- 1Department of Neurology, LMU University Hospital, LMU Munich, Munich, Germany
- 2Department of Anesthesiology, LMU University Hospital, LMU Munich, Munich, Germany
- 3Institute for Digital Medicine, University Hospital Augsburg, Augsburg, Germany
- 4Faculty of Medicine, Pettenkofer School of Public Health, Institute for Medical Information Processing, Biometry and Epidemiology (IBE), LMU Munich, Munich, Germany
Background and objective: Post-stroke delirium (PSD) is a common complication in acute stroke patients, and guidelines recommend routine screening and various preventive and treatment measures. However, there is a substantial lack of standardized approaches in diagnostic and therapeutic management of PSD. Here, we aimed to develop a new pragmatic and easily assessable screening tool to predict PSD based on early parameters, which are already integral to acute stroke diagnostics.
Methods: We enrolled acute stroke patients admitted to our stroke unit or intensive care unit and developed the scoring system using retrospective single-center patient data. The Confusion Assessment Method for the Intensive Care Unit was used for prospective score validation. Logistic regression models were employed to analyze the association of early clinical and paraclinical parameters with PSD development.
Results: N = 525 patients (median age: 76 years; 45.7% female) were enrolled, with 29.7% developing PSD during hospitalization. The resulting score comprises 6 items, including medical history, clinical examination findings, and non-contrast computed tomography results at admission. Scores range from −15 to +15 points, with higher values indicating a higher likelihood of PSD, ranging from 4% to 79%. The accuracy was 0.85, and the area under the curve was 0.89.
Conclusion: The new RAPID (Risk Assessment and PredIction of Delirium in acute stroke patients)-score shows high accuracy in predicting PSD among acute stroke patients and offers precise odds of PSD for each corresponding score value, utilizing routine early clinical and paraclinical parameters. It can identify high-risk populations for clinical study interventions and may be suitable to guide prophylactic PSD measures.
Introduction
Post-stroke delirium (PSD) is a common complication during hospitalization among patients with acute stroke who are admitted to a stroke unit (SU) or intensive care unit (ICU) (1). It is characterized by attention and concentration disorders, rapid onset, fluctuation throughout the day, and additional cognitive impairments and can manifest as hyperactive, hypoactive, or mixed subtype (2, 3). The detailed pathophysiology of delirium remains unclear; however, it is widely accepted that delirium results from a combination of susceptibility factors and external stressors, including surgery, general anesthesia, or critical illnesses such as stroke (3, 4). The incidence of PSD varies across different studies, with rates ranging from approximately 20 to 35% in SU patients and up to 89% in ICU patients (5, 6). Previous research has shown that PSD is strongly linked to extended hospital stays, ranging from 5 to 22 days longer than stroke patients without PSD. Additionally, it has been found to significantly increase the likelihood of poor functional outcomes, with odds ranging from 2 to 5, and substantially elevate mortality rates, with odds ranging from 2 to 15 times higher depending on the study, stroke subtype, comorbidities and ICU or SU admission (1, 7–12). Several risk factors have been identified, including age, stroke severity, illicit drug use, atrial fibrillation, aphasia, and preexisting dementia (13, 14).
For acute stroke patients, current national and international guidelines recommend regular screening for PSD throughout their hospital stay (15, 16). This can be achieved using tools like the Confusion Assessment Method for the Intensive Care Unit (CAM-ICU) or the Intensive Care Delirium Screening Checklist (ICDSC) (15). In addition, a multidimensional approach for prevention and treatment is recommended, involving both non-pharmacological strategies such as early mobilization, maintaining proper sleep hygiene, and providing reorientation measures, e.g., through the presence of relatives, as well as pharmaceutical approaches, including the use of antipsychotic drugs, particularly in cases of productive psychotic symptoms (15, 17).
Despite being highly relevant in the daily care of acute stroke patients, there is a lack of standardized diagnostic and treatment approaches backed by the highest level of evidence. As a result, the treatment of PSD is often left to the discretion of the treating physicians. Furthermore, the actual clinical practice deviates from the established guidelines. Surveys have revealed that only half of the clinicians have implemented standardized PSD processes, and less than a third regularly employ valid PSD assessments (6, 18, 19). The participants cited a lack of knowledge, staff shortages and insufficient time as the most frequent reasons for this discrepancy. Hence, there is a need for readily accessible and time-saving tools to identify acute stroke patients at a high risk of developing PSD during their hospital stay. This may help tailor and guide prophylactic and therapeutic measures, seamlessly integrating them into daily clinical practice. Our objective was to develop and validate a pragmatic clinical scoring system for predicting PSD in acute stroke patients during hospitalization. The goal was to devise a score based on readily accessible clinical and paraclinical parameters that are already part of the standard diagnostic workup in suspected stroke cases. By doing so, we aimed to facilitate easy implementation without the need for additional screening methods.
Methods
Study population and eligibility criteria
The study included consecutive patients admitted to our local SU or ICU from October 2020 to April 2021 for score development and retrospective validation. Additionally, consecutive patients admitted between December 2021 and January 2022 were included for prospective validation (Figure 1). The period between May 2021 and November 2021 was used for score development, retrospective validation and preparation of prospective validation. We enrolled all adult patients with confirmed or suspected acute stroke upon admission. All subjects initially underwent evaluation in our emergency department and were subsequently admitted to either the SU or ICU after receiving emergency diagnostics and treatment. A total of n = 608 patients were initially screened for the study. Out of these, n = 525 patients were enrolled for the final analyses, which included score development and retrospective and prospective score validation. N = 388 patients (93.0%) were admitted to our SU, while n = 37 patients (7.0%) were referred to ICU upon admission. We excluded n = 42 patients who were admitted due to causes other than suspected of confirmed stroke. Additionally, 31 patients were excluded from the retrospective analyses as their data completeness was less than 80%. During the prospective validation phase, n = 5 patients refused to provide consent to participate. Moreover, premodified Rankin Scale (pmRS) and non-contrast computed tomography (NCCT) data at admission were missing in n = 3 and n = 2 patients, respectively in this cohort.
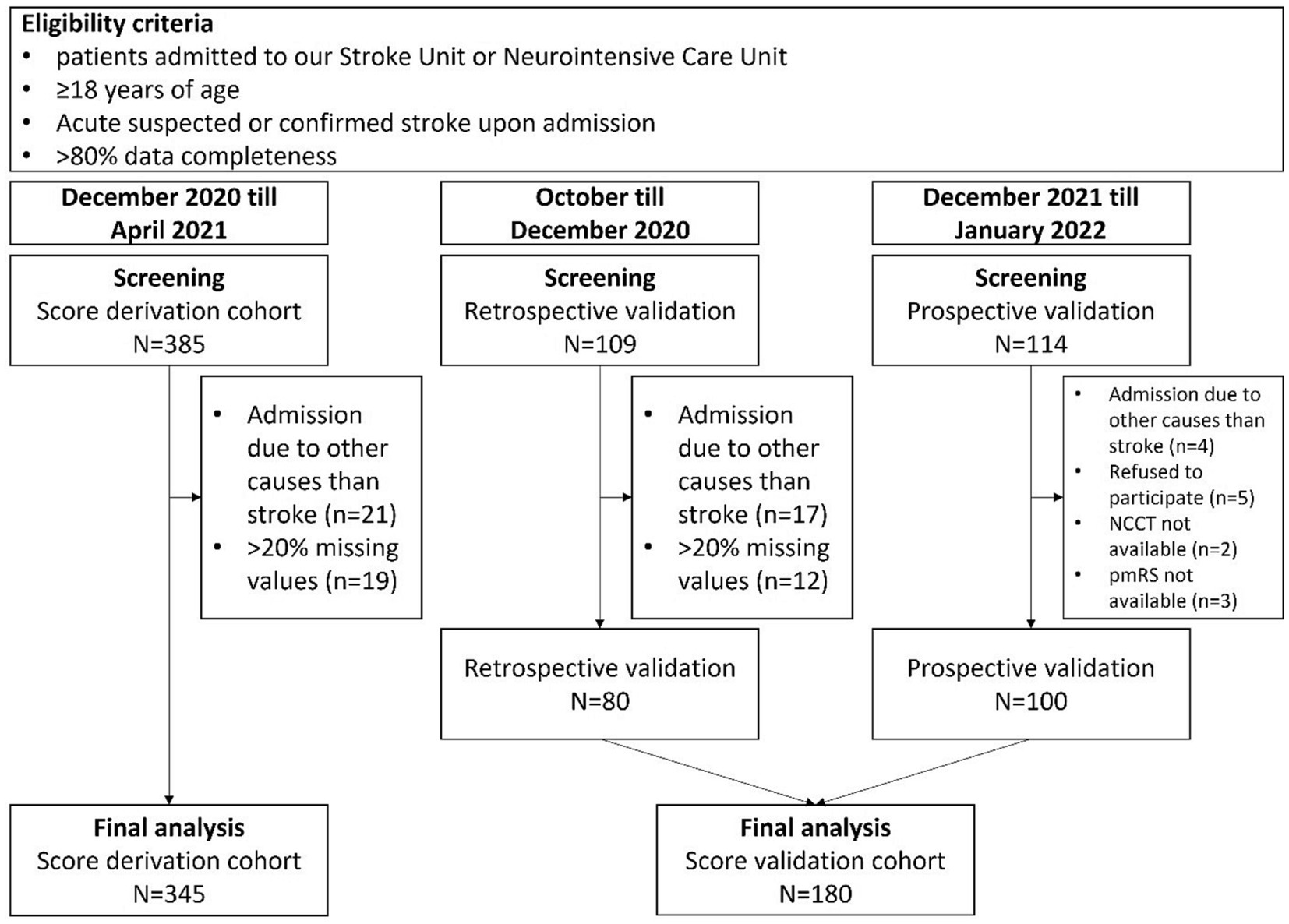
Figure 1. Flowchart for selection and exclusion of patients for final analyses. NCCT, Non contrast computed tomography; pmRS, premodified Rankin Scale.
Score derivation cohort
To develop the scoring system, we retrospectively analyzed consecutive patients (n = 345) between December 2020 and April 2021 using electronic medical records. We examined early clinical and paraclinical parameters, including known risk factors for delirium development, that were available after emergency diagnostics and treatment but prior to admission to the SU or ICU. These parameters included age, sex, medical background and history, findings from clinical examinations, vital signs, ECG (electrocardiogram) findings, NCCT scans, CT-angiography (CTA) results, and basic blood examination findings (Table 1). We considered both self-reported information provided by the patients themselves and information provided by others, such as relatives and witnesses, for the medical history and background. Stroke severity and functional status prior to the event were assessed using the National Institutes of Health Stroke Scale (NIHSS) and the pmRS, respectively. Consciousness level was evaluated using the Glasgow Coma Scale (GCS) and the Richmond Agitation-Sedation Scale (RASS). An elevated troponin of >0.015 ng/mL in combination with a creatine kinase (CK)-MB-activity of >24 U/L was defined as myocardial injury. The degree of microangiopathy was rated using the Fazekas score assessed by NCCT (20, 21). All parameters were included in the analysis to predict the occurrence of delirium during the hospital stay. Diagnosis of delirium during the hospital stay was established according to national and international guidelines, such as DSM-5 criteria (22, 23). Detailed assessments of each patient’s medical record were conducted, specifically focusing on the documentation of the patient’s behavior by physicians, nurses, physiotherapists, speech therapists, and occupational therapists. In cases where the diagnosis of delirium was not explicitly documented in the medical records, but there was strong suspicion based on the documented clinical course of the patients (e.g., behavior, necessity for patient restraint, administration of antipsychotic drugs), delirium was presumed for further analysis. Stroke diagnosis at discharge was confirmed by either CT or magnetic resonance imaging. All radiological images were assessed by both radiologists and neuroradiologists as part of the clinical routine, independent from the study.
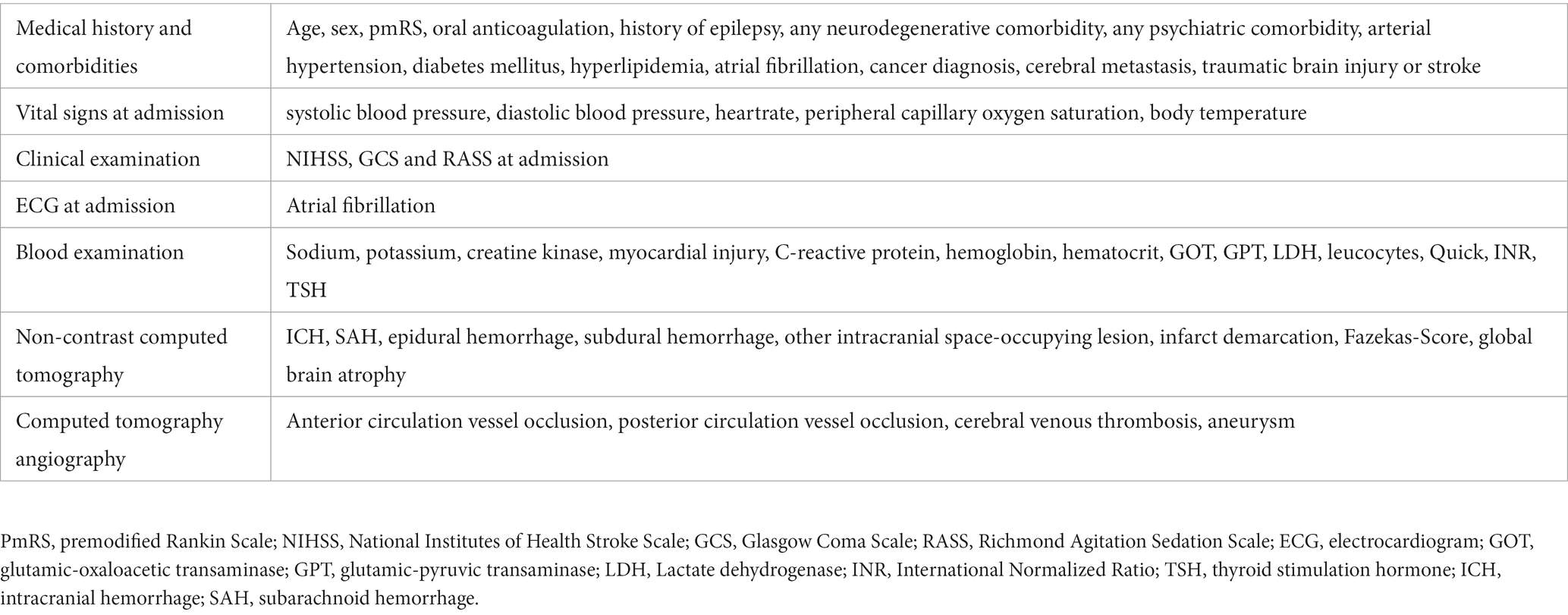
Table 1. Clinical and paraclinical parameters at admission considered for development of the scoring system.
Score validation cohort
To prospectively validate the scoring system, we enrolled eligible patients (n = 100) consecutively admitted to either our SU or ICU between December 2021 and January 2022. Written informed consent was obtained from patients or from their legal representatives in cases where patients were unable to provide consent. In situations where patients initially lacked the capacity to consent but later regained it, informed consent was obtained retrospectively directly from the patients themselves. All variables listed in Table 1 were assessed for each patient. Delirium was assessed employing the DSM-5 criteria and additionally using the CAM-ICU (8, 24, 25) immediately upon admission, as well as at the 24-h and 72-h marks after admission. Radiologists and neuroradiologists conducted assessments of all radiological images without knowledge of the subject’s delirium status, both at the time of imaging and throughout the clinical course. For internal retrospective validation, we analyzed electronic medical records of n = 80 consecutive patients between October 2020 and December 2020 who were separate from those included in the score derivation cohort.
Statistical analysis
Statistical analysis was conducted in Python 3.8.0 with Jupyter Notebooks 6.2.0. The following libraries were used: numpy (version 1.19.5), pandas (1.2.0), matplotlib (3.4.3), seaborn (0.11.1), scipy (1.6.0) and sklearn (0.24.1). The random state was set to 42 and used whenever applicable. We excluded columns with only one unique value. Missing values were imputed using an iterative imputer with 10 iterations. All columns with continuous data were categorized using the 33rd and 66th percentile: less or equal than the 33rd percentile, from 33rd to less than or equal the 66th percentile, or greater than the 66th percentile. In the case of the 33rd percentile being the same as the 66th percentile, we binarized the variable by the maximum value or less. Categorical variables were dummy-coded. A cross-validated logistic regression with 100,000 maximum iterations, a L2 penalty term and a liblinear solver were used for a 5-fold cross-validated recursive feature elimination, using accuracy as the performance metric. The minimum number of features to select was set to three. The number of features corresponding to the first local maximum of accuracy was then selected. A recursive feature elimination using the same logistic regression with the selected number of features was used to identify the parameters used for the following score development. All labels and the selected features were split into a training and a test set with a test size of 0.3. The training set was used to train a cross-validated logistic regression with a maximum of 50,000 iterations, a L2 penalty term and a liblinear solver. For the score development, we scaled all coefficients using and rounded these numbers to the nearest whole number. The positive predictive value, negative predictive value, sensitivity, specificity, accuracy score and AUC ROC score were calculated on the test set. The goodness of fit was tested using the Hosmer-Lemeshow test. The chi-square test of independence was used to test categorical variables between two groups, the Mann–Whitney U test was used to test for differences between continuous variables between two groups. An alpha-level of 0.05 was considered statistically significant. Univariate analysis was conducted, comparing parameters between patients with and without development of delirium during hospital stay. Each variable is displayed with median and interquartile range (IQR) or counts and percentages, where applicable.
Results
Demographics and descriptive statistics
In total, n = 525 patients (median age: 76 years; 45.7% female) were enrolled into final analysis. N = 156 (29.7%) developed delirium during hospitalization and suspected stroke was confirmed in n = 330 patients (62.9%) at discharge. In the score derivation cohort (n = 345; 46.1% female; Table 2), n = 98 patients (28.4%) developed delirium during their hospital stay, while in the score validation cohort (n = 180; 45.0% female), n = 58 (32.2%) patients experienced delirium during hospitalization. While there was no significant difference in the rate of confirmed stroke diagnosis at discharge in the derivation cohort, patients with delirium did experience longer hospital stays (8 vs. 5 days). They were significantly older (80 vs. 73 years) and upon admission, these patients exhibited higher pmRS-Scores (3 vs. 0) and were more likely to have pre-existing medical conditions, including a history of epilepsy (13.3 vs. 4.5%), cancer (31.6 vs. 14.6%), stroke (29.6 vs. 16.2%), neurodegenerative comorbidities (17.3 vs. 2.8%), and atrial fibrillation (32.7 vs. 11.3%). Additionally, a greater number of them were under oral anticoagulation upon admission (31.6 vs. 12.6%) and atrial fibrillation was more frequently detected in the initial admission ECG (33.7 vs. 9.7%). Moreover, patients who developed delirium during their hospital stay more often presented with impaired consciousness and more severe strokes, as indicated by lower GCS (14 vs. 15 points) and RASS scores, while their NIHSS scores were higher (7 vs. 2 points). One relevant distinction in laboratory findings was observed, with a higher rate of myocardial injury in the delirium cohort (20.4 vs. 6.1%). Furthermore, the NCCT at admission more frequently revealed the presence of microangiopathy (defined by a Fazekas-Score ≥ 1 point; 91.8 vs. 49.8%) and global brain atrophy (55.1 vs. 17.4%).
The score development
All parameters were taken into account for score development, except for “epidural hemorrhage in NCCT,” as this condition was not present in any of the patients in our cohort. Among the CTA variables, there were 37 missing values, while thyroid stimulating hormone had 29 missing values, and 24 other variables had a much lower extent of missing data (Supplementary Table). After imputing the missing data, the dataset was transformed and categorized, resulting in a total of 94 parameters for further analysis. The cross-validated recursive feature elimination returned 90 features to be the optimal number (accuracy = 0.7913). The first local maximum of accuracy scores was found for the amount of seven features (0.7101), which was used in a recursive feature elimination to identify the items used for the scoring system. The seventh feature, an INR of ≤0.9, predicted the occurrence of delirium. However, this particular feature was not included in the subsequent score development due to concerns related to clinical plausibility and practicality. The points for each score item were calculated based on the coefficients of the logistic regression.
The scoring system
The scoring system, which we named RAPID (Risk Assessment and PredIction of Delirium in acute stroke patients; Table 3) consists of 6 items and covers a score range from −15 to +15 points. Higher scores on this system indicate a greater likelihood of experiencing delirium during the hospital stay (Figure 2A). A GCS score of <15 at admission adds 6 points, a Fazekas Score ≥ 1 in NCCT at admission adds 5 points, and the presence of global brain atrophy in NCCT contributes 4 points to the total score. On the other hand, being aged ≤68 years deducts 4 points, a NIHSS score ≤ 1 subtracts 5 points, and a pmRS score of 0 takes away 6 points from the total score.
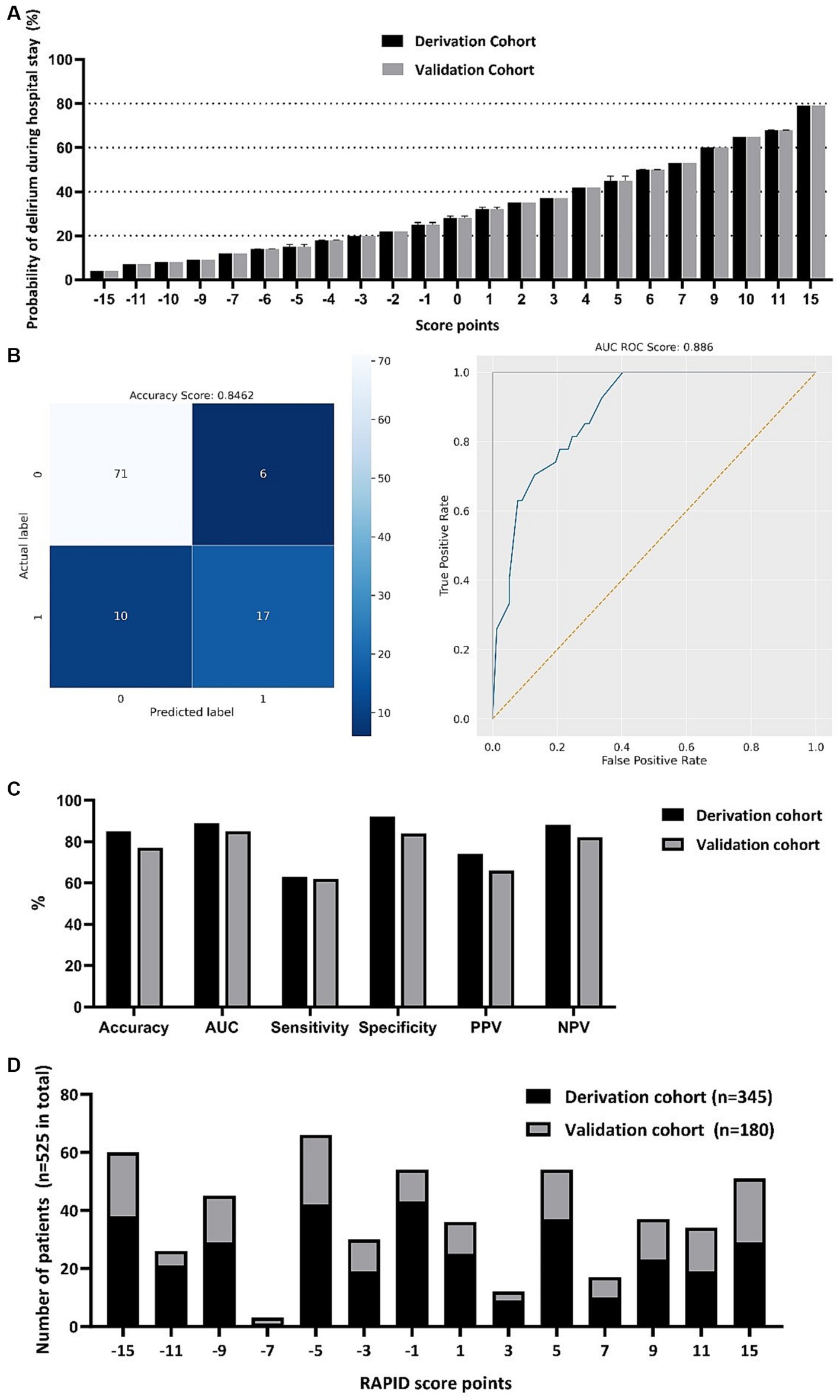
Figure 2. RAPID-score points with corresponding probability of developing delirium during the hospital stay (A). Computed values are depicted for both the derivation and the validation cohort with mean, minimum and maximum. Confusion matrix of the scoring system and receiver operation characteristic (ROC) curve in the score derivation cohort (B). Score performance value displayed for the score derivation and validation cohort (C). Frequency distribution of total RAPID-score points in both the derivation and validation cohort (D). AUC, Area under the curve; PPV, positive predictive value; NPV, negative predictive value.
Score performance and validation
In the derivation cohort, we achieved a computed accuracy of 0.8462 and an area under the curve (AUC) of 0.8860 (Figure 2B). For the validation cohort, the accuracy and AUC were slightly lower at 0.7722 and 0.8498, respectively. Regarding the positive predictive value (PPV) and negative predictive value (NPV), in the derivation cohort, we obtained a PPV of 0.7391 and an NPV of 0.8765. In the validation cohort, the PPV was 0.6545, and the NPV was 0.8220. We found that in the derivation cohort, the sensitivity was 0.6296, and the specificity was 0.9221. In the validation cohort, the sensitivity and specificity were 0.6207 and 0.8443, respectively (Figure 2C). The median RAPID score was −1 in both the derivation and validation cohorts, indicating a 25% probability of delirium development during the hospital stay. The frequency distribution is depicted in Figure 2D.
Discussion
The RAPID-score is a pragmatic clinical screening tool with high accuracy in predicting PSD during the hospital stay of acute ischemic stroke patients. This accuracy was consistent in both the score development and validation cohorts. It incorporates readily available parameters, such as medical history, clinical examination, and NCCT findings taken upon admission, all of which are integral to basic stroke diagnostics and already obtained during emergency department processing. In fact, the RAPID-score enables the prediction of delirium and risk assessment within the first hour after emergency admission of the patient. Furthermore, each RAPID-score point is linked to a precise probability of developing delirium during hospitalization, and this association shows minimal variance among the cohorts included in our study. Its seamless integration into clinical routine makes it easily adaptable, however the RAPID-Score does not aim to replace regular delirium screening during the hospital stay, using the CAM-ICU or ICDSC, as recommended by current guidelines. Instead, it may be a complementary tool for identifying high-risk populations, guiding prophylactic measures, or identifying candidates for future study interventions.
We observed delirium in approximately 30% of the patients, a rate comparable to existing data, considering that our study also included ICU patients, where delirium rates tend to be higher than in SU patients (26). Consistent with previous findings, we identified several factors predicting PSD, including older age, impaired consciousness, and the severity of strokes, as indicated by higher NIHSS scores (14, 27). Furthermore, we conducted univariate analyses for differences in single parameters between delirium and no-delirium patients. Here, we also observed higher rates of preexisting medical conditions in patients who developed PSD, such as atrial fibrillation, previous strokes, epilepsy, cancer, and neurodegenerative comorbidities, which most likely translate into higher pmRS scores in those patients. Moreover, cerebral microangiopathy and global brain atrophy, assessed by NCCT, turned out to be strong predictors for delirium within the scoring system, likely correlating with observed higher rates of preexisting neurodegenerative diseases, such as dementia, which have also previously been associated with an increased risk of delirium (7). However, the association of white matter lesions in acute stroke imaging and post-stroke cognitive decline several months after stroke onset remains subject to ongoing discussions (16). Interestingly, we found that none of the parameters assessed by NCCT and CTA at admission, which define stroke type (e.g., rate of intracerebral hemorrhage, infarction demarcation and side, and type of vessel occlusion), differed significantly between patients with and without PSD during their hospital stay. This suggests that the observed higher NIHSS scores in patients with delirium may likely not solely attributed to more severe stroke types but also to their higher susceptibility to stroke due to preexisting medical conditions.
Similar to other post-stroke prediction scores, our final score incorporated age and stroke severity. However, unlike those scores that only included stroke patients, we did not find a significant association between PSD and specific stroke subtypes (e.g., ICH, anterior vessel occlusion) or any laboratory markers, which could be attributed to the broader inclusion of patients with stroke mimics in our study (28–30). Regarding accuracy, sensitivity, and specificity, our score demonstrated comparable results to those of other prediction scores, however some prediction scores published elsewhere lack external validation and have smaller sample sizes, potentially affecting their generalizability and reliability (16, 28, 29). Moreover, when compared to other scoring systems, our presented score not only offers cut-off values but also provides precise probabilities of PSD development associated with each corresponding score value.
One of the key strengths of our study is the utilization of a relatively large sample size of well-characterized patients with high data completeness. Moreover, the score underwent validation in both retrospective and prospective independent cohorts. Notably, we have included stroke mimics in the study, enhancing the clinical relevance of our findings as it reflects real-world scenarios. Approximately one-third of our enrolled patients were identified as stroke mimics, with infectious causes, peripheral vestibular disorders and seizure attacks being the most common mimic, consistent with previous literature (31). However, inclusion of stroke mimics might have limited sensitivity and specificity, due to increased patient heterogeneity. Despite this, it has improved the generalizability of our results. Interestingly, we observed comparable rates of delirium during hospitalization in patients with stroke mimics, when compared to patients with confirmed strokes, underlining the importance of conducting delirium risk assessments in SU or ICU patients regardless of the specific diagnosis. The primary limitation of our study is the likely underdiagnosis of hypoactive delirium and the differentiation of pre-stroke cognitive decline, delirium and stroke-related cognitive deterioration independent from delirium, which is however a common limitation encountered in delirium assessments and applies to our study as well (3, 32, 33). Furthermore, the CAM-ICU has its weaknesses when applied in stroke patients and is not exactly equivalent to delirium assessment according to DSM-5 criteria (34, 35). Moreover, it is well known, that aphasia, preexisting cognitive impairment and dementia leads to diagnostic uncertainty in delirium assessment. Approximately 14% of our patients exhibited stroke-related aphasia, while 7% of our subjects had a preexisting neurodegenerative comorbidity. These factors may have influenced our findings and could have implications for the accuracy of our results and score performance. Additionally, since the score relies on information from the patient’s medical history, it may not be immediately applicable upon admission in cases where the patient is unable to provide this information independently, and no relatives are present. Furthermore, as our objective was to pragmatically assess scores immediately after patient admission, we only considered factors readily available early in the admission process for score development. Consequently, we cannot dismiss the possibility of overlooking other significant predictors for the development of PSD later in the patient’s clinical course.
Conclusion
The newly introduced RAPID-score is able to predict PSD among acute stroke patients with high accuracy. It incorporates early clinical and paraclinical parameters that are already integral to the standard diagnostic workup for suspected stroke cases, making it broadly adoptable and time efficient. It can identify high-risk individuals for clinical study interventions, and may be used to devise targeted strategies for the prevention of PSD.
Data availability statement
The original contributions presented in the study are included in the article/Supplementary material, further inquiries can be directed to the corresponding author.
Ethics statement
The studies involving humans were approved by Ethics Committee of the LMU Munich, LMU University Hospital Munich, Germany. The studies were conducted in accordance with the local legislation and institutional requirements. The participants provided their written informed consent to participate in this study.
Author contributions
JW: Conceptualization, Formal analysis, Investigation, Methodology, Project administration, Supervision, Validation, Visualization, Writing – original draft, Writing – review & editing. PK: Conceptualization, Data curation, Investigation, Methodology, Writing – review & editing. LH: Conceptualization, Formal analysis, Investigation, Methodology, Supervision, Validation, Writing – review & editing. RT: Conceptualization, Formal analysis, Investigation, Methodology, Supervision, Validation, Writing – review & editing. AB-P: Conceptualization, Formal analysis, Investigation, Methodology, Validation, Writing – original draft, Writing – review & editing. LK: Conceptualization, Investigation, Methodology, Project administration, Resources, Supervision, Validation, Writing – review & editing.
Funding
The author(s) declare that no financial support was received for the research, authorship, and/or publication of this article.
Conflict of interest
LK has received funding for travel or speaker honoraria from Alexion, AstraZeneca, Bayer Vital, Boehringer Ingelheim, Bristol-Meyer-Squibb, Daiichi Sankyo, and Pfizer outside of this study.
The remaining authors declare that the research was conducted in the absence of any commercial or financial relationships that could be construed as a potential conflict of interest.
Publisher’s note
All claims expressed in this article are solely those of the authors and do not necessarily represent those of their affiliated organizations, or those of the publisher, the editors and the reviewers. Any product that may be evaluated in this article, or claim that may be made by its manufacturer, is not guaranteed or endorsed by the publisher.
Supplementary material
The Supplementary material for this article can be found online at: https://www.frontiersin.org/articles/10.3389/fneur.2023.1306520/full#supplementary-material
References
1. Shaw, RC, Walker, G, Elliott, E, and Quinn, TJ. Occurrence rate of delirium in acute stroke settings: systematic review and meta-analysis. Stroke. (2019) 50:3028–36. doi: 10.1161/STROKEAHA.119.025015
2. Stollings, JL, Kotfis, K, Chanques, G, Pun, BT, Pandharipande, PP, and Ely, EW. Delirium in critical illness: clinical manifestations, outcomes, and management. Intensive Care Med. (2021) 47:1089–103. doi: 10.1007/s00134-021-06503-1
3. Haußmann, R, Postler, A, and Mirus, M. Delirium in the context of intensive care medicine-part 2: diagnosis, prevention and treatment. Nervenarzt. (2023) 94:99–105. doi: 10.1007/s00115-022-01399-8
4. Clegg, A, Siddiqi, N, Heaven, A, Young, J, and Holt, R, Cochrane Dementia and Cognitive Improvement Group. Interventions for preventing delirium in older people in institutional long-term care. Cochrane Database Syst Rev. (2014) 1:Cd009537. doi: 10.1002/14651858.CD009537.pub2
5. Svenningsen, H, Egerod, I, Videbech, P, Christensen, D, Frydenberg, M, and Tønnesen, EK. Fluctuations in sedation levels may contribute to delirium in ICU patients. Acta Anaesthesiol Scand. (2013) 57:288–93. doi: 10.1111/aas.12048
6. Nydahl, P, Baumgarte, F, Berg, D, Bergjan, M, Borzikowsky, C, Franke, C, et al. Delirium on stroke units: a prospective, multicentric quality-improvement project. J Neurol. (2022) 269:3735–44. doi: 10.1007/s00415-022-11000-6
7. Qu, J, Chen, Y, Luo, G, Zhong, H, Xiao, W, and Yin, H. Delirium in the acute phase of ischemic stroke: incidence, risk factors, and effects on functional outcome. J Stroke Cerebrovasc Dis. (2018) 27:2641–7. doi: 10.1016/j.jstrokecerebrovasdis.2018.05.034
8. Gusmao-Flores, D, Salluh, JIF, Chalhub, R, and Quarantini, LC. The confusion assessment method for the intensive care unit (CAM-ICU) and intensive care delirium screening checklist (ICDSC) for the diagnosis of delirium: a systematic review and meta-analysis of clinical studies. Crit Care. (2012) 16:R115. doi: 10.1186/cc11407
9. Mansutti, I, Saiani, L, Cargnelutti, D, Petrucco, S, Giannina, V, di Domenico, C, et al. Delirium prevalence, risk factors and outcomes among patients with acute stroke: a multi-centre observational study. J Vasc Nurs. (2022) 40:172–80. doi: 10.1016/j.jvn.2022.09.003
10. Oldenbeuving, AW, de Kort, PLM, Jansen, BPW, Algra, A, Kappelle, LJ, and Roks, G. Delirium in the acute phase after stroke: incidence, risk factors, and outcome. Neurology. (2011) 76:993–9. doi: 10.1212/WNL.0b013e318210411f
11. Zipser, CM, Deuel, JW, Held, JPO, Ernst, J, Schubert, M, Weller, M, et al. Economic impact of poststroke delirium and associated risk factors: findings from a prospective cohort study. Stroke. (2021) 52:3325–34. doi: 10.1161/STROKEAHA.120.033005
12. Miu, DK, and Yeung, JC. Incidence of post-stroke delirium and 1-year outcome. Geriatr Gerontol Int. (2013) 13:123–9. doi: 10.1111/j.1447-0594.2012.00871.x
13. Xiong, X, Fan, M, Ma, J, and Deng, J. Association of atrial fibrillation and cardioembolic stroke with poststroke delirium susceptibility: a systematic review and meta-analysis of observational studies. World Neurosurg. (2022) 167:e378–85. doi: 10.1016/j.wneu.2022.08.025
14. Carin-Levy, G, Mead, GE, Nicol, K, Rush, R, and van Wijck, F. Delirium in acute stroke: screening tools, incidence rates and predictors: a systematic review. J Neurol. (2012) 259:1590–9. doi: 10.1007/s00415-011-6383-4
15. Ringleb, P., Köhrmann, M, and Jansen, O. S2e-Leitlinie: Akuttherapie des ischämischen Schlaganfalls, 2022 Version 1.1, in: DGNeurologie. (Accessed July 22, 2023).
16. Quinn, TJ, Richard, E, Teuschl, Y, Gattringer, T, Hafdi, M, O’Brien, JT, et al. European Stroke Organisation and European Academy of Neurology joint guidelines on post-stroke cognitive impairment. Eur Stroke J. (2021) 6:I–XXXVIII. doi: 10.1177/23969873211042192
17. Nydahl, P, Jeitziner, MM, Vater, V, Sivarajah, S, Howroyd, F, McWilliams, D, et al. Early mobilisation for prevention and treatment of delirium in critically ill patients: systematic review and meta-analysis. Intensive Crit Care Nurs. (2023) 74:103334. doi: 10.1016/j.iccn.2022.103334
18. Nydahl, P, Dewes, M, Dubb, R, Hermes, C, Kaltwasser, A, Krotsetis, S, et al. Survey among critical care nurses and physicians about delirium management. Nurs Crit Care. (2018) 23:23–9. doi: 10.1111/nicc.12299
19. Krotsetis, S, Nydahl, P, Dubb, R, Hermes, C, Kaltwasser, A, and von Haken, R. Status quo of delirium management in German-speaking countries: comparison between intensive care units and wards. Intensive Care Med. (2018) 44:252–3. doi: 10.1007/s00134-017-4945-3
20. Fazekas, F, Chawluk, JB, Alavi, A, Hurtig, HI, and Zimmerman, RA. MR signal abnormalities at 1.5 T in Alzheimer's dementia and normal aging. AJR Am J Roentgenol. (1987) 149:351–6. doi: 10.2214/ajr.149.2.351
21. Rudilosso, S, San Román, L, Blasco, J, Hernández-Pérez, M, Urra, X, and Chamorro, Á. Evaluation of white matter hypodensities on computed tomography in stroke patients using the Fazekas score. Clin Imaging. (2017) 46:24–7. doi: 10.1016/j.clinimag.2017.06.011
22. European Delirium Association, American Delirium Society. The DSM-5 criteria, level of arousal and delirium diagnosis: inclusiveness is safer. BMC Med. (2014) 12:141. doi: 10.1186/s12916-014-0141-2
23. Müller, A, Weiß, B, and Spies, CD. Symptomatic treatment of delirium, anxiety and stress, and protocol based analgesia, sedation and management of sleep in intensive care patients. Anasthesiol Intensivmed Notfallmed Schmerzther. (2015) 50:698–703. doi: 10.1055/s-0041-107321
24. Shaw, R, Drozdowska, B, Taylor-Rowan, M, Elliott, E, Cuthbertson, G, Stott, DJ, et al. Delirium in an acute stroke setting, occurrence, and risk factors. Stroke. (2019) 50:3265–8. doi: 10.1161/STROKEAHA.119.025993
25. Ely, EW, Inouye, SK, Bernard, GR, Gordon, S, Francis, J, May, L, et al. Delirium in mechanically ventilated patients: validity and reliability of the confusion assessment method for the intensive care unit (CAM-ICU). JAMA. (2001) 286:2703–10. doi: 10.1001/jama.286.21.2703
26. Vater, V, Olm, HP, and Nydahl, P. Delirium in stroke: systematic review and meta-analysis. Med Klin Intensivmed Notfmed. (2023). doi: 10.1007/s00063-023-01013-y
27. Liu, SW, Lee, S, Hayes, JM, Khoujah, D, Lo, AX, Doering, M, et al. Head computed tomography findings in geriatric emergency department patients with delirium, altered mental status, and confusion: a systematic review. Acad Emerg Med. (2023) 30:616–25. doi: 10.1111/acem.14622
28. Kostalova, M, Bednarik, J, Mitasova, A, Dušek, L, Michalcakova, R, Kerkovsky, M, et al. Towards a predictive model for post-stroke delirium. Brain Inj. (2012) 26:962–71. doi: 10.3109/02699052.2012.660510
29. Nakamizo, T, Kanda, T, Kudo, Y, Sugawara, E, Hashimoto, E, Okazaki, A, et al. Development of a clinical score, PANDA, to predict delirium in stroke care unit. J Neurol Sci. (2020) 415:116956. doi: 10.1016/j.jns.2020.116956
30. Oldenbeuving, AW, de Kort, PLM, van Eck van der Sluijs, JF, Kappelle, LJ, and Roks, G. An early prediction of delirium in the acute phase after stroke. J Neurol Neurosurg Psychiatry. (2014) 85:431–4. doi: 10.1136/jnnp-2013-304920
31. Pohl, M, Hesszenberger, D, Kapus, K, Meszaros, J, Feher, A, Varadi, I, et al. Ischemic stroke mimics: a comprehensive review. J Clin Neurosci. (2021) 93:174–82. doi: 10.1016/j.jocn.2021.09.025
32. Kappenschneider, T, Meyer, M, Maderbacher, G, Parik, L, Leiss, F, Quintana, LP, et al. Delirium-an interdisciplinary challenge. Orthopade. (2022) 51:106–15. doi: 10.1007/s00132-021-04209-2
33. Cortés-Beringola, A, Vicent, L, Martín-Asenjo, R, Puerto, E, Domínguez-Pérez, L, Maruri, R, et al. Diagnosis, prevention, and management of delirium in the intensive cardiac care unit. Am Heart J. (2021) 232:164–76. doi: 10.1016/j.ahj.2020.11.011
34. von Hofen-Hohloch, J, Awissus, C, Fischer, MM, Michalski, D, Rumpf, JJ, and Classen, J. Delirium screening in neurocritical care and stroke unit patients: a pilot study on the influence of neurological deficits on CAM-ICU and ICDSC outcome. Neurocrit Care. (2020) 33:708–17. doi: 10.1007/s12028-020-00938-y
35. Reznik, ME, Daiello, LA, Thompson, BB, Wendell, LC, Mahta, A, Potter, NS, et al. Fluctuations of consciousness after stroke: associations with the confusion assessment method for the intensive care unit (CAM-ICU) and potential undetected delirium. J Crit Care. (2020) 56:58–62. doi: 10.1016/j.jcrc.2019.12.008
Keywords: delirium, acute stroke, prediction score, stroke unit, intensive care unit, post-stroke delirium
Citation: Wischmann J, Kremer P, Hinske L, Tomasi R, Becker-Pennrich AS and Kellert L (2023) The RAPID-score: Risk Assessment and PredIction of Delirium in acute stroke patients based on very early clinical parameters. Front. Neurol. 14:1306520. doi: 10.3389/fneur.2023.1306520
Edited by:
Christoph Gumbinger, Heidelberg University, GermanyReviewed by:
Annerose Maria Mengel, University of Tübingen, GermanyOmer Ibrahimagic, University Clinical Center Tuzla, Bosnia and Herzegovina
Copyright © 2023 Wischmann, Kremer, Hinske, Tomasi, Becker-Pennrich and Kellert. This is an open-access article distributed under the terms of the Creative Commons Attribution License (CC BY). The use, distribution or reproduction in other forums is permitted, provided the original author(s) and the copyright owner(s) are credited and that the original publication in this journal is cited, in accordance with accepted academic practice. No use, distribution or reproduction is permitted which does not comply with these terms.
*Correspondence: Johannes Wischmann, am9oYW5uZXMud2lzY2htYW5uQG1lZC51bmktbXVlbmNoZW4uZGU=
†These authors have contributed equally to this work