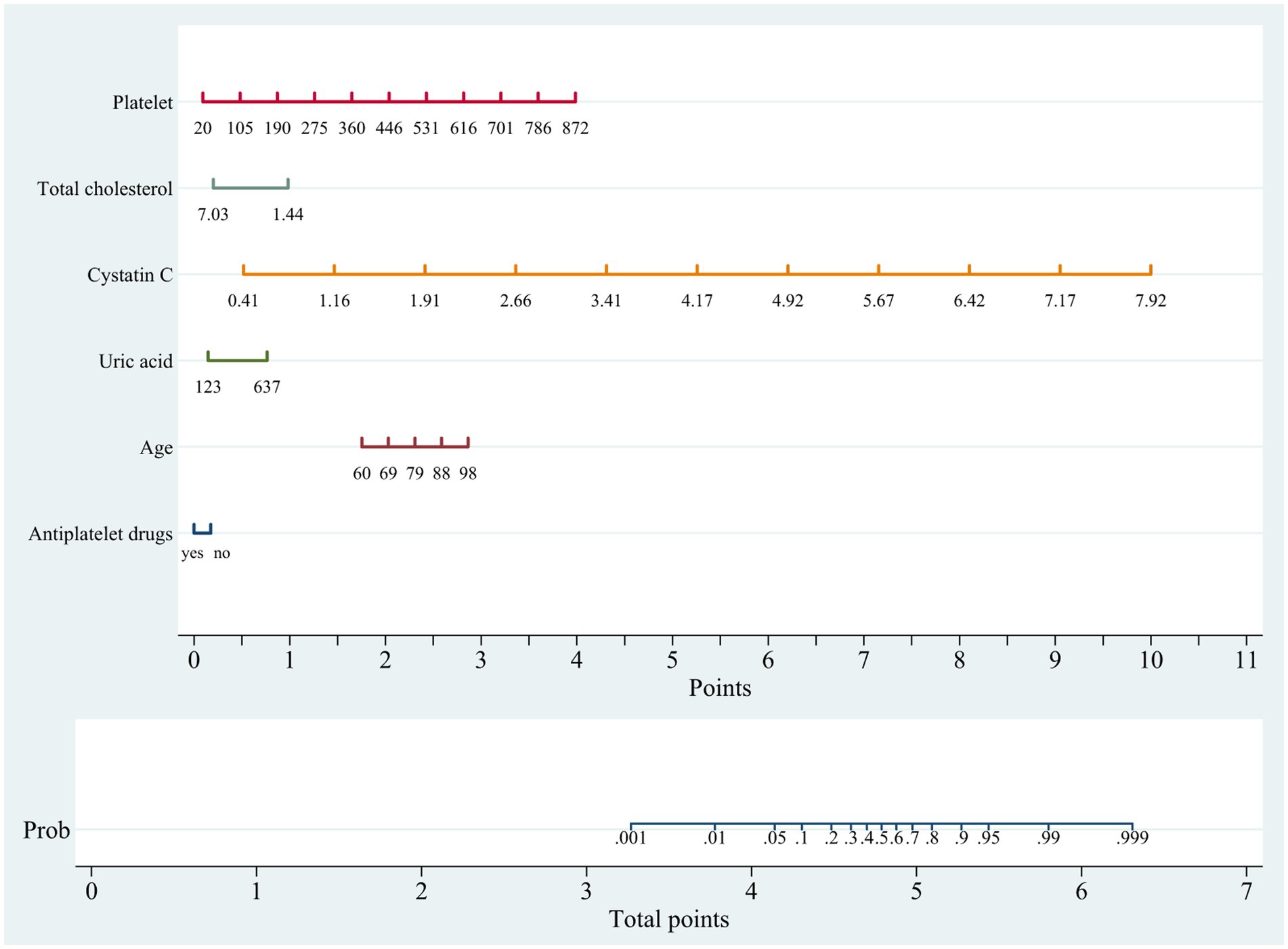
Figure 2. Nomogram for predicting cerebral white matter lesions in training cohort. Points are assigned based on age, cystatin C, total cholesterol, platelet, uric acid and antiplatelet drugs by drawing a line upward from the corresponding values to the “Points” line. The sum of these six points, plotted on the “Total points” line, corresponds to prediction of cerebral white matter lesions.
Assessment, validation of predictive accuracy of the nomogram for WMLs
The ROC (Figures 3A,B) and calibration curve were plotted (Figures 3C,D) to verify the accuracy and discrimination of this model. The AUC in the training cohort was 0.951 (95% CI, 0.929–0.972), while 0.915 (95% CI, 0.864–0.966) in the validation cohort, which suggested that our model had good accuracy. The calibration curve of the training cohort and validation cohort showed that there was excellent consistency between the predicted survival probability and the actual survival probability. The Hosmer-Lemeshow test for the training cohort yielded a p-value of 0.594, suggesting that the nomogram had a perfect fit. The calibration of the nomogram was further confirmed with the validation cohort, for which the Hosmer-Lemeshow test yielded a p-value of 0.178, suggesting no departure from the good fit of the nomogram. DCA was also plotted to evaluate the usefulness of the nomogram in the clinical utility (Figures 4A,B) and the results showed that the predictive nomogram provided sound clinical guidance with a good net benefit.
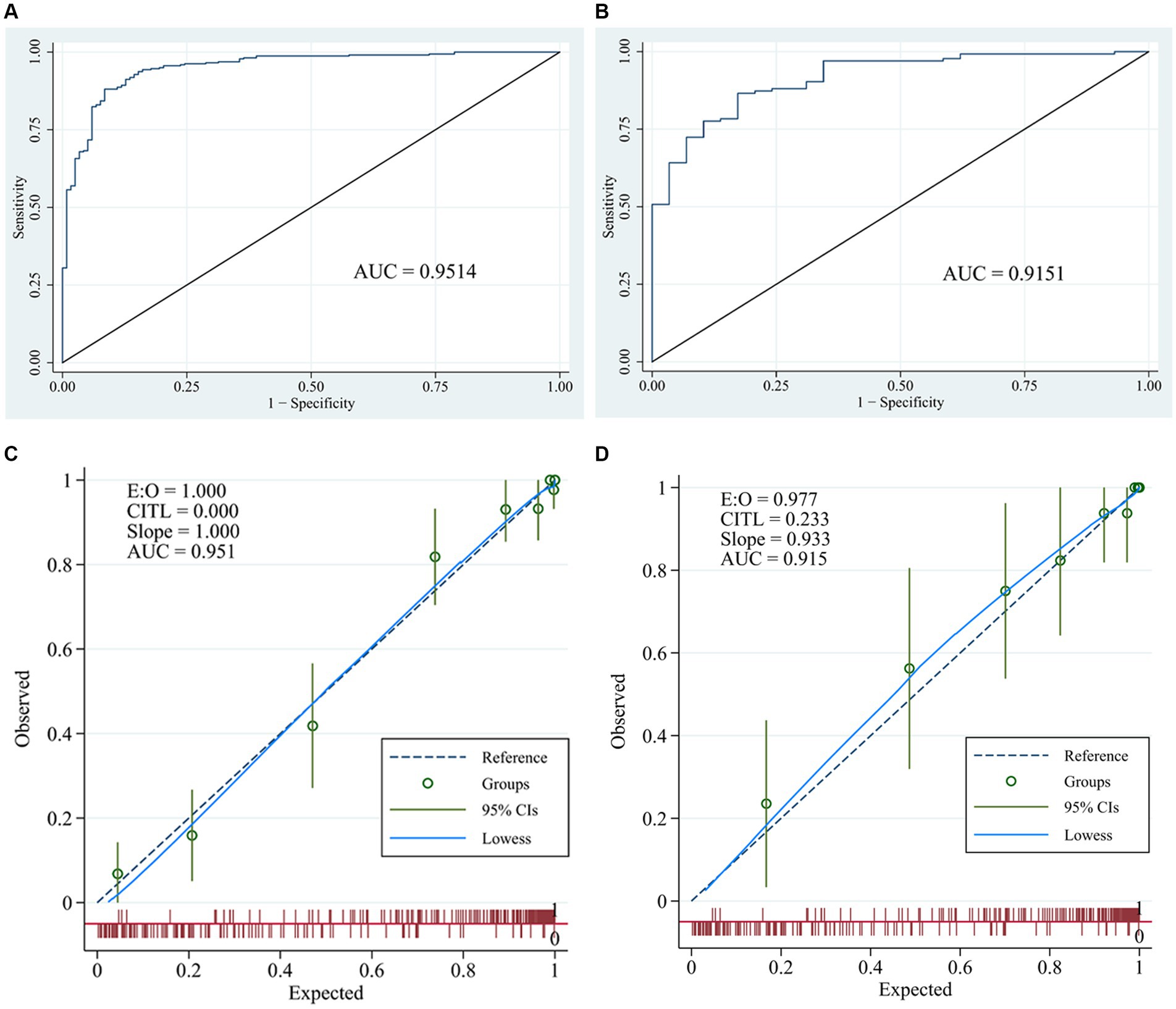
Figure 3. Receiver operating characteristic curves and calibration curves. ROC curve and area under the curve (AUC) in training cohort (A) and validation cohort (B) of the nomogram; calibration curve of training cohort (C) and validation cohort (D) of the nomogram.
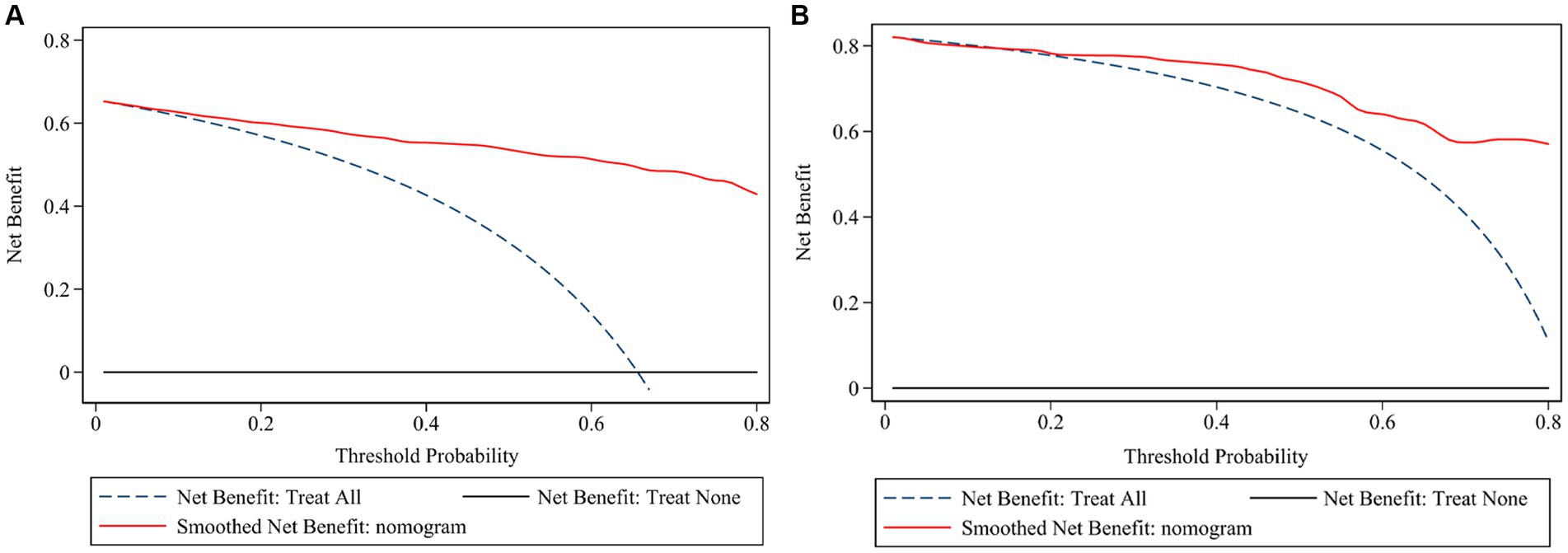
Figure 4. Decision curve analysis of the risk prediction model of white matter lesions. Decision curve of training cohort (A) and validation cohort (B); the net benefit was plotted vs. the threshold probability. The horizontal black dash line depicts net benefit of a strategy of treating no patients. The blue dash line depicts net benefit of a strategy of treating all patients. The red solid line represents the nomogram.
Discussion
In the current study, we developed and validated a predictive nomogram to identify the increased risk of WMLs in the male elderly patients over 60 years old. The newly developed model incorporated several key factors, including age, TC, PLT, Cys C, UA, and use of antiplatelet drugs. The resulting nomogram showed excellent predictive power as well as good discrimination and calibration.
Among these six variables, age, Cys C, and platelet were found to have a greater impact on WMLs. It was expected that age was found as a main contributor of this model, because age has been well demonstrated to be associated with WMLs (13–15). Given the inhibitory effect of increased serum Cys C on the contractile function of arterioles (16), and the damaging effect on the blood–brain barrier (17), the close association of Cys C with WMLs was explainable in the current study. In agreement with prior studies, platelet was found to be associated with WMLs in this study. Kuriyama et al. (18) found that the risk of WMLs in the elderly with high platelet activity increased by about threefold. Higher platelet aggregation rate have been linked to a higher incidence of WMLs, with a risk significantly higher than classical vascular risk factors such as hypertension, diabetes, and age (19). In addition, aspirin administration in patients with WMLs not only prevent cerebral infarction, but also curb the progress of WMLs (20), which was in agreement with the current finding that antiplatelet drug was independently associated with WMLs. Collectively, these results suggest the potential association of platelet and use of antiplatelet drug with WMLs.
In the current study, UA was also found to be associated with WMLs. Prior studies have found that hyperuricemia was an independent risk factor for WMLs (21). The underlying mechanisms included its effect on vascular endothelial cell dysfunction and atherosclerosis (22). Remarkably, our study found that lower TC levels may lead to an increased risk of WMLs, which seems contradictory with previous studies reporting that high cholesterol levels increased the risk of WMLs (23, 24). We argue that there are two possible reasons. First, as the main components of cell membrane and nerve myelin sheath, cholesterol plays a fundamental role in the development of the central nervous system and the creation and maintenance of new synapses (25, 26). This could explain the effect of significantly lower cholesterol levels on chronic cerebral injury, such as the developments of WMLs. Second, many studies have confirmed that intensive lipid reduction will greatly increase the risk of cerebral micro bleeds (CMBs) (27, 28), while there was a close correlation between CMBs and the formation of WMLs (29), which may explain the association of significantly lower cholesterol levels with WMLs. Furthermore, the current finding was supported by some studies reporting that the increase of TC was considered to be a protective factor of WMLs (30, 31). In addition, some studies did not find a correlation between TC and WMLs (32). Although there was a higher prevalence of diabetes and hypertension in the current study, we did not find the association of diabetes, hypertension and blood pressure with WMLs, which were known to be associated with WMLs (33–35). One possible explanation is that the blood pressure and blood glucose were well controlled in this cohort (36, 37). In addition, high prevalence of WMLs in both cohorts due to elderly patients (older than 60) and high prevalence (>60%) of hypertension may mask their associations, so their associations with severity of WMLs deserve to be further investigated. However, some studies did not find a correlation between diabetes and WMLs (38, 39), which was in line with our findings.
Our study possesses two potential strengths. First, our nomogram was created based on analysis of comprehensive and routinely available baseline variables in a training cohort, which was further validated in a follow-up cohort. Second, Cys C, UA, TC, platelet, and the use of antiplatelet drugs were incorporated into the prediction model for the first time and had excellent predictive power, and good discrimination and calibration. Nevertheless, we acknowledge several limitations. First, this was a retrospective observational study, which had common limitations including bias, potential lack of baseline data and inevitable confounding effect, although baseline data were comprehensively collected. Second, this was a single-center study without an external validation cohort, but it was validated by a follow-up cohort. Third, as almost all the patients in our center were male, our study did not include female patients. So, this finding is only applicable to male patients. Fourth, we did not collect the data about other brain lesions or underlying disease, which might have effect on this finding given their associations with WMLs. Finally, further confirmation of these findings in non-Chinese populations would be needed, given the differences in body mass and comorbid factors compared with other populations.
Conclusion
A novel nomogram tool has been developed to predict WMLs with excellent predicting power, good discrimination and calibration. The model should be helpful to identify elderly men at high risk of cerebral WMLs.
Data availability statement
The raw data supporting the conclusions of this article will be made available by the authors, without undue reservation.
Ethics statement
The studies involving humans were approved by the Institutional Review Board of General Hospital of Northern Theater Command. The studies were conducted in accordance with the local legislation and institutional requirements. The ethics committee/institutional review board waived the requirement of written informed consent for participation from the participants or the participants’ legal guardians/next of kin because this is a retrospective study.
Author contributions
Y-FP: Data curation, Formal analysis, Writing – original draft. X-DL: Data curation, Formal analysis, Writing – original draft. Q-YL: Data curation, Formal analysis, Writing – original draft. C-WZ: Formal analysis, Writing – original draft. Y-HW: Data curation, Writing – original draft. M-RC: Writing – original draft. H-SC: Funding acquisition, Supervision, Writing – review & editing.
Funding
The author(s) declare that financial support was received for the research, authorship, and/or publication of this article. The work was supported by grants from Science and Technology Planning Project of Liaoning Province (2022JH2/101500020).
Conflict of interest
The authors declare that the research was conducted in the absence of any commercial or financial relationships that could be construed as a potential conflict of interest.
Publisher’s note
All claims expressed in this article are solely those of the authors and do not necessarily represent those of their affiliated organizations, or those of the publisher, the editors and the reviewers. Any product that may be evaluated in this article, or claim that may be made by its manufacturer, is not guaranteed or endorsed by the publisher.
References
1. Pantoni, L, Poggesi, A, and Inzitari, D. The relation between white-matter lesions and cognition. Curr Opin Neurol. (2007) 20:390–7. doi: 10.1097/WCO.0b013e328172d661
2. Ble, A, Ranzini, M, Zurlo, A, Menozzi, L, Atti, AR, Munari, MR, et al. Leukoaraiosis is associated with functional impairment in older patients with Alzheimer’s disease but not vascular dementia. J Nutr Health Aging. (2006) 10:31–5.
3. Debette, S, and Markus, HS. The clinical importance of white matter hyperintensities on brain magnetic resonance imaging: systematic review and meta-analysis. BMJ. (2010) 341:c3666. doi: 10.1136/bmj.c3666
4. Altaf, N, Daniels, L, Morgan, PS, Lowe, J, Gladman, J, MacSweeney, ST, et al. Cerebral white matter hyperintense lesions are associated with unstable carotid plaques. Eur J Vasc Endovasc Surg. (2006) 31:8–13. doi: 10.1016/j.ejvs.2005.08.026
5. Caunca, MR, De Leon-Benedetti, A, Latour, L, Leigh, R, and Wright, CB. Neuroimaging of cerebral small vessel disease and age-related cognitive changes. Front Aging Neurosci. (2019) 11:145. doi: 10.3389/fnagi.2019.00145
6. Kloppenborg, RP, Nederkoorn, PJ, Geerlings, MI, and van den Berg, E. Presence and progression of white matter hyperintensities and cognition: a meta-analysis. Neurology. (2014) 82:2127–38. doi: 10.1212/WNL.0000000000000505
7. Jagani, M, Kallmes, DF, and Brinjikji, W. Correlation between clot density and recanalization success or stroke etiology in acute ischemic stroke patients. Interv Neuroradiol. (2017) 23:274–8. doi: 10.1177/1591019917694478
8. Onteddu, SR, Goddeau, RJ, Minaeian, A, and Henninger, N. Clinical impact of leukoaraiosis burden and chronological age on neurological deficit recovery and 90-day outcome after minor ischemic stroke. J Neurol Sci. (2015) 359:418–23. doi: 10.1016/j.jns.2015.10.005
9. Briley, DP, Haroon, S, Sergent, SM, and Thomas, S. Does leukoaraiosis predict morbidity and mortality? Neurology. (2000) 54:90–4. doi: 10.1212/WNL.54.1.90
10. Li, J, and Xie, XM. Nomograms for predicting the incidence of white matter lesions: a real-world study based on elderly patients. Eur Rev Med Pharmacol Sci. (2023) 27:151–8. doi: 10.26355/eurrev_202301_30866
11. Sawada, H, Udaka, F, Izumi, Y, Nishinaka, K, Kawakami, H, Nakamura, S, et al. Cerebral white matter lesions are not associated with apoE genotype but with age and female sex in Alzheimer's disease. J Neurol Neurosurg Psychiatry. (2000) 68:653–6. doi: 10.1136/jnnp.68.5.653
12. Fazekas, F, Chawluk, JB, Alavi, A, Hurtig, HI, and Zimmerman, RA. MR signal abnormalities at 1.5 T in Alzheimer's dementia and normal aging. AJR Am J Roentgenol. (1987) 149:351–6. doi: 10.2214/ajr.149.2.351
13. Franchetti, MK, Bharadwaj, PK, Nguyen, LA, van Etten, EJ, Klimentidis, YC, Hishaw, GA, et al. Interaction of age and self-reported physical sports activity on white matter Hyperintensity volume in healthy older adults. Front Aging Neurosci. (2020) 12:576025. doi: 10.3389/fnagi.2020.576025
14. Shinkawa, Y, Yoshida, T, Onaka, Y, Ichinose, M, and Ishii, K. Mathematical modeling for the prediction of cerebral white matter lesions based on clinical examination data. PLoS One. (2019) 14:e215142. doi: 10.1371/journal.pone.0215142
15. Vedala, K, Nagabandi, AK, Looney, S, and Bruno, A. Factors associated with Leukoaraiosis severity in acute stroke patients. J Stroke Cerebrovasc Dis. (2019) 28:1897–901. doi: 10.1016/j.jstrokecerebrovasdis.2019.04.003
16. Lafarge, JC, Naour, N, Clement, K, and Guerre-Millo, M. Cathepsins and cystatin C in atherosclerosis and obesity. Biochimie. (2010) 92:1580–6. doi: 10.1016/j.biochi.2010.04.011
17. Kanhai, DA, de Kleijn, DP, Kappelle, LJ, Uiterwaal, CS, van der Graaf, Y, Pasterkamp, G, et al. Extracellular vesicle protein levels are related to brain atrophy and cerebral white matter lesions in patients with manifest vascular disease: the SMART-MR study. BMJ Open. (2014) 4:e3824. doi: 10.1136/bmjopen-2013-003824
18. Kuriyama, N, Mizuno, T, Yasuike, H, Matsuno, H, Kawashita, E, Tamura, A, et al. CD62-mediated activation of platelets in cerebral white matter lesions in patients with cognitive decline. Arch Gerontol Geriatr. (2016) 62:118–24. doi: 10.1016/j.archger.2015.09.001
19. Fujita, S, and Kawaguchi, T. Association of platelet hyper-aggregability with leukoaraiosis. Acta Neurol Scand. (2002) 105:445–9. doi: 10.1034/j.1600-0404.2002.01208.x
20. Fujita, S, Kawaguchi, T, Uehara, T, and Fukushima, K. Progress of leukoaraiosis is inhibited by correction of platelet hyper-aggregability. Int Psychogeriatr. (2005) 17:689–98. doi: 10.1017/S104161020500164X
21. Sampat, R, Young, S, Rosen, A, Bernhard, D, Millington, D, Factor, S, et al. Potential mechanisms for low uric acid in Parkinson disease. J Neural Transm. (2016) 123:365–70. doi: 10.1007/s00702-015-1503-4
22. Li, JJ, Huang, YH, Lin, YY, Li, MM, Chen, YF, and Cai, RW. The association of uric acid with leukoaraiosis. J Int Med Res. (2017) 45:75–81. doi: 10.1177/0300060516674353
23. Park, K, Yasuda, N, Toyonaga, S, Yamada, SM, Nakabayashi, H, Nakasato, M, et al. Significant association between leukoaraiosis and metabolic syndrome in healthy subjects. Neurology. (2007) 69:974–8. doi: 10.1212/01.wnl.0000266562.54684.bf
24. Dufouil, C, de Kersaint-Gilly, A, Besancon, V, Levy, C, Auffray, E, Brunnereau, L, et al. Longitudinal study of blood pressure and white matter hyperintensities: the EVA MRI cohort. Neurology. (2001) 56:921–6. doi: 10.1212/WNL.56.7.921
25. Goritz, C, Mauch, DH, and Pfrieger, FW. Multiple mechanisms mediate cholesterol-induced synaptogenesis in a CNS neuron. Mol Cell Neurosci. (2005) 29:190–201. doi: 10.1016/j.mcn.2005.02.006
26. Dietschy, JM, and Turley, SD. Cholesterol metabolism in the brain. Curr Opin Lipidol. (2001) 12:105–12. doi: 10.1097/00041433-200104000-00003
27. Mitaki, S, Nagai, A, Oguro, H, and Yamaguchi, S. Serum lipid fractions and cerebral microbleeds in a healthy Japanese population. Cerebrovasc Dis. (2017) 43:186–91. doi: 10.1159/000456623
28. Yano, K, Reed, DM, and MacLean, CJ. Serum cholesterol and hemorrhagic stroke in the Honolulu heart program. Stroke. (1989) 20:1460–5. doi: 10.1161/01.STR.20.11.1460
29. Yamada, S, Saiki, M, Satow, T, Fukuda, A, Ito, M, Minami, S, et al. Periventricular and deep white matter leukoaraiosis have a closer association with cerebral microbleeds than age. Eur J Neurol. (2012) 19:98–104. doi: 10.1111/j.1468-1331.2011.03451.x
30. Ohwaki, K, Yano, E, Tamura, A, Inoue, T, and Saito, I. Hypercholesterolemia is associated with a lower risk of cerebral ischemic small vessel disease detected on brain checkups. Clin Neurol Neurosurg. (2013) 115:669–72. doi: 10.1016/j.clineuro.2012.07.025
31. Jimenez-Conde, J, Biffi, A, Rahman, R, Kanakis, A, Butler, C, Sonni, S, et al. Hyperlipidemia and reduced white matter hyperintensity volume in patients with ischemic stroke. Stroke. (2010) 41:437–42. doi: 10.1161/STROKEAHA.109.563502
32. Saji, N, Shimizu, H, Kawarai, T, Tadano, M, Kita, Y, and Yokono, K. Increased brachial-ankle pulse wave velocity is independently associated with white matter hyperintensities. Neuroepidemiology. (2011) 36:252–7. doi: 10.1159/000328260
33. Marseglia, A, Fratiglioni, L, Kalpouzos, G, Wang, R, Bäckman, L, and Xu, W. Prediabetes and diabetes accelerate cognitive decline and predict microvascular lesions: a population-based cohort study. Alzheimers Dement. (2019) 15:25–33. doi: 10.1016/j.jalz.2018.06.3060
34. Allan, CL, Zsoldos, E, Filippini, N, Sexton, CE, Topiwala, A, Valkanova, V, et al. Lifetime hypertension as a predictor of brain structure in older adults: cohort study with a 28-year follow-up. Br J Psychiatry. (2015) 206:308–15. doi: 10.1192/bjp.bp.114.153536
35. Pantoni, L, and Garcia, JH. The significance of cerebral white matter abnormalities 100 years after Binswanger's report. A review. Stroke. (1995) 26:1293–301. doi: 10.1161/01.STR.26.7.1293
36. Kern, KC, Wright, CB, Bergfield, KL, Fitzhugh, MC, Chen, K, Moeller, JR, et al. Blood pressure control in aging predicts cerebral atrophy related to small-vessel white matter lesions. Front Aging Neurosci. (2017) 9:132. doi: 10.3389/fnagi.2017.00132
37. Del, BA, Ciolli, L, Borgheresi, L, Poggesi, A, Inzitari, D, and Pantoni, L. Is type 2 diabetes related to leukoaraiosis? An updated review. Acta Neurol Scand. (2015) 132:147–55. doi: 10.1111/ane.12398
38. Yu, X, Wang, G, Zhan, J, Zhang, Z, Feng, T, and Xu, J. Risk factors of pure leukoaraiosis and the association with preclinical carotid atherosclerosis. Atherosclerosis. (2018) 275:328–32. doi: 10.1016/j.atherosclerosis.2018.06.869
Keywords: cerebral white matter lesions, prediction model, nomogram, elderly, male
Citation: Pei Y-F, Li X-D, Liu Q-Y, Zhang C-W, Wang Y-H, Chen M-R and Chen H-S (2024) A nomogram for predicting cerebral white matter lesions in elderly men. Front. Neurol. 15:1343654. doi: 10.3389/fneur.2024.1343654
Edited by:
Yuhua Fan, The First Affiliated Hospital of Sun Yat-sen University, ChinaReviewed by:
Alina Gonzalez-Quevedo, Instituto de Neurología y Neurocirugía, CubaRobert Jeenchen Chen, Stanford University, United States
Chanon Ngamsombat, Massachusetts General Hospital and Harvard Medical School, United States
Copyright © 2024 Pei, Li, Liu, Zhang, Wang, Chen and Chen. This is an open-access article distributed under the terms of the Creative Commons Attribution License (CC BY). The use, distribution or reproduction in other forums is permitted, provided the original author(s) and the copyright owner(s) are credited and that the original publication in this journal is cited, in accordance with accepted academic practice. No use, distribution or reproduction is permitted which does not comply with these terms.
*Correspondence: Hui-Sheng Chen, chszh@aliyun.com