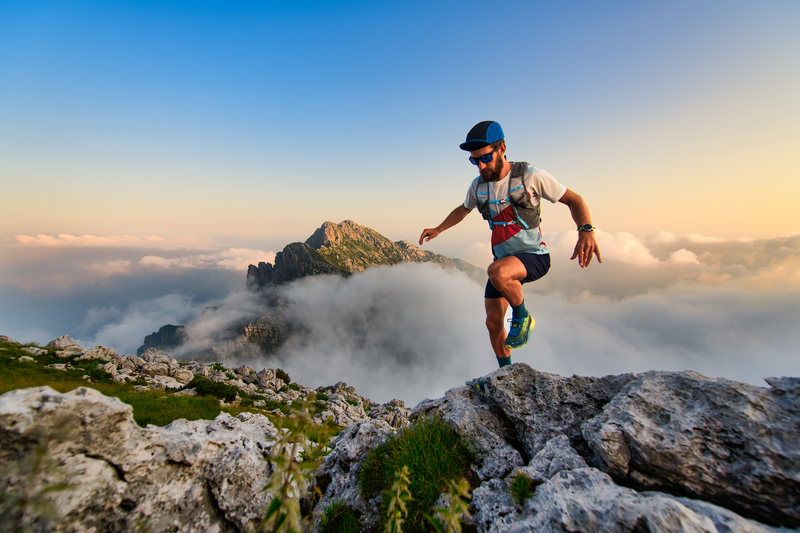
95% of researchers rate our articles as excellent or good
Learn more about the work of our research integrity team to safeguard the quality of each article we publish.
Find out more
ORIGINAL RESEARCH article
Front. Neurol. , 16 February 2024
Sec. Stroke
Volume 15 - 2024 | https://doi.org/10.3389/fneur.2024.1323878
This article is part of the Research Topic Vascular Immunity and Ischemic Stroke View all 15 articles
Objective: Prolonged sleep onset latency (PSOL) and age have been linked to ischemic stroke (IS) severity and the production of chemokines and inflammation, both of which contribute to IS development. This study aimed to explore the relationship between chemokines, inflammation, and the interplay between sleep onset latency (SOL) and age in influencing stroke severity.
Methods: A cohort of 281 participants with mild to moderate IS was enrolled. Stroke severity was assessed using the National Institutes of Health Stroke Scale (NIHSS), and SOL was recorded. Serum levels of macrophage inflammatory protein-1alpha (MIP-1α), macrophage inflammatory protein-1beta (MIP-1β), monocyte chemoattractant protein-1 (MCP-1), interleukin-6 (IL-6), and tumor necrosis factor-alpha (TNF-α) were measured.
Results: NIHSS scores of middle-aged participants with PSOL were significantly higher than those with normal sleep onset latency (NSOL) (p = 0.046). This difference was also observed when compared to both the elderly with NSOL (p = 0.022), and PSOL (p < 0.001). Among middle-aged adults with PSOL, MIP-1β exhibited a protective effect on NIHSS scores (β = −0.01, t = −2.11, p = 0.039, R2 = 0.13). MIP-1α demonstrated a protective effect on NIHSS scores in the elderly with NSOL (β = −0.03, t = −2.27, p = 0.027, R2 = 0.12).
Conclusion: This study reveals a hitherto undocumented association between PSOL and IS severity, along with the potential protective effects of MIP-1β in mitigating stroke severity, especially among middle-aged patients.
Stroke is considered the second leading cause of death worldwide and remains a significant cause of disability in both developed and developing countries (1). Ischemic stroke accounts for almost 70% of all stroke cases (1). There is a rich literature available substantiating that prolonged sleep onset latency (PSOL) and age can determine stroke severity (2–4). However, the precise mechanisms by which PSOL and age impact the development of ischemic stroke (IS) remain incompletely understood.
Sleep onset latency (SOL) is the amount of time it takes a person to fall asleep in bed, and represents an important marker for assessing sleep quality (5). PSOL is one of the main manifestations of sleep structural changes in ischemic stroke (6). Studies have demonstrated a positive correlation between PSOL and the prevalence of stroke; short SOL was associated with a 36% reduction in the risk of stroke, while PSOL was also related to the severity of IS symptoms (7), suggesting that shorter SOL may protect against stroke (2). In addition, it has been shown that sleep onset latency is prolonged with aging (8), and aging is a significant factor affecting stroke (3). Besides, stroke tends to occur predominantly in the elderly, and its incidence and severity are closely related to age (3, 9, 10). Consistently, a study revealed that the severity of strokes tended to increase with increasing age (10). In contrast, a growing body of evidence suggests the “younger stroke” phenomenon is gaining prominence as a pressing public health issue, marked by a rising occurrence of strokes among individuals considered “younger” (those under 50 years of age) (11, 12). Consequently, SOL and age have been established as risk factors for ischemic stroke. Nonetheless, the underlying pathophysiological mechanisms governing their interplay in influencing the severity of IS remain uncertain.
Prolonged sleep onset latency can lead to a series of sleep-related issues that exacerbate stroke severity by triggering a systemic inflammatory response (13–16). In addition, prior investigations have shown that PSOL is exacerbated with age (17). However, recent research has indicated prolonged sleep onset latency even among middle-aged individuals, indicating a close relationship between SOL and age (18). Interestingly, age also impacts stroke severity through its influence on inflammation (19). Chemokines, as small molecular proteins, play a crucial role in the immune and inflammatory responses after stroke, which are involved in the processing of neovascularization, neurogenesis, and neural network reconstruction (20). Chemokines are cytokines attracting selective leukocyte subsets and subgrouping into the four major subfamilies, CC, CXC, C, and CX3C. macrophage inflammatory protein-1 alpha (MIP-1α), macrophage inflammatory protein 1beta (MIP-1β), and monocyte chemoattractant protein-1 (MCP-1) are the three best-known and most extensively studied CC chemokines in primary and secondary inflammatory responses in humans (21, 22). An increasing body of literature suggests that chemokines and cytokines, such as high levels of MIP-1α, MIP-1β, MCP-1, interleukin-6 (IL-6) and tumor necrosis factor-alpha (TNF-α) are associated with poor subjective sleep quality characterized by PSOL (23–27). In this respect, animal experiments have demonstrated that older mice exhibit notably higher MIP-1α and MIP-1β levels than their younger counterparts (28), which indicate that the cytokines and chemokines are also closely related to age. The overexpression of chemokines MIP-1α and MCP-1 can promote the recruitment of inflammatory cytokines IL-6 and TNF-α (29), and the recruitment of pro-inflammatory factors accelerates the development of atherosclerotic plaques, which further aggravates blood–brain barrier injury (30) and leads to brain injury. The chemokine-induced inflammatory response is pivotal in exacerbating stroke outcomes (30, 31). These cumulative factors collectively contribute to the heightened severity of ischemic stroke (32, 33). In essence, the combined influence of sleep onset latency and age on these cytokines may provide insights into explaining the underlying pathophysiology of ischemic stroke.
As described above, most studies have shown that SOL and age are independently correlated with stroke severity (2, 3, 7), and these cytokines played roles in the severity of IS. However, the association between chemokines, inflammation, and the interaction of SOL and age with stroke severity remains elusive, yet it holds crucial significance for preventing ischemic stroke. Therefore, this study aimed to examine how the interplay between sleep onset latency and age impacts chemokine levels and inflammation, with a subsequent exploration of their combined role in determining the severity of strokes.
A total of 281 participants with mild and moderate ischemic stroke admitted to Sinopharm North Hospital from June 2020 to December 2021 were recruited.
Sociodemographic data, such as age, years of education, occupation, and current body mass index (BMI), were collected. Clinical data, such as a history of substance abuse and dependence, were obtained according to medical records and self-reports and confirmed by the next of kin and family members. Data on SOL in the 1–3 months before stroke were collected by self-assessment and report.
The following criteria were used for participant inclusion: individuals aged 45–80 diagnosed with mild and moderate ischemic stroke based on clinical symptoms, physical examination, and imaging findings. Participants with a history of working night shifts, diagnosed with severe stenosis of the internal carotid artery, external carotid artery, subclavian artery, and vertebral artery as evident by cranial MRA and vascular color ultrasound, individuals diagnosed with tumors, those experiencing significant and persistent sleep problems along with diagnosed sleep disorders, or those taking medications and healthcare products known to affect sleep patterns were excluded. In addition, participants with severe and very severe ischemic stroke were excluded due to the high prevalence of altered consciousness, such as coma, which would hinder the accurate assessment of sleep patterns. The exclusion criteria also included a history of any substance abuse or dependence, as well as any neurological and psychiatric disorders diagnosed by the Statistical Manual of Mental Disorders-V (DSM-V).
The present study was approved by the Institutional Review Board of the Sinopharm North Hospital (Approval number: GYBFYY-LL-2020006) and was performed in accordance with the Declaration of Helsinki, and written informed consent was obtained. No financial compensation was provided to the subjects in this study.
The National Institutes of Health Stroke Scale (NIHSS) contains 15 items, a reliable, valid, and responsive tool for measuring stroke severity (34). The NIHSS includes the following domains: level of consciousness, eye movements, integrity of visual fields, facial movements, arm and leg muscle strength, sensation, coordination, language, speech, and neglect. Each impairment is scored on an ordinal scale ranging from 0 to 2, 0 to 3, or 0 to 4. The cumulative scores yield a total ranging from 0 to 42, with higher scores indicating more severe strokes (35). Stroke severity was categorized as follows: mild (NIHSS score 0–5), moderate (NIHSS score 6–14), severe (NIHSS score 15–24), and very severe (NIHSS score 25) (36, 37).
Recognizing that sleep onset latencies exceeding 30 min are associated with sleep difficulties in middle-aged and older adults (38), the present study categorized participants based on this established criterion (38). Participants with a sleep onset latency of more than 30 min were grouped as the PSOL group (n = 153), and those who had an SOL of 30 min or less constituted the normal sleep onset latency (NOSL) group (n = 127).
High-density lipoprotein (HDL), low-density lipoprotein (LDL), total cholesterol (TC), and triglyceride (TG) levels were obtained from routine tests to assess the participants’ physical condition in relation to ischemic stroke. SOL data and NIHSS scores were collected after peripheral metabolic markers were measured on the first day of admission. Participants were admitted to the hospital either on the day of the onset of physical symptoms or the next day.
Peripheral blood samples were obtained upon admission. The serum was separated and immediately frozen at −80°C. Analyses were performed to measure the serum levels of MIP1α, MIP1β, MCP1, IL-6, and TNFα using ELISA kits (Shanghai Xinle Biotechnology Co., LTD, Shanghai, China). Laboratory technicians conducting the analyses were blinded to clinical data.
Data were presented as mean ± standard deviation (SD) for continuous variables and as frequencies and percentages for categorical variables. The comparison of categorical variables was performed by the chi-squared test. The normality of all variables was assessed using the Shapiro–Wilk test. Levene’s test verified the homoscedasticity of residual variances, confirming the equal distribution of residuals (all p > 0.05). As a result, an analysis of covariance (ANCOVA) was employed to compare differences in inflammatory markers between groups (see Table 1). Partial correlation analysis was used to examine the correlation between inflammatory markers and NIHSS scores.
In addition, general linear models (GLMs) were applied to test the significance of the interaction between SOL and age and their effect on NIHSS scores. Current BMI was included as a covariate in all models. Model comparisons and testing were carried out using an F-statistic.
All statistical analyses were performed using IBM SPSS Statistics for Windows, Version 22.0 (IBM Corp., Armonk, NY, United States). Figures were generated using GraphPad Prism version 8 (GraphPad Software Inc.). All tests were two-sided, and the significance threshold was set at p < 0.05.
An ANCOVA was conducted with BMI as the covariate to identify disparities in sociodemographic, clinical variables, and inflammatory markers across various groups (Table 1). In contrast to participants with NSOL, those with PSOL exhibited a higher proportion of females (50.7% vs. 27.1%, p < 0.001). Participants in the PSOL group reported lower rates of smoking than those in the NSOL group (39.5% vs. 60.5%, p < 0.001), while no difference was observed in other sociodemographic and clinical characteristics between both groups.
The participants were divided into age groups [Middle-aged (aged 45–65) and Elderly (aged 65+)] and presence of PSOL/NSOL which resulted in four distinct groups:Middle-aged with PSOL (n = 62), Middle-aged with NSOL (n = 59), Elderly with PSOL (n = 91), and Elderly with NSOL (n = 68).
The homogeneity of variance for the NIHSS scores variable, determined through Levene’s test, yielded a p-value greater than 0.05. Thus, ANCOVA was employed to compare differences in NIHSS scores between the groups. Taking current BMI as the covariate, the impacts of SOL and age on NIHSS scores were found to be significant (F = 6.51, p = 0.011). In this regard, the NIHSS scores in the Middle-aged with PSOL group were notably higher than those in both the Elderly with NSOL group and the Elderly with PSOL group (p = 0.015 and p < 0.001, respectively).
To explore potential interactions between SOL and age in relation to stroke severity, GLM analyses of NIHSS scores were performed while controlling for current BMI. GLM analysis revealed strong interactions for NIHSS scores between SOL and age within the dataset. Notably, the NIHSS scores of participants in the Middle-aged with PSOL group were significantly higher compared to the Middle-aged with NSOL group (p = 0.046). Furthermore, the NIHSS scores of participants in the Middle-aged with PSOL group were significantly elevated compared to those in both the Elderly with NSOL group (p = 0.022) and the Elderly with PSOL group (p < 0.001; Table 2, Figure 1).
Figure 1. The difference in National Institutes of Health Stroke Scale (NIHSS) scores between groups. (A) The differences among the NIHSS scores of the participants in Middle-aged with prolonged sleep onset latency (PSOL) and Middle-aged with normal sleep onset latency (NSOL); (B) The differences among the NIHSS scores of the participants in Middle-aged with PSOL and Elderly with NSOL; (C) The differences among the NIHSS scores of the participants in Middle-aged with PSOL and Elderly with PSOL. *p < 0.05.
After adjusting for current BMI, a partial correlation analysis was conducted to assess the relationship between NIHSS scores and inflammatory markers within each group. Notably, a negative correlation was observed between MIP-1β levels and NIHSS scores in the Middle-aged with PSOL group (r = −0.30, p = 0.020). Similarly, a negative correlation was found between MIP-1α levels and NIHSS scores in the Elderly with NSOL group (r = −0.27, p = 0.029; Table 3, Figure 2).
Figure 2. The correlation of MIP-1β levels and MIP-1α levels with NIHSS scores. (A) The negative correlation of macrophage inflammatory protein 1beta (MIP-1β) levels with the NIHSS scores (r = −0.30, p = 0.020) in group of middle-aged with PSOL; (B) The negative correlation of macrophage inflammatory protein-1 alpha (MIP-1α) levels with the NIHSS Scores (r = −0.27, p = 0.029) in the group of Elderly with NSOL.
A hierarchical stepwise linear regression analysis revealed noteworthy findings, with BMI as the initial covariate and NIHSS score as the dependent variable. MIP-1β levels emerged as a protective factor for NIHSS scores in Middle-aged adults with PSOL (β = −0.01, 95%CI [−0.01 ~ 0.00], t = −2.11, p = 0.039, R2 = 0.13). Additionally, MIP-1α levels were identified as a protective factor for NIHSS scores in the Elderly with NSOL group (β = −0.03, 95%CI [−0.05 ~ 0.00], t = −2.27, p = 0.027, R2 = 0.12).
This pioneering study aims to shed light on the hitherto underexplored relationship between sleep onset latency, age, and stroke severity by investigating the pathophysiological mechanisms potentially driving this association. Importantly, we substantiated the association between PSOL and the severity in middle-aged IS participants, with higher NIHSS scores associated with PSOL and lower levels of MIP-1β.
Our findings suggest that middle-aged stroke participants with PSOL are at greater risk of experiencing a severe stroke, and MIP-1β plays a protective role against IS. Over the years, studies have emphasized that high-risk factors for stroke occurrence (39) and increased stroke severity (5, 10, 40) include sleep difficulties and advanced age. Notably, while stroke has conventionally been linked to older age, recent years have witnessed a substantial decline in the average age of stroke onset, coupled with a rise in stroke incidence and hospitalization rates among middle-aged individuals. This phenomenon of “younger-age stroke” has emerged as a significant public health challenge (12, 41–43), consistent with the results of this study. Indeed, middle-aged people with PSOL face an elevated risk of more severe strokes, possibly attributable to several factors. Firstly, compared to older individuals, middle-aged individuals necessitate efficient and higher sleep quality to sustain bodily functions and metabolism (44–46). Hence, when middle-aged stroke patients with PSOL experience a range of sleep-related issues such as diminished sleep quality, insomnia, and inadequate sleep (15), their sleep requirements are unmet, significantly impeding the recovery from cerebral ischemia-induced reversible or irreversible synaptic and membrane failures, which, influences neuroplasticity and post-stroke recovery (47). Secondly, older individuals usually have more flexible morning routines due to retirement, alleviating the impact of PSOL-related sleep shortage (18). Conversely, middle-aged individuals contend with heightened work pressures, constrained wake-up times and are more prone to insufficient sleep and subpar sleep quality (18). Moreover, middle-aged individuals tend to engage in more social activities, potentially adopting unhealthy lifestyles like high-calorie diets, smoking, and alcohol consumption (48). Besides, the compounded effects of sleep deprivation, stress, and unhealthy habits are widely acknowledged to exacerbate stroke severity (49–51).
In addition, this study found that MIP-1β was negatively associated with NIHSS scores in the middle-aged group with PSOL, indicating that elevated levels of MIP-1β could protect against severe strokes in this cohort. Previous research has indicated the potential involvement of MIP-1β in monocyte recruitment within atherosclerotic plaques, where heightened serum MIP-1β levels have been associated with the progression of IS (52). Despite the established association between PSOL and stroke severity, our study suggests that elevated serum MIP-1β levels could potentially mitigate the severity of stroke events in middle-aged patients with PSOL. Several underlying mechanisms could account for this phenomenon. First, the mRNA and protein expression of the chemokine MIP-1β has been reported to be inhibited by prostaglandin E2 (PGE2) (53). PGE2, a pivotal endogenous anti-inflammatory mediator linked to sleep regulation, demonstrates wakefulness-promoting properties (53). Notably, its concentration is markedly higher during wakefulness compared to slow-wave sleep (54). In this context, participants grappling with PSOL are prone to extended periods of wakefulness (15), leading to heightened PGE2 levels and diminished MIP-1β levels. Intriguingly, PGE2’s impact extends further, potentially playing a dual role. PGE2 has been identified as a disruptor of Na(+)-Ca(2+) exchange and Ca(2+) homeostasis through the EP1 receptor, thereby contributing to excessive Ca(2+) accumulation. This effect also extends to the induction of neuronal cell death and the augmentation of ischemic-induced neurodegeneration (55), ultimately amplifying stroke severity. Indeed, it is highly conceivable that the deleterious influence of MIP-1β on stroke severity in middle-aged individuals with PSOL might be attenuated by PGE2, potentially even manifesting as a protective function. However, this study did not observe a correlation between serum MIP-1β levels and NIHSS scores in older adults with PSOL, attributed to the confounding impact of age. In this regard, one animal study unveiled heightened expression of MIP-1β levels in older mice (28), while another investigation highlighted an accelerated decline in serum MIP-1β levels with aging (56). Consequently, aging appears to disrupt the relationship between MIP-1β and NIHSS scores in older individuals with PSOL. In essence, the protective role of MIP-1β against stroke severity seems to be confined to middle-aged patients with PSOL.
Besides, the NSOL group displayed a potential protective effect against stroke in the elderly, as evidenced by the negative association between MIP-1α and NIHSS scores. Previous studies have shown that, compared to middle-aged mice, chemokine MIP-1α levels are highly expressed in older mice (28). Interestingly, it has been found that MIP-1α levels were significantly reduced in the brain tissue of older patients with IS (57), and MIP-1α tended to decline with age in this patient population (56). At the same time, the NIHSS score at admission increased significantly with age (58). Therefore, the negative correlation between serum MIP-1α level and NIHSS score in senile stroke patients with NSOL may be due to aging. However, our study did not observe the correlation between serum MIP-1α and NIHSS score in elderly patients with PSOL, attributed to the fact that individuals suffering from PSOL exhibited suboptimal sleep quality, consequently experiencing extended periods of wakefulness, which led to an increase in PGE2. The increased PGE2 led to a decline in the chemokine MIP-1α level (59), thus disrupting the age-related negative correlation typically observed between serum MIP-1α levels and NIHSS scores.
Besides, we found no association between MCP-1, IL-6, and TNF-α and NIHSS scores across the four groups. However, prior research on individuals with severe IS demonstrated a correlation between elevated levels of these factors and the severity of IS (60–62). Discrepancies in outcomes might stem from the inclusion of subjects with mild to moderate IS in this current study. Moreover, a prior investigation examining stroke severity 7 days after admission demonstrated a positive association between elevated MCP-1 levels and heightened stroke severity at the same time point (61), Conversely, the present study did not reveal a connection between MCP-1 and stroke severity. This discrepancy may be attributable to the timing of MCP-1 measurement; in this study, samples were collected on day one after admission, whereas the previous study assessed MCP-1 levels 7 days post-stroke. The temporal dynamics of ischemic brain cell damage likely influence the correlation between MCP-1 and stroke severity. In cases where initial ischemic attack results in more severe injury, cytokine and chemokine production may be suppressed. This offers a potential explanation for the absence of a correlation between MCP-1 and NIHSS scores in the current study (61).
Several limitations need consideration within this study. Firstly, the participant pool only comprised Chinese individuals residing in the northern inland region, regardless of whether they were experiencing their first episode or recurrence. Future research should prioritize geographically diverse recruitment and larger sample sizes to improve generalizability of results. Additionally, it is essential to differentiate between first-episode patients and those with recurrent episodes and to conduct stratification analyses based on the number of episodes to bolster result accuracy. Secondly, since sleep patterns influence IS over an extended duration, this study only retrospectively gathered SOL data from 1 to 3 months preceding the IS onset. While data from this brief interval might not comprehensively capture the impact, collecting recent-stage sleep data retrospectively was more feasible, and patient cooperation was facilitated. Lastly, most IS participants in this study exhibited mild to moderate stroke. Consequently, it is important to acknowledge that the generalizability of our study findings may be limited to cases of milder stroke severity. Nevertheless, the presented results provide valuable guidance for the development of targeted preventive interventions for individuals at risk of such strokes.
The present study provides strong evidence of the association between PSOL and the severity of IS and the potential protective effects of MIP-1β in reducing stroke severity, especially in middle-aged patients, suggesting that falling asleep quickly might contribute to low ischemic stroke severity. In the future, the role of other subfamilies of chemokines in the interaction of sleep onset latency and age on IS severity should be further explored to improve and supplement this study.
The original contributions presented in the study are included in the article/supplementary material, further inquiries can be directed to the corresponding authors.
The studies involving humans were approved by the Institutional Review Board of the Sinopharm North Hospital (Approval number: GYBFYY-LL-2020006). The studies were conducted in accordance with the local legislation and institutional requirements. The participants provided their written informed consent to participate in this study.
FW: Conceptualization, Funding acquisition, Writing – review & editing. YZ: Conceptualization, Data curation, Writing – original draft. XH: Writing – original draft. QM: Writing – original draft. LX: Methodology, Writing – review & editing. YW: Data curation, Funding acquisition, Writing – review & editing. CL: Conceptualization, Writing – review & editing, Methodology. YL: Conceptualization, Writing – review & editing, Methodology.
The author(s) declare financial support was received for the research, authorship, and/or publication of this article. This work was supported by the following grants: The Technology Support Project of Xinjiang (2017E0267), the 10th Inner Mongolia Autonomous Region “Prairie excellence” Project, Natural Science Foundation of Xinjiang Province (2018D01C228), Xinjiang Outstanding Youth Science Grant (2017Q007), Natural Science Foundation of China (81560229), Beijing Natural Science Foundation (7152074), the “Qingmiao” program of Beijing Municipal Hospital Management Center (QML20212003), and the Youth Scientific Research Foundation of Beijing Huilongguan Hospital (LY202106).
We thank all the participants for their willingness to participate in the study and the time that they devoted to the study.
The authors declare that the research was conducted in the absence of any commercial or financial relationships that could be construed as a potential conflict of interest.
All claims expressed in this article are solely those of the authors and do not necessarily represent those of their affiliated organizations, or those of the publisher, the editors and the reviewers. Any product that may be evaluated in this article, or claim that may be made by its manufacturer, is not guaranteed or endorsed by the publisher.
1. Song, Y , Zhang, X , Li, C , Xu, S , Zhou, B , and Wu, X . Is bilirubin associated with the severity of ischemic stroke? A dose response Meta-analysis. J Clin Med. (2022) 11:3262. doi: 10.3390/jcm11123262
2. Kadier, K , Qin, L , Ainiwaer, A , Rehemuding, R , Dilixiati, D , Du, YY, et al. Association of Sleep-Related Disorders with cardiovascular disease among adults in the United States: a cross-sectional study based on National Health and nutrition examination survey 2005-2008. Front Cardiovasc Med. (2022) 9:954238. doi: 10.3389/fcvm.2022.954238
3. Guo, Y , Wang, H , Tian, Y , Wang, Y , and Lip, GY . Multiple risk factors and Ischaemic stroke in the elderly Asian population with and without atrial fibrillation. An analysis of 425,600 Chinese individuals without prior stroke. Thromb Haemost. (2016) 115:184–92. doi: 10.1160/TH15-07-0577
4. Terzoudi, A , Vorvolakos, T , Heliopoulos, I , Livaditis, M , Vadikolias, K , and Piperidou, H . Sleep architecture in stroke and relation to outcome. Eur Neurol. (2009) 61:16–22. doi: 10.1159/000165344
5. Zhong, X , Gou, F , Jiao, H , Zhao, D , and Teng, J . Association between night sleep latency and hypertension: a cross-sectional study. Medicine (Baltimore). (2022) 101:e31250. doi: 10.1097/MD.0000000000031250
6. Miano, S , Fanfulla, F , Nobili, L , Heinzer, R , Haba-Rubio, J , Berger, M, et al. Sas care 1: sleep architecture changes in a cohort of patients with ischemic stroke/Tia. Sleep Med. (2022) 98:106–13. doi: 10.1016/j.sleep.2022.06.002
7. Kim, WH , Yoo, YH , Lim, JY , Kang, SG , Jung, HY , Bae, JN, et al. Objective and subjective sleep problems and quality of life of rehabilitation in patients with mild to moderate stroke. Top Stroke Rehabil. (2020) 27:199–207. doi: 10.1080/10749357.2019.1673591
8. Habte-Gabr, E , Wallace, RB , Colsher, PL , Hulbert, JR , White, LR , and Smith, IM . Sleep patterns in rural elders: demographic, health, and Psychobehavioral correlates. J Clin Epidemiol. (1991) 44:5–13. doi: 10.1016/0895-4356(91)90195-f
9. Wang, F , Wang, J , Han, Y , Shi, X , Xu, X , Hou, C, et al. Triglyceride-glucose index and stroke recurrence in elderly patients with ischemic stroke. Front Endocrinol (Lausanne). (2022) 13:1005614. doi: 10.3389/fendo.2022.1005614
10. Chen, RL , Balami, JS , Esiri, MM , Chen, LK , and Buchan, AM . Ischemic stroke in the elderly: an overview of evidence. Nat Rev Neurol. (2010) 6:256–65. doi: 10.1038/nrneurol.2010.36
11. Aigner, A , Grittner, U , Rolfs, A , Norrving, B , Siegerink, B , and Busch, MA . Contribution of established stroke risk factors to the burden of stroke in young adults. Stroke. (2017) 48:1744–51. doi: 10.1161/STROKEAHA.117.016599
12. Maaijwee, NA , Rutten-Jacobs, LC , Schaapsmeerders, P , van Dijk, EJ , and de Leeuw, FE . Ischaemic stroke in young adults: risk factors and long-term consequences. Nat Rev Neurol. (2014) 10:315–25. doi: 10.1038/nrneurol.2014.72
13. Petrov, KK , Hayley, A , Catchlove, S , Savage, K , and Stough, C . Is poor self-rated sleep quality associated with elevated systemic inflammation in healthy older adults? Mech Ageing Dev. (2020) 192:111388. doi: 10.1016/j.mad.2020.111388
14. Schloss, MJ , Swirski, FK , and Nahrendorf, M . Modifiable cardiovascular risk, hematopoiesis, and innate immunity. Circ Res. (2020) 126:1242–59. doi: 10.1161/CIRCRESAHA.120.315936
15. Hawes, NJ , Wiggins, AT , Reed, DB , and Hardin-Fanning, F . Poor sleep quality is associated with obesity and depression in farmers. Public Health Nurs. (2019) 36:270–5. doi: 10.1111/phn.12587
16. Newton, TL , and Fernandez-Botran, R . Promoting health by improving subjective sleep quality? Reduction in depressive symptoms and inflammation as potential mechanisms and implications for trauma-exposed persons. Front Psych. (2016) 7:76. doi: 10.3389/fpsyt.2016.00076
17. Ohayon, MM , Carskadon, MA , Guilleminault, C , and Vitiello, MV . Meta-analysis of quantitative sleep parameters from childhood to old age in healthy individuals: developing normative sleep values across the human lifespan. Sleep. (2004) 27:1255–73. doi: 10.1093/sleep/27.7.1255
18. Myllyntausta, S , Salo, P , Kronholm, E , Pentti, J , Oksanen, T , Kivimaki, M, et al. Does removal of work stress explain improved sleep following retirement? The Finnish retirement and aging study. Sleep. (2019) 42:zsz109. doi: 10.1093/sleep/zsz109
19. Finger, CE , Moreno-Gonzalez, I , Gutierrez, A , Moruno-Manchon, JF , and McCullough, LD . Age-related immune alterations and cerebrovascular inflammation. Mol Psychiatry. (2022) 27:803–18. doi: 10.1038/s41380-021-01361-1
20. Lin, YT , Chen, HD , Ai, QD , Yang, YT , Zhang, Z , Chu, SF, et al. Characteristics and pathogenesis of chemokines in the post-stroke stage. Int Immunopharmacol. (2023) 116:109781. doi: 10.1016/j.intimp.2023.109781
21. Zaremba, J , Ilkowski, J , and Losy, J . Serial measurements of levels of the chemokines Ccl2, Ccl3 and Ccl5 in serum of patients with acute Ischaemic stroke. Folia Neuropathol. (2006) 44:282–9.
22. Florholmen, J , Kristiansen, MG , Steigen, SE , Sorbye, SW , Paulssen, EJ , Kvamme, JM, et al. A rapid chemokine response of macrophage inflammatory protein (Mip)-1alpha, Mip-1beta and the regulated on activation, Normal T expressed and secreted chemokine is associated with a sustained Virological response in the treatment of chronic hepatitis C. Clin Microbiol Infect. (2011) 17:204–9. doi: 10.1111/j.1469-0691.2010.03206.x
23. Liu, X , Chen, B , Huang, Z , Duan, R , Li, H , Xie, L, et al. Effects of poor sleep on the immune cell landscape as assessed by single-cell analysis. Commun Biol. (2021) 4:1325. doi: 10.1038/s42003-021-02859-8
24. Sprecher, KE , Koscik, RL , Carlsson, CM , Zetterberg, H , Blennow, K , Okonkwo, OC, et al. Poor sleep is associated with Csf biomarkers of amyloid pathology in cognitively Normal adults. Neurology. (2017) 89:445–53. doi: 10.1212/WNL.0000000000004171
25. Huang, WY , Huang, CC , Chang, CC , Kor, CT , Chen, TY , and Wu, HM . Associations of self-reported sleep quality with circulating interferon gamma-inducible protein 10, interleukin 6, and high-sensitivity C-reactive protein in healthy menopausal women. PLoS One. (2017) 12:e0169216. doi: 10.1371/journal.pone.0169216
26. Milrad, SF , Hall, DL , Jutagir, DR , Lattie, EG , Ironson, GH , Wohlgemuth, W, et al. Poor sleep quality is associated with greater circulating pro-inflammatory cytokines and severity and frequency of chronic fatigue syndrome/Myalgic encephalomyelitis (Cfs/me) symptoms in women. J Neuroimmunol. (2017) 303:43–50. doi: 10.1016/j.jneuroim.2016.12.008
27. Prather, AA , Epel, ES , Cohen, BE , Neylan, TC , and Whooley, MA . Gender differences in the prospective associations of self-reported sleep quality with biomarkers of systemic inflammation and coagulation: findings from the heart and soul study. J Psychiatr Res. (2013) 47:1228–35. doi: 10.1016/j.jpsychires.2013.05.004
28. Porcher, L , Bruckmeier, S , Burbano, SD , Finnell, JE , Gorny, N , Klett, J, et al. Aging triggers an upregulation of a multitude of cytokines in the male and especially the female rodent Hippocampus but more discrete changes in other brain regions. J Neuroinflammation. (2021) 18:219. doi: 10.1186/s12974-021-02252-6
29. Wang, Q , Tang, XN , and Yenari, MA . The inflammatory response in stroke. J Neuroimmunol. (2007) 184:53–68. Epub 2006/12/26. doi: 10.1016/j.jneuroim.2006.11.014
30. Huang, Y , Chen, S , Luo, Y , and Han, Z . Crosstalk between inflammation and the Bbb in stroke. Curr Neuropharmacol. (2020) 18:1227–36. doi: 10.2174/1570159X18666200620230321
31. Przykaza, L . Understanding the connection between common stroke comorbidities, their associated inflammation, and the course of the cerebral ischemia/reperfusion Cascade. Front Immunol. (2021) 12:782569. doi: 10.3389/fimmu.2021.782569
32. Jones, KL , Maguire, JJ , and Davenport, AP . Chemokine receptor Ccr5: from aids to atherosclerosis. Br J Pharmacol. (2011) 162:1453–69. doi: 10.1111/j.1476-5381.2010.01147.x
34. Kasner, SE . Clinical interpretation and use of stroke scales. Lancet Neurol. (2006) 5:603–12. doi: 10.1016/S1474-4422(06)70495-1
35. Kwah, LK , and Diong, J . National Institutes of Health stroke scale (Nihss). J Physiother. (2014) 60:61. doi: 10.1016/j.jphys.2013.12.012
36. Reinholdsson, M , Palstam, A , and Sunnerhagen, KS . Prestroke physical activity could influence acute stroke severity (part of Papsigot). Neurology. (2018) 91:e1461–7. doi: 10.1212/WNL.0000000000006354
37. Lindley, RI , Wardlaw, JM , Whiteley, WN , Cohen, G , Blackwell, L , Murray, GD, et al. Alteplase for acute ischemic stroke: outcomes by clinically important subgroups in the third international stroke trial. Stroke. (2015) 46:746–56. doi: 10.1161/STROKEAHA.114.006573
38. Scinicariello, F , Buser, MC , Feroe, AG , and Attanasio, R . Antimony and sleep-related disorders: Nhanes 2005-2008. Environ Res. (2017) 156:247–52. doi: 10.1016/j.envres.2017.03.036
39. Mai, X , and Liang, X . Risk factors for stroke based on the National Health and nutrition examination survey. J Nutr Health Aging. (2020) 24:791–5. doi: 10.1007/s12603-020-1430-4
40. Zhang, Y , Xia, X , Zhang, T , Zhang, C , Liu, R , Yang, Y, et al. Relationship between sleep disorders and the prognosis of neurological function after stroke. Front Neurol. (2022) 13:1036980. doi: 10.3389/fneur.2022.1036980
41. Krishnamurthi, RV , Moran, AE , Feigin, VL , Barker-Collo, S , Norrving, B , Mensah, GA, et al. Stroke prevalence, mortality and disability-adjusted life years in adults aged 20-64 years in 1990-2013: data from the global burden of disease 2013 study. Neuroepidemiology. (2015) 45:190–202. doi: 10.1159/000441098
42. Kissela, BM , Khoury, JC , Alwell, K , Moomaw, CJ , Woo, D , Adeoye, O, et al. Age at stroke: temporal trends in stroke incidence in a large, biracial population. Neurology. (2012) 79:1781–7. doi: 10.1212/WNL.0b013e318270401d
43. George, MG , Tong, X , Kuklina, EV , and Labarthe, DR . Trends in stroke hospitalizations and associated risk factors among children and young adults, 1995-2008. Ann Neurol. (2011) 70:713–21. doi: 10.1002/ana.22539
44. Taillard, J , Gronfier, C , Bioulac, S , Philip, P , and Sagaspe, P . Sleep in Normal aging, homeostatic and circadian regulation and vulnerability to sleep deprivation. Brain Sci. (2021) 11:1003. doi: 10.3390/brainsci11081003
45. Mander, BA , Winer, JR , and Walker, MP . Sleep and Human Aging. Neuron. (2017) 94:19–36. doi: 10.1016/j.neuron.2017.02.004
46. Hirshkowitz, M , Whiton, K , Albert, SM , Alessi, C , Bruni, O , DonCarlos, L, et al. National Sleep Foundation's updated sleep duration recommendations: final report. Sleep Health. (2015) 1:233–43. doi: 10.1016/j.sleh.2015.10.004
47. Hofmeijer, J , van Kaam, R , Vermeer, SE , and van Putten, M . Severely disturbed sleep in patients with acute ischemic stroke on stroke units: a pilot study. Front Neurol. (2019) 10:1109. doi: 10.3389/fneur.2019.01109
48. Gao, Y , Jia, Z , Zhao, L , and Han, S . The effect of activity participation in middle-aged and older people on the trajectory of depression in later life: National Cohort Study. JMIR Public Health Surveill. (2023) 9:e44682. doi: 10.2196/44682
49. Reddin, C , Murphy, R , Hankey, GJ , Judge, C , Xavier, D , Rosengren, A, et al. Association of Psychosocial Stress with risk of acute stroke. JAMA Netw Open. (2022) 5:e2244836. doi: 10.1001/jamanetworkopen.2022.44836
50. McDermott, M , Brown, DL , and Chervin, RD . Sleep disorders and the risk of stroke. Expert Rev Neurother. (2018) 18:523–31. doi: 10.1080/14737175.2018.1489239
51. Ding, D , Rogers, K , van der Ploeg, H , Stamatakis, E , and Bauman, AE . Traditional and emerging lifestyle risk behaviors and all-cause mortality in middle-aged and older adults: evidence from a large population-based Australian cohort. PLoS Med. (2015) 12:e1001917. doi: 10.1371/journal.pmed.1001917
52. Mirabelli-Badenier, M , Braunersreuther, V , Viviani, GL , Dallegri, F , Quercioli, A , Veneselli, E, et al. Cc and cxc chemokines are pivotal mediators of cerebral injury in Ischaemic stroke. Thromb Haemost. (2011) 105:409–20. doi: 10.1160/TH10-10-0662
53. Hayaishi, O . Molecular mechanisms of sleep-wake regulation: roles of prostaglandins D2 and E2. FASEB J. (1991) 5:2575–81. doi: 10.1096/fasebj.5.11.1907936
54. Gerozissis, K , De Saint, HZ , Orosco, M , Rouch, C , and Nicolaidis, S . Changes in hypothalamic prostaglandin E2 may predict the occurrence of sleep or wakefulness as assessed by parallel Eeg and microdialysis in the rat. Brain Res. (1995) 689:239–44. doi: 10.1016/0006-8993(95)00583-c
55. Yagami, T , Koma, H , and Yamamoto, Y . Pathophysiological roles of cyclooxygenases and prostaglandins in the central nervous system. Mol Neurobiol. (2016) 53:4754–71. doi: 10.1007/s12035-015-9355-3
56. Swift, ME , Burns, AL , Gray, KL , and DiPietro, LA . Age-related alterations in the inflammatory response to dermal injury. J Invest Dermatol. (2001) 117:1027–35. doi: 10.1046/j.0022-202x.2001.01539.x
57. Sieber, MW , Claus, RA , Witte, OW , and Frahm, C . Attenuated inflammatory response in aged mice brains following stroke. PLoS One. (2011) 6:e26288. doi: 10.1371/journal.pone.0026288
58. Purroy, F , Vena, A , Forne, C , de Arce, AM , Davalos, A , Fuentes, B, et al. Age- and sex-specific risk profiles and in-hospital mortality in 13,932 Spanish stroke patients. Cerebrovasc Dis. (2019) 47:151–64. doi: 10.1159/000500205
59. Takayama, K , Garcia-Cardena, G , Sukhova, GK , Comander, J , Gimbrone, MA Jr, and Libby, P . Prostaglandin E2 suppresses chemokine production in human macrophages through the Ep4 receptor. J Biol Chem. (2002) 277:44147–54. doi: 10.1074/jbc.M204810200
60. Xu, P , Zhang, S , Kan, X , Shen, X , Mao, J , Fang, C, et al. Changes and roles of Il-17a, Vegf-a and Tnf-alpha in patients with cerebral infarction during the acute phase and early stage of recovery. Clin Biochem. (2022) 107:67–72. doi: 10.1016/j.clinbiochem.2022.05.001
61. Bonifacic, D , Toplak, A , Benjak, I , Tokmadzic, VS , Lekic, A , and Kucic, N . Monocytes and monocyte chemoattractant protein 1 (Mcp-1) as early predictors of disease outcome in patients with cerebral ischemic stroke. Wien Klin Wochenschr. (2016) 128:20–7. doi: 10.1007/s00508-015-0878-4
62. Shaafi, S , Sharifipour, E , Rahmanifar, R , Hejazi, S , Andalib, S , Nikanfar, M, et al. Interleukin-6, a reliable prognostic factor for ischemic stroke. Iran J Neurol. (2014) 13:70–6.
Keywords: sleep onset latency, age, ischemic stroke, inflammation, interaction
Citation: Zhou Y, Han X, Mu Q, Xing L, Wu Y, Li C, Liu Y and Wang F (2024) The effect of the interaction of sleep onset latency and age on ischemic stroke severity via inflammatory chemokines. Front. Neurol. 15:1323878. doi: 10.3389/fneur.2024.1323878
Received: 18 October 2023; Accepted: 07 February 2024;
Published: 16 February 2024.
Edited by:
Yanlin Zhang, Second Affiliated Hospital of Soochow University, ChinaReviewed by:
Xingguang Luo, Yale University, United StatesCopyright © 2024 Zhou, Han, Mu, Xing, Wu, Li, Liu and Wang. This is an open-access article distributed under the terms of the Creative Commons Attribution License (CC BY). The use, distribution or reproduction in other forums is permitted, provided the original author(s) and the copyright owner(s) are credited and that the original publication in this journal is cited, in accordance with accepted academic practice. No use, distribution or reproduction is permitted which does not comply with these terms.
*Correspondence: Fan Wang, ZmFud2FuZ0Biam11LmVkdS5jbg==; Yanlong Liu, YmVuamFtaW5seWxAd211LmVkdS5jbg==; Cunbao Li, ODUwOTg0MDg0QHFxLmNvbQ==
†These authors have contributed equally to this work
Disclaimer: All claims expressed in this article are solely those of the authors and do not necessarily represent those of their affiliated organizations, or those of the publisher, the editors and the reviewers. Any product that may be evaluated in this article or claim that may be made by its manufacturer is not guaranteed or endorsed by the publisher.
Research integrity at Frontiers
Learn more about the work of our research integrity team to safeguard the quality of each article we publish.