Corrigendum: Digital neuropsychological measures by defense automated neurocognitive assessment: reference values and clinical correlates
- 1Department of Anatomy and Neurobiology, Boston University Chobanian & Avedisian School of Medicine, Boston, MA, United States
- 2The Framingham Heart Study, Boston University Chobanian & Avedisian School of Medicine, Boston, MA, United States
- 3Department of Neurology, Boston University Chobanian & Avedisian School of Medicine, Boston, MA, United States
- 4School of Public Health and Tropical Medicine, Tulane University, New Orleans, LA, United States
- 5Department of Epidemiology, Boston University School of Public Health, Boston, MA, United States
- 6Department of Medicine, Boston University Chobanian & Avedisian School of Medicine, Boston, MA, United States
- 7Department of Computer Science, Faculty of Computing & Data Sciences, Boston University, Boston, MA, United States
- 8Slone Epidemiology Center, Boston University Chobanian & Avedisian School of Medicine, Boston, MA, United States
- 9Department of Medicine, University of Massachusetts Chan Medical School, Worcester, MA, United States
Introduction: Although the growth of digital tools for cognitive health assessment, there’s a lack of known reference values and clinical implications for these digital methods. This study aims to establish reference values for digital neuropsychological measures obtained through the smartphone-based cognitive assessment application, Defense Automated Neurocognitive Assessment (DANA), and to identify clinical risk factors associated with these measures.
Methods: The sample included 932 cognitively intact participants from the Framingham Heart Study, who completed at least one DANA task. Participants were stratified into subgroups based on sex and three age groups. Reference values were established for digital cognitive assessments within each age group, divided by sex, at the 2.5th, 25th, 50th, 75th, and 97.5th percentile thresholds. To validate these values, 57 cognitively intact participants from Boston University Alzheimer’s Disease Research Center were included. Associations between 19 clinical risk factors and these digital neuropsychological measures were examined by a backward elimination strategy.
Results: Age- and sex-specific reference values were generated for three DANA tasks. Participants below 60 had median response times for the Go-No-Go task of 796 ms (men) and 823 ms (women), with age-related increases in both sexes. Validation cohort results mostly aligned with these references. Different tasks showed unique clinical correlations. For instance, response time in the Code Substitution task correlated positively with total cholesterol and diabetes, but negatively with high-density lipoprotein and low-density lipoprotein cholesterol levels, and triglycerides.
Discussion: This study established and validated reference values for digital neuropsychological measures of DANA in cognitively intact white participants, potentially improving their use in future clinical studies and practice.
1 Introduction
Alzheimer’s disease (AD) is a debilitating neurodegenerative disorder characterized by progressive cognitive decline. It is a major public health concern, affecting millions of individuals worldwide (1). Unfortunately, to date, there is no definitive cure or highly effective treatment for AD. Given the lack of effective therapeutic options, early detection of the disease is of paramount importance. Timely diagnosis allows for interventions and strategies that can potentially slow down disease progression and improve the quality of life for affected individuals. As such, there is a growing recognition in the scientific and medical communities of the critical need for accurate and early detection methods in the fight against AD.
Traditional neuropsychological (NP) testing has been the primary method for measuring cognitive function, but digital tools have emerged as a promising and convenient alternative (2). Among these tools, the Defense Automated Neurobehavioral Assessment (DANA) stands out as an innovative mobile application developed for the evaluation of neurobehavioral functioning in military personnel (3). DANA was conceptualized to address the pressing need for efficient, objective, and reliable assessment methods capable of monitoring cognitive performance and detecting subtle changes in neurobehavioral functioning over time. Its early deployments within military contexts have yielded valuable insights into the impact of stress-related mental health issues, including conditions such as post-traumatic stress disorder (4), post-concussive symptoms (5), and the effects of blast wave exposure resulting from the use of heavy weapon systems (6). However, DANA’s versatility quickly became apparent, leading to its adoption in a wide range of applications across clinical and research domains. Beyond its original military focus, DANA could be applied in diverse scenarios, including the assessment of traumatic brain injury (7), caregiver burden (8), hypoxic burden at high altitudes (9), and cognition changes in mental health treatments (10). The psychometric properties of the DANA test batteries have been thoroughly assessed and documented, confirming DANA as a reliable and valid tool for neuropsychological assessment (3, 11–14). The adaptability of DANA has transformed it from a specialized military tool into a versatile instrument capable of investigating a wide spectrum of neurocognitive aspects. This expanded utility underscores its significance in addressing various cognitive health-related challenges and outcomes across different clinical and research settings.
One notable challenge is the specialized expertise required to effectively interpret the digital neuropsychological measures obtained through tools like DANA. The outputs of digital tools typically comprise raw numerical values or complex algorithms. Consequently, it necessitates individuals well-versed in neurocognitive functioning and skilled in statistical analysis to extract meaningful insights from these results. This level of expertise is crucial to ensure accurate interpretation and prevent misinterpretation of the findings. Another challenge is the potential absence of established norms or benchmarks for these digital neuropsychological measures. In contrast to traditional neuropsychological tests, which benefit from established norms based on factors such as age and sex, digital neuropsychological measures often lack such reference values. This absence makes the interpretation of results and the establishment of benchmarks for future assessments a complex endeavor. As technology continues to advance and new assessment tools emerge, the development of standardized reference values may require time and concerted effort. Furthermore, the exploration of clinical risk factors linked to these digital neuropsychological measures remains largely unexplored within clinically characterized populations. The absence of established norms and clinical correlates can pose difficulties in determining the clinical implications of the digital measures obtained, emphasizing the importance of addressing this challenge as digital cognitive assessment tools become increasingly prevalent.
Therefore, the primary objective of this study is to establish reference values and investigate the association of clinical risk factors with digital neuropsychological measures derived from DANA in the Framingham Heart Study (FHS). This cohort has undergone a series of DANA tasks and comprehensive characterization of clinical risk factors. We further validated the reference values in participants from the Boston University Alzheimer’s Disease Research Center (BU ADRC).
2 Materials and methods
2.1 Study population
The FHS is a long-standing community-based prospective cohort study that initiated in 1948. Its primary objective is to identify risk factors of cardiovascular disease as well as dementia within the community. Remarkably, this study has successfully enrolled participants from three distinct generations (15), marking its enduring commitment to advancing our understanding of these critical health issues. In the FHS, cognitive status of participants is determined through a comprehensive dementia review process. This involves a specialized panel, consisting of a neurologist and a neuropsychologist, who meticulously evaluate each case of potential cognitive decline and dementia. Their assessment incorporates a variety of sources, including serial neurologic and neuropsychological evaluations, interviews with caregivers conducted over the phone, medical records, neuroimaging data, and autopsy results when available. The details of dementia review process can be referred to previous studies (16, 17). In this study, 932 cognitively intact white participants from the Framingham Heart Study, including the Offspring, Generation 3, and New Offspring Spouse sub-cohorts, formed the reference cohort.
Additionally, a secondary cohort of 57 cognitively intact white participants who have completed at least one assessment of DANA tasks was recruited from the BU ADRC. These participants were diagnosed based on the National Alzheimer’s Coordinating Center diagnostic procedures (18). Situated in the urban area of Boston, the BU ADRC focuses on older adults living in the community. It is one of 33 ADRCs funded by the National Institute on Aging. These centers contribute data to the National Alzheimer’s Coordinating Center with the aim of fostering collaborative research on AD. More detailed description of the BU ADRC is available in previous publications (19, 20).
2.2 Digital neuropsychological measures
This study utilized eight digital measures from three DANA tasks, including the Code Substitution task, the Go-No-Go task, and the Simple Reaction Time task. The Code Substitution task, gauges cognitive flexibility, reflecting how well an individual can transition between tasks or ideas. The Go-No-Go task evaluates inhibitory control, i.e., the ability to inhibit automatic or overlearned responses. The Simple Reaction Time task is a straightforward assessment of reaction speed, focusing on the response time to a given stimulus. For more information on these digital cognitive assessment methods, please refer to the study details (3). Participants will be asked to complete these tasks on a tablet provided to them by study staff during the in-clinic study visit. The details of study design and participation rate can be found in prior study (2). All touch screen and stylus responses will be recorded through the Linus Health app installed on the tablet. The tasks are composed of several trials during each session. Distinct files are produced for each task, encapsulating the various trials that make up one complete round of the cognitive assessment. The FHS and BU ADRC operate independent infrastructure pipelines while utilizing a shared database server, where data is stored in separate databases. For each task, through structured query language, data from individual trials is converted into aggregated examination datasets, with each dataset representing the mean value of the trials. This transformation includes recalculating metrics, conducting quality control, deriving extra metrics, and ensuring uniformity between the two cohorts. For each participant, the first examination of each DANA test was included in this study.
2.3 Statistical analyses
We derived reference values in the FHS cohort and stratified participants by sex and age groups: younger than 60 years old (<60), between 60 and 69 years (60–69), and aged 70 years or older (≥70). We employed t-tests to examine sex differences in digital neuropsychological measures. Additionally, one-way analysis of variance (ANOVA) was used to investigate the variations in these measures across different age groups. The reference values for each digital neuropsychological measure were defined as the median (2.5th and 97.5th percentiles) in each stratified group (21, 22). We also examined the association between digital cognitive measures and clinical factors by a backward elimination approach. These clinical factors included age, sex, body mass index, Mini-Mental State Examination (MMSE), smoking, hypertension treatment, systolic blood pressure (SBP), diastolic blood pressure (DBP), total cholesterol (TC), low-density lipoprotein cholesterol (LDL), high-density lipoprotein cholesterol (HDL), the TC to HDL ratio, blood glucose levels, triglyceride levels, diabetes treatment, as well as prevalent cardiovascular disease, myocardial infarction (MI), congestive heart failure (CHF), and stroke. Our analytical process initiated with the inclusion of all these clinical factors in the linear regression model. We then assessed the association of each factor with digital measures. Subsequently, we identified and removed the factor with the least significant association, followed by a reassessment of the relationships between the remaining clinical factors and digital measures. This stepwise elimination procedure was repeated until all remaining clinical factors achieved at least a nominal level of significance (p < 0.05). In all our models, we forced age and sex as covariates to account for their potential confounding effects on the observed associations.
3 Results
3.1 Cohort descriptive
In this study, we included 932 participants from the FHS as the reference cohort (mean age 63.0 ± 9.4 years, range 39.0–88.0 years, 57.3% women). Their clinical characteristics detailed in Table 1. Of these participants, 66.6% had received college education or higher. In addition, we included 57 individuals from BU ADRC as the validation cohort (mean age 74.5 ± 8.7 years, range 52.1–92.5 years, 64.9% women). Among them, 80.7% had a college education or higher.
3.2 Reference values of digital neuropsychological measures
We established reference values for digital neuropsychological measures across three DANA tasks. These values, including the upper (97.5th quantile), median (50th quantile), and lower (2.5th quantile) reference limits, have been categorized by three distinct age intervals for both men and women, as presented in Tables 2–4. Among participants aged under 60, we observed that in the Code Substitution task, the median (2.5th percentile, 97.5th percentile) average response time for all correct test trials (ART_correct) was 1,674 ms (1,147, 2,377) for men and 1,664 ms (1,188, 2,321) for women. Interestingly, in the Code Substitution task, women exhibited shorter reaction times, indicative of better cognitive function when compared to men within the same age group [t(930) = 2.14, p = 0.03]. However, men displayed quicker reaction times than women in the Go-No-Go [t(904) = 2.28, p = 0.02] and Simple Reaction Time tasks [t(450) = 1.99, p = 0.05]. Furthermore, our findings revealed that reaction times tend to slow down with increasing age (ANOVA, F = 178.77, p = 2.1 × 10−66), a phenomenon observed in both males (ANOVA, F = 91.75, p = 1.9 × 10−33) and females (ANOVA, F = 89.34, p = 3.6 × 10−34). These trends and age-related variations are illustrated in Figures 1–3, offering a comprehensive overview of the distribution of digital neuropsychological measures across different age groups in men and women. For a comprehensive summary of the median digital measures across the three DANA tasks, please refer to Supplementary Tables S1–S3. Furthermore, we categorized the participants into two education groups: those without a college degree and those with college or higher education. Significant differences were found in digital measures between these groups (t-test, all p < 0.05). The reference values of these two groups can be found in Supplementary Tables S4–S9. Skewness estimates corresponding to partitions in Tables 2–4 are provided in the Supplementary Table S10. Participants with college and higher degree have faster reaction time than the participants with no college degree. Figure 4 represents the proportion of participants from the BU ADRC validation cohort who fall within the normal range (between the 2.5th and 97.5th percentiles) of each digital cognitive measure. The heatmap is stratified by sex and age groups. While the reference values for some subgroups’ digital measures do not fully encompass the validation cohort, most of the measures are within the range of reference values.
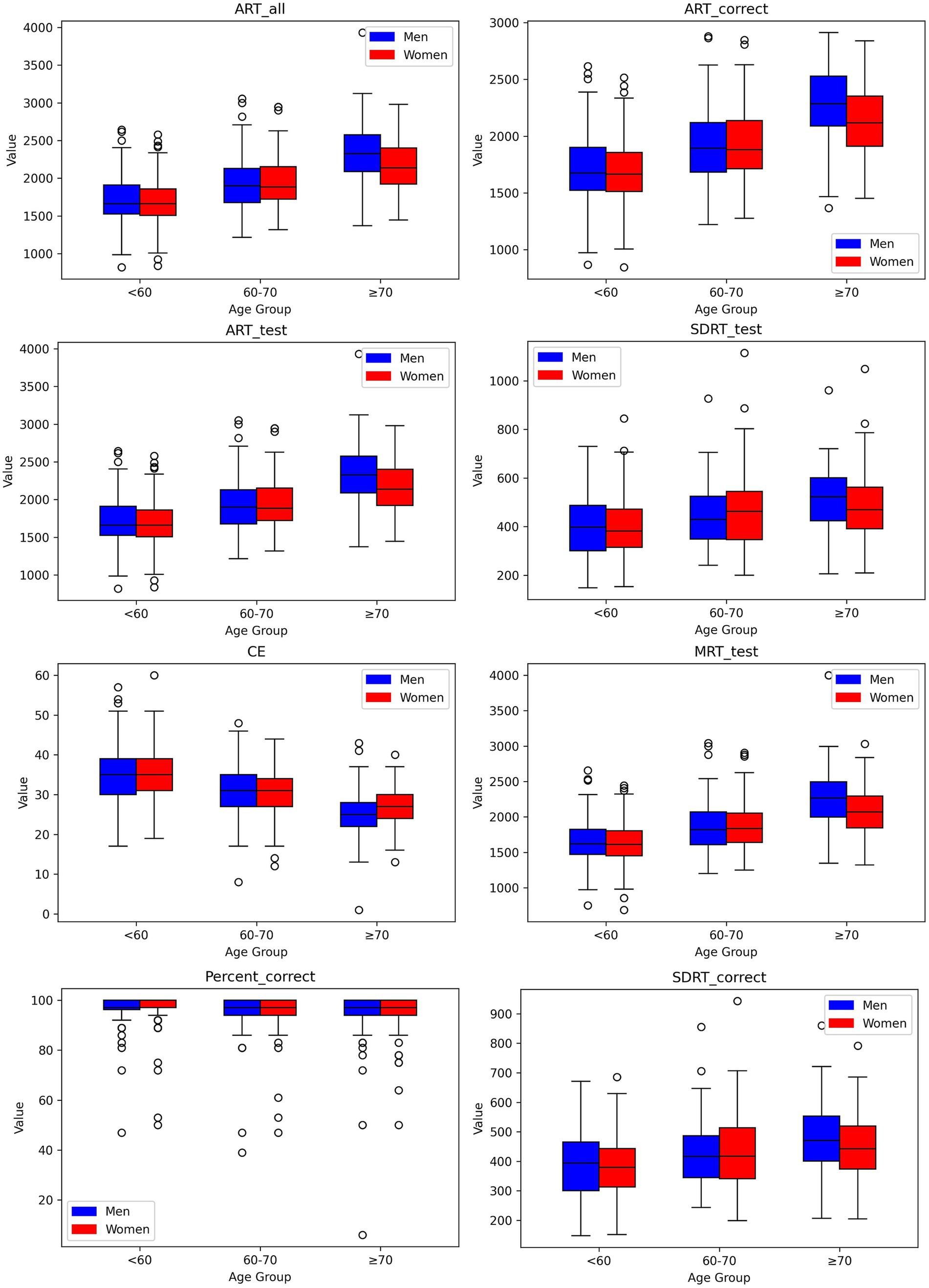
Figure 1. Box-and-whisker plots showing distributions of digital measures of Code Substitution task by age group for men and women. Each box encompasses the middle 50% of the dataset, specifically the second and third quartiles. The reference value (median) is indicated by the internal horizontal line. Whiskers extend from the boxes to the highest and lowest values within 1.5 times the IQR from the upper and lower quartiles, respectively.
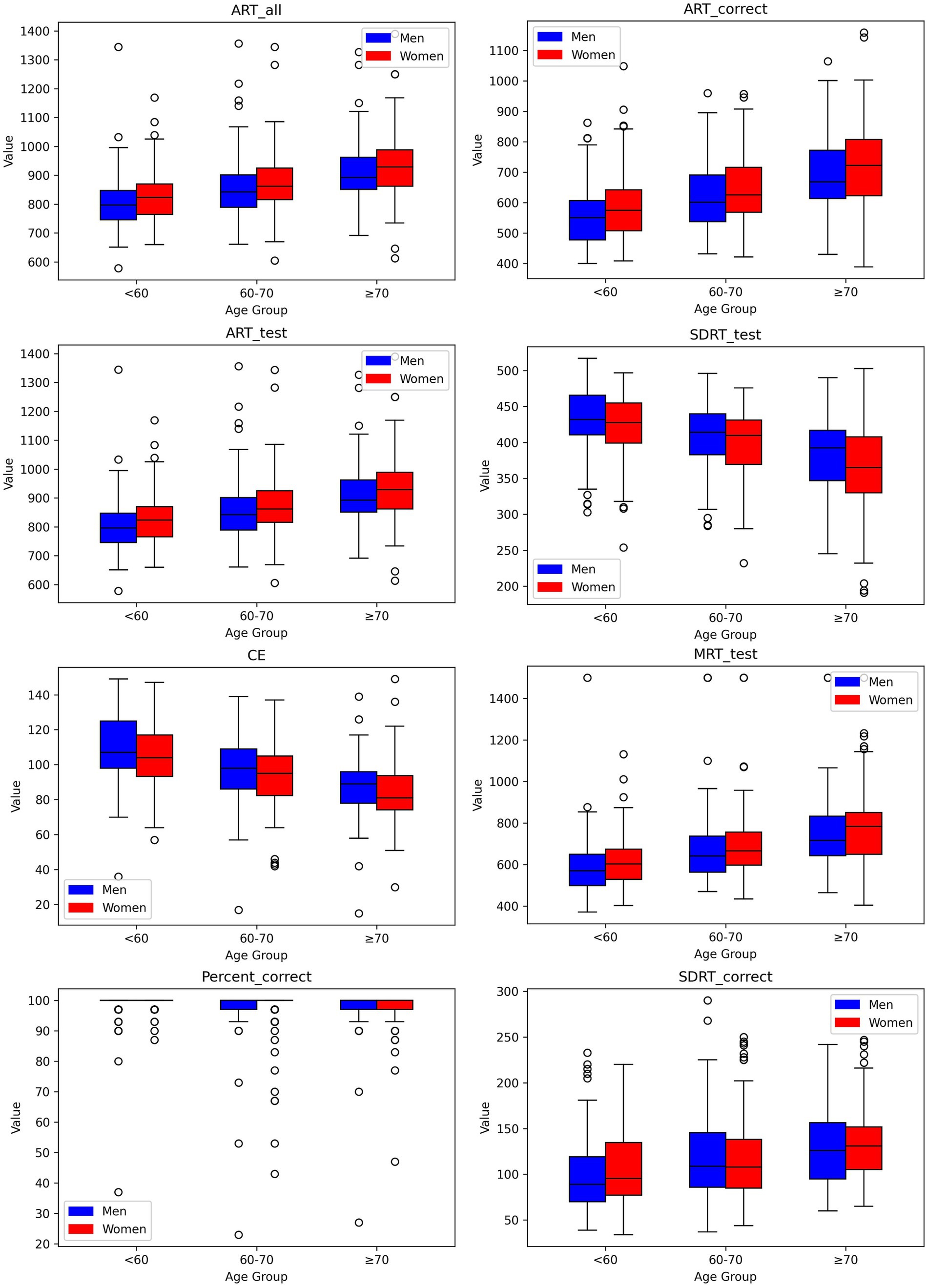
Figure 2. Box-and-whisker plots showing distributions of digital measures of Go-No-Go by age group for men and women. Each box encompasses the middle 50% of the dataset, specifically the second and third quartiles. The reference value (median) is indicated by the internal horizontal line. Whiskers extend from the boxes to the highest and lowest values within 1.5 times the IQR from the upper and lower quartiles, respectively.
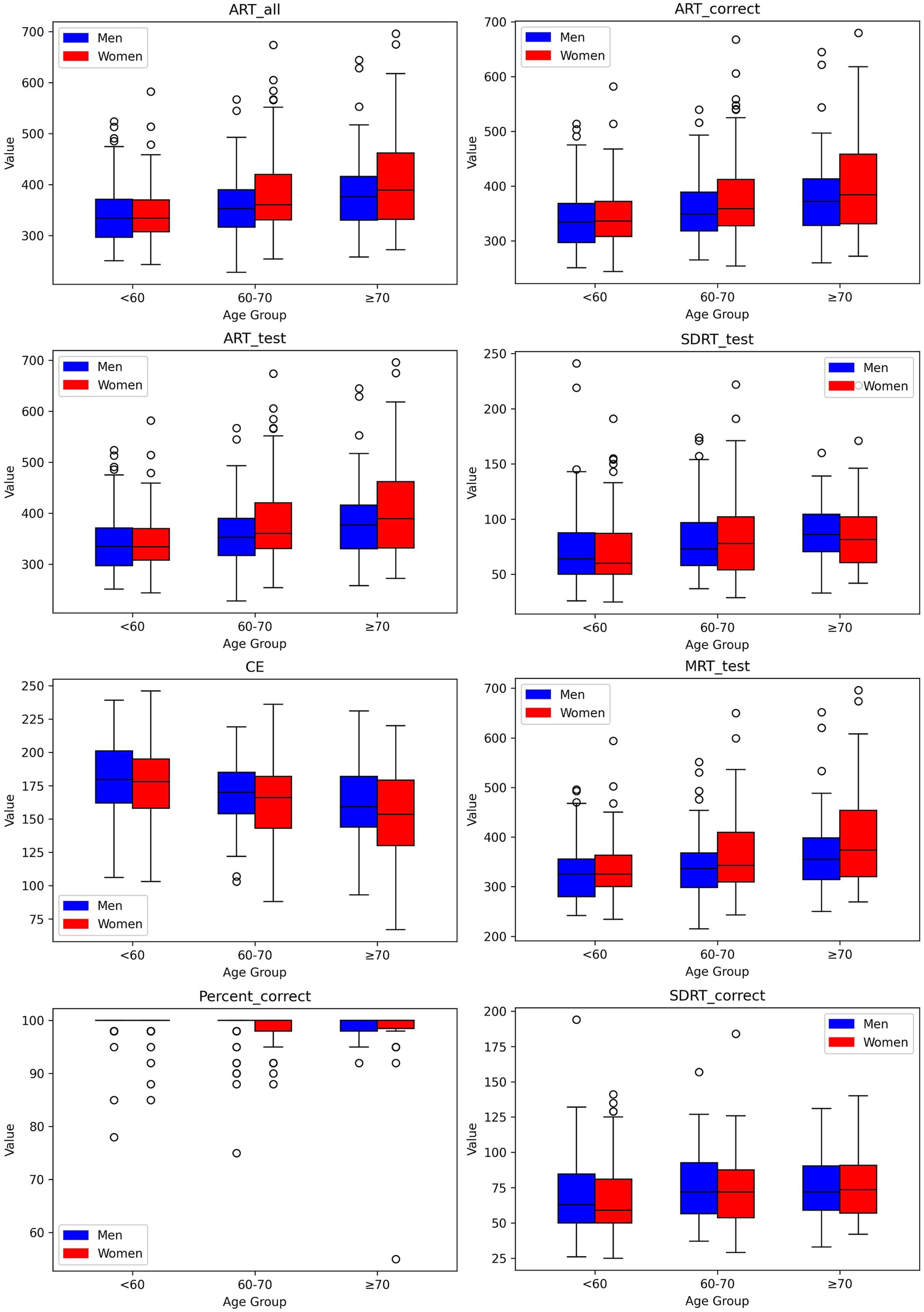
Figure 3. Box-and-whisker plots showing distributions of digital measures of Simple Reaction Time by age group for men and women. Each box encompasses the middle 50% of the dataset, specifically the second and third quartiles. The reference value (median) is indicated by the internal horizontal line. Whiskers extend from the boxes to the highest and lowest values within 1.5 times the IQR from the upper and lower quartiles, respectively.
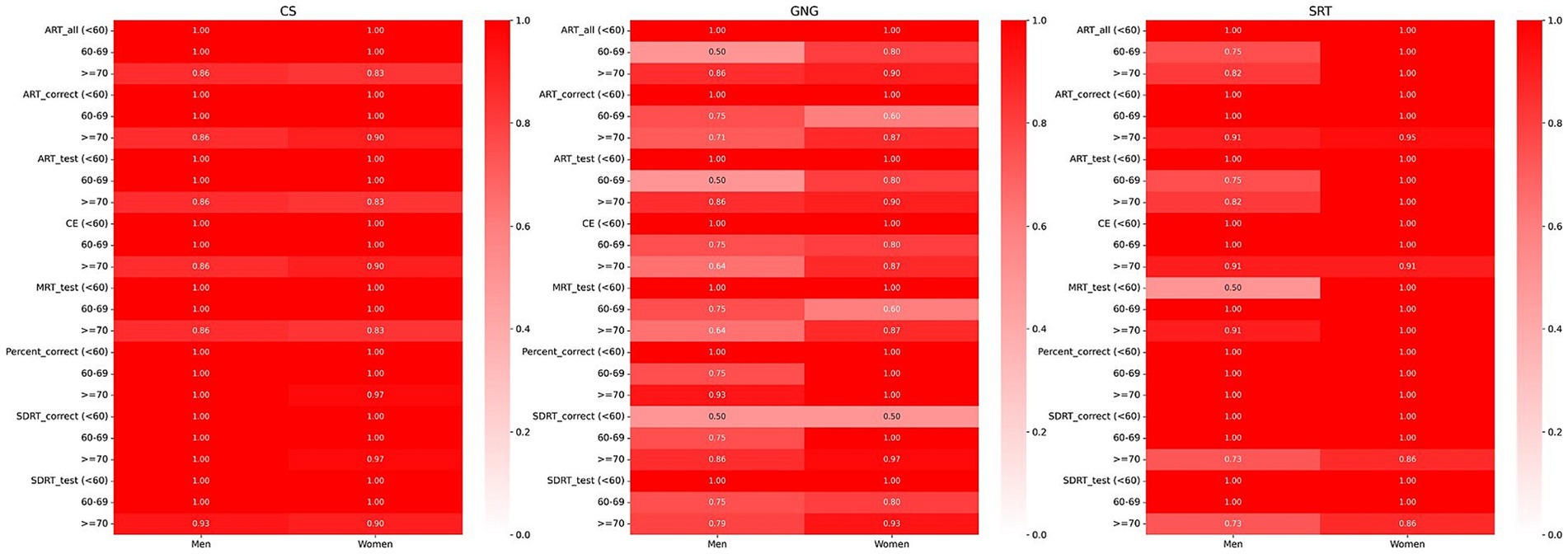
Figure 4. Heatmap illustrating the percentage of BU ADRC participants who scored within the normal range on digital measures, segmented by sex and age and confined to the 2.5th to 97.5th percentile range. A deep red color signifies that 100% (a proportion of 1) of the participants in that particular demographic and digital measures are within the normal range, set between the 2.5th and 97.5th percentiles. Conversely, a white color denotes a 0% proportion, meaning that none of the participants in the group fell within the normal range for that specific digital measure. Shades of lighter red indicate proportions between 0 and 1, reflecting the varying percentages of participants within the normal range.
3.3 Association of digital neuropsychological measures with clinical risk factors
The same digital cognitive measures observed across all three DANA tasks demonstrate distinct clinical correlates. Notably, for the average response time, it exhibited distinct associations within each task. In the Code Substitution task, the average response time for all correct test trials (ART_correct) was positively associated with diabetes and total cholesterol, and negatively associated with LDL, HDL, and triglycerides. Conversely, in Go-No-Go task, ART_correct was positively associated with blood glucose, and negatively associated with SBP and MMSE. Lastly, in the Simple Reaction Time task, it exhibited a positive association with DBP and the prevalence of MI and stroke, while negatively associated with the prevalence of CHF. In the Code Substitution task, three digital neuropsychological measures demonstrated significant associations with four or more clinical factors. The Go-No-Go task revealed that two digital measures were significantly linked to three clinical risk factors. Meanwhile, the Simple Reaction Time task had seven digital measures significantly associated with three or more clinical risk factors. For a comprehensive breakdown of the clinical factors significantly related to each digital neuropsychological measure within the three DANA tasks, refer to Tables 5–7. The model’s Akaike information criterion (AIC) value for each iteration is depicted in Supplementary Figures S1–S3.
4 Discussion
In this study, we established the reference values for digital neuropsychological measures for three DANA tasks in a large community-based cohort. These reference values were categorized by three age intervals, separately for men and women. Our findings provide new insights into the digital cognitive performance of individuals in different age groups.
Our results align with previous research in the field of cognitive assessment. Similar to our observations, studies have reported sex-based differences in cognitive function, where women often outperform men in tasks involving verbal memory and attention (23, 24), such as the Code Substitution task (3, 25). A possible explanation for this difference between sex is organization of memory, with is more bilateral in females, but left-lateralized in the male brain (26). It is suggested that women may have higher connectivity between social motivation, attention, and memory subnetworks (27). However, these gender differences tend to diminish or reverse in tasks requiring spatial processing and reaction time (28, 29), such as the Go-No-Go and Simple Reaction Time tasks (30, 31). It is proposed that males perform better in spatial processing or orientation tasks due to a preference for Euclidian strategies, or two-dimensional geometry (28). Other potential contributing factors include environmental context. For instance, males were more inclined to have greater familiarity with 3D computer simulations used to assess spatial processing, possibly owing to their more prevalent exposure to video games (28, 29). Furthermore, our study adds to the existing body of research by providing comprehensive age-specific reference values. These findings are consistent with prior investigations that have highlighted the impact of aging on cognitive performance (32, 33). The observed decline in reaction times with advancing age echoes the well-documented age-related cognitive changes seen in both sexes (34, 35).
Our study also identified clinical risk factors associated with digital neuropsychological measures across three DANA tasks, revealing noteworthy distinctions among them. Particularly, the average response time, a key neuropsychological measure, exhibited distinct clinical correlates within each task. Our findings regarding the associations between average response time and lipid profile (including total cholesterol) in Code Substitution task were in line with prior studies that have explored the relationship between cholesterol levels and cognitive function (36, 37). Notably, higher total cholesterol levels have been linked to cognitive impairment, emphasizing the importance of cardiovascular health in cognitive outcomes. The positive association between average response time and the prevalence of myocardial infarction and stroke in the Simple Reaction Time task underscores the intricate interplay between cardiovascular health and cognitive processing speed (38, 39). These findings aligned with studies emphasizing the impact of cardiovascular risk factors on cognitive function. Moreover, our analysis revealed that different digital cognitive measures were significantly associated with varying numbers of clinical risk factors across the three DANA tasks. These results emphasize the multifaceted nature of cognitive assessment and highlight the need for a comprehensive evaluation of clinical risk factors in understanding cognitive performance. In the Code Substitution task, there was a negative correlation observed between the average response time for all correct trials and levels of LDL and triglycerides. The relationship between LDL, triglycerides, and cognitive performance has yielded mixed outcomes in various studies. While several research findings indicate a link between high LDL cholesterol and a heightened risk of dementia or reduced cognitive function (40–43), other studies have reported contrary effects (44, 45), or found no conclusive correlation (46). In the case of triglycerides, some investigations suggest that elevated triglyceride levels correlate with cognitive decline (47) and diminished cognitive performance (48). However, other study has not established a connection with dementia or cognitive decline (49). These disparate findings suggest that there are various factors at play, including reverse causation and age effects, in the relationship between LDL, triglycerides, and cognitive function, pointing to the need for more comprehensive data in future research to unravel these mechanisms.
This study has several advantages. First, the study employed eight digital measures from each of the three DANA tasks in a large well-characterized cohort, offering a comprehensive digital assessment of participants’ decision-making and reaction times. These measures provided a detailed and multifaceted view of cognitive function, allowing for a more nuanced analysis. An additional cohort was used as an external validation cohort to validate the reference values. Second, a comprehensive list of clinical risk factors has been considered. The study utilized advanced statistical methods, including a backward elimination approach, to investigate clinical correlates. These robust analytical techniques enhance the accuracy and reliability of the study’s results.
We also acknowledge several limitations in this study. The study exclusively involved White participants due to the limited availability of DANA data on other ethnicities from the FHS and BU ADRC. Future efforts should aim to establish reference values for digital cognitive assessments across a more diverse range of ethnic groups. While the study categorizes participants into three age groups (<60, 60–69, and ≥70), this grouping may not capture more nuanced age-related cognitive changes. As more data becomes available, a finer age stratification could provide a more detailed insight of human performance on digital cognitive assessment. Our findings indicate that education level has an influence on individuals’ performance in DANA tasks. To establish reliable reference values stratified by education, further studies with larger sample sizes will be essential. While our research offers valuable insights into cognitive health assessments using the DANA tool, it is crucial to acknowledge that these measurements exclude assessments of episodic memory—a key cognitive domain impacted by AD. This limitation implies that while our findings contribute to understanding specific aspects of cognitive health, they may not comprehensively cover the cognitive landscape affected by AD. Regarding participant recruitment for our study, we extended invitations to all cognitively intact individuals within the FHS cohort. The essential criteria for participation included owning a smartphone, being proficient in English, and having WiFi access. We recognized that the decision to participate could be influenced by the participants’ cognitive and socioeconomic status. Those who chose to partake in the study may have had a relatively better cognitive condition, enabling them to engage with the study requirements. This scenario raises the possibility of selection bias, suggesting that our sample may not entirely represent the broader population of individuals with intact cognition.
In summary, this study provides valuable insights into digital neuropsychological measures, their reference values, and clinical correlates in well-established cohorts. These insights contribute to the growing body of knowledge in the field of digital cognitive assessment and offer valuable guidance for future research and clinical practice.
Data availability statement
The data analyzed in this study is subject to the following licenses/restrictions: the datasets analyzed for this study could be requested through a formal research application to the Framingham Heart Study. Requests to access these datasets should be directed to https://www.framinghamheartstudy.org/fhs-for-researchers/.
Ethics statement
The studies involving humans were approved by the Institutional Review Boards of Boston University Medical Center. The studies were conducted in accordance with the local legislation and institutional requirements. The participants provided their written informed consent to participate in this study.
Author contributions
HD: Conceptualization, Formal analysis, Investigation, Methodology, Project administration, Writing – original draft, Writing – review & editing. MK: Writing – review & editing. ES: Data curation, Software, Writing – review & editing. PS: Writing – review & editing. IA-D: Writing – review & editing. SL: Writing – review & editing. ZP: Writing – review & editing. PH: Writing – review & editing. ZL: Data curation, Writing – review & editing. KG: Writing – review & editing. LH: Writing – review & editing. JM: Writing – review & editing. SR: Writing – review & editing. AI: Writing – review & editing. VK: Writing – review & editing. RA: Writing – review & editing. HL: Conceptualization, Supervision, Writing – review & editing.
Funding
The author(s) declare financial support was received for the research, authorship, and/or publication of this article. This work was supported by the National Heart, Lung, and Blood Institute Contract (N01-HC-25195) and by grants from the National Institute on Aging AG-008122, AG-16495, AG-062109, AG-049810, AG-068753, AG054156, 2P30AG013846, P30AG073107, R01AG080670, U01AG068221 and from the National Institute of Neurological Disorders and Stroke, NS017950, and from the Alzheimer’s Association (Grant AARG-NTF-20-643020), and the American Heart Association (20SFRN35360180). It was also supported by Defense Advanced Research Projects Agency contract (FA8750-16-C-0299); Pfizer, Inc. The funding agencies had no role in study design, data collection and analysis, decision to publish, or preparation of the manuscript.
Acknowledgments
The authors acknowledge the FHS and BU ADRC participants for their dedication. This study would not be possible without them. The authors also thank the researchers in FHS and BU ADRC for their efforts over the years in the examination of subjects.
Conflict of interest
RA is a scientific advisor to Signant Health and NovoNordisk, and a consultant to Biogen and the Davos Alzheimer’s Collaborative; she also serves as Director of the Global Cohort Development program for the Davos Alzheimer’s Collaborative.
The remaining authors declare that the research was conducted in the absence of any commercial or financial relationships that could be construed as a potential conflict of interest.
Publisher’s note
All claims expressed in this article are solely those of the authors and do not necessarily represent those of their affiliated organizations, or those of the publisher, the editors and the reviewers. Any product that may be evaluated in this article, or claim that may be made by its manufacturer, is not guaranteed or endorsed by the publisher.
Author disclaimer
The views expressed in this manuscript are those of the authors and do not necessarily represent the views of the National Institutes of Health or the US Department of Health and Human Services or the US Department of Veterans Affairs.
Supplementary material
The Supplementary material for this article can be found online at: https://www.frontiersin.org/articles/10.3389/fneur.2024.1340710/full#supplementary-material
References
1. Nichols, E , Szoeke, CEI , Vollset, SE , Abbasi, N , Abd-Allah, F , Abdela, J, et al. Global, regional, and national burden of Alzheimer’s disease and other dementias, 1990–2016: a systematic analysis for the global burden of disease study 2016. Lancet Neurol. (2019) 18:88–106. doi: 10.1016/S1474-4422(18)30403-4
2. de Anda-Duran, I , Hwang, PH , Popp, ZT , Low, S , Ding, H , Rahman, S, et al. Matching science to reality: how to deploy a participant-driven digital brain health platform. Front Dement. (2023) 2:1135451. doi: 10.3389/frdem.2023.1135451
3. Lathan, C , Spira, JL , Bleiberg, J , Vice, J , and Tsao, JW . Defense automated neurobehavioral assessment (DANA)—psychometric properties of a new field-deployable neurocognitive assessment tool. Mil Med. (2013) 178:365–71. doi: 10.7205/MILMED-D-12-00438
4. Coffman, I , Resnick, HE , Drane, J , and Lathan, CE . Computerized cognitive testing norms in active-duty military personnel: potential for contamination by psychologically unhealthy individuals. Appl Neuropsychol Adult. (2018) 25:497–503. doi: 10.1080/23279095.2017.1330749
5. Spira, JL , Lathan, CE , Bleiberg, J , and Tsao, JW . The impact of multiple concussions on emotional distress, post-concussive symptoms, and neurocognitive functioning in active duty United States marines independent of combat exposure or emotional distress. J Neurotrauma. (2014) 31:1823–34. doi: 10.1089/neu.2014.3363
6. Skotak, M , LaValle, C , Misistia, A , Egnoto, MJ , Chandra, N , and Kamimori, G . Occupational blast wave exposure during multiday 0.50 caliber rifle course. Front Neurol. (2019) 10:797. doi: 10.3389/fneur.2019.00797
7. Nelson, LD , Furger, RE , Gikas, P , Lerner, EB , Barr, WB , Hammeke, TA, et al. Prospective, head-to-head study of three computerized neurocognitive assessment tools part 2: utility for assessment of mild traumatic brain injury in emergency department patients. J Int Neuropsychol Soc. (2017) 23:293–303. doi: 10.1017/S1355617717000157
8. Lathan, CE , Coffman, I , Sidel, S , and Alter, C . A pilot virtual case-management intervention for caregivers of persons with Alzheimer’s disease. J Hosp Manag Health Policy. (2018) 2:1–6. doi: 10.21037/jhmhp.2018.04.02
9. Roach, EB , Bleiberg, J , Lathan, CE , Wolpert, L , Tsao, JW , and Roach, RC . AltitudeOmics: decreased reaction time after high altitude cognitive testing is a sensitive metric of hypoxic impairment. Neuroreport. (2014) 25:814–8. doi: 10.1097/WNR.0000000000000169
10. Hollinger, KR , Woods, SR , Adams-Clark, A , Choi, SY , Franke, CL , Susukida, R, et al. Defense automated neurobehavioral assessment accurately measures cognition in patients undergoing electroconvulsive therapy for major depressive disorder. J ECT. (2018) 34:14–20. doi: 10.1097/YCT.0000000000000448
11. Russo, C , and Lathan, C . An evaluation of the consistency and reliability of the defense automated neurocognitive assessment tool. Appl Psychol Meas. (2015) 39:566–72. doi: 10.1177/0146621615577361
12. LaValle, CR , Carr, WS , Egnoto, MJ , Misistia, AC , Salib, JE , Ramos, AN, et al. Neurocognitive performance deficits related to immediate and acute blast overpressure exposure. Front Neurol. (2019) 10:949. doi: 10.3389/fneur.2019.00949
13. Haran, FJ , Dretsch, MN , and Bleiberg, J . Performance on the defense automated neurobehavioral assessment across controlled environmental conditions. Appl Neuropsychol Adult. (2016) 23:411–7. doi: 10.1080/23279095.2016.1166111
14. Kelly, MP , Coldren, RL , Parish, RV , Dretsch, MN , and Russell, ML . Assessment of acute concussion in the combat environment. Arch Clin Neuropsychol. (2012) 27:375–88. doi: 10.1093/arclin/acs036
15. Feinleib, M , Kannel, WB , Garrison, RJ , McNamara, PM , and Castelli, WP . The Framingham offspring study. Design and preliminary data. Prev Med. (1975) 4:518–25. doi: 10.1016/0091-7435(75)90037-7
16. Seshadri, S , Beiser, A , Au, R , Wolf, PA , Evans, DA , Wilson, RS, et al. Operationalizing diagnostic criteria for Alzheimer’s disease and other age-related cognitive impairment—part 2. Alzheimers Dement. (2011) 7:35–52. doi: 10.1016/j.jalz.2010.12.002
17. Satizabal, CL , Beiser, AS , Chouraki, V , Chêne, G , Dufouil, C , and Seshadri, S . Incidence of dementia over three decades in the Framingham heart study. N Engl J Med. (2016) 374:523–32. doi: 10.1056/NEJMoa1504327
18. Morris, JC , Weintraub, S , Chui, HC , Cummings, J , DeCarli, C , Ferris, S, et al. The uniform data set (UDS): clinical and cognitive variables and descriptive data from Alzheimer disease centers. Alzheimer Dis Assoc Disord. (2006) 20:210–6. doi: 10.1097/01.wad.0000213865.09806.92
19. Ashendorf, L , Jefferson, AL , Green, RC , and Stern, RA . Test-retest stability on the WRAT-3 reading subtest in geriatric cognitive evaluations. J Clin Exp Neuropsychol. (2009) 31:605–10. doi: 10.1080/13803390802375557
20. Gavett, BE , Lou, KR , Daneshvar, DH , Green, RC , Jefferson, AL , and Stern, RA . Diagnostic accuracy statistics for seven neuropsychological assessment battery (NAB) test variables in the diagnosis of Alzheimer’s disease. Appl Neuropsychol Adult. (2012) 19:108–15. doi: 10.1080/09084282.2011.643947
21. Kornej, J , Magnani, JW , Preis, SR , Soliman, EZ , Trinquart, L , Ko, D, et al. P-wave signal-averaged electrocardiography: reference values, clinical correlates, and heritability in the Framingham heart study. Heart Rhythm. (2021) 18:1500–7. doi: 10.1016/j.hrthm.2021.05.009
22. Cheng, S , Larson, MG , McCabe, E , Osypiuk, E , Lehman, BT , Stanchev, P, et al. Age-and sex-based reference limits and clinical correlates of myocardial strain and synchrony: the Framingham heart study. Circ Cardiovasc Imaging. (2013) 6:692–9. doi: 10.1161/CIRCIMAGING.112.000627
23. Loprinzi, PD , and Frith, E . The role of sex in memory function: considerations and recommendations in the context of exercise. J Clin Med. (2018) 7:132. doi: 10.3390/jcm7060132
24. Gur, RC , and Gur, RE . Complementarity of sex differences in brain and behavior: from laterality to multimodal neuroimaging. J Neurosci Res. (2017) 95:189–99. doi: 10.1002/jnr.23830
25. Pryweller, JR , Baughman, BC , Frasier, SD , O’Conor, EC , Pandhi, A , Wang, J, et al. Performance on the DANA brief cognitive test correlates with MACE cognitive score and may be a new tool to diagnose concussion. Front Neurol. (2020) 11:839. doi: 10.3389/fneur.2020.00839
26. Kimura, D . Sex differences in cerebral organization for speech and praxic functions. Can J Psychol. (1983) 37:19–35. doi: 10.1037/h0080696
27. Tunç, B , Solmaz, B , Parker, D , Satterthwaite, TD , Elliott, MA , Calkins, ME, et al. Establishing a link between sex-related differences in the structural connectome and behaviour. Philos Trans R Soc B. (2016) 371:20150111. doi: 10.1098/rstb.2015.0111
28. Coluccia, E , and Louse, G . Gender differences in spatial orientation: a review. J Environ Psychol. (2004) 24:329–40. doi: 10.1016/j.jenvp.2004.08.006
29. Clements-Stephens, AM , Rimrodt, SL , and Cutting, LE . Developmental sex differences in basic visuospatial processing: differences in strategy use? Neurosci Lett. (2009) 449:155–60. doi: 10.1016/j.neulet.2008.10.094
30. Otaki, M , and Shibata, K . The effect of different visual stimuli on reaction times: a performance comparison of young and middle-aged people. J Phys Ther Sci. (2019) 31:250–4. doi: 10.1589/jpts.31.250
31. Miller, J , Schäffer, R , and Hackley, SA . Effects of preliminary information in a go versus no-go task. Acta Psychol. (1991) 76:241–92. doi: 10.1016/0001-6918(91)90022-R
32. Murman, DL . The impact of age on cognition. Semin Hear. (2015) 36:111–21. doi: 10.1055/s-0035-1555115
33. Salthouse, TA . When does age-related cognitive decline begin? Neurobiol Aging. (2009) 30:507–14. doi: 10.1016/j.neurobiolaging.2008.09.023
34. Reas, ET , Laughlin, GA , Bergstrom, J , Kritz-Silverstein, D , Barrett-Connor, E , and McEvoy, LK . Effects of sex and education on cognitive change over a 27-year period in older adults: the Rancho Bernardo Study. Am J Geriatr Psychiatry. (2017) 25:889–99. doi: 10.1016/j.jagp.2017.03.008
35. Graves, WW , Coulanges, L , Levinson, H , Boukrina, O , and Conant, LL . Neural effects of gender and age interact in reading. Front Neurosci. (2019) 13:1115. doi: 10.3389/fnins.2019.01115
36. Stough, C , Pipingas, A , Camfield, D , Nolidin, K , Savage, K , Deleuil, S, et al. Increases in total cholesterol and low density lipoprotein associated with decreased cognitive performance in healthy elderly adults. Metab Brain Dis. (2019) 34:477–84. doi: 10.1007/s11011-018-0373-5
37. Yu, Y , Yan, P , Cheng, G , Liu, D , Xu, L , Yang, M, et al. Correlation between serum lipid profiles and cognitive impairment in old age: a cross-sectional study. Gen Psychiatr. (2023) 36:e101009. doi: 10.1136/gpsych-2023-101009
38. Leritz, EC , McGlinchey, RE , Kellison, I , Rudolph, JL , and Milberg, WP . Cardiovascular disease risk factors and cognition in the elderly. Curr Cardiovasc Risk Rep. (2011) 5:407–12. doi: 10.1007/s12170-011-0189-x
39. Ginty, AT , Phillips, AC , der, G , Deary, IJ , and Carroll, D . Cognitive ability and simple reaction time predict cardiac reactivity in the west of Scotland Twenty-07 study. Psychophysiology. (2011) 48:1022–7. doi: 10.1111/j.1469-8986.2010.01164.x
40. Helzner, EP , Luchsinger, JA , Scarmeas, N , Cosentino, S , Brickman, AM , Glymour, MM, et al. Contribution of vascular risk factors to the progression in Alzheimer disease. Arch Neurol. (2009) 66:343–8. doi: 10.1001/archneur.66.3.343
41. Lesser, GT , Haroutunian, V , Purohit, DP , Schnaider Beeri, M , Schmeidler, J , Honkanen, L, et al. Serum lipids are related to Alzheimer’s pathology in nursing home residents. Dement Geriatr Cogn Disord. (2009) 27:42–9. doi: 10.1159/000189268
42. Moroney, JT , Tang, MX , Berglund, L , Small, S , Merchant, C , Bell, K, et al. Low-density lipoprotein cholesterol and the risk of dementia with stroke. JAMA. (1999) 282:254–60. doi: 10.1001/jama.282.3.254
43. Yaffe, K , Barrett-Connor, E , Lin, F , and Grady, D . Serum lipoprotein levels, statin use, and cognitive function in older women. Arch Neurol. (2002) 59:378–84. doi: 10.1001/archneur.59.3.378
44. Henderson, V , Guthrie, J , and Dennerstein, L . Serum lipids and memory in a population based cohort of middle age women. J Neurol Neurosurg Psychiatry. (2003) 74:1530–5. doi: 10.1136/jnnp.74.11.1530
45. Packard, CJ , Westendorp, RGJ , Stott, DJ , Caslake, MJ , Murray, HM , Shepherd, J, et al. Association between apolipoprotein E4 and cognitive decline in elderly adults. J Am Geriatr Soc. (2007) 55:1777–85. doi: 10.1111/j.1532-5415.2007.01415.x
46. Reitz, C , Tang, MX , Luchsinger, J , and Mayeux, R . Relation of plasma lipids to Alzheimer disease and vascular dementia. Arch Neurol. (2004) 61:705–14. doi: 10.1001/archneur.61.5.705
47. de Frias, CM , Bunce, D , Wahlin, A , Adolfsson, R , Sleegers, K , Cruts, M, et al. Cholesterol and triglycerides moderate the effect of apolipoprotein E on memory functioning in older adults. J Gerontol B. (2007) 62:P112–8. doi: 10.1093/geronb/62.2.P112
48. Sims, R , Madhere, S , Callender, C , and Campbell, A Jr. Patterns of relationships between cardiovascular disease risk factors and neurocognitive function in African Americans. Ethn Dis. (2008) 18:471–6.
Keywords: cognitive health, defense automated neurocognitive assessment, digital neuropsychological measures, reference values, clinical correlates
Citation: Ding H, Kim M, Searls E, Sunderaraman P, De Anda-Duran I, Low S, Popp Z, Hwang PH, Li Z, Goyal K, Hathaway L, Monteverde J, Rahman S, Igwe A, Kolachalama VB, Au R and Lin H (2024) Digital neuropsychological measures by defense automated neurocognitive assessment: reference values and clinical correlates. Front. Neurol. 15:1340710. doi: 10.3389/fneur.2024.1340710
Edited by:
Jennifer S. Yokoyama, University of San Francisco, United StatesReviewed by:
Emily W. Paolillo, University of California, San Francisco, United StatesHiroko H. Dodge, Massachusetts General Hospital and Harvard Medical School, United States
Copyright © 2024 Ding, Kim, Searls, Sunderaraman, De Anda-Duran, Low, Popp, Hwang, Li, Goyal, Hathaway, Monteverde, Rahman, Igwe, Kolachalama, Au and Lin. This is an open-access article distributed under the terms of the Creative Commons Attribution License (CC BY). The use, distribution or reproduction in other forums is permitted, provided the original author(s) and the copyright owner(s) are credited and that the original publication in this journal is cited, in accordance with accepted academic practice. No use, distribution or reproduction is permitted which does not comply with these terms.
*Correspondence: Honghuang Lin, aG9uZ2h1YW5nLmxpbkB1bWFzc21lZC5lZHU=