- 1Department of Neurobiology, School of Medicine, Neuroscience Institute, Atlanta, GA, United States
- 2Department of Chemistry and Biochemistry, Texas Tech University, Lubbock, TX, United States
- 3Department of Biological Sciences Faculty of Science, Kuwait University, Safat, Kuwait
- 4Department of Pathology and Anatomical Sciences, University of Missouri School of Medicine, Columbia, MO, United States
- 5Alabama College of Osteopathic Medicine, Dothan, AL, United States
- 6Division of Neurobiology, Free University of Berlin, Berlin, Germany
- 7Department of Biomedical and Dental Sciences and Morphofunctional Imaging, University of Messina, Messina, Italy
Neuroproteomics, an emerging field at the intersection of neuroscience and proteomics, has garnered significant attention in the context of neurotrauma research. Neuroproteomics involves the quantitative and qualitative analysis of nervous system components, essential for understanding the dynamic events involved in the vast areas of neuroscience, including, but not limited to, neuropsychiatric disorders, neurodegenerative disorders, mental illness, traumatic brain injury, chronic traumatic encephalopathy, and other neurodegenerative diseases. With advancements in mass spectrometry coupled with bioinformatics and systems biology, neuroproteomics has led to the development of innovative techniques such as microproteomics, single-cell proteomics, and imaging mass spectrometry, which have significantly impacted neuronal biomarker research. By analyzing the complex protein interactions and alterations that occur in the injured brain, neuroproteomics provides valuable insights into the pathophysiological mechanisms underlying neurotrauma. This review explores how such insights can be harnessed to advance personalized medicine (PM) approaches, tailoring treatments based on individual patient profiles. Additionally, we highlight the potential future prospects of neuroproteomics, such as identifying novel biomarkers and developing targeted therapies by employing artificial intelligence (AI) and machine learning (ML). By shedding light on neurotrauma’s current state and future directions, this review aims to stimulate further research and collaboration in this promising and transformative field.
Highlights
- Neuroproteomics represents a new arrow to the precision medicine bow, allowing us to characterize neurological disorders more precisely and tailor medical treatments to specific individual patient needs.
- Combination of neuroproteomics with novel powerful computational tools and artificial intelligence can address the highly complex networks that underlie pathobiological mechanisms of brain injury.
1 Introduction
1.1 General overview of neuroproteomics
Several platforms have been used for decades to carry out protein-specific research. Proteomics has been introduced to study the proteome of a given biological system’s expression, interaction, functions, and modifications (1). Among the primary endeavors of proteomics include identifying and discovering new molecular protein hits that can indicate a specific homeostatic state. Over its development, proteomics has become an optimal approach for accurate diagnostic and prognostic technology, reflected in the logarithmic advancement in this field and its technological applications (2–5).
The vast history of neuroproteomics has developed over a long period, dating back to the initial development of genome and genetic studies. The human genome project paved the way for initiating thes human proteome project. This project explored the human proteome’s many biological and functional properties associated with approximately 20,300 protein-coding genes (6). As such, this allowed for studying the role of these gene-coded proteins in both healthy and pathological conditions using proteomics, systems biology, and bioinformatics tools (7). These previous projects highlighted the proteome’s complexity, where a single gene can translate into several protein isoforms (2, 8). Several factors contributing to this diversity in protein isoforms include alternative splicing and post-translational modifications (PTMs).
The modern field of proteomics is complementary to the genomics field. Proteomics represents a downstream transition of the genome map and has been used to evaluate the biological system’s genotype signature (8). By validating the translation of its proposed altered genomic map and assessing the phenotypic output, we can link the genome with the proteome. Nevertheless, this association may be affected by several confounding factors, such as different physiological compartments, conditions, PTMs, and other external factors, thereby leading to different protein structures and chemical isoforms (2).
Proteomics studies are conducted in-vivo and in-vitro through various approaches and can create models for multiple conditions related to protein concentration levels and structure modifications. PTMs are crucial for the characterization and analysis of numerous diseases, including neurodegenerative disorders (9).
Ultimately, proteomics’ development spans a vast history and has developed a new and more efficient technique for protein identification. Today, proteomics research and technological advancements can be paired together to advance precision medicine and clinical applications. With the introduction of Artificial Intelligence (AI) and Machine Learning (ML), technology can organize a more accurate form of personalized medicine, providing the most accurate implications for individualized and unique treatment options for every patient (10). This review will discuss several aspects of proteomics and link its potential role in technology-based personalized medicine.
Neuroproteomics is a field that studies the nervous system to understand disorders like neuropsychiatric, degenerative disorders, and neurotrauma-related injuries (11–13), i.e., traumatic brain injuries (TBI) (14), spinal cord injury (SCI) (15), and stroke (16). It is classified into four categories: Expression Neuroproteomics, Functional Neuroproteomics, Clinical Neuroproteomics, and Neuroproteomics Informatics (2). The first category focuses on profiling the proteome, the second one investigates the functional properties of individual sets of proteins (9), the third aims at discovering drugs and novel biomarkers for pathological conditions (17, 18), and the fourth is dedicated to computational tools and specific databases enabling the analysis of proteomics data sets (19–22). With information from all four categories, a neuroscientist can propose new algorithms and faster outputs to conduct clinical prognosis and diagnosis.
In the neuroproteomics fields’ development and advancements, some significant challenges arise from the CNS (23). Analyzing the CNS is difficult due to the presence of over 20,000 proteins in the brain that are differentially expressed within different regions. Overall, it becomes incredibly challenging to comprehensively study the brain proteome and its dynamic function without using high-resolution protein identification and separation techniques. Neuroproteomics composition analysis challenges have arisen with the neural complexity created by the network structure of axons, dendrites, and synapses. Challenges in neuroproteomics composition include difficulty analyzing different brain regions due to limitations in the number of samples to be obtained for analysis (24). Therefore, identifying proteins that are expressed in small quantities is exceptionally challenging. Technological advancements, clinical trials, and precision tools have been developed to combat these challenges in the neuroproteomics (25).
In continuation, due to the nonlinear relation between the genome and the proteome, it is challenging to draw a direct correlation and association between mRNA expression and protein translation (2, 3, 26–30). This is attributable to different factors, including alternative splicing, which is highly frequent in brain tissue, generating thousands of copies of positively related splices from a single gene. For example, the protein Cadherin has 18 different isoforms linked to morphogenic and functional roles in developing the nervous system (27, 30–32). An average of 10 protein isoforms can be generated within a single gene, owing to the proteome’s complexity compared to the genome (33, 34). This complexity is amplified by the numerous dynamic PTMs, which can reach around 400 possible modifications (27, 35).
Mass spectrometry (MS)-based proteomics has proven to be an indispensable tool for molecular and cellular biology, as well as for the emerging field of system biology (36). It has been successful in a variety of applications, including studying protein–protein interactions, mapping organelles, and generating quantitative protein profiles from diverse species (36). With its ability to identify and quantify thousands of proteins from complex samples, MS is expected to have a significant impact on the fields of biology and medicine (36). Classic approaches in proteomics use MS coupled with advanced separation techniques to analyze protein interactions and structures (37–39). MS-based techniques involve bottom-up or top-down analysis (40). In the former analysis, proteins undergo enzymatic digestion first, followed by fragment identification via shotgun-proteomics methods that involve nanoflow liquid chromatography (nanoLC) (41). However, the latter goes without enzymatic digestion, where the entire intact protein undergoes analysis. Interestingly, other tagging techniques have been coupled to MS, such as isobaric tags for relative and absolute quantitation (iTRAQ) (42) and stable isotope labeling with amino acids in cell culture (SILAC) (43). These allow for proteomics changes and PTM assessment analyzes as with phosphorylation-dependent activation. T.
Another field approach relies on antibody-based techniques without MS and involves targeted biomarker proteins through an antibody panel or array platforms (24, 44). Although this offers high specificity and sensitivity in identifying the proteins, it cannot identify novel protein biomarkers. These technologies include high-throughput immunoblotting (HTPI) (45, 46) and antibody panel/microarray (47). The former utilizes unstable channels where samples can be identified via immunoblotting systems that use PAGE followed by an antibody probing. The latter technology is based on DNA microarrays or ELISA arrays, where pre-labeled proteins in samples with differential fluorescent dyes are probed against an antibody platform. A mixture of qualitative characterization (PTM, disease characterization, injury severity scores) and quantitative techniques (MS, iTRAQ, SILAC, ELISA, immunoglobulin assays). Figure 1 illustrates the general proteomics quantification methodologies. Neuroproteomics utilizes many molecular techniques to quantify and characterize protein concentration and quantity. SDS-Page Gel Separation (Western Blot), ELISA, Mass Spectrometry, Bioinformatic data, and neuroimaging correlations utilize quantitative data collection methods within proteomics.
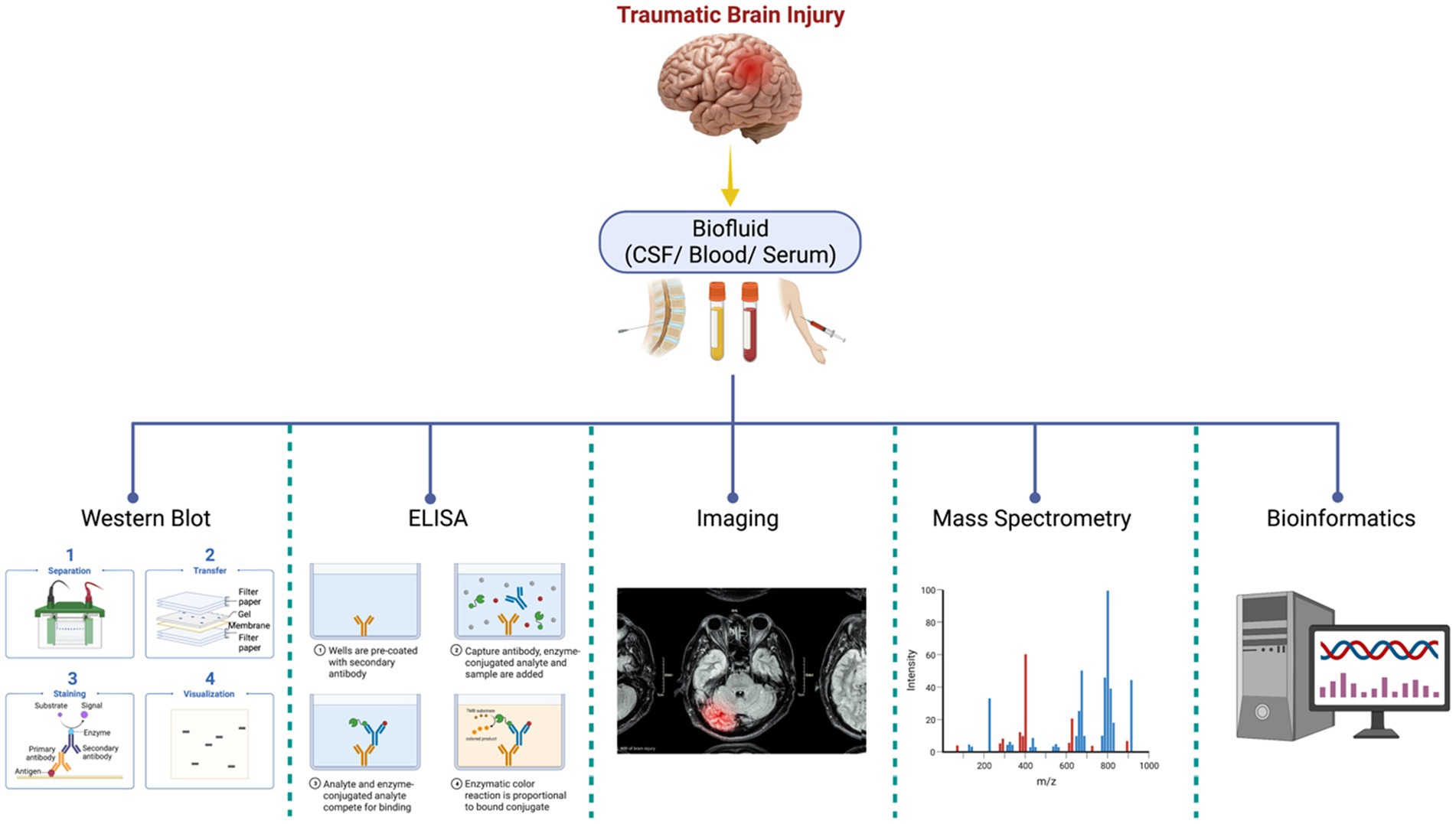
Figure 1. Neuroproteomics quantification methodologies. Neuroproteomics utilizes many molecular techniques to quantify and characterize protein concentration and quantity. SDS-Page Gel Separation (Western Blot), ELISA, Mass Spectrometry, Bioinformatic data, and neuroimaging correlations utilize quantitative data collection methods within proteomics.
Neuroproteomics is a process that analyzes the concentration and structure of different biomarkers using specific protocols and methodologies. The process involves extracting biosamples through invasive and non-invasive procedures, collecting biofluids for analysis, and identifying and quantifying proteins. Scientists use various methods, such as SDS-PAGE, Western blots, and mass spectrometry, to identify and quantify the proteins. They also use bioinformatics and machine databases to confirm and classify the quantified proteomics datasets (2, 11). By analyzing potential biomarkers and evaluating their concentration under independent conditions, scientists can pinpoint what is causing neurodegeneration. Neuroproteomics helps neuroscientists diagnose conditions efficiently and identify novel therapeutic targets to develop personalized medicine (48). Figure 2 presents the general neuroproteomics workflow for discovering disease biomarkers.
Importantly, the advancement and adaptation of these technologies can permit the characterize PTM, such as glycosylation. This largely unexplored field has great potential for the identification of novel biomarkers while uncovering their biological and pathobiological role. In the next section, we focus on this specific aspect illustrating proteomics methods and approaches to accurately and reliably identify and profile the brain glycoproteome.
1.2 Protein glycosylation
Glycosylation is one of the most predominant post-translational modifications of proteins. As protein sequencing data suggest, the glycosylation rate of mammalian cell proteins is estimated to be greater than 50% (49–51). In the glycosylation process, carbohydrates are added to lipids, proteins, and other organic molecules within or outside the cells (49–51). Glycosylation is a tightly regulated process, as it is a site-specific enzymatic modification (52). A variety of protein properties, such as solubility, are affected by glycans of secreted glycoproteins, whereas cell surface glycosylated proteins have been implicated in various cellular processes, such as cell-to-cell communication (53). The major glycans of glycoproteins are classified into two groups based on their glycan–peptide bonds, namely the N-glycan and O-glycan. The amino sugar N-acetylglucosamine (GlcNAc) is linked to the asparagine amide group to form N-glycans, whereas O-glycans are linked to the hydroxyl groups of the serine and threonine amino acids of polypeptides by N-acetylgalactosamine (49–51, 54).
Aberrant protein glycosylation has been linked to many diseases such as Alzheimer’s Disease (AD) (55, 56), TBI (57), Parkinson’s Disease (PD) (58), congenital/metabolic disorders (59, 60), diabetes (61, 62), inflammation (63), bacterial/viral infectious diseases (64–66), and various cancers (67–71). Aside from this, due to structural heterogeneity, the presence of isomeric glycans complicates the structural analysis of glycans and glycoproteins (72). It is also known that structural heterogeneity affects the biological roles of these glycans/glycoproteins in various diseases (73). Hence, studying the isomeric forms of glycans and glycopeptides is crucial. The importance of proteins and glycoproteins in biological processes, as well as the correlation between their altered expression and a wide range of diseases, makes proteomics and glycoproteomics promising frontiers in the development of biomarkers (74). These biomarkers offer unparalleled opportunities for refinement in clinical characterization and improve disease phenotyping. Such improved characterization and stratification will enable more targeted treatments.
As the first step for the characterization of glycosylated proteins, they need to be isolated from complex biological samples that include both glycosylated and nonglycosylated proteins. Upon isolation, glycoproteins/glycopeptides are enriched, digested by proteolysis, and detected/identified via mass spectrometry-based techniques using glycoproteomics platforms, a subset of the proteomics (75). For the purification and separation of glycoproteins, various types of HPLC are available, including ion exchange methods, hydrophobic interactions, size exclusions, and affinity chromatography. It is necessary to develop fast and robust analytical techniques to study the altered glycosylation profiles induced by a specific disease (76). A number of techniques have previously been used to characterize glycoproteins, including lectin affinity, hydrazide chemistry, and peptide or protein enrichment, which involves deglycosylation and other chemical modifications (77, 78).
It is pertinent to note that mass spectrometry provides valuable information regarding proteins with PTMs, and the importance of this is especially evident when comparing two or more samples quantitatively. Detecting changes in disease-associated glycosylation patterns requires sensitive, quick, reliable, and robust analytical techniques. Even though many methods are available for identifying glycoproteins, glycoproteomics remains a challenging field that holds much promise. With the advent of MS techniques, glycoprotein profiling has been significantly enhanced, especially when dealing with complex samples such as plasma, serum, and body fluids (77, 79). Recent advancements in MS technology have also made it possible to use more accurate approaches to characterize glycoproteins. A glycopeptide-based analysis provides site-specific information regarding the location of the glycan attachment on the protein, which is then used to determine its putative functional role and properties. Figure 3 illustrates the general workflow for analyzing glycoproteins in neurotrauma samples using separation techniques coupled with mass spectrometry.
1.3 Peptidomics
Peptidomics is a branch of proteomics that focuses on endogenous peptide fragments and is responsible for studying all peptides in a biological sample (80, 81). Peptidomics is considered a separate domain with its applications and analytic approaches (82). The term peptidomics was first used in February 2000 at the Association of Biomolecular Resource Facilities (ABRF) conference (83–85). The peptides’ life cycle is controlled by different processes and events, primarily determined by proteases and opposed-regulated protease inhibitors (85). Although peptidomics does not need enzymatic digestion in the preparation step, it is much more complicated than proteomics. A peptide in a peptidome analysis does not contain a uniform basic C-terminal (Lys/Arg) because of the absence of tryptic digestion used in proteomics approaches (86).
The ultimate aim is generally to classify all peptides, even though they originated from the same precursor (87, 88). Both fields, proteomics and peptidomics share the same limitation in dealing with the changeable features of the proteins and peptides. In a biological sample, peptidomics examination is usually hindered by protein degradation (enzymatically or non-enzymatically), interfering with the original peptides in that sample. Peptides are generally found at low levels, making any minor degradation of proteins interfere with the endogenous peptides’ signals in the MS analysis (89). To overcome this limitation, an affinity column for peptide enrichment can be used (90, 91). Moreover, heating tissues during extraction via an ordinary microwave oven result in the rapid inactivation of the proteases responsible for the protein degradation (92, 93). Tissues high in peptides and low in digestive enzymes, e.g., the pituitary, act as perfect targets (92, 93).
Neuropeptides affect several physiological functions, including body weight, sleep, anxiety, learning, and reward systems (94). Previously, neuropeptides were identified and quantified using several methods; one of these techniques was the radioimmunoassay (RIA) (95). It is an antibody-based sensitive technique that is not unique to a particular isoform of peptide. RIAs cannot distinguish between modifications if a specific antibody for the different isoforms is used (phosphorylation, sulfation, acetylation, and glycosylation) (96). The N-terminal sequences of proteins or peptides are determined using the Edman sequencing (97). The automated Edman degradation method was utilized in several different samples to sequence the neuropeptides (97). Although it was precise and its analysis was clear, it can only study pure peptides and remove peptides with N-terminal modifications (as a result of acetylation, formylation, or pyroglutamination, for example). Consequently, RIA has been entirely replaced by MS, which has higher throughput and sensitivity even with protein mixtures (98). MS usually use one of two standard techniques; the first one is electrospray ionization (ESI), which can produce multiple charge state for the separated ions, and the second one is MALDI, which lessen the complexity of identification because of single charged ion. Usually, peptides less than 10en kilodaltons (kDa) cannot be detected in a two-dimensional product (58, 60). polyacrylamide gel electrophoresis (2D-PAGE) that is used in the proteomics approach (99). High-performance liquid chromatography (HPLC) is a more reliable, precise, and easily automated technique that is considered adequate for separating peptides from mixtures (100). Micro or nanoscale LC avoids peptide co-elution in complicated mixtures and improves the low abundance LMW peptides identification (101).
Peptidomics reported significant progress, especially in neuropeptide studies, enabling the isolation and the classification of thousands of neuropeptides in one controlled experiment using LC–MS/MS. (102) Neuropeptidomics is a broad term for a method of characterizing neuropeptides on a global scale, often under particular physiological conditions. The first comprehensive analysis was achieved by Anna Secher et al. (103). Numerous (full-length) neuropeptides were analyzed by single LC–MS/MS analysis for 32 rats by perfusion protease inhibitors, which resulted in the identifications of 14,416 unique peptide sequences (104). The list of peptides was classified by their involvement in protein groups according to an established mammalian orthologous community structure to differentiate between neuropeptides and peptides produced from tissue damage (105). This allowed an overall grouping of high data levels from publicly accessible databases of proteins across different species (Uniprot.org, SwePep, and Neuropeptides.nl) (104). The initial peptide identification approach is based on data-dependent shotgun sequencing that compares the generated tandem mass spectra to an entire proteome database (103).
There are two types of peptidomics studies that can conclude the discovered peptides’ function. The first one is adhering to a singular cell or a specific cell type with a known function (such as pancreatic beta cells). The second one indicates the peptidomics quantifications (89). Neuropeptides levels, which can be affected by food intake, are directly correlated with physiological conditions. In fact, peptides can be considered as modulators for energy balance (89). MS can give the relative amount or intensity of each peptide either by isotope labeling and/or label-free quantification approaches (89), which will be discussed in the following sections.
Peptidome analysis was used to study and classify differentially expressed peptides in neonates with hypoxic–ischemic brain injury (HIBD) or controls in cerebrospinal fluid (CSF) in order to provide a basis for identifying new promising neonatal HIBD treatments (106). A total of 35 differentially expressed peptides were detected using (ITRAQ LC–MS/MS). A fragment of heat shock protein 90-alpha (HSP90α/HSP90AA1) has been shown to be a decreased peptide in neonatal HIBD (HSQFIGYPITLFVEKER) (106). This peptide, called Hypoxic–ischemic brain damage-associated peptide (HIBDAP), is a highly stable hydrophilic peptide in mammalian reticulocytes and has a 3.5-h half-life. This identification may significantly affect and contribute to the development of novel therapeutic targets for neonatal HIBD. Control CSF samples were taken from comparable infants with no identified neurological condition (106).
1.4 Proteomics and peptidomics technologies in personalized medicine
The primary aim of personalized medicine is to define diseases more accurately to support precise diagnosis and individualized medical treatments tailored to the patient characteristics and needs, ultimately maximizing efficiency and benefits (48). Proteomics-based personalized medicine is more complicated than genetic medicine and other omics fields because of the proteome’s complex nature and its dynamic components, PTM, tissue, cellular, and organelle-specific expression. Besides, the proteome profile differs in a healthy state compared to a disease state, especially in neurodegenerative diseases (107, 108).
Biomarkers are crucial to the advancement of personalized medicine. Since they may be used as a starting point for drug development and diagnosis, protein and peptide biomarkers may be used for early diagnosis and prevention strategies (109). They can also be used to monitor a patient’s reaction to therapy. Proteomics and peptidomics technology advancements may have aided the improvement of personalized medicine by recognizing protein and peptide biomarkers and improving biochemical diagnostics. The connection between diagnosis and treatment is critical for personalized medicine; proteomics and peptidomics are expected to show high efficiency in linking diagnostics and therapeutics (110). Further research is needed in this field.
1.5 Single-cell proteomics
Single-cell proteomics (SCP) is a technique used to understand the phenotype of a specific cell, which is a result of genetic interactions within this cell. Such a technique enabled the identification of a vast amount of proteins being expressed in a single cell at a certain point in time (111). Protein analysis of a single cell may show the presence or the progression of a disease through the detection of protein concentration in the blood or the detection of PTMs of a specific protein. Also, the change in the structure or the kinetics of a protein could lead to the knowledge of the disease progression, change in the immune response, and cell differentiation (112–114). Cellular heterogeneity denotes the protein expression in a disease or healthy state in which these proteins can be encountered as parameters in identifying the pathology of the disease (115). There are many methods used to detect proteins within a cell “proteome,” such as chromatography (e.g., liquid chromatography), gel electrophoresis (e.g., SDS-PAGE), and MS-based methods. Although these methods are potent, they are incompatible with single-cell detections, so it is unhelpful in the cell heterogeneity (1, 116).
SCP is a technology that identifies the complete protein profile within a single cell, the target cell, at a specific condition or time (111, 117). The benefits of single-cell proteomics rely on expanding our knowledge for understanding cellular functions and regulation with the characterization of protein phenotypes within the cell. A single-cell analysis requires sensitive methods and an optimized workflow starting with efficient sample isolation, liquid-phase separation followed by ionization, gas-phase separation, and MS. (118)
To perform SCP, the single cell must be first isolated from a population of cells. The properties of the isolated cell must be established, such as cell size, cell density, and antigen status (111, 117). Techniques used in cell isolation for SCP are fluorescence-activated cell sorter, microfluidics, limiting dilution, manual cell picking, high-density microarray, laser capture microdissection, aptamer binding, and central magnetic-activated cell sorting (MACS) (113, 119) (Figure 4). Many reagents are used in the sample preparation for SCP, such as dithiothreitol (DTT), iodoacetamide (IAA), trypsin, and urea.
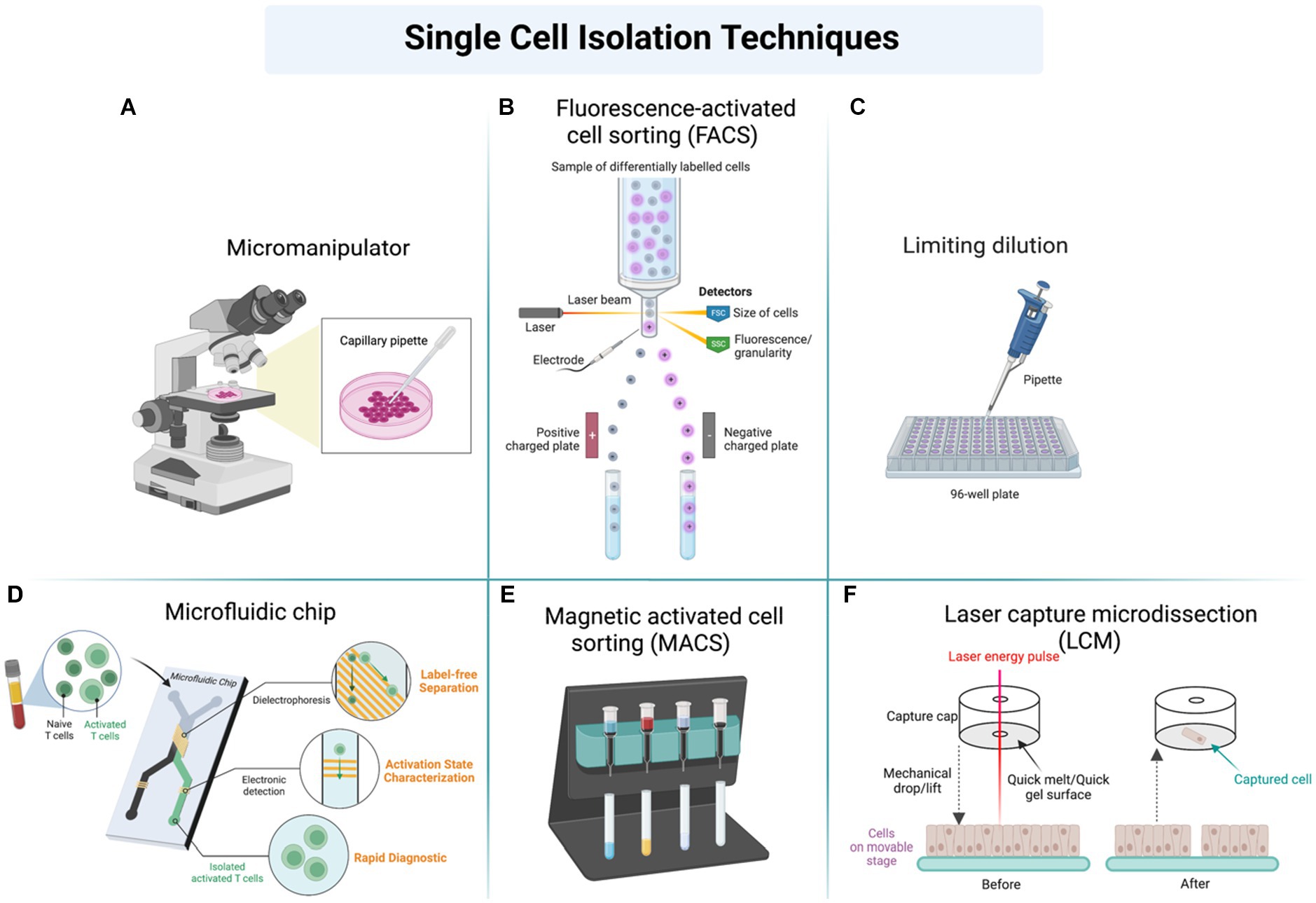
Figure 4. Different techniques used in sample preparation and cell isolation for further analysis of single-cell proteomics (SCP) by using (A) Micromanipulator, (B) Fluorescence-activated cell sorting (FACS), (C) Limiting dilution, (D) Microfluidic chip, (E) Magnetic activated cell sorting, (F) Laser capture microdissection (LCM).
The separation of proteins is a necessary step in SCP, and it can be performed either using gel chromatography or electrophoresis. These conventional methods are impractical for the detection of low-abundant proteins. Other methods, such as capillary electrophoresis (CE) or microfluidic chips, are more precise in separating the whole proteome in a single cell (120).
The SCP’s analytical tools can be divided into either qualitative or semi-qualitative. An example of the qualitative method is the ELISpot technique, an antibody-based method where the antibody–antigen interaction detects the secreted proteins (121). The other semi-qualitative tool includes the flow cytometry methods (imaging and mass-based techniques) that detect many proteins expressed in a single cell (122–124). Techniques in the label-free analysis of SCP include matrix-assisted laser desorption/ionization (MALDI-MS), electrospray ionization MS (ESI-MS), secondary ion MS (SIMS), and laser/desorption/ionization (LDI-MS) (125, 126).
There are two alternative methods in sample preparation for the LC–MS label-free quantification of a single cell. These preparative methods, called integrated proteome analysis devices (iPad), inject the cell into the capillary (lysed and digested) that is coupled to nano LC–MS/MS. (127) It is very accurate in determining cell heterogeneity (128). The other method for the SCP sample preparation is using a pre-treated chip called the nanoliter-scale oil-air droplet (OAD). The OAD is based on microfluidics combined with proteomics shotgun analysis (129).
SCP in neurology was performed to assess microglial activation within TBI-induced animal models. Single-cell quantitative measurements assessed the activated and inactivated microglia. The morphology was changed in neurons following TBI insult, and the unaffected neurons had normal microglial density and morphology (130).
1.6 Quantitative proteomics
Quantification methods in proteomics include label-based quantification, such as the stable isotope labeling by amino acids in the cell culture (SILAC), tandem mass tag (TMT), iTRAQ, and the isotope-coded affinity tag (ICAT). Label-free mass spectrometric methods are also considered a quantification method (131, 132).
Stable isotopic labeling is one method in proteomics quantitation. It is based on applying a label to the peptides. The difference between labeled and unlabeled is mass, so the labeling can either be introduced in the culture (SILAC) or into fragmented peptides, called chemical labeling (133). The first labeling was introduced into Drosophila and Caenorhabditis elegans in the proteomics field by feeding (134). The SILAC quantitation method is combined with mass spectrometry and bioinformatics. It is well suited to be applied as a biochemistry-based approach (135). The principle of the SILAC is that there are two populations of cells. The first population contains light amino acids (light isotopes), and the other culture contains heavy amino acids (heavy isotopes of nitrogen, oxygen, and carbon). The heavy amino acids of the same peptides have the same properties except for the mass, so the two sets’ proteomes can be distinguishable by the mass shift (135). Many studies were performed to quantify proteins within the brain, such as a study that used SILAC labeling of Neuro2A cells (136). Another study quantified the phosphotyrosine associated with the neurotrophic factor (BDNF) by the concept of SILAC (137). SILAC labeling was also applied in TBI quantification, such as in a study by Wu et al. which aimed to detect and quantify the overall proteome within TBI-induced rat models. 18O-water labeling and mass spectrometry, the study could identify 1,002 common proteins (in control and TBI samples). These proteins are essential in cellular assembly and morphology. The study pointed out that 200 proteins were dysregulated in TBI samples, of which 124 were increased (up-regulated) and 76 were decreased (down-regulated). Up-regulated proteins were involved in the actin-related cytoskeleton and neurons’ structure, development, and transport. Down-regulated proteins were identified as enzymes associated with the glycolytic pathway, Kreb cycle, and oxidation phosphorylation (138).
Chemical labeling, such as iTRAQ, ICAT, and TMT, is another type of quantitation proteomics, called isobaric mass tagging, in which these added mass tags are labeled and detected in case of fragmentation by trypsin (139, 140). The advantage of labeling a peptide (by iTRAQ, ICAT, or TMT) is that this labeled peptide is detected in the mass spectrometry as a single peak, even when one or more samples are mixed (133, 141). iTRAQ proteomics was applied in many areas, including phospho-proteomics of plant cells (142, 143). The most significant advantage of iTRAQ labeling is that it can cover many peptides within the sample, affecting the sample and peptide identification and quantification of up to eight samples simultaneously. This labeling type also improved accuracy in detecting b and y ions in the MS/MS spectrum (132, 144, 145). Alternatively, the ICAT method, which is based on using isotope-coded affinity tags, has the same concept of labeling peptides, but what is unique is that it is specific for the enrichment of cysteine-containing peptides by comparing two samples with either heavy tag or light tag, these tags are labeled (146). The labeling of peptides using the ICAT method is performed at the sample preparation step before the digestion step, and it can be performed enzymatically (trypsin) or chemically. The labeled and digested proteins are then detected by mass spectrometry using peak intensity or peak area (146–148). Additionally, TMTs are also becoming more and more popular for large-scale proteomics studies. Such experiments, which focus on proteoform analysis in drug time courses or perturbation studies or in large patient cohorts, can greatly benefit from the reproducible quantification of single peptides across samples (149).
In TBI, assessing the protein changes in mild TBI patients was an essential step in qualifying and quantifying the proteins compared to a control. A study used chemical labeling (iTRAQ) to screen the global proteome within a rat’s brain. The animal model containing mild TBI (mTBI) showed that 237 proteins were changed significantly. Some of these proteins were associated with cAMP signaling (adenylyl cyclase pathway–a G protein-coupled receptor triggered signaling cascade used in cell communication), and some were associated with cell adhesion, autophagy, myelination, microtubule depolymerization, and brain development (150). In another study, TMT-based proteomics was utilized to screen the potential biomarkers of acute-phase TBI in rats (151). Based on proteomics findings, the acute phase of TBI showed significant influences on oxygen transport, acute-phase response, and negative regulation of endopeptidase activity. Additionally, pathways related to the scavenging of heme from plasma, binding, and uptake of ligands by scavenger receptors were highly enriched in all time points of TBI samples.
The label-free approach is another protein quantifying approach without labeling the peptides, which applies to all biological samples (152). This method needs long computational methods for independent mass spectrometry runs, which is required for data collection and interpretation (153). When label-free quantification methods quantify a mixture of peptides, the detection method can be performed by determining the area under the curve (AUC) or spectral counting (154). The AUC method is based on measuring the peak of an eluted peptide or protein from the chromatography (based on the retention time) (155, 156) (Figure 5). This method’s disadvantage is seen when there are multiple peptide signals. Also, this quantification method seems unhelpful with inaccuracy in the retention time within a specific peptide or the MS spectrum intensity. Also, some non-specific background noise affects the accuracy of the label-free methods (154). The second type, spectral counting, is based on selecting the most abundant peptides available within the sample for further fragmentation and generating spectra. The abundance of the peptide reflects the number of spectra it generates (157). The protein abundance index (PAI) factor calculation estimates the protein abundance, representing the number of peptides divided by the tryptic peptides of such a protein (158). A study developed the proteome platform for post-TBI neurons. The study aimed to report a quantitative assessment platform for the identified peptides using the label-free data-independent acquisition (DIA) method. The quantitation of 18,651 peptides revealed that 3,587 were statistically dysregulated upon TBI induction, and 634 (approximately 18%) were modified by PTM, such as phosphorylation and acetylation methylation (159).
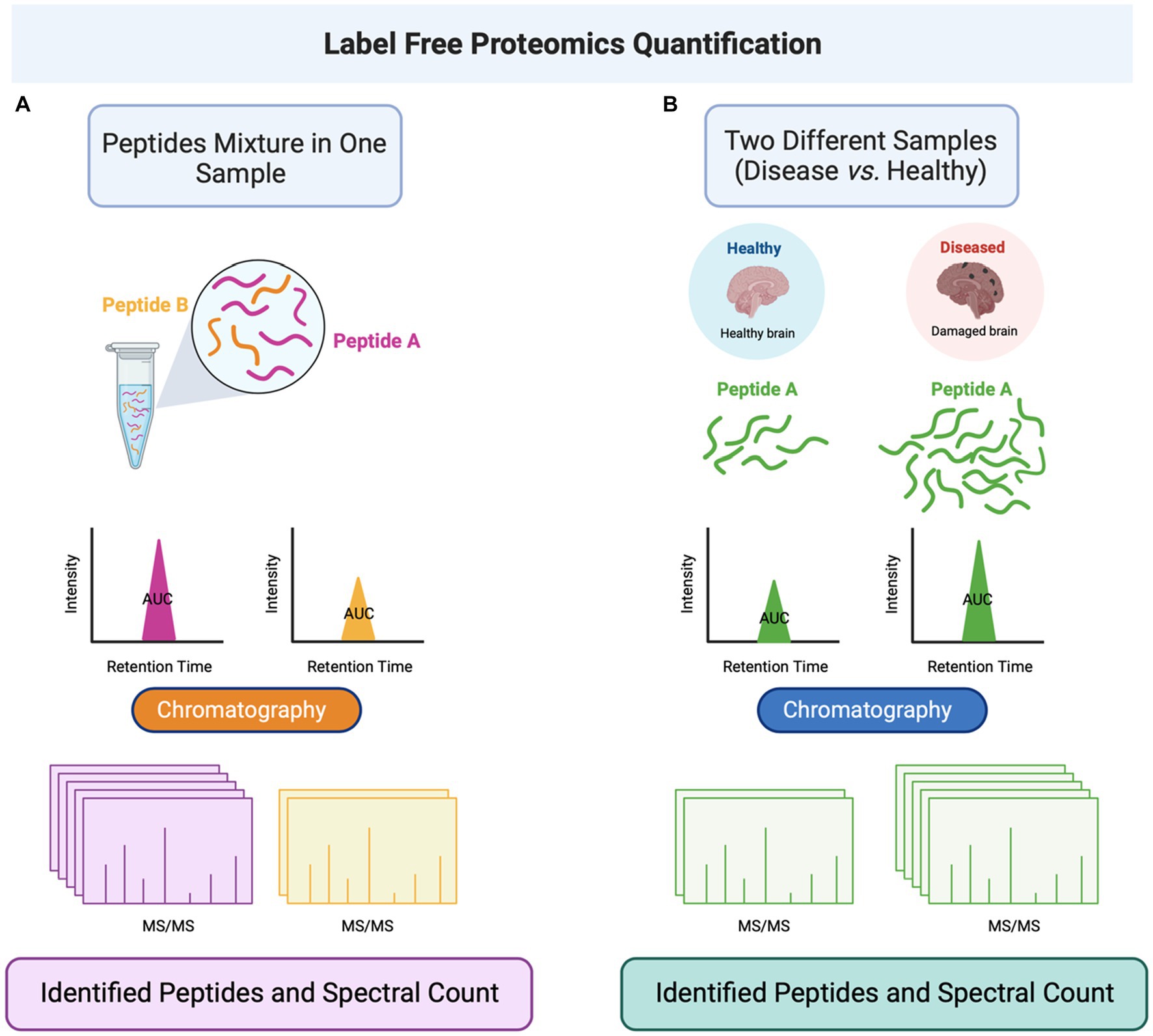
Figure 5. Label-free quantitation proteomics is performed by determining the area under the curve (AUC) of peptides eluted from the liquid chromatography and conjugated to MS/MS. (A) The label-free method can be applied to a single sample containing a mixture of peptides. (B) The method can also be applied to different samples (such as healthy and disease-representing cells) to quantify a specific peptide in the two cell populations. Abbreviations: Tandem mass spectrometry (MS/MS).
Untargeted DIA mass spectrometry was also utilized to study whether examining the trajectory of TBI-responsive peptides secreted into urine could produce a predictive model of functional recovery during TBI rehabilitation (160). The produced models demonstrated high sensitivity and specificity, reflecting neuroplasticity and diminished cell death and neuroinflammation. The models can inform on rehabilitation progress after TBI and warrant further investigation. Besides, the acquired DIA mass spectrometry data used in a study on rats with mTBI showed that repeated injuries caused immediate cognitive problems, long-term movement issues, elevated levels of neurofilament light, changes to proteins in the hippocampus leading to brain inflammation, and widespread changes to white matter (WM) (161). Additionally, the other study aimed to investigate the differential effects of TBI if only the gray matter (GM) is damaged or if the injury also involves the WM. The researchers performed stab wound injuries affecting GM and WM (GM+) and one restricted to the GM (GM-) in the adult murine cerebral cortex and examined glial reactivity in the regions affected. Unbiased proteomics analyzes further corroborate their findings in support of a profound difference in GM reactivity when WM is also injured and revealed MIF as a key regulator of NG2 glia proliferation (162). Another study investigated the changes in axons during brain development in young rats and post-TBI in adult rats (163). The study found multiple similarities in the changes in axonal microtubule (MT) through tubulin post-translational modifications and MT-associated proteins (MAPs), such as tau and MAP6, during both development and TBI. Quantitative proteomics in this study uncovered similar signaling pathways of axon degeneration and growth/repair, including protein clusters and networks (163). This comparison approach shows how a focused examination of developmental processes can provide insight into the pathways initiated by TBI.
It is worth mentioning that neuroproteomics offers a global molecular approach to deducing the complex post-translational processes that underlie secondary events after TBI. By employing the DIA approach, there is a study that assessed the use of artificial neural networks and functional enrichment analyzes to discretize the temporal response across some 2047 significantly impacted proteins and supports the therapeutic promise of KCC2-targeted intervention for positive functional recovery after brain injury (164). Synthetic peptides are also used in quantitation, representing the same amino acid sequence of the desired peptide to be quantified. Stable isotopes can label these synthetic peptides upon proteolytic cleavage, and the labeled isotopic synthetic peptide will represent the amount of peptide within the sample. The advantage of this method is that it has a short analytical time with high throughput results (165, 166).
Many quantitative approaches are employed in proteomics to examine biological materials for relative quantitative proteomics, including label-free data-dependent acquisition (DDA) and isobaric multiplex labeling procedures (using iTRAQ or TMT reagents) (159, 167). Laser microdissection (LMD) or mechanical dissection can be used in neuroproteomics to extract brain areas for data collection from AD brains or control groups (168). DDA or isobaric multiplexed labeling techniques were used for sample preparation for relative quantitative proteomics and precise peptide measurements by LC–MS/MS, along with further data processing (165, 166). MaxQuant (169), Morpheus (170), and Perseus (171) are just a few of the database search engines available. DIA has taken the place of the DDA proteomics approach. For example, missing values can be solved using sequential window acquisition of all theoretical mass spectra (SWATH-MS). Implementing SWATH-MS quantification across the entire MS1 spectrum allows the classification of all measurable peptides within a given mass range. To eliminate missing values, the SWATH-MS approach enables an inclusive and reliable measurement of detected proteins in the sample (172–174). Therefore, MS2 spectra in DIA are more challenging to analyze than DDA spectra and need an advanced and specialized computation (174) to adopt this approach for quantification of complex brain proteomes in progress. This technique analyzes thousands of proteins in 1–2 h (175, 176). A recent study conducted a proteomics analysis on ventricular cerebrospinal fluid (vCSF) proteins following acute brain injury (ABI) and their association with pathophysiological pathways and potential biomarkers that can predict unfavorable outcomes (177). In this study, DIA and SWATH-MS was employed to compare differences in protein expression in patients with ABI and patients without ABI and in patients with traumatic and nontraumatic ABI. The results revealed that an unregulated expression of vCSF proteins after ABI could be linked to an increased risk of severe intracranial hypertension (ICH) and death. The study also identified specific vCSF proteins that were associated with increased inflammation, apoptosis, oxidative stress, and cellular response to hypoxia and injury.
1.7 Neuroproteomics in central nervous system injury
Different biomarkers correlate to different pathophysiological conditions. During neurological injuries, genetic modifications and ruptures of the blood–brain barrier (BBB) create post-translational covalent/ non-covalent protein modification in a variety of protein structures, such as glial fibrillary acidic protein (GFAP), myelin-oligodendrocyte glycoprotein (MOG), myelin-associated glycoprotein (MAG), ubiquitin carboxyl-terminal hydrolase isozyme L1 (UCH-L1), microtubule-associated proteins (MAP-2), neuron-specific enolase (NSE), α-II spectrin, and tau proteins. Different proteolytic fragments create endogenous high molecular weight (HMW) and low molecular weight (LMW) protein fragment mixtures within a variety of different biofluids (178). Endogenous proteolytic fragments can be detected in the nervous system cerebrospinal fluid (CSF), blood serum, saliva, urine, tears, and various other biofluids, all acting as potential samples for proteomics and diagnostic analysis (179).
1.8 Traumatic brain injury
Traumatic brain injury (TBI) is a major cause of health complications and death in young people and has a significant socioeconomic impact. When a severe TBI happens, there is a significant association with 30% mortality and disability among survivors (180). The severity of the TBI is categorized as mild, moderate, or severe, depending on many factors, such as hypoxic and ischemic damage, raised intracranial pressure, cerebral edema, infection, and hydrocephalus (181, 182). TBI’s signs and symptoms may include loss of consciousness, amnesia, nausea, dizziness, headaches, cognitive decline, structural brain damage, and other neurological symptoms (181). A brain injury, such as TBI, starts with applying mechanical force to the head, which can occur with or without loss of consciousness. This mechanical force then triggers a series of cerebral events that depend on the nature and location of the injury (183). The TBI mechanism starts with the impact on the brain tissue caused by mechanical force, which leads to the loss of cerebral vascular autoregulation that leads to abnormality in cerebral blood flow and metabolism. It also affects the mitochondrial function, which causes the accumulation of lactate and disturbs the balance of Ca2+, which affects the cell’s ability to maintain ATP (184, 185).
Neuroproteomics has also been efficient in investigating processes involved in CNS injury. TBI injury events occur in two phases mediated by different sets of proteins activating several pathways that shift the balance from pro-survival to pro-apoptotic/necrotic inflammatory states (186–188). These events are mediated by activated cysteine proteases that act on brain-specific proteins leading to an overall neural injury (189, 190). The neural cell death events involve primary and secondary injury phases involving different neural brain cells’ components accompanied by dysregulation of different neural proteins. The use of neuroproteomics applications on brain injury helps to understand altered protein dynamics, especially in biomarker research. TBI is a complex disorder that is hard to assess by current clinical techniques, including the computer tomography (CT) scan and magnetic resonance imaging (MRI), which are expensive instrumentations and are not universally available (191). Therefore, searching for brain injury biomarkers is crucial for diagnostic and prognostic purposes.
Many efforts were performed to clarify the complexity and progression of TBI. Sensitive and specific biomarkers are extensively used for the prognosis of neurotrauma. This can be achieved using immunoassays, including enzyme-linked immunoassay (ELISA) and western blotting (WB) (192).
Neuroproteomics, combined with bioinformatics, has shown to be a powerful tool in identifying pathways associated with TBI pathogenesis. Besides, it allowed scientists to figure out the biomarkers and drug target genes (2, 4, 5, 20, 193, 194). The identification and the analysis of blood biomarkers after TBI are maintained by sequential steps, starting with the release of proteins into the extracellular fluid or the blood from the damaged cells, where the detection of high-concentration biomarker proteins is measurable (21). Once the biomarkers reach the bloodstream, the exact concentration is determined by knowing the clearance rate or estimating the half-life of any protein.
In order to identify peptides within a cell, a separation technique must be applied, such as 2D-PAGE (194–198). An alternative method is the use of an ion-exchange chromatography (199). Mass spectrometry and chromatography showed more robust methods than conventional protein separation methods (200, 201). It was shown that mass spectrometry methods were more effective at tracing TBI-associated proteins to the disease progression, which would also make it easier to manage and target therapy for the disease (4). This powerful method separates the peptides according to the m/z ratio. These peptides are then identified by aligning them into a database. The antibody-based methods are an alternative to the mass spectrometry methods, divided into antibody microarray and immune-blotting.
A study reported identifying the global proteome in the hippocampal tissue by using SDS-PAGE-Capillary LC–MS/MS. (21) The global proteome strategy to identify and sequence neural biomarkers employed the cation-anion exchange chromatography, followed by 1D gel electrophoresis before the LC–MS/MS of the tryptic digested peptides (bottom-up) (11, 202). Proteome identification using the bottom-up method showed 59 proteins, which were differentially expressed. The study reported that 21 proteins were downregulated and 38 were upregulated. The global proteome strategy to identify and sequence neural biomarkers employed the cation-anion exchange chromatography, followed by 1D gel electrophoresis before the LC–MS/MS of the tryptic digested peptides (bottom-up) (20). For example, a proteome study identified 59 expressed proteins using the bottom-up method, which were differentially expressed and suggested that 21 proteins were decreased and 38 were increased (20). The MS/MS method showed high importance in identifying the change in protein expression and TBI progression (21).
Furthermore, quantitative proteomics was applied in a study to quantify the protein levels associated with TBI-induced cells, followed by targeted temperature management (TTM, mild hypothermia, 32°C). The study showed that by the label-based quantitation by iTRAQ, the proteins significantly associated with TTM were plasminogen, antithrombin III, and fibrinogen gamma chain transthyretin (203).
A study performed by Xu et al. was able to identify 4,031 proteins in TBI patients that are important in glial cell differentiation (e.g., myelin proteolipid protein and myelin basic protein), complement activation (e.g., complement decay-accelerating factor and complement C4-B), and apolipoprotein catalysis (APO) in the statin pathway (204).
Proteomics can also be used to identify therapeutic agents in TBI. One of the therapeutic agents is a Chinese medication called the XFZYD. LC–MS/MS, WB, and TMT-quantitation have been used to explore the mechanism of how the XFZYD is used to treat TBI and which proteins are targeted by XFZYD medication. The same study demonstrated by using bioinformatics before proteomics that “XFZYD” target proteins mainly involved in biological processes, cellular components, and molecular function (205).
In one study using 2D-PAGE coupled with matrix-assisted laser desorption ionization time-of-flight (MALDI-TOF) MS analysis, Siman et al. performed neuroproteomics analysis of CSF from the rat model with mild/moderate TBI (206). The results showed different proteins leaked into the CSF, including tau protein fragment of 17 kDa and αII-spectrin breakdown products (BDP150 and SBDP120). Another study by Burgess et al. located 229 proteins, 172 of which were novel hits, in healthy human postmortem immunoaffinity-depleted CSF (207). Results were validated by immunoblotting and sandwich enzyme-linked immunosorbent assay (ELISA) methods. Similarly, Kobeissy et al. designed an offline multidimensional separation platform termed cation-anion exchange chromatography followed by a 1D-PAGE separation (CAX-PAGE) (20). CAX-PAGE allowed for sample analysis without mixing to increase the mass range. This technique was tested on rat cortical samples and yielded results of 59 protein alterations and other novel protein breakdown products.
To investigate molecular pathological pathways underlying the progression of brain injury mechanisms, Yu et al. used a bioinformatics systems biology strategy based on assessing four distinct high-throughput gene expression studies of experimental TBI (208). Canonical pathways and the protein-interaction network were assessed as a scaffold to predict protein markers and identify novel molecular mechanisms involved in TBI. Results indicated that a subnetwork of 58 proteins related to synaptic capacity was identified, including postsynaptic density protein 95 (PSD 95), nitric oxide synthase 1 (NOS 1), and disrupted in schizophrenia 1 (DISC 1). These were validated using a penetrating ballistic-like brain injury rat model reaffirming the predictive bioinformatics model of protein interaction (208). In one study by Xu et al., where proteomics and bioinformatics techniques were combined, variations in protein expression levels were assessed in a Chinese TBI cohort (204). Tandem mass tags (TMT) labeling followed by LC–MS/MS was used to identify 4,031 proteins, including 160 that were overexpressed and 5 that were down-expressed. Upregulated proteins included myelin basic protein (MBP) and myelin proteolipid protein (MYPR), which play a role in glial cell differentiation pathways. Along with matrix metallopeptidase 9 (MMP9) and s100 calcium-binding protein A8 (S100A8) associated with inflammatory mechanisms.
Moreover, Thelin et al. evaluated the protein profile using an antibody bead suspension array in a rat model of severe TBI (209). During the initial day post-injury, complement factor 9 (C9) and complement factor B (CFB), which are involved in the innate complement system, were identified. Also, aldolase c (ALDOC) was found to be increased early on after the injury, while hypoxia-inducing factor (HIF)1α, amyloid precursor protein (APP), and Williams-Beuren syndrome chromosome region 17 (WBSCR17) protein were shown to be elevated weeks following the insult (209).
PTMs in TBI have also been assessed through proteomics, like in the case of one research by Lazarus et al., which focused on determining the brain regions susceptible to carbonylation (210). A study used the brains of female and male rats subject to injury through controlled cortical impact (CCI) and was immune-stained for protein-related structural changes. Directly in the injury site’s area, astrocytes and ependymal cells lining the third dorsal ventricle and the third ventricle floor above the median eminence displayed the highest protein carbonylation levels. The study presented male rats’ significant protein carbonylation at sites distant from the lesion, showing that hormonal protection is probable in oxidative stress. Ultimately, GFAP, dihydropyrimidinase-related protein 2, fructose-bisphosphate aldolase C (ALDOC), and fructose bisphosphate aldolase A (ALDOA) were identified in the study to be the most affected proteins by carbonylation in TBI. However, oligodendrocytes, microglia, and macrophages lacked this PTM (210). More recently, Mondello et al. have performed a comprehensive, in-depth profile and characterization of the N-glycome in serial blood samples of patients with moderate to severe TBI. This discovery study demonstrated a TBI-specific glycofingerprint reflecting molecular events and pathobiological mechanisms underpinning brain injury and recovery and contributing to patient endophenotyping. Moreover, N-glycans with important prognostic values that may represent novel targets for intervention were identified (57). This work paves the way for mapping the brain glycoproteome with the goal of enhancing our understanding of pathobiological mechanisms underpinning TBI and contributing to patient endophenotyping with significant implications for precision medicine.
1.9 Mass spectrometry imaging techniques
Neurotrauma, encompassing TBI and SCI, presents significant challenges in both diagnosis and treatment. Understanding the molecular changes occurring in the injured nervous system is crucial for developing effective therapeutic strategies. Mass spectrometry imaging (MSI) techniques, particularly MALDI, Desorption Electrospray Ionization (DESI), and SIMS have emerged as indispensable tools in neuroproteomics research, offering detailed insights into the molecular alterations following neurotrauma (211, 212). Moreover, the application of these techniques in precision medicine has paved the way for personalized interventions, optimizing treatment outcomes for individual patients (212, 213).
MALDI-MSI enables the comprehensive mapping of proteins, lipids, and metabolites in neurotrauma-affected tissues (214). In the context of TBI, MALDI-MSI has been instrumental in studying the spatiotemporal distribution of proteins associated with inflammation, neuronal damage, and repair processes (214). By analyzing specific protein expression patterns in different regions of the injured brain, researchers can identify potential therapeutic targets (215). Furthermore, MALDI-MSI facilitates the discovery of biomarkers indicative of injury severity and prognosis, aiding clinicians in making informed decisions about patient care and treatment strategies (212).
DESI-MSI offers distinct advantages in studying lipids, which play pivotal roles in neuronal membrane integrity, signaling, and inflammation (216). Following neurotrauma, lipidomic changes occur, influencing the progression of secondary injury processes (217). DESI-MSI allows for the direct analysis of lipid species in traumatized neural tissues, providing valuable information about lipid composition alterations (218). Understanding these changes is critical for developing interventions that promote neuronal survival and repair, making DESI-MSI a valuable tool in neurotrauma research.
SIMS imaging has also emerged as a powerful analytical technique in the field of neurotrauma research. By bombarding a sample surface with a focused primary ion beam, SIMS generates secondary ions representative of the sample’s elemental and molecular composition (219). In the context of neurotrauma, SIMS imaging offers unprecedented insights into the biochemical alterations occurring in injured neural tissues (216). Researchers utilize SIMS to map the distribution of specific biomolecules, such as neurotransmitters, lipids, and proteins, at subcellular resolutions (216). This detailed molecular profiling aids in understanding the complex mechanisms underlying neurotrauma, shedding light on cellular responses, metabolic changes, and signaling pathways associated with brain injuries.
In the realm of precision medicine, MSI techniques have far-reaching implications (220). By characterizing the molecular signatures of individual patients’ neurotrauma lesions, clinicians can tailor treatment approaches based on unique biochemical profiles. This personalized strategy enables targeted drug delivery, optimized rehabilitation protocols, and individualized neuroprotective interventions (221). Moreover, MSI can aid in monitoring treatment responses over time, allowing for adaptive modifications to therapeutic regimens and ensuring the best possible outcomes for patients suffering from neurotrauma (212). Therefore, these techniques have revolutionized neuroproteomics research in the context of neurotrauma. Their ability to unravel the complex molecular landscape of injured neural tissues not only enhances our understanding of injury mechanisms but also facilitates the development of personalized treatment strategies in precision medicine. By integrating these innovative techniques into clinical practice, healthcare providers can offer tailored interventions, ultimately improving the quality of life for patients affected by neurotrauma.
2 Technological advances in the field of neuroproteomics
In addition to the standard methodologies of biochemical and proteomics analyzes, technology and bioinformatics are rapidly being tied to medical research. There is a need for higher data analysis levels and evaluation, as the limitation of simple human-based trials and research is not sufficient for a comprehensive knowledge of the dynamic human brain. ML programs and AI present the opportunity to fill the gaps presented by traditional statistical analyzes, allowing for increased sophistication when dealing with complicated data sets. The field of computational analysis has only recently begun to gain traction within the biomarker research scope but shows clear promise in terms of application and utility.
ML is a term used to describe many computational models and AI programs that take user-defined information to generate predictions and other similar data (222). These models rely on a series of base algorithms that can quickly extrapolate results from datasets rich in complexity and volume. This makes them highly effective when dealing with topics that require a multivariate approach, such as clinical outcomes or novel data simulations. Additionally, many different ML analysis types allow a significant degree of freedom when addressing research direction. Many studies use several different ML algorithms, the main selections being: Random Forest, Decision Trees, Naïve Bayesian models, Logistic Regression (LR), Support Vector Machines (SVM), and Artificial Neural Networks (ANN). ANNs, LR, and SVMs are the programs that have been mainly used so far to explore outcomes within clinical research, each providing unique approaches to interpreting interrelated datasets (223). Most results show that the most effective analyzes are SVM and ANN, so these will be the main focus when discussing predictive ML models. LR will be discussed as it is the most common model that studies use as a comparison model because of its simplicity as a traditional ML program. LR is a widely used supervised learning tool that makes predictions based on input data’s logistic functions. However, ML techniques have recently outperformed traditional regression models when dealing with multiple datasets.
Chong et al. (224) ran a predictive analysis of 39 pediatric severe TBI cases. They aimed to develop a predictive model using a series of binary predictor variables, such as loss of consciousness and skull fracture. However, they ran two separate analyzes: one using traditional LR and another using a novel ML algorithm (225) designed initially to predict acute cardiac complications. They developed both models using parameters defined in their study and ran a ROC curve analysis comparing them. The effectiveness of an ML model can be measured by the relationship between its sensitivity (true positive occurrence) and specificity (true negative occurrence), as well as the AUC statistic generated by a ROC analysis of the generated results.
It was found that the ML model outperformed the LR model, most noticeable in terms of sensitivity (94.9% vs. 82.1%) and positive predictive value (90.2% vs. 72.7%). However, the ML model considered three extra variables that the LR model did not: clinical indication of seizures, confusion, and skull fracturing. When using an ML model, a risk to keep in mind is that the original predictions that ML algorithms learn from are derived from user-defined positive predictions. If the original predictions are flawed, the ML predictions may seem accurate but be just as flawed. These results are a testament to most ML models’ ability to handle large amounts of predictive data, more than most traditional statistical analyzes. Raj et al. (226) developed a pair of simple ML algorithms by expanding upon LR’s statistical concept. Instead of binary variables, clinical ICU measurements were used, namely intracranial pressure, mean arterial pressure, cerebral perfusion pressure, and Glasgow Coma Scale (GCS). One algorithm used the first three predictors, and the other included the GCS in its predictions. Designed as a prognostic tool for TBI patient mortality, these algorithms exhibited accuracies of 81% when identifying survivors and 84% when identifying deaths. Although this algorithm is simple compared to other ML models, it suggests that a move toward more dynamic and advanced analyzes could provide more effective tools to clinicians and researchers. Feng et al. (227) performed a more comprehensive comparative study in which 22 different ML models were compared to a LR model. The ML models included decision trees, discriminant analysis, SVM, and k-nearest neighbor algorithms. The goal was to predict the outcome of severe TBI patients using a combination of 40 different predictors defined as risk factors. When they performed their evaluations, the lowest accuracy rating for the ML models was 86.3% (quadratic discriminant), while the most accurate programs boasted an accuracy of 94% (linear, cubic, and quadratic SVM). LR showed an accuracy of 88%, falling short of 20 out of the 22 ML algorithms. ROC analyzes were performed, and LR showed an AUC of 0.83, while the average AUC of the ML programs was 0.82. However, when outlying algorithms that showed poor performance were removed (AUC 0.3, 0.47, 0.57), the average ML AUC was 0.88. The AUC values for the ML programs with the highest accuracies were 0.93, 0.94, and 0.93, respectively. This analysis supports ML algorithms’ use over LR models as prognostic tools in TBI. It also shows the enhanced performance of SVMs over the other ML models explored in the study. However, Gravesteijn et al. (228) found that there are no significant differences between flexible MLs and LR performance when dealing with a low number of predictors. They found that random forest models generate worse performances when compared to LR models under these conditions. They used IMPACT-II and CENTER TBI databases to perform their calculations, both of which have variables with thousands of data entries to use. Despite the large datasets, the low number of predictor values did not allow for increased performance, regardless of ML complexity. It is noted that in high-dimensional analyzes (using a high number of predictors), complex ML programs have been known to outperform LR. LR has still proven to be valuable for recent protein biomarker studies.
Thelin et al. (229) used a series of univariate LRs to compare the predictive usefulness of six protein biomarkers: S100B, NSE, GFAP, UCH-L1, tau, and neurofilament-light (NF-L). The programs’ predictions were dichotomized based on the Glasgow Outcome Scale (GOS) scoring (1–3 vs. 4–5, 1 vs. 2–5). UCH-L1 showed marginally better performance than the other biomarkers, with higher AUC values in all categories. The analyzes’ results were also used to assess trajectory curves and association strengths between each biomarker toward the outcome GOS scoring prediction. The biomarkers that were determined to have the highest predictive strength also showed the highest levels among patients with unfavorable outcomes, supporting the LR results. These biomarkers were also compared against traditional TBI predictors (GCS, CTC Scan, Glucose levels, etc.). Specifically, within the first 5 days, almost all biomarkers performed better as outcome predictors than the traditional criteria. Despite its simplicity and limitations, LR is still a handy prognostic tool. ML programs can create powerful new avenues for biomarker applications when coupled with temporal analyzes and the proper assessments.
Regarding dataset complexity, an element that is somewhat lacking among many TBI ML studies is continuous variables (Table 1). Much of the clinical data collected falls under binary or discrete variables, and the present continuous variables do not seem particularly specific to TBI prognostics. This disparity in data is partly because most traditional models cannot efficiently absorb large amounts of continuous data and compare it in a nonlinear or multivariate fashion. ML programs like SVM can become useful to remove this confusion in data organization. SVMs design a hyperplane based on the number of features or predictors used, and they then attempt to find a plane at the maximum distance between those points to develop a margin of error for future data points.
SVMs are ML techniques that have begun to gain momentum recently. These programs excel at reading nonlinear relationships between input values if they are calibrated correctly. Kayhanian et al. (232) used SVM to design a 6-month prognostic tool for severe TBI in pediatric patients. They gathered patient blood test data and used 14 serum parameters as predictor values, all of which were non-discrete. The GOS was dichotomized (4–5 being good, 1–3 being poor) and used as the outcome variable. The maximal information coefficient and the absolute correlation coefficient for all parameters were found and plotted to narrow the algorithms’ focus. Three variables contributed noticeably more to the outcome predictions than the rest: glucose, lactate, and H+. Two SVM models were developed, one using all 14 parameters and the other using only the three highest contributors. The all-encompassing model exhibited a sensitivity of 63% and a specificity of 100%, while the triple-parameter model had values of 80 and 99%, respectively, making it the most accurate model. This study’s emphasis on measurable, continuous predictors in serum composition levels hints at the prospect of using SVM to predict outcomes and one for clinicians to use in mediation. Targeted interventions using these levels may help improve TBI outcomes rather than simply estimating them. Matsuo et al. (233) performed a similar analysis using a combination of discrete and continuous predictor variables. Fourteen different classifications were chosen, 3 of which fell under the category of regularly collected laboratory samples as in the Kayhanian et al. (232) study. These predictors were input into nine different ML algorithms, including SVM. The objective was to determine which ML program provided the best prediction for poor outcomes and in-hospital death and which predictors had the highest contributions to the models. This study’s results were varied compared to many of the other studies involving ML and TBI predictions. For the morbidity (poor outcome) model, random forest models showed the highest sensitivity (97.2%), and the highest specificity was achieved by the Gaussian Naïve Bayesian model (82.8%). The highest accuracy value was achieved by the Gradient Boosting Model (0.87). According to ROC analysis, SVM exhibited the third-highest sensitivity (0.97), the fourth-highest specificity (0.59), the second-highest accuracy (0.86), and the highest AUC (0.89). In the mortality models, SVM showed the second-highest sensitivity (0.78), the third-highest specificity (0.97), the highest accuracy (0.89), and the fourth-highest AUC (0.94).
No model proved superior when discerning the best ML algorithm, but the best predictors based on the models were age, GCS, fibrin/fibrinogen degradation product (FDP) levels, and glucose levels. Age and GCS are typically input as discrete variables, whereas the lab levels are continuous. This study shows that despite the diverse nature of the variables (discrete and continuous), the ML algorithms still showed good performances. The potential of SVM also reaches toward nontraditional means of prediction. An imaging biomarker study was conducted by Vergara et al. (231) compare resting-state functional network connectivity (rsFNC) to diffusion magnetic resonance imaging (dMRI). Brain scans of patients with mild TBI (mTBI) were used to test whether an SVM algorithm could be used to sort through image data and detect evidence of mTBI. Individual scans were defined to be separated into functional classifications (sensorimotor, visual, etc.) within the algorithm matrices. An SVM was then designed to separate the image data into two classifications: mTBI or healthy controls. The model that used rsFNC showed the most robust results, with an accuracy of 84.1%, a sensitivity of 89.4%, and a specificity of 78.8%. The models that used dMRI exhibited markedly lower performance. These results represent the potential for SVM beyond its functionality as a prediction tool. They show the ability to diagnose mTBI in a highly accurate manner via brain scanning and ML combinations, highlighting the importance of creative applications when using these programs.
While SVMs have proved to be powerful tools in many studies, other ML programs have proven superior under certain conditions. Rau et al. (230) performed a comparative study in 2018 to develop ML models that could predict mortality in isolated moderate to severe TBI (sTBI) patients. The study tested several different algorithms, including LR and SVM. However, this study also included ANN in its analysis. ANNs mimic the function of human neural networks to analyze large datasets. They are unidirectional and include inputs, at least one hidden layer, and output nodes.
Their complexity characterizes the major differences between ANNs and more in-depth deep learning; deep learning approaches have more hidden layers that are not immediately visible to researchers (labeled versus unlabeled datasets and algorithms). All the models tested in the study showed an accuracy above 90%, though the ANN showed a high value of 92%. The focus was shifted onto sensitivity, specificity, and AUC values because of the high accuracy ratings. The ANN’s sensitivity (84.38%) was about 20% higher than the second most sensitive algorithm. It also exhibited a high specificity at 92.8%, and its AUC was significantly higher than all other ML models at 0.97. For comparison, corresponding SVM values were 92.5, 65.6, 95.2%, and 0.93, respectively. Because of its high performance in both the training and the test sets across all measures, the ANN has been deemed the most effective prediction model. An ANN is more effective than typical injury scoring models such as TRISS when predicting survival for trauma patients (234) or GCS and GOS when giving 6-month TBI outcome predictions in children (235). The consistent strength of ANN when making outcome predictions in injury patients suggests its usefulness as a prognostic tool for clinicians, potentially more effective for clinicians and researchers to use over traditional injury scoring methods currently used. However, there are caveats. Raju et al. (239) stress the need for neurosurgeons to have competencies in neurosurgery expertise, statistical knowledge, and computation skills to utilize the potential of ML. Bertolaccini et al. (240) found that using ANNs in the medical literature has often been performed inaccurately, resulting in misleading results. ANNs do not require prior knowledge or statistical distribution assumptions to accurately establish input–output relationships (238), which acts as a great advantage with large datasets, especially nonlinear distributions. Compared to LR models, ANNs can have difficulty overfitting the model during the learning time and can be limited by computing power and available time when analyzing large datasets. Biomarker injury studies that use ANNs, especially within TBIs, are currently sparse.
However, Rashidi et al. (236) explored the possibility of using ML techniques as an early recognition system for acute kidney injury within burning and trauma patients. They combined it with exploring a novel polypeptide biomarker NGAL, along with other traditional blood AKI biomarkers (NT-proBNP, creatinine, and UOP). When testing the NGAL biomarker by itself, their ML methods proved to be extremely capable. Four of the five algorithms achieved an accuracy of at least 92%, with sensitivity values of at least 73% and specificities of 97%. The AUC for these models was at least 85%. They found that DNN (Deep Neural Network, an advanced type of artificial neural network) and LR models performed the best. Once a combination analysis of NGAL with other biomarkers was performed, performance statistics (particularly AUC) increased dramatically. DNN showed an incredible performance through its AUC values, with 7 out of 11 of the combined analyzes being at least 90%. The second cohort of patients was used to test for overfitting and other modeling errors. DNN performed noticeably worse with this cohort, with AUC values never reaching above 88% and having an all-time low of 49%. However, it was noted that this second cohort also contained non-burn trauma patients, which the DNN was originally not trained against. The introduction of novel characteristics would naturally hinder the trained DNN’s ability to make accurate predictions. The study speaks volumes about using ML programs, particularly neural networks when using blood and protein biomarkers. The generalizability of these programs to other sources of injury, such as TBI, marks the field’s potential (Table 2). Using several biomarkers at once in a highly efficient and automated analysis to output an equally accurate series of predictions paves the way for new avenues of prognosis.
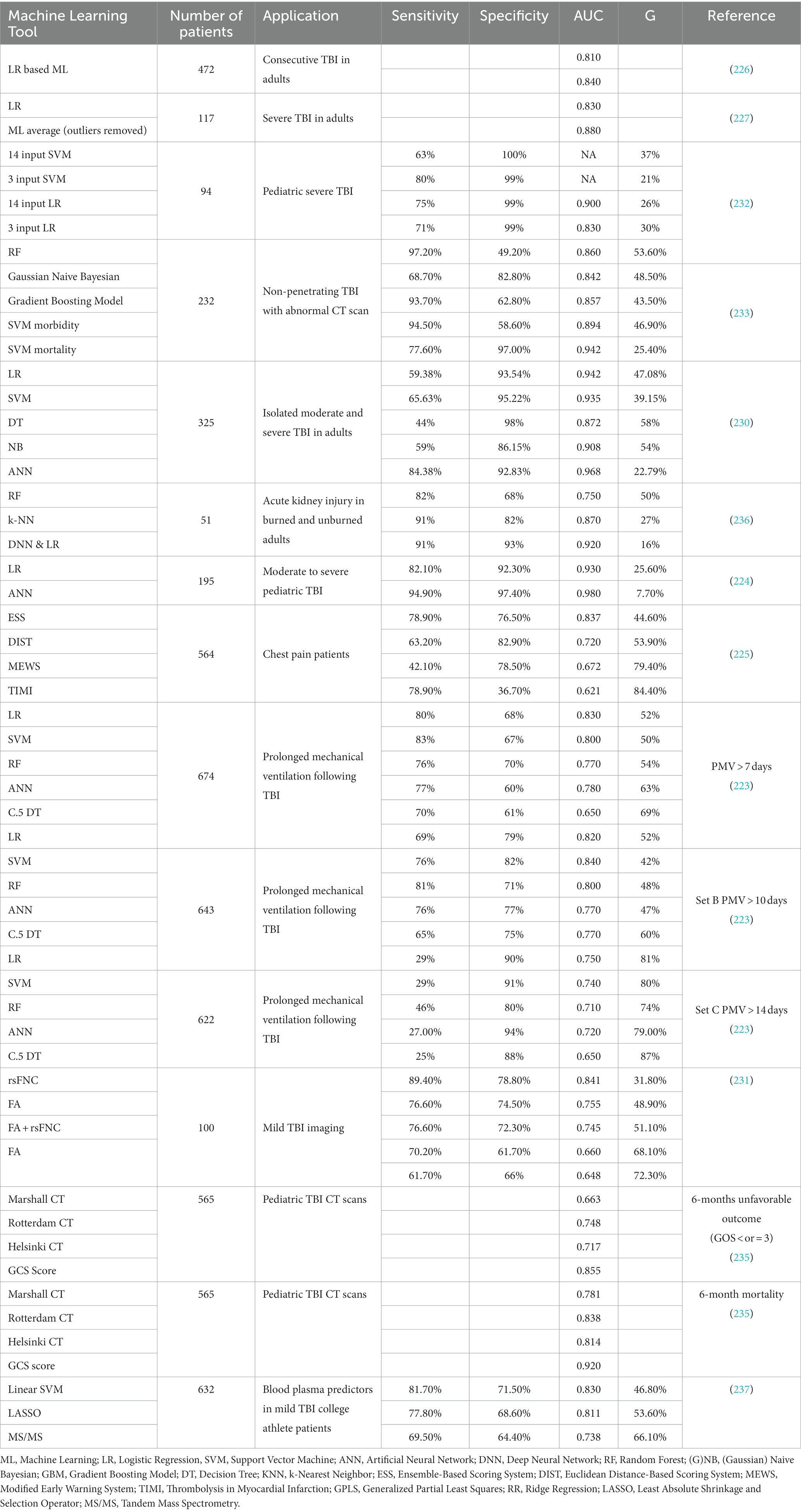
Table 2. Summary of applied machine learning programs and corresponding receiver operating characteristic analyzes.
A key feature of ML programs is their flexibility of use. ML programs have proven to be very effective when making predictions using clinical datasets, but they also work well when combined with other advanced techniques, such as mass spectrometry. Mass spectrometry is a vital proteomics technique involving the fractioning of protein complexes via electrophoresis or chromatography. It allows for accumulating massive amounts of proteome data, making it particularly relevant to proteomics and genomics biomarker research (241). When coupled with powerful tools like ML, a wide variety of new explorative uses are made available. Fiandaca et al. (237) performed a study in which student-athletes were monitored for concussive events. Once an event occurred and a concussion was confirmed, blood plasma samples were taken less than 6 h after the event, 2 days after injury, 3 days, and 7 days. The samples’ metabolites were then isolated and identified using metabolic mass spectrometry. The metabolite readings were then analyzed using SVM, partial least-squares discriminant analysis (PLS-DA), and random forest analysis. The study aimed to develop ML programs that could isolate specific metabolites used as biomarkers for mTBI. The MS program originally came up with 2,811 possible metabolite biomarkers, which were reduced to 294 using MS reduction programs. This number was further reduced to the ten best-fitting metabolites using six different ML programs, including SVM and LR. These metabolites were analyzed among different athlete cohorts by the ML algorithms, and six main biomarkers were isolated as the best predictors of mTBI between all the study cohorts. These results represent a massive step toward rapid TBI diagnosis using a less invasive method (phlebotomy). This method also overrides the current diagnosis methods, such as GCS or GOS scoring, in terms of mechanistic application and quantifiable analysis. MS also has exhibited a remarkable ability for brain reconstruction. Nampei et al. (242) trained a series of ML programs to take in principle component analysis (PCA) data generated through MS and automatically reconstruct images of rat brains’ white and gray matter tracts. Mallah et al. (243) reconstructed and analyzed injured rat brains via MS to measure lipid differences within different post-TBI brain regions. A combination of these two studies’ techniques suggests the eve of a surge in biomarker research using MS and possibly ML to identify and spatially isolate potential biomarkers effectively. ML would also prove an effective tool in determining the biomarkers’ predictive and diagnostic value once data has been collected. It is essential to note in one area that current predictive clinical ML is found to be lacking in prediction “exactness. Many of the algorithms sort data into two categories: good or poor outcomes. It would be advantageous to design a classification system (possibly using GCS or GOS criteria) that ML could sort data into so that a particular value or prediction can be used to indicate certain symptomologies’ more detailed prognoses.
In addition, in the realm of neuroproteomics, AI has emerged as a groundbreaking tool, revolutionizing the way researchers analyze and interpret complex biological data (244). By harnessing the power of ML algorithms, AI enables scientists to process vast datasets with unparalleled efficiency and accuracy, leading to profound advancements in our understanding of the brain’s proteomics landscape (245, 246). One of the key applications of AI in neuroproteomics involves the identification and characterization of proteins associated with neurological disorders such as Alzheimer’s disease, Parkinson’s disease, and epilepsy (247, 248). Traditional methods for analyzing mass spectrometry data are often time-consuming and prone to errors. AI algorithms, however, excel at recognizing intricate patterns within these datasets, swiftly identifying potential biomarkers indicative of specific neurological conditions (10, 249). This capability not only accelerates the pace of biomarker discovery but also holds immense promise for early disease diagnosis and the development of targeted therapies, ultimately enhancing patient outcomes in the field of neurology (250).
Furthermore, AI-driven approaches in neuroproteomics extend beyond mere data analysis; they facilitate the prediction of protein–protein interactions (251), protein functions (252), and intricate signaling pathways within the brain (253). These predictions are invaluable for elucidating the underlying molecular mechanisms of neurological diseases. AI algorithms can integrate diverse omics data, including genomics, transcriptomics, and proteomics, enabling a comprehensive understanding of the complex interplay between genes, proteins, and pathways in neurological disorders (254, 255). Such holistic insights are pivotal for identifying novel therapeutic targets and designing personalized treatment strategies tailored to individual patient’s unique molecular profiles. As AI technologies continue to advance, their integration with neuroproteomics not only enhances our fundamental understanding of the brain’s intricate biology but also holds the potential to revolutionize clinical practice, ushering in a new era of precision medicine in neurology (256).
3 Neuroproteomics in personalized and precise medicine
With the development of benchwork proteomics studies and advancements in AI and ML, neurologists and biotechnologists are combining neuroproteomics and bioinformatics to develop the personalized medicine (226, 227). Personalized medicine expands precision medicine, creating unique prognostic, diagnostic, and therapeutic medical decisions customized uniquely for each patient (226, 227). With millions of patients every year, and individual responses from each patient, understanding how to respond to specific scenarios is complicated. Combining the use of biomarker quantitative statistical analysis, bioinformatics ML, and AI, the field of neuroproteomics can develop groundbreaking tools for diagnostic and theragnostic outputs for each unique individual (257, 258).
Today, physicians’ ability to diagnose specific diseases is limited, especially with complicated disorders such as TBI (mild vs. severe) (259). The genome variations, pathophysiological responses, and classifications for different people are currently unclear and undiagnosable. Most physicians utilize psycho-diagnostic scoring systems (such as the GCS and Marshall Scales) and compare their findings to those presented by neuroimaging (CT, MRI, and fMRI scans) (260). Limit ranges of knowledge and gray areas on the true difference between mild, moderate, and severe degeneration on a protein/psychological quantitative level are limited, leading to many false positive and false negative diagnoses (260). Utilizing hundreds of different variables and high-order machine organizations incorporates specific patients’ conditions, specialized gene variants, and various conditions on a personalized level. This organization and correlation schematic will help physicians understand how to proceed in certain neurological conditions (239).
Neuroproteomics incorporated personalized medicine can use AI and ML to analyze the interaction of hundreds of potential variables to provide the unique output for diagnosis and therapy. The AI and ML techniques described in the previous section can correlate specific protein concentrations, characteristic post-translational modifications, severity score index (SSI) ranges, injury types, time-dependence, protein-medicine interactions, and identify potential characteristics of diseases (259). Molecular diagnostic, nanoproteomics, pharmacoproteomics, genomics, metabolomics, and system biology data are all being collected in mass efforts for large databases (21). Taken together, the collection of mass neuroproteomics data and the development of higher-order systems will help classify precise neurological conditions for a more accurate form of personalized medicine (2, 20). Connecting the data collected from each field and associating patient characteristics through neuroproteomics technology will create more effective diagnostic claims for each unique patient, thereby advancing the field of personalized medicine (110, 257, 258) (Figure 6).
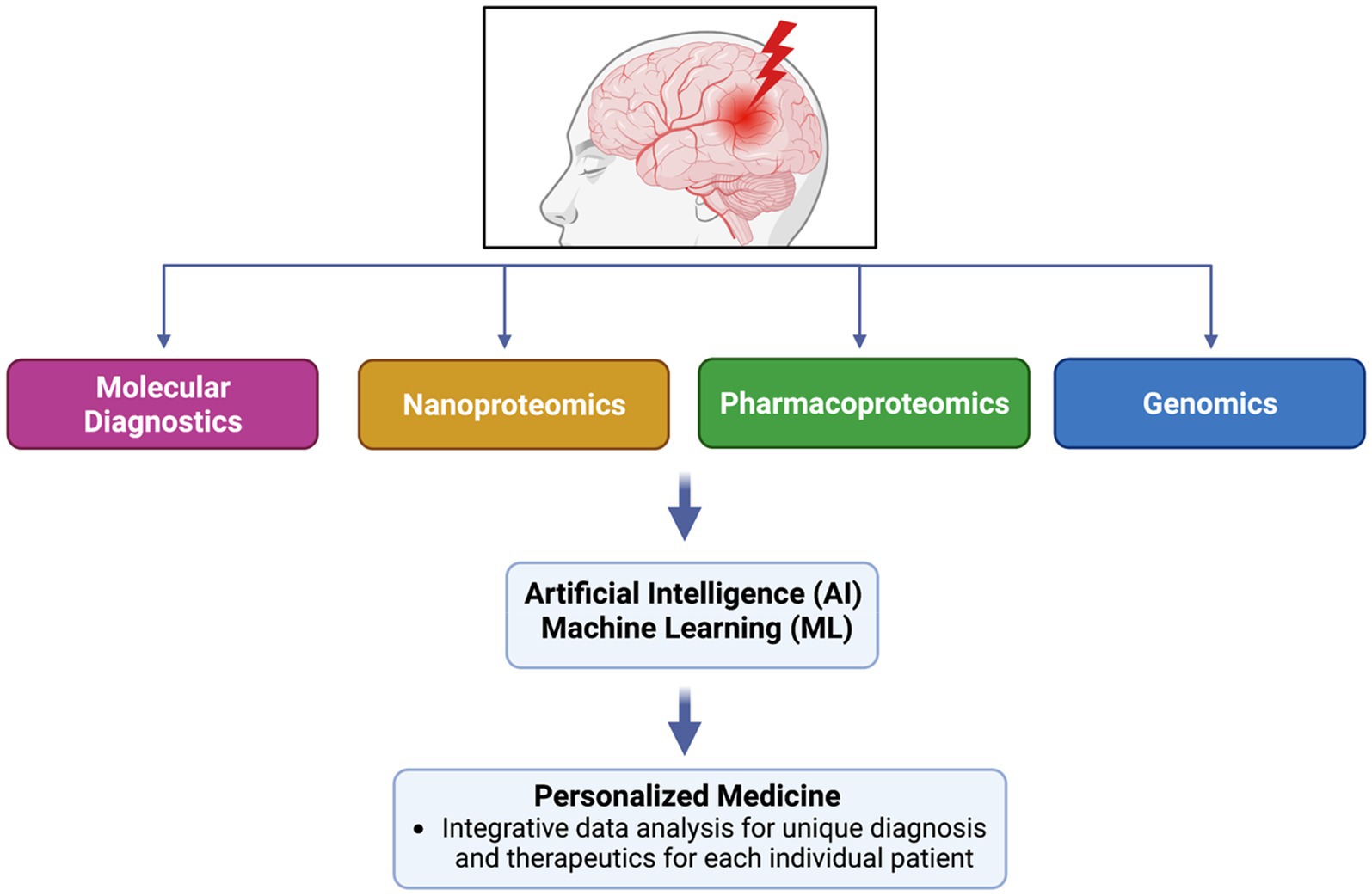
Figure 6. Artificial intelligence incorporated proteomics in personalized medicine. Once molecular diagnostic, nanoproteomics, pharmacoproteomic, and genomic data is collected correlations, AUC comparison, and significant tests are utilized to identify potential relationships between data. Data storage through AI and ML technology analyze large data sets to create faster and more accurate predictions with prognostic, diagnostic, and theragnostic value, all essential for effective personalized medicine.
As precision medicine has been well adapted to genomics, proteomics precision medicine displays further complexity due to the proteome’s previously discussed dynamic nature. The steady control state’s proteome differs from the post-disease state that involved PTMs and protein–protein interactions. These intricate interactions are especially apparent in neuro-related disorders like AD and TBI. For example, AD displays a latent phase where no signs and symptoms are observed. However, pathophysiological processes still occur, leading to a prodromal phase with MCI and dementia (261). Identifying this latent phase marks a fundamental approach to dealing with the disease and requires tracking biomarkers to present critical steps in individualized medicine.
The application of neuroproteomics in clinical studies eliminates treatment response variability and provides a more targeted therapy approach. As the main goal of personalized medicine is to match the patient response with the best treatment to make sure the optimal outcomes are obtained, it is crucial to understand the challenges faced by proteomics compared to genomics, i.e., high cost and instrument robustness, to obtain the optimal outcomes (262). This is along with the fact that many biofluid proteomics studies rely on sample pooling, where result validation is performed by randomly selecting individual samples. As an example, the recent breakthrough in personalized medicine for AD as one of the significant public health issues involves the application of genomics and proteomics profiling to identify the specific biomarkers associated with the disease (263). Scientists have made significant progress in decoding the genetic variations and protein signatures unique to individuals with Alzheimer’s, enabling a more precise understanding of the disease’s heterogeneity (263). This personalized approach allows for early detection of Alzheimer’s risk, often before clinical symptoms appear, enabling timely interventions and lifestyle modifications. Moreover, targeted therapies tailored to the individual’s genetic and proteomics profile are being developed, aiming to slow down disease progression and improve cognitive functions. By customizing treatment strategies based on the patient’s molecular makeup, these advancements represent a paradigm shift in Alzheimer’s research and care, offering hope for more effective, personalized, and patient-specific interventions in the battle against this devastating neurodegenerative disorder (263).
In the realm of TBI research, precision medicine techniques have garnered substantial interest, although their translation into clinical practice remains a challenge (180). To date, only limited clinical studies have explored the potential of precision medicine approaches for TBI, and none have transitioned into the routine clinical application (264). Despite this, the scientific community is actively engaged in numerous preclinical studies, focusing on identifying and targeting the common genetic risk factors associated with TBI (264, 265). This formidable field offers several advantages translating into clinical research, a crucial step toward scientific applications (Table 3). Unlike the retrospective study of individual proteins/genes in post-mortem patient specimens, neuroproteomics allows for discovering novel proteins in a compassionate and unbiased manner. Remarkably, its ability to investigate these novel proteins does not require the formulation of specific hypotheses before experimentation. It also allows for building and analyzing extensive databases that allow data exploration from several centers. Hopefully, these ongoing investigations hold promise for the future, indicating a shift toward personalized therapeutic strategies tailored to individual genetic profiles, ultimately enhancing the prognosis and treatment outcomes for TBI patients.
4 Neuroproteomics human clinical trials
Finally, in addition to neuroproteomics analysis abilities in labs and animal models, neuroproteomics has recently become a significant field in clinical practice. Many studies have used neuroproteomics for clinical diagnostic tools and theragnostic approaches. The use of human clinical research is key to seeing how biomarker detection within biofluids can be used for testing effective diagnosis, prospective therapies, and potential human cures. Clinical trials take the techniques and treatments developed in scientific bench work and apply them to animal and real-life human scenarios. Clinical trials require subject to consent, approval, and adequate resources. Once these are acquired, clinical trials are mandatory to develop potential cures and treatments, the next steps in the neuroproteomics research (2, 272, 273).
Neuroproteomics clinical trials have progressed over the last couple of years, with many testing novels and more efficient methods for diagnosing TBI and other neurodegenerative diseases. With hundreds of clinical trials introducing neuroproteomics approaches, the field is continuing to expand rapidly. Today, neuroscientists utilize neuroproteomics and protein correlation analysis to record protein levels and immunological responses to several disorders and symptoms within TBI and its relative proteinopathies (amyloidosis, prion diseases, tauopathies, Alzheimer’s disease, Parkinson’s disease). Multiple clinical trials have already evaluated variables such as demographic factors (pediatric TBI, geriatric TBI, age-related TBI, race, gender), injury types (concussion, mTBI, moderate TBI, sTBI), military TBI, blast wave TBI, injury severity diagnostic matrix scores including the GCS, Marshall CT scales, Pediatric Glasgow Scales, and Standardized Assessment of Concussion (SAC), vitals (temperature, weight, height, biomarkers concentrations, injury phenotypes), and a variety of different factors. AI and ML categorization of multiple variables and effective personalized medicine has led to effective prognosis, analysis, and diagnosis of different neurodegenerative diseases (2, 274–282).
Neuroproteomics and clinical research continue to utilize new biofluids, different biomarkers, and various tests to identify new correlations and algorithms for significant diagnostic measures in the development span. In recent human clinical trials, neuroscientists have taken amounts of blood, cerebrospinal fluid (CSF) samples, and other biofluids from patients in hopes of collecting and analyzing different biomarker levels (21, 283) (Table 4).
It has been demonstrated in clinical trials that biofluids and protein biomarkers provide valuable information for pathophysiological prognostic, and diagnostic (Table 4). Compared to all the different types of biofluids extracted from clinical trial subjects, CSF serum seems to be the most useful in finding correlations between neuropathy and TBI, as it provides the most direct data from the CNS (283). Most trials utilize the cadaver CSF samples; however, recent studies have also utilized patients’ consent to take samples from external ventricular drains (EVD) (283). With EVD, patient CSF is drained from catheters as a routine procedure to reduce intracranial pressure (ICP). EVD and ICP reduction is systematic and causes no harm to the patient while also helping obtain potential samples for the clinical neuroproteomics research (276, 284, 285). Although less invasive, blood samples, urine, saliva, and many other biofluids are also collected to test respective proteins (276, 285). Each biofluid provides valuable concentrations of different biomarker proteins, necessary for proteomics analysis. Different biofluids and biomarkers have been collected and analyzed, leading to groundbreaking algorithms for physicians’ prognosis and diagnosis (272, 286) (Table 4).
Today, various peptidomics approaches have been investigated successfully, as discussed earlier. Scientists continue to find correlations between high levels of specific proteins and their links to potential diseases (286). Levels of specific biomarkers such as tau, p-tau, GFAP, UCH-L1, and many others have been critical for clinical predictions (25, 279, 287, 288). Many studies have already associated different biomarker trends with specific age groups (adult TBI, pediatric TBI, geriatric TBI), injury types (blast injury, military injury, physical injury, gunshot, sports), and times of recovery (1 h, 2 h, 8 h, 12 h, 24 h, 48 h) (274–280, 282). The use of proteomics, as seen in many correlational studies, is vital for the future prognostication and diagnosis of a variety of different types of currently undiagnosable TBI: mTBI, moderate TBI, sTBI, pediatric TBI, geriatric TBI, and a variety of other neurotrauma (274, 277, 280, 289).
With various clinical studies, different quantification techniques, and diverse neurodegenerative outcomes, scientists have outperformed standard diagnosis matrices essential for the precision medicine (277, 282). Targeting treatments, molecular biology, and neuroproteomics approaches have led to novel diagnostic and treatment protocols. Researching various approaches, combining the data through ML/AI, and performing various proteomics studies will all be essential to developing these future advancements in clinical proteomics and precision medicine (273, 277, 286) (Figure 7).
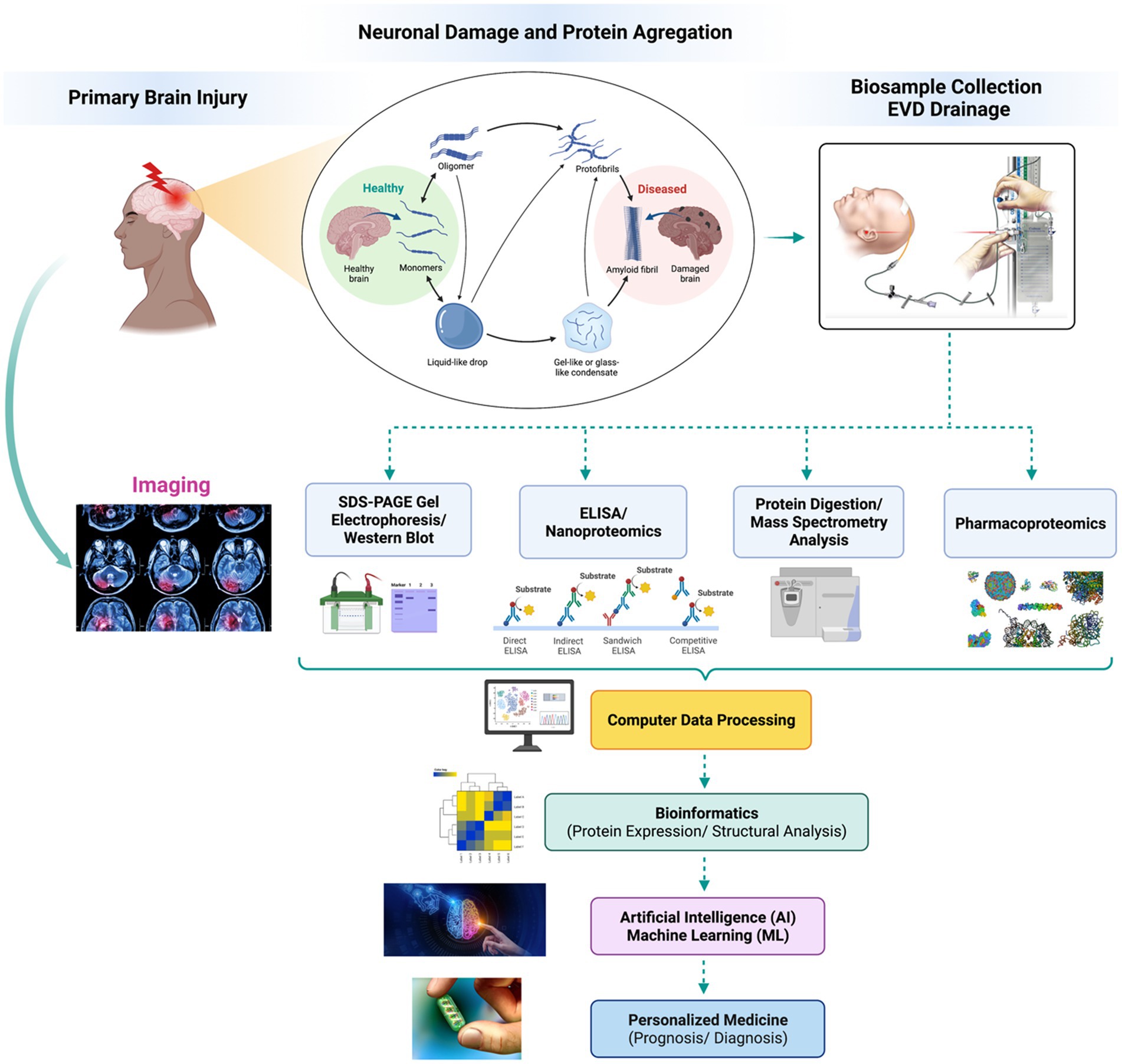
Figure 7. Proteomics, machine learning, and artificial intelligence in personalized medicine. In human clinical trials, neuroscientist study the proteomics of primary injury brain samples. After a primary injury, homeostatic conditions within the brain lead to kinase enzyme activation, PTM addition, and NFT formation, leading to plaque and malformation. Evidence of the damage was once primarily collected through neuroimaging, however faster and more effective technique have been developed through proteomics data analysis, computer data processing, AI and ML. Today, the collection of different data from a variety of tests provides significant data for special cases of TBI and can even provide better prognostic and diagnostic outputs for personalized medicine.
5 Future directions and limitations
In the field of personalized medicine for neurotrauma, the implementation of neuroproteomics has emerged as a revolutionary approach. Neuroproteomics involves the large-scale study of proteins in the nervous system, allowing researchers to understand the intricate molecular mechanisms underlying neurotrauma at an individual level (290, 291). By analyzing the unique protein profiles in patients, clinicians can tailor treatments more precisely, optimizing therapeutic strategies for better outcomes. This personalized approach ensures that interventions are specifically designed to match the molecular intricacies of each patient’s conditions, leading to more effective and targeted treatments. Neuroproteomics, therefore, plays a pivotal role in advancing personalized medicine for neurotrauma by providing valuable insights into the diverse protein signatures associated with brain injuries, guiding clinicians toward a more informed decision-making (287, 292).
Moreover, the integration of AI and ML techniques significantly enhances the potential of neuroproteomics in personalized medicine (293). AI algorithms can process vast datasets, identify intricate patterns within protein profiles, and predict patient responses to different treatments (294). ML algorithms, on the other hand, can learn and adapt from these patterns, enabling real-time analysis and personalized treatment recommendations (295). This synergy between neuroproteomics and AI/ML technologies accelerates the pace of research, leading to more accurate diagnostic tools, targeted therapies, and predictive models (296). Looking ahead, the future of personalized medicine in neurotrauma lies in the continued advancement of these AI and ML applications, enabling rapid and precise decision-making based on a comprehensive molecular data (297). However, it is essential to acknowledge the limitations, including the need for large diverse datasets, ethical considerations, and the interpretability of AI-driven results (298). Addressing these challenges will be crucial for the seamless integration of neuroproteomics and AI/ML in personalized medicine, ensuring its transformative potential is fully realized.
It is also worth mentioning that although developing an effective tool in neuroscientific research, neuroproteomics and coupled technological advancements still have their limitations, human neuroproteomics research constraints lie in sample collection, subject availability, filtration, and identification (283).
First, finding potential human subjects and analyzing the impact of neurodegeneration on living humans is difficult due to the ethical standards of sample collection. Studying samples from live humans is extremely difficult due to the intravenous procedures necessary to conduct studies (284). Obtaining samples for proteomics research through intervention can be a complicated process. Most scientific data today rely on cadaver samples, EVD serum samples, animal models, and the unicellular proteomics (283, 284). While new methods of collecting samples are evaluated with studies like TRACK-TBI and CENTER-TBI, obtaining consent and sufficient samples for evaluation is still complicated due to the ethics behind obtaining informed consent, maintaining privacy, and attaining access to the rights of individual data. It is essential to tackle global data issues and create a global consensus on regulating privacy and consent among different political and healthcare systems (283).
Second, the collection of global human data is vast, and AI and ML would need to be updated to their most robust versions. With a problem holding extensive data, updated computer systems and robust tools will be essential for depositing and accessing necessary data. Innovators of such systems must be wary of automated backup and ensure the uniformity of data. All medical practices should also follow standard operating procedures of data collection, input, and analysis to maintain controlled and accurate diagnosis and the best medical practices worldwide.
In continuation, elaborating on the processes of sample purification, the filtration of proteins is challenging because many samples contain a mixture of different proteins and various impurities. Purification of protein is complex because contamination of nucleotides, peptides, lipids, and biofluids disrupts the isolation of specific protein structures. ELISA, western blot, mass spectrometry, protein sequencing, bioinformatics, and all the proteomic tests require pure protein filtration. Experimenting and identifying methods to purify specific proteins are being studied and continue to be a major advancement within proteomics. Basic protocols for purification methods, enzyme degradation, filtration, and treatment are continually evaluated in many studies (283, 299).
After sample purification, the last evaluation stage also has a few limitations. Bioinformatics, proteomics, and their respective databases are not all fully curated. Evaluation of bioinformatics databases and the use of technological prediction must always be carefully monitored. Support from multiple databases and experimentation is critical for supportive evidence. It is critical to utilize multiple tests, evaluation protocols, and various techniques in bioinformatics to create the most precise predictions and conclusions (283, 299).
Finally, although there are many limitations, the benefits of neuroproteomics-based personalized medicine outweigh the overall effects. Innovation and technological advancement in science will always require funding, and scientists must predict such endeavors’ costs. Conflicts may arise among multidisciplinary, multi-institutional, and multinational groups pursuing grants to support personalized medicine. Regardless of the conflicts and limitations, innovating such technologies and collaborating on a global scale will benefit the future of precision-based and personalized medicine.
6 Conclusion
Despite the drawbacks discussed, the field of proteomics is developing rapidly. In future endeavors, neuroscientists hope to find a fast, efficient, and significant in-vitro, non-intravenous protocol for neuroproteomics clinical diagnosis. Techniques like unicellular neuroproteomics have been coupled with AI and ML to identify the significant correlations and patterns within neuroproteomics data to accomplish these goals. Today, scientists are continuing their studies on what to improve in the subfield of neuroproteomics and how it can be utilized for neurodiagnostic, prognostic, and theragnostic protocols (258, 283).
Today different fields of unicellular proteomics are being utilized to conduct neuroproteomics studies. As described in many previous examples, unicellular proteomics focuses on specific cell types and analyzes the proteins that come from them (283). In many TBI-based studies, scientists evaluate the concentrations of cytokines, macrophages, antibodies, and other immuno-based proteins as potential indicators of neurodegenerative diseases (276, 284, 287, 288). Analyzing innate immune responses to foreign conglomerated proteins and experimenting with specific unicellular protein structures are all potential futures (274). Other factors are analyzed, like the effect of time, secondary injuries, and the concentrations of immunological biomarkers, all essential data for effective clinical prediction models (258, 283, 300).
In continuation, AI and ML systems’ contribution has already been discussed but are also essential, as they will gather large sets of data and analyze them through the system biology (21). AI and ML will tie together proteomics data and carry out significant correlations with the knowledge built on by hundreds of studies. Understanding how to correlate proteomics trends and qualitative diagnostic scores through technological means will revolutionize medicine, especially in the neurology and neurotrauma (222, 227, 239, 258, 283).
In summary, novel processes and protocols continue progressing in neuroproteomics and biotechnology. New techniques and procedures will help spread new therapies, prognostic tools, diagnostic biotechnologies, and future insights for efficient, accurate, and more precise personalized medicine (258, 283, 299).
7 Expert opinion
The value of neuroproteomics as a powerful tool to identify proteins reflecting and involved with specific neuropathobiological processes in TBI has been demonstrated in recent years. Neuroproteomics characterization of biomarkers (i.e., UCH-L1 and GFAP) (185, 301–303) that are now, for the first time, approved by regulatory authorities to aid in the diagnosis of TBI and play a key role in clinical studies are notable examples (304–306). Nonetheless, the molecular complexity of the post-translational modifications (e.g., phosphorylation and glycosylation) and related impact on protein function, pathophysiological processes, and brain interaction networks remain largely unexplored (57, 74). This knowledge holds critical potential for understanding the underlying pathobiology of TBI, paving the way to discover new biomarkers that are also therapeutic targets. To this end, bioinformatic handling of the large volumes of data generated by high-throughput technologies coupled with AI and ML statistical approaches will be essential to meaningful interpret information with respect to the functional and mechanistic value as well as to disentangle data’s heterogeneity and reveal pathways, expression patterns, and phenotypes toward precision medicine.
Tremendous progress has been made in the technologies employed in proteomics studies (high-resolution LC/MS, protein arrays, chip-based technologies, and single-cell proteomics, among others). These tools have become available not only to laboratory researchers but also to clinical settings; however, the ultimate output is to deliver clinically useful point-of-care tests capable of sensitive, accurate, rapid, inexpensive, and reproducible assessment across large patient cohorts.
Importantly, the main problem associated with neuroproteomics in TBI is the inconsistencies and lack of reproducibility of research findings due to the variable quality of the studies and differences in methodology. Therefore, there is an urgent need to standardize methodologies and define rigorous protocols for the bioinformatic and advanced computational analyzes to magnify the impact of the neuroproteomics data. Finally, international multidisciplinary collaborations combining different and complementary expertise and involving physicians, scientists, and programmers may have an instrumental role in analyzing the data proteomics in TBI and developing an effective framework for successful clinical implementation.
Author contributions
FK: Conceptualization, Visualization, Writing – original draft, Writing – review & editing. MG: Conceptualization, Visualization, Writing – original draft, Writing – review & editing. HY: Conceptualization, Visualization, Writing – original draft, Writing – review & editing. ZS: Writing – review & editing. MK: Writing – review & editing. MH: Writing – review & editing. SA-R: Writing – review & editing. SM: Writing – review & editing. KW: Writing – review & editing. YM: Conceptualization, Funding acquisition, Visualization, Writing – review & editing.
Funding
The author(s) declare financial support was received for the research, authorship, and/or publication of this article. This work was supported by the National Institutes of Health 1R01GM130091–07 and 1R01GM130091–04. This work is also supported by the Robert A. Welch Foundation under grant no. D-0005 and The CH Foundation.
Conflict of interest
The authors declare that the research was conducted in the absence of any commercial or financial relationships that could be construed as a potential conflict of interest.
The author(s) declared that they were an editorial board member of Frontiers, at the time of submission. This had no impact on the peer review process and the final decision.
Publisher’s note
All claims expressed in this article are solely those of the authors and do not necessarily represent those of their affiliated organizations, or those of the publisher, the editors and the reviewers. Any product that may be evaluated in this article, or claim that may be made by its manufacturer, is not guaranteed or endorsed by the publisher.
References
1. Chandramouli, K, and Qian, PY. Proteomics: challenges, techniques and possibilities to overcome biological sample complexity. Hum Genomics Proteomics. (2009) 2009:239204. doi: 10.4061/2009/239204
2. Alaaeddine, R, Fayad, M, Nehme, E, Bahmad, HF, and Kobeissy, F. The emerging role of proteomics in precision medicine: applications in neurodegenerative diseases and Neurotrauma. Adv Exp Med Biol. (2017) 1007:59–70. doi: 10.1007/978-3-319-60733-7_4
3. Denslow, N, Michel, ME, Temple, MD, Hsu, CY, Saatman, K, and Hayes, RL. Application of proteomics technology to the field of neurotrauma. J Neurotrauma. (2003) 20:401–7. doi: 10.1089/089771503765355487
4. Ottens, AK, Kobeissy, FH, Fuller, BF, Liu, MC, Oli, MW, Hayes, RL, et al. Novel neuroproteomic approaches to studying traumatic brain injury. Prog Brain Res. (2007) 161:401–18. doi: 10.1016/S0079-6123(06)61029-7
5. Ottens, AK, Kobeissy, FH, Golden, EC, Zhang, Z, Haskins, WE, Chen, S-S, et al. Neuroproteomics in neurotrauma. Mass Spectrom Rev. (2006) 25:380–408. doi: 10.1002/mas.20073
6. Omenn, GS. The strategy, organization, and progress of the HUPO human proteome project. J Proteome. (2014) 100:3–7. doi: 10.1016/j.jprot.2013.10.012
7. Al-Amrani, S, Al-Jabri, Z, Al-Zaabi, A, Alshekaili, J, and Al-Khabori, M. Proteomics: concepts and applications in human medicine. World J Biol Chem. (2021) 12:57–69. doi: 10.4331/wjbc.v12.i5.57
8. Manzoni, C, Kia, DA, Vandrovcova, J, Hardy, J, Wood, NW, Lewis, PA, et al. Genome, transcriptome and proteome: the rise of omics data and their integration in biomedical sciences. Brief Bioinform. (2018) 19:286–302. doi: 10.1093/bib/bbw114
9. Husain, I, Ahmad, W, Ali, A, Anwar, L, Nuruddin, SM, Ashraf, K, et al. Functional Neuroproteomics: an imperative approach for unravelling protein implicated complexities of brain. CNS Neurol Disord Drug Targets. (2021) 20:613–24. doi: 10.2174/1871527320666210202121624
10. Mann, M, Kumar, C, Zeng, WF, and Strauss, MT. Artificial intelligence for proteomics and biomarker discovery. Cell Syst. (2021) 12:759–70. doi: 10.1016/j.cels.2021.06.006
11. Ashraf, GM, Athanasios, A, Barreto, GE, and Uddin, MS. Neuroproteomics on the rise (part II). Curr Protein Pept Sci. (2021) 22:441. doi: 10.2174/138920372206211116162544
12. Pomilio, AB, Vitale, AA, and Lazarowski, AJ. Neuroproteomics Chip-based mass spectrometry and other techniques for Alzheimer's disease biomarkers - update. Curr Pharm Des. (2022) 28:1124–51. doi: 10.2174/1381612828666220413094918
13. van Gelder, C, and Altelaar, M. Neuroproteomics of the synapse: subcellular quantification of protein networks and signaling dynamics. Mol Cell Proteomics. (2021) 20:100087. doi: 10.1016/j.mcpro.2021.100087
14. Wang, KK, Ottens, AK, Liu, MC, Lewis, SB, Meegan, C, Oli, MW, et al. Proteomic identification of biomarkers of traumatic brain injury. Expert Rev Proteomics. (2005) 2:603–14. doi: 10.1586/14789450.2.4.603
15. Albayar, AA, Roche, A, Swiatkowski, P, Antar, S, Ouda, N, Emara, E, et al. Biomarkers in spinal cord injury: prognostic insights and future potentials. Front Neurol. (2019) 10:27. doi: 10.3389/fneur.2019.00027
16. Hochrainer, K, and Yang, W. Stroke proteomics: from discovery to diagnostic and therapeutic applications. Circ Res. (2022) 130:1145–66. doi: 10.1161/CIRCRESAHA.122.320110
17. Medina, M. An overview on the clinical development of tau-based therapeutics. Int J Mol Sci. (2018) 19:1160. doi: 10.3390/ijms19041160
18. Uddin, MS, Athanasios, A, Barreto, GE, and Ashraf, GM. Neuroproteomics on the rise (part I). Curr Protein Pept Sci. (2020) 21:1144–5. doi: 10.2174/138920372112201210094133
19. Devaux, S, Cizkova, D, Quanico, J, Franck, J, Nataf, S, Pays, L, et al. Proteomic analysis of the Spatio-temporal based molecular kinetics of acute spinal cord injury identifies a time-and segment-specific window for effective tissue repair. Mol Cell Proteomics. (2016) 15:2641–70. doi: 10.1074/mcp.M115.057794
20. Kobeissy, FH, Ottens, AK, Zhang, Z, Liu, MC, Denslow, ND, Dave, JR, et al. Novel differential Neuroproteomics analysis of traumatic brain injury in rats. Mol Cell Proteomics. (2006) 5:1887–98. doi: 10.1074/mcp.M600157-MCP200
21. Kobeissy, FH, Guingab-Cagmat, JD, Zhang, Z, Moghieb, A, Glushakova, OY, Mondello, S, et al. Neuroproteomics and systems biology approach to identify temporal biomarker changes post experimental traumatic brain injury in rats. Front Neurol. (2016) 7:198. doi: 10.3389/fneur.2016.00198
22. Ren, C, Guingab-Cagmat, J, Kobeissy, F, Zoltewicz, S, Mondello, S, Gao, M, et al. A neuroproteomic and systems biology analysis of rat brain post intracerebral hemorrhagic stroke. Brain Res Bull. (2014) 102:46–56. doi: 10.1016/j.brainresbull.2014.02.005
24. Ramadan, N, Ghazale, H, El-Sayyad, M, El-Haress, M, and Kobeissy, FH. Neuroproteomics studies: challenges and updates. Methods Mol Biol. (2017) 1598:3–19. doi: 10.1007/978-1-4939-6952-4_1
25. Shevchenko, G, Konzer, A, Musunuri, S, and Bergquist, J. Neuroproteomics tools in clinical practice. Biochim Biophys Acta. (2015) 1854:705–17. doi: 10.1016/j.bbapap.2015.01.016
26. Freeman, WM, Brebner, K, Amara, SG, Reed, MS, Pohl, J, and Phillips, AG. Distinct proteomic profiles of amphetamine self-administration transitional states. Pharmacogenomics J. (2005) 5:203–14. doi: 10.1038/sj.tpj.6500309
27. Morrison, RS, Kinoshita, Y, Johnson, MD, Uo, T, Ho, JT, McBee, JK, et al. Proteomic analysis in the neurosciences. Mol Cell Proteomics. (2002) 1:553–60. doi: 10.1074/mcp.R200004-MCP200
28. Paulson, AF, Prasad, MS, Thuringer, AH, and Manzerra, P. Regulation of cadherin expression in nervous system development. Cell Adhes Migr. (2014) 8:19–28. doi: 10.4161/cam.27839
29. Wang, KK, Ottens, A, Haskins, W, Liu, MC, Kobeissy, F, Denslow, N, et al. Proteomics studies of traumatic brain injury. Int Rev Neurobiol. (2004) 61:215–40. doi: 10.1016/S0074-7742(04)61009-9
30. Wu, Q, and Maniatis, T. A striking Organization of a Large Family of human neural cadherin-like cell adhesion genes. Cells. (1999) 97:779–90. doi: 10.1016/S0092-8674(00)80789-8
31. Hünnerkopf, R, Grassl, J, and Thome, J. Proteomics-Biomarkerforschung in der Psychiatrie. Fortschritte Neurol Psychiatr. (2007) 75:579–85. doi: 10.1055/s-2007-959249
32. Missler, M, and Südhof, TC. Neurexins: three genes and 1001 products. Trends Genet. (1998) 14:20–6. doi: 10.1016/S0168-9525(97)01324-3
33. Kim, SI, Voshol, H, Van Oostrum, J, Hastings, TG, Cascio, M, and Glucksman, MJ. Neuroproteomics: expression profiling of the Brain's proteomes in health and disease. Neurochem Res. (2004) 29:1317–31. doi: 10.1023/B:NERE.0000023618.35579.7c
34. Williams, K, Wu, T, Colangelo, C, and Nairn, AC. Recent advances in neuroproteomics and potential application to studies of drug addiction. Neuropharmacology. (2004) 47:148–66. doi: 10.1016/j.neuropharm.2004.07.009
35. Patton, WF. Detection technologies in proteome analysis. J Chromatogr B Analyt Technol Biomed Life Sci. (2002) 771:3–31. doi: 10.1016/S1570-0232(02)00043-0
36. Aebersold, R, and Mann, M. Mass spectrometry-based proteomics. Nature. (2003) 422:198–207. doi: 10.1038/nature01511
37. Cao, Z, and Yu, LR. Mass spectrometry-based proteomics for biomarker discovery. Methods Mol Biol. (2022) 2486:3–17. doi: 10.1007/978-1-0716-2265-0_1
38. Cifani, P, and Kentsis, A. Towards comprehensive and quantitative proteomics for diagnosis and therapy of human disease. Proteomics. (2017) 17:79. doi: 10.1002/pmic.201600079
39. Guerrera, IC, and Kleiner, O. Application of mass spectrometry in proteomics. Biosci Rep. (2005) 25:71–93. doi: 10.1007/s10540-005-2849-x
40. Scigelova, M, and Makarov, A. Orbitrap mass analyzer–overview and applications in proteomics. Proteomics. (2006) 6:16–21. doi: 10.1002/pmic.200600528
41. Hanrieder, J, Nyakas, A, Naessén, T, and Bergquist, J. Proteomic analysis of human follicular fluid using an alternative bottom-up approach. J Proteome Res. (2008) 7:443–9. doi: 10.1021/pr070277z
42. Hultin-Rosenberg, L, Forshed, J, Branca, RM, Lehtiö, J, and Johansson, HJ. Defining, comparing, and improving iTRAQ quantification in mass spectrometry proteomics data. Mol Cell Proteomics. (2013) 12:2021–31. doi: 10.1074/mcp.M112.021592
43. Chen, X, Wei, S, Ji, Y, Guo, X, and Yang, F. Quantitative proteomics using SILAC: principles, applications, and developments. Proteomics. (2015) 15:3175–92. doi: 10.1002/pmic.201500108
44. Qi, H, Xue, JB, Lai, DY, Li, A, and Tao, SC. Current advances in antibody-based serum biomarker studies: from protein microarray to phage display. Proteomics Clin Appl. (2022) 16:e2100098. doi: 10.1002/prca.202100098
45. Liu, MC, Akle, V, Zheng, W, Dave, JR, Tortella, FC, Hayes, RL, et al. Comparing calpain-and caspase-3-mediated degradation patterns in traumatic brain injury by differential proteome analysis. Biochem J. (2006) 394:715–25. doi: 10.1042/BJ20050905
46. Yao, C, Williams, AJ, Ottens, AK, May Lu, XC, Chen, R, Wang, KK, et al. Detection of protein biomarkers using high-throughput immunoblotting following focal ischemic or penetrating ballistic-like brain injuries in rats. Brain Inj. (2008) 22:723–32. doi: 10.1080/02699050802304706
47. Wang, L, and Li, PC. Microfluidic DNA microarray analysis: a review. Anal Chim Acta. (2011) 687:12–27. doi: 10.1016/j.aca.2010.11.056
48. Mathur, S, and Sutton, J. Personalized medicine could transform healthcare. Biomed Rep. (2017) 7:3–5. doi: 10.3892/br.2017.922
49. Dong, X, Huang, Y, Cho, BG, Zhong, J, Gautam, S, Peng, W, et al. Advances in mass spectrometry-based glycomics. Electrophoresis. (2018) 39:3063–81. doi: 10.1002/elps.201800273
50. Donohoo, KB, Wang, J, Goli, M, Yu, A, Peng, W, Hakim, MA, et al. Advances in mass spectrometry-based glycomics-an update covering the period 2017-2021. Electrophoresis. (2022) 43:119–42. doi: 10.1002/elps.202100199
51. Goli, M, Yu, A, Cho, BG, Gautam, S, Wang, J, Gutierrez-Reyes, CD, et al. LC-MS/MS in glycomics and glycoproteomics analyses In: Z El Rassi, editor. Carbohydrate analysis by modern liquid phase separation techniques. Amsterdam: Elsevier (2021). 391–441.
52. Karve, TM, and Cheema, AK. Small changes huge impact: the role of protein posttranslational modifications in cellular homeostasis and disease. J Amino Acids. (2011) 2011:207691. doi: 10.4061/2011/207691
53. Kobata, A. Glycobiology in the field of aging research–introduction to glycogerontology. Biochimie. (2003) 85:13–24. doi: 10.1016/S0300-9084(03)00003-8
54. Abou-Abbass, H, Abou-El-Hassan, H, Bahmad, H, Zibara, K, Zebian, A, Youssef, R, et al. Glycosylation and other PTMs alterations in neurodegenerative diseases: current status and future role in neurotrauma. Electrophoresis. (2016) 37:1549–61. doi: 10.1002/elps.201500585
55. Kizuka, Y, Kitazume, S, and Taniguchi, N. N-glycan and Alzheimer's disease. Biochim Biophys Acta Gen Subj. (2017) 1861:2447–54. doi: 10.1016/j.bbagen.2017.04.012
56. Schedin-Weiss, S, Winblad, B, and Tjernberg, LO. The role of protein glycosylation in Alzheimer disease. FEBS J. (2014) 281:46–62. doi: 10.1111/febs.12590
57. Mondello, S, Sandner, V, Goli, M, Czeiter, E, Amrein, K, Kochanek, PM, et al. Exploring serum glycome patterns after moderate to severe traumatic brain injury: a prospective pilot study. EClinicalMedicine. (2022) 50:101494. doi: 10.1016/j.eclinm.2022.101494
58. Russell, AC, Šimurina, M, Garcia, MT, Novokmet, M, Wang, Y, Rudan, I, et al. The N-glycosylation of immunoglobulin G as a novel biomarker of Parkinson's disease. Glycobiology. (2017) 27:501–10. doi: 10.1093/glycob/cwx022
59. Freeze, HH, Chong, JX, Bamshad, MJ, and Ng, BG. Solving glycosylation disorders: fundamental approaches reveal complicated pathways. Am J Hum Genet. (2014) 94:161–75. doi: 10.1016/j.ajhg.2013.10.024
60. Van Scherpenzeel, M, Willems, E, and Lefeber, DJ. Clinical diagnostics and therapy monitoring in the congenital disorders of glycosylation. Glycoconj J. (2016) 33:345–58. doi: 10.1007/s10719-015-9639-x
61. Bermingham, ML, Colombo, M, McGurnaghan, SJ, Blackbourn, LAK, Vučković, F, Pučić Baković, M, et al. N-glycan profile and kidney disease in type 1 diabetes. Diabetes Care. (2018) 41:79–87. doi: 10.2337/dc17-1042
62. Everest-Dass, AV, Moh, ESX, Ashwood, C, Shathili, AMM, and Packer, NH. Human disease glycomics: technology advances enabling protein glycosylation analysis - part 2. Expert Rev Proteomics. (2018) 15:341–52. doi: 10.1080/14789450.2018.1448710
63. Chandrasekaran, R, and Lacy, DB. The role of toxins in Clostridium difficile infection. FEMS Microbiol Rev. (2017) 41:723–50. doi: 10.1093/femsre/fux048
64. Blomme, B, Van Steenkiste, C, Callewaert, N, and Van Vlierberghe, H. Alteration of protein glycosylation in liver diseases. J Hepatol. (2009) 50:592–603. doi: 10.1016/j.jhep.2008.12.010
65. Orlova, OV, Drutsa, VL, Spirin, PV, Prasolov, VS, Rubtsov, PM, Kochetkov, SN, et al. The role of HCV e2 protein glycosylation in functioning of virus envelope proteins in insect and mammalian cells. Acta Nat. (2015) 7:87–97. doi: 10.32607/20758251-2015-7-1-87-97
66. Vigerust, DJ. Protein glycosylation in infectious disease pathobiology and treatment. Cent Eur J Biol. (2011) 6:802. doi: 10.2478/s11535-011-0050-8
67. Guo, H, and Abbott, KL. Functional impact of tumor-specific N-linked glycan changes in breast and ovarian cancers. Adv Cancer Res. (2015) 126:281–303. doi: 10.1016/bs.acr.2014.11.006
68. Kailemia, MJ, Park, D, and Lebrilla, CB. Glycans and glycoproteins as specific biomarkers for cancer. Anal Bioanal Chem. (2017) 409:395–410. doi: 10.1007/s00216-016-9880-6
69. Mechref, Y, Hu, Y, Garcia, A, and Hussein, A. Identifying cancer biomarkers by mass spectrometry-based glycomics. Electrophoresis. (2012) 33:1755–67. doi: 10.1002/elps.201100715
70. Mehta, A, Herrera, H, and Block, T. Glycosylation and liver cancer. Adv Cancer Res. (2015) 126:257–79. doi: 10.1016/bs.acr.2014.11.005
71. Pan, S, Brentnall, TA, and Chen, R. Glycoproteins and glycoproteomics in pancreatic cancer. World J Gastroenterol. (2016) 22:9288–99. doi: 10.3748/wjg.v22.i42.9288
72. Peng, W, Gutierrez Reyes, CD, Gautam, S, Yu, A, Cho, BG, Goli, M, et al. MS-based glycomics and glycoproteomics methods enabling isomeric characterization. Mass Spectrom Rev. (2023) 42:577–616. doi: 10.1002/mas.21713
73. Peng, W, Goli, M, Mirzaei, P, and Mechref, Y. Revealing the biological attributes of N-glycan isomers in breast Cancer brain metastasis using porous graphitic carbon (PGC) liquid chromatography-tandem mass spectrometry (LC-MS/MS). J Proteome Res. (2019) 18:3731–40. doi: 10.1021/acs.jproteome.9b00429
74. Kobeissy, F, Kobaisi, A, Peng, W, Barsa, C, Goli, M, Sibahi, A, et al. Glycomic and Glycoproteomic techniques in neurodegenerative disorders and Neurotrauma: towards personalized markers. Cells. (2022) 11:581. doi: 10.3390/cells11030581
75. Kim, EH, and Misek, DE. Glycoproteomics-based identification of cancer biomarkers. Int J Proteomics. (2011) 2011:601937. doi: 10.1155/2011/601937
76. Wei, X, and Li, L. Comparative glycoproteomics: approaches and applications. Brief Funct Genomic Proteomic. (2009) 8:104–13. doi: 10.1093/bfgp/eln053
77. Hood, BL, Malehorn, DE, Conrads, TP, and Bigbee, WL. Serum proteomics using mass spectrometry. Methods Mol Biol. (2009) 520:107–28. doi: 10.1007/978-1-60327-811-9_8
78. Ongay, S, Boichenko, A, Govorukhina, N, and Bischoff, R. Glycopeptide enrichment and separation for protein glycosylation analysis. J Sep Sci. (2012) 35:2341–72. doi: 10.1002/jssc.201200434
79. Yang, H, Zhou, B, Deng, H, Prinz, M, and Siegel, D. Body fluid identification by mass spectrometry. Int J Legal Med. (2013) 127:1065–77. doi: 10.1007/s00414-013-0848-1
80. Descamps, A, Van der Borght, K, De Spiegeleer, A, Wynendaele, E, and De Spiegeleer, B. Peptidomics: LC-MS operational parameters do matter. J Pharm Biomed Anal. (2023) 229:115348. doi: 10.1016/j.jpba.2023.115348
81. Romanova, EV, and Sweedler, JV. Peptidomics for the discovery and characterization of neuropeptides and hormones. Trends Pharmacol Sci. (2015) 36:579–86. doi: 10.1016/j.tips.2015.05.009
82. Baggerman, G, Verleyen, P, Clynen, E, Huybrechts, J, De Loof, A, and Schoofs, L. Peptidomics. J Chromatogr B Analyt Technol Biomed Life Sci. (2004) 803:3–16. doi: 10.1016/j.jchromb.2003.07.019
83. Li, L, Wu, J, Lyon, CJ, Jiang, L, and Hu, TY. Clinical peptidomics: advances in instrumentation, analyses, and applications. BME Front. (2023) 4:19. doi: 10.34133/bmef.0019
84. Schrader, M, and Schulz-Knappe, P. Peptidomics technologies for human body fluids. Trends Biotechnol. (2001) 19:S55–60. doi: 10.1016/S0167-7799(01)01800-5
85. Schulz-Knappe, P, Schrader, M, and Zucht, HD. The peptidomics concept. Comb Chem High Throughput Screen. (2005) 8:697–704. doi: 10.2174/138620705774962418
86. Le, TT, Lehnert, S, and Colgrave, ML. Neuropeptidomics applied to studies of mammalian reproduction. Peptidomics. (2014) 1:1–13. doi: 10.2478/ped-2013-0001
87. Baggerman, G, Boonen, K, Verleyen, P, De Loof, A, and Schoofs, L. Peptidomic analysis of the larvalDrosophila melanogaster central nervous system by two-dimensional capillary liquid chromatography quadrupole time-of-flight mass spectrometry. J Mass Spectrom. (2005) 40:250–60. doi: 10.1002/jms.744
88. Eipper, BA, Mains, RE, and Herbert, E. Peptides in the nervous system. Trends Neurosci. (1986) 9:463–8.
89. Fricker, LD, Lim, J, Pan, H, and Che, FY. Peptidomics: identification and quantification of endogenous peptides in neuroendocrine tissues. Mass Spectrom Rev. (2006) 25:327–44. doi: 10.1002/mas.20079
90. Che, FY, and Fricker, LD. Quantitative peptidomics of mouse pituitary: comparison of different stable isotopic tags. J Mass Spectrom. (2005) 40:238–49. doi: 10.1002/jms.743
91. Desiderio, DM. Mass spectrometric analysis of neuropeptidergic systems in the human pituitary and cerebrospinal fluid. J Chromatogr B Biomed Sci Appl. (1999) 731:3–22. doi: 10.1016/S0378-4347(99)00172-3
92. Che, FY, Lim, J, Pan, H, Biswas, R, and Fricker, LD. Quantitative neuropeptidomics of microwave-irradiated mouse brain and pituitary. Mol Cell Proteomics. (2005) 4:1391–405. doi: 10.1074/mcp.T500010-MCP200
93. Svensson, M, Sköld, K, Svenningsson, P, and Andren, PE. Peptidomics-based discovery of novel neuropeptides. J Proteome Res. (2003) 2:213–9. doi: 10.1021/pr020010u
94. Reichmann, F, and Holzer, P. Neuropeptide Y: A stressful review. Neuropeptides. (2016) 55:99–109. doi: 10.1016/j.npep.2015.09.008
95. Sandberg, M, and Weber, SG. Techniques for neuropeptide determination. Trends Analyt Chem. (2003) 22:522–7. doi: 10.1016/S0165-9936(03)00910-5
96. Zhao, Y, and Jensen, ON. Modification-specific proteomics: strategies for characterization of post-translational modifications using enrichment techniques. Proteomics. (2009) 9:4632–41. doi: 10.1002/pmic.200900398
97. Miyashita, M, Presley, JM, Buchholz, BA, Lam, KS, Lee, YM, Vogel, JS, et al. Attomole level protein sequencing by Edman degradation coupled with accelerator mass spectrometry. Proc Natl Acad Sci U S A. (2001) 98:4403–8. doi: 10.1073/pnas.071047998
98. Kumar, M, Joseph, SR, Augsburg, M, Bogdanova, A, Drechsel, D, Vastenhouw, NL, et al. MS Western, a method of multiplexed absolute protein quantification is a practical alternative to Western blotting. Mol Cell Proteomics. (2018) 17:384–96. doi: 10.1074/mcp.O117.067082
99. Rabilloud, T, and Lelong, C. Two-dimensional gel electrophoresis in proteomics: a tutorial. J Proteome. (2011) 74:1829–41. doi: 10.1016/j.jprot.2011.05.040
100. Jorgenson, JW. Capillary liquid chromatography at ultrahigh pressures. Annu Rev Anal Chem (Palo Alto, Calif). (2010) 3:129–50. doi: 10.1146/annurev.anchem.1.031207.113014
101. de Jong, EP, and Griffin, TJ. Online nanoscale ERLIC-MS outperforms RPLC-MS for shotgun proteomics in complex mixtures. J Proteome Res. (2012) 11:5059–64. doi: 10.1021/pr300638n
102. Colgrave, ML, Xi, L, Lehnert, SA, Flatscher-Bader, T, Wadensten, H, Nilsson, A, et al. Neuropeptide profiling of the bovine hypothalamus: thermal stabilization is an effective tool in inhibiting post-mortem degradation. Proteomics. (2011) 11:1264–76. doi: 10.1002/pmic.201000423
103. Secher, A, Kelstrup, CD, Conde-Frieboes, KW, Pyke, C, Raun, K, Wulff, BS, et al. Analytic framework for peptidomics applied to large-scale neuropeptide identification. Nat Commun. (2016) 7:11436. doi: 10.1038/ncomms11436
104. Fälth, M, Sköld, K, Norrman, M, Svensson, M, Fenyö, D, and Andren, PE. SwePep, a database designed for endogenous peptides and mass spectrometry. Mol Cell Proteomics. (2006) 5:998–1005. doi: 10.1074/mcp.M500401-MCP200
105. Sauer, CS, and Li, L. Multiplexed quantitative neuropeptidomics via DiLeu isobaric tagging. Methods Enzymol. (2022) 663:235–57. doi: 10.1016/bs.mie.2021.10.011
106. Hou, X, Yuan, Z, Wang, X, Cheng, R, Zhou, X, and Qiu, J. Peptidome analysis of cerebrospinal fluid in neonates with hypoxic-ischemic brain damage. Mol Brain. (2020) 13:133. doi: 10.1186/s13041-020-00671-9
107. Colinge, J, Rix, U, Bennett, KL, and Superti-Furga, G. Systems biology analysis of protein-drug interactions. Proteomics Clin Appl. (2012) 6:102–16. doi: 10.1002/prca.201100077
108. Özdemir, V, Dove, ES, Gürsoy, UK, Şardaş, S, Yıldırım, A, Yılmaz, ŞG, et al. Personalized medicine beyond genomics: alternative futures in big data—proteomics, environtome and the social proteome. J Neural Transm. (2017) 124:25–32. doi: 10.1007/s00702-015-1489-y
109. Ziegler, A, Koch, A, Krockenberger, K, and Grosshennig, A. Personalized medicine using DNA biomarkers: a review. Hum Genet. (2012) 131:1627–38. doi: 10.1007/s00439-012-1188-9
110. Jain, KK. Role of proteomics in the development of personalized medicine. Adv Protein Chem Struct Biol. (2016) 102:41–52. doi: 10.1016/bs.apcsb.2015.09.002
111. Minakshi, P, Kumar, R, Ghosh, M, Saini, HM, Ranjan, K, Brar, B, et al. Single-cell proteomics: Technology and applications In: D Barh and O Azevedo, editors. Single-cell omics. Amsterdam: Elsevier (2019). 283–318.
112. Bendall, SC, Simonds, EF, Qiu, P, Amir, EAD, Krutzik, PO, Finck, R, et al. Single-cell mass cytometry of differential immune and drug responses across a human hematopoietic continuum. Science. (2011) 332:687–96. doi: 10.1126/science.1198704
113. Su, Y, Shi, Q, and Wei, W. Single cell proteomics in biomedicine: high-dimensional data acquisition, visualization, and analysis. Proteomics. (2017) 17:1600267. doi: 10.1002/pmic.201600267
114. Wu, M, and Singh, AK. Single-cell protein analysis. Curr Opin Biotechnol. (2012) 23:83–8. doi: 10.1016/j.copbio.2011.11.023
115. Templer, RH, and Ces, O. New frontiers in single-cell analysis. J R Soc Interface. (2008) 5 Suppl 2:S111–2. doi: 10.1098/rsif.2008.0279.focus
116. Goldman, AR, Beer, LA, Tang, HY, Hembach, P, Zayas-Bazan, D, and Speicher, DW. Proteome analysis using gel-LC-MS/MS. Curr Protoc Protein Sci. (2019) 96:e93. doi: 10.1002/cpps.93
117. Milo, R. What is the total number of protein molecules per cell volume? A call to rethink some published values. BioEssays. (2013) 35:1050–5. doi: 10.1002/bies.201300066
118. Klaeger, S, Apffel, A, Clauser, KR, Sarkizova, S, Oliveira, G, Rachimi, S, et al. Optimized liquid and gas phase fractionation increases HLA-Peptidome coverage for primary cell and tissue samples. Mol Cell Proteomics. (2021) 20:100133. doi: 10.1016/j.mcpro.2021.100133
119. Ma, C, Fan, R, and Elitas, M. Single cell functional proteomics for assessing immune response in cancer therapy: technology, methods, and applications. Front Oncol. (2013) 3:133. doi: 10.3389/fonc.2013.00133
120. Fonslow, BR, and Yates, JR. Capillary electrophoresis applied to proteomic analysis. J Sep Sci. (2009) 32:1175–88. doi: 10.1002/jssc.200800592
121. Gackler, A, Mulling, N, Volk, K, Wilde, B, Eisenberger, U, Rohn, H, et al. Establishment of an ELISpot assay to detect cellular immunity against S. pneumoniae in vaccinated kidney transplant recipients. Vaccine. (2021) 9:1438. doi: 10.3390/vaccines9121438
122. De Rosa, SC, Herzenberg, LA, and Roederer, M. 11-color, 13-parameter flow cytometry: identification of human naive T cells by phenotype, function, and T-cell receptor diversity. Nat Med. (2001) 7:245–8. doi: 10.1038/84701
123. Perez, OD, and Nolan, GP. Simultaneous measurement of multiple active kinase states using polychromatic flow cytometry. Nat Biotechnol. (2002) 20:155–62. doi: 10.1038/nbt0202-155
124. Perfetto, SP, Chattopadhyay, PK, and Roederer, M. Seventeen-colour flow cytometry: unravelling the immune system. Nat Rev Immunol. (2004) 4:648–55. doi: 10.1038/nri1416
125. Papagiannopoulou, C, Parchen, R, Rubbens, P, and Waegeman, W. Fast pathogen identification using single-cell matrix-assisted laser desorption/ionization-aerosol time-of-flight mass spectrometry data and deep learning methods. Anal Chem. (2020) 92:7523–31. doi: 10.1021/acs.analchem.9b05806
126. Schober, Y, Guenther, S, Spengler, B, and Rompp, A. Single cell matrix-assisted laser desorption/ionization mass spectrometry imaging. Anal Chem. (2012) 84:6293–7. doi: 10.1021/ac301337h
127. Tuli, L, and Ressom, HW. LC-MS based detection of differential protein expression. J Proteomics Bioinform. (2009) 02:416–38. doi: 10.4172/jpb.1000102
128. Shao, X, Wang, X, Guan, S, Lin, H, Yan, G, Gao, M, et al. Integrated proteome analysis device for fast single-cell protein profiling. Anal Chem. (2018) 90:14003–10. doi: 10.1021/acs.analchem.8b03692
129. Li, ZY, Huang, M, Wang, XK, Zhu, Y, Li, JS, Wong, CCL, et al. Nanoliter-scale oil-air-droplet Chip-based single cell proteomic analysis. Anal Chem. (2018) 90:5430–8. doi: 10.1021/acs.analchem.8b00661
130. Wofford, KL, Harris, JP, Browne, KD, Brown, DP, Grovola, MR, Mietus, CJ, et al. Rapid neuroinflammatory response localized to injured neurons after diffuse traumatic brain injury in swine. Exp Neurol. (2017) 290:85–94. doi: 10.1016/j.expneurol.2017.01.004
131. Gunawardena, HP, O'Brien, J, Wrobel, JA, Xie, L, Davies, SR, Li, S, et al. QuantFusion: novel unified methodology for enhanced coverage and precision in quantifying global proteomic changes in whole tissues. Mol Cell Proteomics. (2016) 15:740–51. doi: 10.1074/mcp.O115.049791
132. Liu, T, Hu, J, and Li, H. iTRAQ-based shotgun neuroproteomics. Methods Mol Biol. (2009) 566:201–16. doi: 10.1007/978-1-59745-562-6_14
133. Schulze, WX, and Usadel, B. Quantitation in mass-spectrometry-based proteomics. Annu Rev Plant Biol. (2010) 61:491–516. doi: 10.1146/annurev-arplant-042809-112132
134. Krijgsveld, J, Ketting, RF, Mahmoudi, T, Johansen, J, Artal-Sanz, M, Verrijzer, CP, et al. Metabolic labeling of C. elegans and D. melanogaster for quantitative proteomics. Nat Biotechnol. (2003) 21:927–31. doi: 10.1038/nbt848
135. Mann, M. Functional and quantitative proteomics using SILAC. Nat Rev Mol Cell Biol. (2006) 7:952–8. doi: 10.1038/nrm2067
136. Ishihama, Y, Sato, T, Tabata, T, Miyamoto, N, Sagane, K, Nagasu, T, et al. Quantitative mouse brain proteomics using culture-derived isotope tags as internal standards. Nat Biotechnol. (2005) 23:617–21. doi: 10.1038/nbt1086
137. Hoedt, E, Zhang, G, and Neubert, TA. Stable isotope labeling by amino acids in cell culture (SILAC) for quantitative proteomics. Adv Exp Med Biol. (2014) 806:93–106. doi: 10.1007/978-3-319-06068-2_5
138. Wu, P, Zhao, Y, Haidacher, SJ, Wang, E, Parsley, MO, Gao, J, et al. Detection of structural and metabolic changes in traumatically injured hippocampus by quantitative differential proteomics. J Neurotrauma. (2013) 30:775–88. doi: 10.1089/neu.2012.2391
139. Brunner, AM, Tweedie-Cullen, RY, and Mansuy, IM. Epigenetic modifications of the neuroproteome. Proteomics. (2012) 12:2404–20. doi: 10.1002/pmic.201100672
140. Pappireddi, N, Martin, L, and Wühr, M. A review on quantitative multiplexed proteomics. Chembiochem. (2019) 20:1210–24. doi: 10.1002/cbic.201800650
141. Thompson, A, Schäfer, J, Kuhn, K, Kienle, S, Schwarz, J, Schmidt, G, et al. Tandem mass tags: a novel quantification strategy for comparative analysis of complex protein mixtures by MS/MS. Anal Chem. (2003) 75:1895–904. doi: 10.1021/ac0262560
142. Jones, AM, Bennett, MH, Mansfield, JW, and Grant, M. Analysis of the defence phosphoproteome of Arabidopsis thaliana using differential mass tagging. Proteomics. (2006) 6:4155–65. doi: 10.1002/pmic.200500172
143. Nühse, TS, Bottrill, AR, Jones, AM, and Peck, SC. Quantitative phosphoproteomic analysis of plasma membrane proteins reveals regulatory mechanisms of plant innate immune responses. Plant J. (2007) 51:931–40. doi: 10.1111/j.1365-313X.2007.03192.x
144. Hu, J, Qian, J, Borisov, O, Pan, S, Li, Y, Liu, T, et al. Optimized proteomic analysis of a mouse model of cerebellar dysfunction using amine-specific isobaric tags. Proteomics. (2006) 6:4321–34. doi: 10.1002/pmic.200600026
145. Shadforth, IP, Dunkley, TPJ, Lilley, KS, and Bessant, C. I-tracker: for quantitative proteomics using iTRAQ™. BMC Genomics. (2005) 6:145. doi: 10.1186/1471-2164-6-145
146. Gygi, SP, Rist, B, Gerber, SA, Turecek, F, Gelb, MH, and Aebersold, R. Quantitative analysis of complex protein mixtures using isotope-coded affinity tags. Nat Biotechnol. (1999) 17:994–9. doi: 10.1038/13690
147. Griffin, TJ, Gygi, SP, Rist, B, Aebersold, R, Loboda, A, Jilkine, A, et al. Quantitative proteomic analysis using a MALDI quadrupole time-of-flight mass spectrometer. Anal Chem. (2001) 73:978–86. doi: 10.1021/ac001169y
148. Lill, J. Proteomic tools for quantitation by mass spectrometry. Mass Spectrom Rev. (2003) 22:182–94. doi: 10.1002/mas.10048
149. Zecha, J, Satpathy, S, Kanashova, T, Avanessian, SC, Kane, MH, Clauser, KR, et al. TMT labeling for the masses: a robust and cost-efficient, in-solution labeling approach. Mol Cell Proteomics. (2019) 18:1468–78. doi: 10.1074/mcp.TIR119.001385
150. Song, H, Fang, S, Gao, J, Wang, J, Cao, Z, Guo, Z, et al. Quantitative proteomic study reveals up-regulation of cAMP signaling pathway-related proteins in mild traumatic brain injury. J Proteome Res. (2018) 17:858–69. doi: 10.1021/acs.jproteome.7b00618
151. Wang, H, Chen, J, Gao, C, Chen, W, Chen, G, Zhang, M, et al. TMT-based proteomics analysis to screen potential biomarkers of acute-phase TBI in rats. Life Sci. (2021) 264:118631. doi: 10.1016/j.lfs.2020.118631
152. Anand, S, Samuel, M, Ang, CS, Keerthikumar, S, and Mathivanan, S. Label-based and label-free strategies for protein quantitation. Methods Mol Biol. (2017) 1549:31–43. doi: 10.1007/978-1-4939-6740-7_4
153. Lausted, C, Lee, I, Zhou, Y, Qin, S, Sung, J, Price, ND, et al. Systems approach to neurodegenerative disease biomarker discovery. Annu Rev Pharmacol Toxicol. (2014) 54:457–81. doi: 10.1146/annurev-pharmtox-011613-135928
154. Neilson, KA, Ali, NA, Muralidharan, S, Mirzaei, M, Mariani, M, Assadourian, G, et al. Less label, more free: approaches in label-free quantitative mass spectrometry. Proteomics. (2011) 11:535–53. doi: 10.1002/pmic.201000553
155. Chelius, D, and Bondarenko, PV. Quantitative profiling of proteins in complex mixtures using liquid chromatography and mass spectrometry. J Proteome Res. (2002) 1:317–23. doi: 10.1021/pr025517j
156. Podwojski, K, Eisenacher, M, Kohl, M, Turewicz, M, Meyer, HE, Rahnenführer, J, et al. Peek a peak: a glance at statistics for quantitative label-free proteomics. Expert Rev Proteomics. (2010) 7:249–61. doi: 10.1586/epr.09.107
157. Liu, H, Sadygov, RG, and Yates, JR. A model for random sampling and estimation of relative protein abundance in shotgun proteomics. Anal Chem. (2004) 76:4193–201. doi: 10.1021/ac0498563
158. Rappsilber, J, Ryder, U, Lamond, AI, and Mann, M. Large-scale proteomic analysis of the human spliceosome. Genome Res. (2002) 12:1231–45. doi: 10.1101/gr.473902
159. Cortes, DF, Landis, MK, and Ottens, AK. High-capacity peptide-centric platform to decode the proteomic response to brain injury. Electrophoresis. (2012) 33:3712–9. doi: 10.1002/elps.201200341
160. Patel, PD, Stafflinger, JE, Marwitz, JH, Niemeier, JP, and Ottens, AK. Secreted peptides for diagnostic trajectory assessments in brain injury rehabilitation. Neurorehabil Neural Repair. (2021) 35:169–84. doi: 10.1177/1545968320975428
161. Pham, L, Wright, DK, O'Brien, WT, Bain, J, Huang, C, Sun, M, et al. Behavioral, axonal, and proteomic alterations following repeated mild traumatic brain injury: novel insights using a clinically relevant rat model. Neurobiol Dis. (2021) 148:105151. doi: 10.1016/j.nbd.2020.105151
162. Mattugini, N, Merl-Pham, J, Petrozziello, E, Schindler, L, Bernhagen, J, Hauck, SM, et al. Influence of white matter injury on gray matter reactive gliosis upon stab wound in the adult murine cerebral cortex. Glia. (2018) 66:1644–62. doi: 10.1002/glia.23329
163. Song, H, Chen, C, Kelley, B, Tomasevich, A, Lee, H, Dolle, JP, et al. Traumatic brain injury recapitulates developmental changes of axons. Prog Neurobiol. (2022) 217:102332. doi: 10.1016/j.pneurobio.2022.102332
164. Lizhnyak, PN, Muldoon, PP, Pilaka, PP, Povlishock, JT, and Ottens, AK. Traumatic brain injury temporal proteome guides KCC2-targeted therapy. J Neurotrauma. (2019) 36:3092–102. doi: 10.1089/neu.2019.6415
165. Barnidge, DR, Tschumper, RC, Theis, JD, Snyder, MR, Jelinek, DF, Katzmann, JA, et al. Monitoring M-proteins in patients with multiple myeloma using heavy-chain variable region clonotypic peptides and LC–MS/MS. J Proteome Res. (2014) 13:1905–10. doi: 10.1021/pr5000544
166. Hoofnagle, AN, Whiteaker, JR, Carr, SA, Kuhn, E, Liu, T, Massoni, SA, et al. Recommendations for the generation, quantification, storage, and handling of peptides used for mass spectrometry–based assays. Clin Chem. (2016) 62:48–69. doi: 10.1373/clinchem.2015.250563
167. Li, K, Ganz, AB, and Smit, AB. Proteomics of neurodegenerative diseases: analysis of human post-mortem brain. J Neurochem. (2019) 151:435–45. doi: 10.1111/jnc.14603
168. Garcia-Berrocoso, T, Llombart, V, Colas-Campas, L, Hainard, A, Licker, V, Penalba, A, et al. Single cell Immuno-laser microdissection coupled to label-free proteomics to reveal the Proteotypes of human brain cells after ischemia. Mol Cell Proteomics. (2018) 17:175–89. doi: 10.1074/mcp.RA117.000419
169. Tyanova, S, Temu, T, and Cox, J. The MaxQuant computational platform for mass spectrometry-based shotgun proteomics. Nat Protoc. (2016) 11:2301–19. doi: 10.1038/nprot.2016.136
170. Wenger, CD, and Coon, JJ. A proteomics search algorithm specifically designed for high-resolution tandem mass spectra. J Proteome Res. (2013) 12:1377–86. doi: 10.1021/pr301024c
171. Tyanova, S., and Cox, J. (2018). \. Cancer Syst Biol, 1711: 133–148. doi: 10.1007/978-1-4939-7493-1_7
172. Crowgey, EL, Matlock, A, Venkatraman, V, Fert-Bober, J, and Van Eyk, JE. Mapping biological networks from quantitative data-independent acquisition mass spectrometry: Data to knowledge pipelines. Methods Mol Biol. (2017) 1558:395–413. doi: 10.1007/978-1-4939-6783-4_19
173. Meyer, JG, and Schilling, B. Clinical applications of quantitative proteomics using targeted and untargeted data-independent acquisition techniques. Expert Rev Proteomics. (2017) 14:419–29. doi: 10.1080/14789450.2017.1322904
174. Rayaprolu, S, Higginbotham, L, Bagchi, P, Watson, CM, Zhang, T, Levey, AI, et al. Systems-based proteomics to resolve the biology of Alzheimer’s disease beyond amyloid and tau. Neuropsychopharmacology. (2021) 46:98–115. doi: 10.1038/s41386-020-00840-3
175. Kelstrup, CD, Bekker-Jensen, DB, Arrey, TN, Hogrebe, A, Harder, A, and Olsen, JV. Performance evaluation of the Q exactive HF-X for shotgun proteomics. J Proteome Res. (2018) 17:727–38. doi: 10.1021/acs.jproteome.7b00602
176. Koopmans, F, Ho, JT, Smit, AB, and Li, KW. Comparative analyses of data independent acquisition mass spectrometric approaches: DIA, WiSIM-DIA, and untargeted DIA. Proteomics. (2018) 18:1700304. doi: 10.1002/pmic.201700304
177. Santacruz, CA, Vincent, JL, Duitama, J, Bautista, E, Imbault, V, Bruneau, M, et al. The cerebrospinal fluid proteomic response to traumatic and nontraumatic acute brain injury: a prospective study. Neurocrit Care. (2022) 37:463–70. doi: 10.1007/s12028-022-01507-1
178. Ramazi, S, and Zahiri, J. Posttranslational modifications in proteins: resources, tools and prediction methods. Database (Oxford). (2021) 2021:12. doi: 10.1093/database/baab012
179. Bauca, JM, Martinez-Morillo, E, and Diamandis, EP. Peptidomics of urine and other biofluids for cancer diagnostics. Clin Chem. (2014) 60:1052–61. doi: 10.1373/clinchem.2013.211714
180. Maas, AI, Menon, DK, Manley, GT, Abrams, M, Åkerlund, C, Andelic, N, et al. Traumatic brain injury: progress and challenges in prevention, clinical care, and research. Lancet Neurol. (2022) 21:1004–60. doi: 10.1016/S1474-4422(22)00309-X
181. Blennow, K, Brody, DL, Kochanek, PM, Levin, H, McKee, A, Ribbers, GM, et al. Traumatic brain injuries. Nat Rev Dis Primers. (2016) 2:1–19. doi: 10.1038/nrdp.2016.84
182. Graham, DI, Lantos, PL, and Greenfield, JG. Greenfield’s neuropathology. London: Arnold (1997).
183. Corps, KN, Roth, TL, and McGavern, DB. Inflammation and neuroprotection in traumatic brain injury. JAMA Neurol. (2015) 72:355–62. doi: 10.1001/jamaneurol.2014.3558
184. Bramlett, HM, and Dietrich, WD. Pathophysiology of cerebral ischemia and brain trauma: similarities and differences. J Cereb Blood Flow Metab. (2004) 24:133–50. doi: 10.1097/01.WCB.0000111614.19196.04
185. Manek, R, Moghieb, A, Yang, Z, Kumar, D, Kobessiy, F, Sarkis, GA, et al. Protein biomarkers and neuroproteomics characterization of microvesicles/exosomes from human cerebrospinal fluid following traumatic brain injury. Mol Neurobiol. (2018) 55:6112–28. doi: 10.1007/s12035-017-0821-y
186. Lotocki, G, Alonso, OF, Frydel, B, Dietrich, WD, and Keane, RW. Monoubiquitination and cellular distribution of XIAP in neurons after traumatic brain injury. J Cereb Blood Flow Metab. (2003) 23:1129–36. doi: 10.1097/01.WCB.0000086938.68719.E0
187. Shimamura, M, Garcia, JM, Prough, DS, Dewitt, DS, Uchida, T, Shah, SA, et al. Analysis of long-term gene expression in neurons of the hippocampal subfields following traumatic brain injury in rats. Neuroscience. (2005) 131:87–97. doi: 10.1016/j.neuroscience.2004.10.041
188. Sullivan, PG, Rabchevsky, AG, Waldmeier, PC, and Springer, JE. Mitochondrial permeability transition in CNS trauma: cause or effect of neuronal cell death? J Neurosci Res. (2005) 79:231–9. doi: 10.1002/jnr.20292
189. Czogalla, A, and Sikorski, AF. Spectrin and calpain: a ‘target’ and a ‘sniper’ in the pathology of neuronal cells. Cell Mol Life Sci CMLS. (2005) 62:1913–24. doi: 10.1007/s00018-005-5097-0
190. Pineda, JA, Wang, KKW, and Hayes, RL. Biomarkers of proteolytic damage following traumatic brain injury. Brain Pathol. (2004) 14:202–9. doi: 10.1111/j.1750-3639.2004.tb00054.x
191. Wilkins, MR, Pasquali, C, Appel, RD, Ou, K, Golaz, O, Sanchez, J-C, et al. From proteins to proteomes: large scale protein identification by two-dimensional electrophoresis and Arnino acid analysis. Nat Biotechnol. (1996) 14:61–5. doi: 10.1038/nbt0196-61
192. Anada, RP, Wong, KT, Jayapalan, JJ, Hashim, OH, and Ganesan, D. Panel of serum protein biomarkers to grade the severity of traumatic brain injury. Electrophoresis. (2018) 39:2308–15. doi: 10.1002/elps.201700407
193. Guingab-Cagmat, JD, Newsom, K, Vakulenko, A, Cagmat, EB, Kobeissy, FH, Zoltewicz, S, et al. In vitro MS-based proteomic analysis and absolute quantification of neuronal-glial injury biomarkers in cell culture system. Electrophoresis. (2012) 33:3786–97. doi: 10.1002/elps.201200326
194. Kochanek, AR, Kline, AE, Gao, WM, Chadha, M, Lai, Y, Clark, RS, et al. Gel-based hippocampal proteomic analysis 2 weeks following traumatic brain injury to immature rats using controlled cortical impact. Dev Neurosci. (2006) 28:410–9. doi: 10.1159/000094167
195. Buonocore, G, Liberatori, S, Bini, L, Mishra, OP, Delivoria-Papadopoulos, M, Pallini, V, et al. Hypoxic response of synaptosomal proteins in term guinea pig fetuses. J Neurochem. (1999) 73:2139–48. doi: 10.1046/j.1471-4159.1999.02139.x
196. Conti, A, Sanchez-Ruiz, Y, Bachi, A, Beretta, L, Grandi, E, Beltramo, M, et al. Proteome study of human cerebrospinal fluid following traumatic brain injury indicates fibrin(ogen) degradation products as trauma-associated markers. J Neurotrauma. (2004) 21:854–63. doi: 10.1089/0897715041526212
197. Jenkins, LW, Peters, GW, Dixon, CE, Zhang, X, Clark, RS, Skinner, JC, et al. Conventional and functional proteomics using large format two-dimensional gel electrophoresis 24 hours after controlled cortical impact in postnatal day 17 rats. J Neurotrauma. (2002) 19:715–40. doi: 10.1089/08977150260139101
198. Satchell, MA, Zhang, X, Kochanek, PM, Dixon, CE, Jenkins, LW, Melick, J, et al. A dual role for poly-ADP-ribosylation in spatial memory acquisition after traumatic brain injury in mice involving NAD+ depletion and ribosylation of 14-3-3γ. J Neurochem. (2003) 85:697–708. doi: 10.1046/j.1471-4159.2003.01707.x
199. Levine, M, and Tjian, R. Transcription regulation and animal diversity. Nature. (2003) 424:147–51. doi: 10.1038/nature01763
200. Rozanova, S, Barkovits, K, Nikolov, M, Schmidt, C, Urlaub, H, and Marcus, K. Quantitative mass spectrometry-based proteomics: an overview. Methods Mol Biol. (2021) 2228:85–116. doi: 10.1007/978-1-0716-1024-4_8
201. Shen, S, Loo, RR, Wanner, IB, and Loo, JA. Addressing the needs of traumatic brain injury with clinical proteomics. Clin Proteomics. (2014) 11:11. doi: 10.1186/1559-0275-11-11
202. Uddin, MS, Kabir, MT, Jakaria, M, Sobarzo-Sanchez, E, Barreto, GE, Perveen, A, et al. Exploring the potential of Neuroproteomics in Alzheimer's disease. Curr Top Med Chem. (2020) 20:2263–78. doi: 10.2174/1568026620666200603112030
203. Cheng, SX, Xu, ZW, Yi, TL, Sun, HT, Yang, C, Yu, ZQ, et al. iTRAQ-based quantitative proteomics reveals the new evidence base for traumatic brain injury treated with targeted temperature management. Neurotherapeutics. (2018) 15:216–32. doi: 10.1007/s13311-017-0591-2
204. Xu, B, Tian, R, Wang, X, Zhan, S, Wang, R, Guo, Y, et al. Protein profile changes in the frontotemporal lobes in human severe traumatic brain injury. Brain Res. (2016) 1642:344–52. doi: 10.1016/j.brainres.2016.04.008
205. Zhou, D, Liu, J, Hang, Y, Li, T, Li, P, Guo, S, et al. TMT-based proteomics analysis reveals the protective effects of Xuefu Zhuyu decoction in a rat model of traumatic brain injury. J Ethnopharmacol. (2020) 258:112826. doi: 10.1016/j.jep.2020.112826
206. Siman, R, McIntosh, TK, Soltesz, KM, Chen, Z, Neumar, RW, and Roberts, VL. Proteins released from degenerating neurons are surrogate markers for acute brain damage. Neurobiol Dis. (2004) 16:311–20. doi: 10.1016/j.nbd.2004.03.016
207. Burgess, JA, Lescuyer, P, Hainard, A, Burkhard, PR, Turck, N, Michel, P, et al. Identification of brain cell death associated proteins in human post-mortem cerebrospinal fluid. J Proteome Res. (2006) 5:1674–81. doi: 10.1021/pr060160v
208. Yu, C, Boutté, A, Yu, X, Dutta, B, Feala, JD, Schmid, K, et al. A systems biology strategy to identify molecular mechanisms of action and protein indicators of traumatic brain injury. J Neurosci Res. (2015) 93:199–214. doi: 10.1002/jnr.23503
209. Thelin, EP, Just, D, Frostell, A, Häggmark-Månberg, A, Risling, M, Svensson, M, et al. Protein profiling in serum after traumatic brain injury in rats reveals potential injury markers. Behav Brain Res. (2018) 340:71–80. doi: 10.1016/j.bbr.2016.08.058
210. Lazarus, RC, Buonora, JE, Jacobowitz, DM, and Mueller, GP. Protein carbonylation after traumatic brain injury: cell specificity, regional susceptibility, and gender differences. Free Radic Biol Med. (2015) 78:89–100. doi: 10.1016/j.freeradbiomed.2014.10.507
211. Buchberger, AR, DeLaney, K, Johnson, J, and Li, L. Mass spectrometry imaging: a review of emerging advancements and future insights. Anal Chem. (2018) 90:240–65. doi: 10.1021/acs.analchem.7b04733
212. Mallah, K, Zibara, K, Kerbaj, C, Eid, A, Khoshman, N, Ousseily, Z, et al. Neurotrauma investigation through spatial omics guided by mass spectrometry imaging: target identification and clinical applications. Mass Spectrom Rev. (2023) 42:189–205. doi: 10.1002/mas.21719
213. Hu, Y, Wang, Z, Liu, L, Zhu, J, Zhang, D, Xu, M, et al. Mass spectrometry-based chemical mapping and profiling toward molecular understanding of diseases in precision medicine. Chem Sci. (2021) 12:7993–8009. doi: 10.1039/D1SC00271F
214. Schnackenberg, LK, Thorn, DA, Barnette, D, and Jones, EE. MALDI imaging mass spectrometry: an emerging tool in neurology. Metab Brain Dis. (2022) 37:105–21. doi: 10.1007/s11011-021-00797-2
215. Hanrieder, J, Zetterberg, H, and Blennow, K. MALDI imaging mass spectrometry: neurochemical imaging of proteins and peptides. Neuroproteomics. (2019) 2019:179–97. doi: 10.1007/978-1-4939-9662-9_15
216. Vaysse, PM, Heeren, RMA, Porta, T, and Balluff, B. Mass spectrometry imaging for clinical research - latest developments, applications, and current limitations. Analyst. (2017) 142:2690–712. doi: 10.1039/C7AN00565B
217. Hogan, SR, Phan, JH, Alvarado-Velez, M, Wang, MD, Bellamkonda, RV, Fernández, FM, et al. Discovery of Lipidome alterations following traumatic brain injury via high-resolution metabolomics. J Proteome Res. (2018) 17:2131–43. doi: 10.1021/acs.jproteome.8b00068
218. Eberlin, LS, Ferreira, CR, Dill, AL, Ifa, DR, and Cooks, RG. Desorption electrospray ionization mass spectrometry for lipid characterization and biological tissue imaging. Biochim Biophys Acta. (2011) 1811:946–60. doi: 10.1016/j.bbalip.2011.05.006
219. Agüi-Gonzalez, P, Jähne, S, and Phan, NT. SIMS imaging in neurobiology and cell biology. J Anal At Spectrom. (2019) 34:1355–68. doi: 10.1039/C9JA00118B
220. Gonçalves, JPL, Bollwein, C, and Schwamborn, K. Mass spectrometry imaging spatial tissue analysis toward personalized medicine. Life. (2022) 12:1037. doi: 10.3390/life12071037
221. Castellino, S, Lareau, NM, and Groseclose, MR. The emergence of imaging mass spectrometry in drug discovery and development: making a difference by driving decision making. J Mass Spectrom. (2021) 56:e4717. doi: 10.1002/jms.4717
222. Liu, NT, and Salinas, J. Machine learning for predicting outcomes in trauma. Shock. (2017) 48:504–10. doi: 10.1097/SHK.0000000000000898
223. Abujaber, A, Fadlalla, A, Gammoh, D, Abdelrahman, H, Mollazehi, M, and El-Menyar, A. Using trauma registry data to predict prolonged mechanical ventilation in patients with traumatic brain injury: machine learning approach. PLoS One. (2020) 15:e0235231. doi: 10.1371/journal.pone.0235231
224. Chong, SL, Liu, N, Barbier, S, and Ong, ME. Predictive modeling in pediatric traumatic brain injury using machine learning. BMC Med Res Methodol. (2015) 15:22. doi: 10.1186/s12874-015-0015-0
225. Liu, N, Koh, ZX, Chua, EC, Tan, LM, Lin, Z, Mirza, B, et al. Risk scoring for prediction of acute cardiac complications from imbalanced clinical data. IEEE J Biomed Health Inform. (2014) 18:1894–902. doi: 10.1109/JBHI.2014.2303481
226. Raj, R, Luostarinen, T, Pursiainen, E, Posti, JP, Takala, RSK, Bendel, S, et al. Machine learning-based dynamic mortality prediction after traumatic brain injury. Sci Rep. (2019) 9:17672. doi: 10.1038/s41598-019-53889-6
227. Feng, JZ, Wang, Y, Peng, J, Sun, MW, Zeng, J, and Jiang, H. Comparison between logistic regression and machine learning algorithms on survival prediction of traumatic brain injuries. J Crit Care. (2019) 54:110–6. doi: 10.1016/j.jcrc.2019.08.010
228. Gravesteijn, BY, Nieboer, D, Ercole, A, Lingsma, HF, Nelson, D, van Calster, B, et al. Machine learning algorithms performed no better than regression models for prognostication in traumatic brain injury. J Clin Epidemiol. (2020) 122:95–107. doi: 10.1016/j.jclinepi.2020.03.005
229. Thelin, E, Al Nimer, F, Frostell, A, Zetterberg, H, Blennow, K, Nyström, H, et al. A serum protein biomarker panel improves outcome prediction in human traumatic brain injury. J Neurotrauma. (2019) 36:2850–62. doi: 10.1089/neu.2019.6375
230. Rau, CS, Kuo, PJ, Chien, PC, Huang, CY, Hsieh, HY, and Hsieh, CH. Mortality prediction in patients with isolated moderate and severe traumatic brain injury using machine learning models. PLoS One. (2018) 13:e0207192. doi: 10.1371/journal.pone.0207192
231. Vergara, VM, Mayer, AR, Damaraju, E, Kiehl, KA, and Calhoun, V. Detection of mild traumatic brain injury by machine learning classification using resting state functional network connectivity and fractional anisotropy. J Neurotrauma. (2017) 34:1045–53. doi: 10.1089/neu.2016.4526
232. Kayhanian, S, Young, AMH, Mangla, C, Jalloh, I, Fernandes, HM, Garnett, MR, et al. Modelling outcomes after paediatric brain injury with admission laboratory values: a machine-learning approach. Pediatr Res. (2019) 86:641–5. doi: 10.1038/s41390-019-0510-9
233. Matsuo, K, Aihara, H, Nakai, T, Morishita, A, Tohma, Y, and Kohmura, E. Machine learning to predict in-hospital morbidity and mortality after traumatic brain injury. J Neurotrauma. (2020) 37:202–10. doi: 10.1089/neu.2018.6276
234. DiRusso, SM, Sullivan, T, Holly, C, Cuff, SN, and Savino, J. An artificial neural network as a model for prediction of survival in trauma patients: validation for a regional trauma area. J Trauma Acute Care Surg. (2000) 49:212–23. doi: 10.1097/00005373-200008000-00006
235. Hale, AT, Stonko, DP, Brown, A, Lim, J, Voce, DJ, Gannon, SR, et al. Machine-learning analysis outperforms conventional statistical models and CT classification systems in predicting 6-month outcomes in pediatric patients sustaining traumatic brain injury. Neurosurg Focus. (2018) 45:E2. doi: 10.3171/2018.8.FOCUS17773
236. Rashidi, HH, Sen, S, Palmieri, TL, Blackmon, T, Wajda, J, and Tran, NK. Early recognition of burn-and trauma-related acute kidney injury: a pilot comparison of machine learning techniques. Sci Rep. (2020) 10:205. doi: 10.1038/s41598-019-57083-6
237. Fiandaca, MS, Mapstone, M, Mahmoodi, A, Gross, T, Macciardi, F, Cheema, AK, et al. Plasma metabolomic biomarkers accurately classify acute mild traumatic brain injury from controls. PLoS One. (2018) 13:e0195318. doi: 10.1371/journal.pone.0195318
238. Chatzimichail, E, Matthaios, D, Bouros, D, Karakitsos, P, Romanidis, K, Kakolyris, S, et al. γ-H2AX: a novel prognostic marker in a prognosis prediction model of patients with early operable non-small cell lung Cancer. Int J Genomics. (2014) 2014:160236. doi: 10.1155/2014/160236
239. Raju, B, Jumah, F, Ashraf, O, Narayan, V, Gupta, G, Sun, H, et al. Big data, machine learning, and artificial intelligence: a field guide for neurosurgeons. J Neurosurg. (2020) 1:1–11. doi: 10.3171/2020.5.JNS201288
240. Bertolaccini, L, Solli, P, Pardolesi, A, and Pasini, A. An overview of the use of artificial neural networks in lung cancer research. J Thorac Dis. (2017) 9:924–31. doi: 10.21037/jtd.2017.03.157
241. Martinez, BI, and Stabenfeldt, SE. Current trends in biomarker discovery and analysis tools for traumatic brain injury. J Biol Eng. (2019) 13:16. doi: 10.1186/s13036-019-0145-8
242. Nampei, M, Horikawa, M, Ishizu, K, Yamazaki, F, Yamada, H, Kahyo, T, et al. Unsupervised machine learning using an imaging mass spectrometry dataset automatically reassembles grey and white matter. Sci Rep. (2019) 9:13213. doi: 10.1038/s41598-019-49819-1
243. Mallah, K, Quanico, J, Trede, D, Kobeissy, F, Zibara, K, Salzet, M, et al. Lipid changes associated with traumatic brain injury revealed by 3D MALDI-MSI. Anal Chem. (2018) 90:10568–76. doi: 10.1021/acs.analchem.8b02682
244. Han, H, and Liu, W. The coming era of artificial intelligence in biological data science. BMC Bioinformatics. (2019) 20:1–2. doi: 10.1186/s12859-019-3225-3
245. Agoston, DV. Chapter 4 - big data, artificial intelligence, and machine learning in Neurotrauma In: F Kobeissy, A Alawieh, FA Zaraket, and K Wang, editors. Leveraging biomedical and healthcare data. New York: Academic Press (2019). 53–75.
246. Winchester, LM, Harshfield, EL, Shi, L, Badhwar, A, Khleifat, AA, Clarke, N, et al. Artificial intelligence for biomarker discovery in Alzheimer’s disease and dementia. Alzheimer’s Dement. (2023) 2023:13390. doi: 10.1002/alz.13390
247. Gupta, R, Kumari, S, Senapati, A, Ambasta, RK, and Kumar, P. New era of artificial intelligence and machine learning-based detection, diagnosis, and therapeutics in Parkinson’s disease. Ageing Res Rev. (2023) 90:102013. doi: 10.1016/j.arr.2023.102013
248. Raghavendra, U, Acharya, UR, and Adeli, H. Artificial intelligence techniques for automated diagnosis of neurological disorders. Eur Neurol. (2020) 82:41–64. doi: 10.1159/000504292
249. Mikdadi, D, O’Connell, KA, Meacham, PJ, Dugan, MA, Ojiere, MO, Carlson, TB, et al. Applications of artificial intelligence (AI) in ovarian cancer, pancreatic cancer, and image biomarker discovery. Cancer Biomark. (2022) 33:173–84. doi: 10.3233/CBM-210301
250. Verma, RK, Pandey, M, Chawla, P, Choudhury, H, Mayuren, J, Bhattamisra, SK, et al. An insight into the role of artificial intelligence in the early diagnosis of Alzheimer’s disease. CNS Neurol Disord Drug Targets. (2022) 21:901–12. doi: 10.2174/1871527320666210512014505
251. Li, S, Wu, S, Wang, L, Li, F, Jiang, H, and Bai, F. Recent advances in predicting protein–protein interactions with the aid of artificial intelligence algorithms. Curr Opin Struct Biol. (2022) 73:102344. doi: 10.1016/j.sbi.2022.102344
252. Ramanathan, A, Ma, H, Parvatikar, A, and Chennubhotla, SC. Artificial intelligence techniques for integrative structural biology of intrinsically disordered proteins. Curr Opin Struct Biol. (2021) 66:216–24. doi: 10.1016/j.sbi.2020.12.001
253. Jena, B, Saxena, S, Nayak, GK, Balestrieri, A, Gupta, N, Khanna, NN, et al. Brain tumor characterization using radiogenomics in artificial intelligence framework. Cancers. (2022) 14:4052. doi: 10.3390/cancers14164052
254. Bettencourt, C, Skene, N, Bandres-Ciga, S, Anderson, E, Winchester, LM, Foote, IF, et al. Artificial intelligence for dementia genetics and omics. Alzheimer’s Dement. (2023) 2023:13427. doi: 10.1002/alz.13427
255. He, X, Liu, X, Zuo, F, Shi, H, and Jing, J. Artificial intelligence-based multi-omics analysis fuels cancer precision medicine. Semin Cancer Biol. (2023) 88:187–200. doi: 10.1016/j.semcancer.2022.12.009
256. Kaur, P, Singh, A, and Chana, I. Computational techniques and tools for omics data analysis: state-of-the-art, challenges, and future directions. Arch Comput Methods Eng. (2021) 28:4595–631. doi: 10.1007/s11831-021-09547-0
257. Krochmal, M, Kontostathi, G, Magalhães, P, Makridakis, M, Klein, J, Husi, H, et al. Urinary peptidomics analysis reveals proteases involved in diabetic nephropathy. Sci Rep. (2017) 7:15160. doi: 10.1038/s41598-017-15359-9
258. Nice, EC. From proteomics to personalized medicine: the road ahead. Expert Rev Proteomics. (2016) 13:341–3. doi: 10.1586/14789450.2016.1158107
259. Yadikar, H, Johnson, C, Pafundi, N, Mouhawasse, E, Nguyen, L, Torres, I, et al. Novel Peptidomic approach for identification of low and high molecular weight Tauopathy peptides following Calpain digestion, and primary culture neurotoxic challenges. Int J Mol Sci. (2019) 20:5213. doi: 10.3390/ijms20205213
260. Wang, K. Neurotrauma: A comprehensive textbook on traumatic brain injury and spinal cord injury. Oxford: Oxford University Press (2018).
261. Veenstra, TD. Neuroproteomic tools for battling Alzheimer's disease. Proteomics. (2016) 16:2847–53. doi: 10.1002/pmic.201600211
262. Duarte, TT, and Spencer, CT. Personalized proteomics: the future of precision medicine. Proteomes. (2016) 4:29. doi: 10.3390/proteomes4040029
263. Hampel, H, Vergallo, A, Perry, G, Lista, S, and Initiative, APM. The Alzheimer precision medicine initiative. J Alzheimers Dis. (2019) 68:1–24. doi: 10.3233/JAD-181121
264. Reddi, S, Thakker-Varia, S, Alder, J, and Giarratana, AO. Status of precision medicine approaches to traumatic brain injury. Neural Regen Res. (2022) 17:2166–71. doi: 10.4103/1673-5374.335824
265. Figaji, AA, Graham Fieggen, A, Mankahla, N, Enslin, N, and Rohlwink, UK. Targeted treatment in severe traumatic brain injury in the age of precision medicine. Childs Nerv Syst. (2017) 33:1651–61. doi: 10.1007/s00381-017-3562-3
266. Opii, WO, Nukala, VN, Sultana, R, Pandya, JD, Day, KM, Merchant, ML, et al. Proteomic identification of oxidized mitochondrial proteins following experimental traumatic brain injury. J Neurotrauma. (2007) 24:772–89. doi: 10.1089/neu.2006.0229
267. Zhang, P, Zhu, S, Li, Y, Zhao, M, Liu, M, Gao, J, et al. Quantitative proteomics analysis to identify diffuse axonal injury biomarkers in rats using iTRAQ coupled LC-MS/MS. J Proteome. (2016) 133:93–9. doi: 10.1016/j.jprot.2015.12.014
268. Attilio, PJ, Flora, M, Kamnaksh, A, Bradshaw, DJ, Agoston, D, and Mueller, GP. The effects of blast exposure on protein Deimination in the brain. Oxidative Med Cell Longev. (2017) 2017:8398072. doi: 10.1155/2017/8398072
269. Chen, M, Song, H, Cui, J, Johnson, CE, Hubler, GK, DePalma, RG, et al. Proteomic profiling of mouse brains exposed to blast-induced mild traumatic brain injury reveals changes in axonal proteins and phosphorylated tau. J Alzheimers Dis. (2018) 66:751–73. doi: 10.3233/JAD-180726
270. Wright, DK, Brady, RD, Kamnaksh, A, Trezise, J, Sun, M, McDonald, SJ, et al. Repeated mild traumatic brain injuries induce persistent changes in plasma protein and magnetic resonance imaging biomarkers in the rat. Sci Rep. (2019) 9:14626. doi: 10.1038/s41598-019-51267-w
271. Song, H, Chen, M, Chen, C, Cui, J, Johnson, CE, Cheng, J, et al. Proteomic analysis and biochemical correlates of mitochondrial dysfunction after low-intensity primary blast exposure. J Neurotrauma. (2019) 36:1591–605. doi: 10.1089/neu.2018.6114
272. Kondo, T, and Hirohashi, S. Application of 2D-DIGE in cancer proteomics toward personalized medicine. Methods Mol Biol. (2009) 577:135–54. doi: 10.1007/978-1-60761-232-2_11
273. Kwon, HJ, Fehniger, TE, and Marko-Varga, G. Building the basis for proteomics in personalized medicine for targeted treatment. Clin Transl Med. (2016) 5:19. doi: 10.1186/s40169-016-0096-3
274. Brophy, GM, Mondello, S, Papa, L, Robicsek, SA, Gabrielli, A, Tepas, J 3rd, et al. Biokinetic analysis of ubiquitin C-terminal hydrolase-L1 (UCH-L1) in severe traumatic brain injury patient biofluids. J Neurotrauma. (2011) 28:861–70. doi: 10.1089/neu.2010.1564
275. Fraser, DD, Close, TE, Rose, KL, Ward, R, Mehl, M, Farrell, C, et al. Severe traumatic brain injury in children elevates glial fibrillary acidic protein in cerebrospinal fluid and serum. Pediatr Crit Care Med. (2011) 12:319–24. doi: 10.1097/PCC.0b013e3181e8b32d
276. Goyal, A, Failla, MD, Niyonkuru, C, Amin, K, Fabio, A, Berger, RP, et al. S100b as a prognostic biomarker in outcome prediction for patients with severe traumatic brain injury. J Neurotrauma. (2013) 30:946–57. doi: 10.1089/neu.2012.2579
277. Lo, TY, Jones, PA, and Minns, RA. Pediatric brain trauma outcome prediction using paired serum levels of inflammatory mediators and brain-specific proteins. J Neurotrauma. (2009) 26:1479–87. doi: 10.1089/neu.2008.0753
278. McCrea, M, Broglio, SP, McAllister, TW, Gill, J, Giza, CC, Huber, DL, et al. Association of Blood Biomarkers with Acute Sport-Related Concussion in collegiate athletes: findings from the NCAA and Department of Defense CARE consortium. JAMA Netw Open. (2020) 3:e1919771. doi: 10.1001/jamanetworkopen.2019.19771
279. Rubenstein, R, Chang, B, Yue, JK, Chiu, A, Winkler, EA, Puccio, AM, et al. Comparing plasma Phospho tau, Total tau, and Phospho tau-Total tau ratio as acute and chronic traumatic brain injury biomarkers. JAMA Neurol. (2017) 74:1063–72. doi: 10.1001/jamaneurol.2017.0655
280. Thomann, PA, Kaiser, E, Schönknecht, P, Pantel, J, Essig, M, and Schröder, J. Association of total tau and phosphorylated tau 181 protein levels in cerebrospinal fluid with cerebral atrophy in mild cognitive impairment and Alzheimer disease. J Psychiatry Neurosci. (2009) 34:136–42.
281. Vina, J, and Lloret, A. Why women have more Alzheimer's disease than men: gender and mitochondrial toxicity of amyloid-β peptide. J Alzheimers Dis. (2010) 20:S527–33. doi: 10.3233/JAD-2010-100501
282. Wang, KK, Yang, Z, Yue, JK, Zhang, Z, Winkler, EA, Puccio, AM, et al. Plasma anti-glial fibrillary acidic protein autoantibody levels during the acute and chronic phases of traumatic brain injury: a transforming research and clinical knowledge in traumatic brain injury pilot study. J Neurotrauma. (2016) 33:1270–7. doi: 10.1089/neu.2015.3881
283. Yadikar, H, Sarkis, GA, Kurup, M, Kobeissy, F, and Wang, KK. Peptidomics and traumatic brain injury: Biomarker utilities for a theragnostic approach In: H Yadikar, editor. Biomarkers for Traumatic Brain Injury. Amsterdam: Elsevier (2020). 419–30.
284. Muralidharan, R. External ventricular drains: management and complications. Surg Neurol Int. (2015) 6:S271–4. doi: 10.4103/2152-7806.157620
285. Yang, Z, Zhu, T, Mondello, S, Akel, M, Wong, AT, Kothari, IM, et al. Serum-based Phospho-Neurofilament-heavy protein as Theranostic biomarker in three models of traumatic brain injury: an operation brain trauma therapy study. J Neurotrauma. (2019) 36:348–59. doi: 10.1089/neu.2017.5586
286. Niyonkuru, C, Wagner, AK, Ozawa, H, Amin, K, Goyal, A, and Fabio, A. Group-based trajectory analysis applications for prognostic biomarker model development in severe TBI: a practical example. J Neurotrauma. (2013) 30:938–45. doi: 10.1089/neu.2012.2578
287. Shoemaker, LD, Achrol, AS, Sethu, P, Steinberg, GK, and Chang, SD. Clinical neuroproteomics and biomarkers: from basic research to clinical decision making. Neurosurgery. (2012) 70:518–25. doi: 10.1227/NEU.0b013e3182333a26
288. Wang, KK, Yang, Z, Zhu, T, Shi, Y, Rubenstein, R, Tyndall, JA, et al. An update on diagnostic and prognostic biomarkers for traumatic brain injury. Expert Rev Mol Diagn. (2018) 18:165–80. doi: 10.1080/14737159.2018.1428089
289. Tomar, GS, Singh, GP, Lahkar, D, Sengar, K, Nigam, R, Mohan, M, et al. New biomarkers in brain trauma. Clin Chim Acta. (2018) 487:325–9. doi: 10.1016/j.cca.2018.10.025
290. Bayés, A, and Grant, SG. Neuroproteomics: understanding the molecular organization and complexity of the brain. Nat Rev Neurosci. (2009) 10:635–46. doi: 10.1038/nrn2701
291. Jain, KK, and Jain, K. Personalized therapy of neurological disorders In: KK Jain, editor. Textbook of Personalized Medicine. Berlin: Springer (2021). 213–62.
292. Kochanek, PM, Bramlett, HM, Shear, DA, Dixon, CE, Mondello, S, Dietrich, WD, et al. Synthesis of findings, current investigations, and future directions: operation brain trauma therapy. J Neurotrauma. (2016) 33:606–14. doi: 10.1089/neu.2015.4133
293. Davenport, T, and Kalakota, R. The potential for artificial intelligence in healthcare. Future Healthcare J. (2019) 6:94–8. doi: 10.7861/futurehosp.6-2-94
294. Johnson, KB, Wei, WQ, Weeraratne, D, Frisse, ME, Misulis, K, Rhee, K, et al. Precision medicine, AI, and the future of personalized health care. Clin Transl Sci. (2021) 14:86–93. doi: 10.1111/cts.12884
295. Sebastiani, M, Vacchi, C, Manfredi, A, and Cassone, G. Personalized medicine and machine learning: a roadmap for the future. J Clin Med. (2022) 11:4110. doi: 10.3390/jcm11144110
296. Allami, RH, and Yousif, MG. Integrative AI-driven strategies for advancing precision medicine in infectious diseases and beyond: a novel multidisciplinary approach. ArXiv. (2023) 2023:15228. doi: 10.48550/arXiv.2307.15228
297. Sundaravadhanan, S. Neurotrauma: A Futuristic Perspective. Indian J Neurotrauma. (2018) 15:78–81. doi: 10.1055/s-0039-1694297
298. Ramudu, K, Mohan, VM, Jyothirmai, D, Prasad, D, Agrawal, R, and Boopathi, S. Machine learning and artificial intelligence in disease prediction: Applications, challenges, limitations, case studies, and future directions. Contemporary Applications of Data Fusion for Advanced Healthcare Informatics, IGI Global, pp. 297–318. (2023).
299. Lozano, D, Gonzales-Portillo, GS, Acosta, S, de la Pena, I, Tajiri, N, Kaneko, Y, et al. Neuroinflammatory responses to traumatic brain injury: etiology, clinical consequences, and therapeutic opportunities. Neuropsychiatr Dis Treat. (2015) 11:97–106. doi: 10.2147/NDT.S65815
300. Ferrer, I, Andrés-Benito, P, Zelaya, MV, Aguirre, MEE, Carmona, M, Ausín, K, et al. Familial globular glial tauopathy linked to MAPT mutations: molecular neuropathology and seeding capacity of a prototypical mixed neuronal and glial tauopathy. Acta Neuropathol. (2020) 139:735–71. doi: 10.1007/s00401-019-02122-9
301. Azar, S, Hasan, A, Younes, R, Najdi, F, Baki, L, Ghazale, H, et al. Biofluid proteomics and biomarkers in traumatic brain injury. Methods Mol Biol. (2017) 1598:45–63. doi: 10.1007/978-1-4939-6952-4_3
302. Jaber, Z, Aouad, P, Al Medawar, M, Bahmad, H, Abou-Abbass, H, Ghandour, H, et al. Role of systems biology in brain injury biomarker discovery: Neuroproteomics application. Methods Mol Biol. (2016) 1462:157–74. doi: 10.1007/978-1-4939-3816-2_10
303. Mondello, S, Muller, U, Jeromin, A, Streeter, J, Hayes, RL, and Wang, KK. Blood-based diagnostics of traumatic brain injuries. Expert Rev Mol Diagn. (2011) 11:65–78. doi: 10.1586/erm.10.104
304. Czeiter, E, Amrein, K, Gravesteijn, BY, Lecky, F, Menon, DK, Mondello, S, et al. Blood biomarkers on admission in acute traumatic brain injury: relations to severity, CT findings and care path in the CENTER-TBI study. EBioMedicine. (2020) 56:102785. doi: 10.1016/j.ebiom.2020.102785
305. Mondello, S, Sorinola, A, Czeiter, E, Vámos, Z, Amrein, K, Synnot, A, et al. Blood-based protein biomarkers for the Management of Traumatic Brain Injuries in adults presenting to emergency departments with mild brain injury: a living systematic review and Meta-analysis. J Neurotrauma. (2021) 38:1086–106. doi: 10.1089/neu.2017.5182
306. Wang, KK, Munoz Pareja, JC, Mondello, S, Diaz-Arrastia, R, Wellington, C, Kenney, K, et al. Blood-based traumatic brain injury biomarkers - clinical utilities and regulatory pathways in the United States, Europe and Canada. Expert Rev Mol Diagn. (2021) 21:1303–21. doi: 10.1080/14737159.2021.2005583
Keywords: proteomics, neuroproteomics, neurotrauma, personalized medicine (PM), traumatic brain injuries (TBI), artificial intelligence (AI), machine learning (ML)
Citation: Kobeissy F, Goli M, Yadikar H, Shakkour Z, Kurup M, Haidar MA, Alroumi S, Mondello S, Wang KK and Mechref Y (2023) Advances in neuroproteomics for neurotrauma: unraveling insights for personalized medicine and future prospects. Front. Neurol. 14:1288740. doi: 10.3389/fneur.2023.1288740
Edited by:
Fang Bian, The University of Texas at Dallas, United StatesReviewed by:
Andrew K. Ottens, Virginia Commonwealth University, United StatesHailong Song, University of Pennsylvania, United States
Copyright © 2023 Kobeissy, Goli, Yadikar, Shakkour, Kurup, Haidar, Alroumi, Mondello, Wang and Mechref. This is an open-access article distributed under the terms of the Creative Commons Attribution License (CC BY). The use, distribution or reproduction in other forums is permitted, provided the original author(s) and the copyright owner(s) are credited and that the original publication in this journal is cited, in accordance with accepted academic practice. No use, distribution or reproduction is permitted which does not comply with these terms.
*Correspondence: Yehia Mechref, Yehia.Mechref@ttu.edu
†These authors have contributed equally to this work