- 1Department of Respiratory and Critical Care Medicine, The First Affiliated Hospital of Wenzhou Medical University, Wenzhou, China
- 2Department of Neurosurgery, The First Affiliated Hospital of Wenzhou Medical University, Wenzhou, China
Background: Postoperative pneumonia (POP) is a common complication after aneurysmal subarachnoid hemorrhage (aSAH) associated with increased mortality rates, prolonged hospitalization, and high medical costs. It is currently understood that identifying pneumonia early and implementing aggressive treatment can significantly improve patients' outcomes. The primary objective of this study was to explore risk factors and develop a logistic regression model that assesses the risks of POP.
Methods: An internal cohort of 613 inpatients with aSAH who underwent surgery at the Neurosurgical Department of First Affiliated Hospital of Wenzhou Medical University was retrospectively analyzed to develop a nomogram for predicting POP. We assessed the discriminative power, accuracy, and clinical validity of the predictions by using the area under the receiver operating characteristic curve (AUC), the calibration curve, and decision curve analysis (DCA). The final model was validated using an external validation set of 97 samples from the Medical Information Mart for Intensive Care IV (MIMIC-IV) database.
Results: Among patients in our internal cohort, 15.66% (n = 96/613) of patients had POP. The least absolute shrinkage and selection operator (LASSO) regression analysis identified the Glasgow Coma Scale (GCS), mechanical ventilation time (MVT), albumin, C-reactive protein (CRP), smoking, and delayed cerebral ischemia (DCI) as potential predictors of POP. We then used multivariable logistic regression analysis to evaluate the effects of these predictors and create a final model. Eighty percentage of patients in the internal cohort were randomly assigned to the training set for model development, while the remaining 20% of patients were allocated to the internal validation set. The AUC values for the training, internal, and external validation sets were 0.914, 0.856, and 0.851, and the corresponding Brier scores were 0.084, 0.098, and 0.143, respectively.
Conclusion: We found that GCS, MVT, albumin, CRP, smoking, and DCI are independent predictors for the development of POP in patients with aSAH. Overall, our nomogram represents a reliable and convenient approach to predict POP in the patient population.
Introduction
According to the Global Burden of Diseases, Injuries, and Risk Factors Study (GBD), the annual number of strokes and deaths due to stroke increased substantially from 1990 to 2019 (1). Following the methodology in the GBD, in 2019, there were 3.94 million (95% uncertainty interval 3.43–4.58) new stroke cases in China. The incidence rate of stroke increased by 86.0% (73.2–99.0) from 1990, reaching 276.7 (241.3–322.0) per 100,000 population in 2019 (2). In addition, since 2015, stroke has become the leading cause of death and disability in China, posing a significant threat to the health of its citizens as a major chronic non-communicable disease (3). Therefore, stroke-related research is very much needed. Aneurysmal subarachnoid hemorrhage (aSAH) is a serious condition frequently caused by intracranial aneurysm rupture and is associated with high rates of morbidity and mortality (4). The incidence of aSAH is estimated to be ~9.1 cases per 100,000 individuals, accounting for ~5% of all strokes (5, 6). Although the advent of endovascular coil embolization and microsurgery represents significant improvements in treating aSAH patients, postoperative complications remain challenging, affecting patient prognosis and increasing hospitalization costs. Postoperative pneumonia (POP) is a common complication affecting 13.2–29.15% of aSAH patients and is associated with prognosis (7–11). Therefore, this research sought to identify early risk factors for POP, which can help identify high-risk patients who may benefit from proactive monitoring and therapeutic interventions. Previous studies have shown that increased incidence of POP in aSAH patients was associated with factors such as high preoperative inflammatory markers, high levels of lactate dehydrogenase (LDH), the use of mechanical ventilation (MV), and high World Federation of Neurosurgical Societies (WFNS) grade (8, 12). While several risk factors for POP have been identified, predicting its occurrence in patients with aSAH remains challenging. To address this issue, one potential solution is to construct a mathematical model for predicting the probability of occurrence of POP. A nomogram, a graphical representation of regression models, can simplify risk assessment, map the probability of events to individual patients, and enhance clinical decision-making for medical staff and patients (13). This retrospective study aimed to identify the preoperative factors associated with POP and develop a nomogram based on perioperative information to predict the likelihood of POP, providing clinicians with a useful tool for preventing and treating this patient population.
Materials and methods
Data source
This retrospective study consisted of two cohorts: an internal cohort (n = 613) and an external cohort (n = 97). A total of 613 adult patients from the internal cohort were newly diagnosed with aSAH between 1 January 2019 and 5 February 2022 at the First Affiliated Hospital of Wenzhou Medical University. The study samples and treatment information were obtained from the database of the neurosurgical departments. The blood laboratory examination was collected within 24 h after patient's admission and before the surgery. The external cohort (n = 97) was extracted from the Medical Information Mart for Intensive Care IV (MIMIC-IV) (version 2.2) database, derived from a large, freely accessible critical care database comprising 299,712 patients who were admitted to the ICU or the emergency department of Beth Israel Deaconess Medical Center between 2008 and 2019 (https://mimic.physionet.org/) (14, 15).
Patient enrollment
Patients enrolled in the internal cohort met the following requirements: (1) aged 18 years or older, experiencing their first-ever subarachnoid hemorrhage, and admitted to the hospital within 24 h of symptoms onset; (2) received confirmation of aSAH through computed tomography (CT), computed tomographic angiography (CTA), or digital subtraction angiography (DSA); and (3) underwent embolization of intracranial aneurysms. Some of them also received intracranial stenting and/or craniotomy. Among the patients that met the above conditions (n = 763), 150 were excluded for the following reasons: (1) the presence of other potential causes of SAH [arteriovenous malformation (n = 90), craniocerebral trauma (n = 3), and hypertensive intracerebral hemorrhage (n = 2)]; (2) having a history of malignant tumors, severe heart, hepatic, or renal failure (n = 10); (3) previous use of antibiotics, systemic glucocorticoids, immunosuppressive agents, or immunotherapy within 1 month before admission (n = 3); (4) diagnosed with pneumonia according to the modified Centers for Disease Control and Prevention (CDC) criteria before admission (n = 30) (9, 16); and (5) died within 24 h after surgery or incomplete records (n = 12). Finally, 613 patients were included in the internal cohort, with 80% randomly assigned to the training set for model development. The remaining 20% constituted the validation set that was used to evaluate the nomogram model.
In the external cohort, the inclusion criteria were as follows: (1) patients aged 18 years or older and (2) those whose diagnosis matches the International Classification of Diseases (ICD) code associated with aSAH among MIMIC-IV (ICD-9 code 430, and ICD-10 codes I60 to I609). A total of 1,172 patients met the above conditions. The exclusion criteria were as follows: (1) non-first admission (n = 33); (2) a history of malignant tumors, severe heart, hepatic, or renal failure (n = 120); (3) length of stay of < 24 h (n = 84); and (4) patients lacking clinical data (n = 838). Finally, 97 patients were assigned to the external validation set to validate the nomogram. Figure 1 shows the detailed flowchart.
According to the modified CDC criteria (16), POP was defined as the set of lower respiratory tract infections that occur within 30 days after an operative procedure, which pertains to the following: (1) A probable POP could not be diagnosed based on the admission or the follow-up chest x-ray, and it could neither be explained by another diagnosis and (2) proven POP had a confirmed change in diagnosis on at least one image of the chest x-ray. Patients with probable/proven pneumonia were considered cases for this study by the modified CDC criteria (17, 18), whereas all patients with pneumonia before admission were excluded.
Variable selection and nomogram construction
In this study, various clinical data were collected on the internal cohort, including age, gender, smoking, drinking, aneurysm location [anterior communicating artery (ACoA), internal carotid artery (ICA), middle cerebral artery (MCA), posterior communicating artery (PCoA), vertebrobasilar artery (VBA)], the Hunt and Hess Scale grades, the modified Fisher Scale (mFS), the Glasgow Coma Scale (GCS), hypertension, chronic obstructive pulmonary disease (COPD), coronary heart disease (CHD), diabetes, delayed cerebral ischemia (DCI), deep vein thrombosis (DVT), POP, intracranial stenting, with craniotomy, mechanical ventilation time (MVT), neutrophil count, monocyte count, lymphocyte count, hemoglobin, blood platelet count, mean corpuscular volume (MCV), albumin, glucose, triglyceride, total cholesterol, uric acid, D-dimer, and C-reactive protein (CRP). The optimal predictive variables were selected using the least absolute shrinkage and selection operator (LASSO) regression. Multivariable logistic regression analysis was then generated using selected predictors from LASSO analysis to develop a nomogram for predicting the risks of POP in aSAH patients.
The nomogram's predictive performance was validated using an external cohort of patients from the MIMIC-IV database. The data of the external cohort included POP and six final predictors: GCS, MVT, albumin, CRP, smoking, and DIC.
Statistical analysis
In this study, continuous variables were described in terms of mean ± SD or median with the interquartile range (IQR), while categorical variables were expressed as frequency and percentage. A LASSO regression model was used to select the optimal predictive variables to address collinearity among the candidate variables (19). Predictors selected from the LASSO analysis were used to generate a multivariate logistic regression analysis, where the features were represented by odds ratio (OR) and 95% confidence intervals (CIs). The receiver operating characteristic curve (ROC) was plotted, and the area under the ROC curve (AUC) was determined to evaluate the discrimination of the nomogram. Decision curve analysis (DCA) was used to determine the nomogram's clinical validity and net benefit (20). The calibration of the nomogram model was evaluated using the Brier score and the calibration curve, with 1,000 bootstrap resamples employed. A two-sided P-value of < 0.05 was considered to be statistically significant.
Results
Population characteristics
Table 1 shows the clinical characteristics of the study population derived from the internal cohort. A total of 613 patients were included in this study and divided into a training set (n = 490) and an internal validation set (n = 123). The overall incidence of POP in the internal cohort was ~15.66%.
Table 2 shows the characteristics of six final predictors and POP in the internal and external cohorts. The overall incidence of POP in the external cohort was ~20.62%, which is similar to the internal cohort.
Table 3 shows the length of stay of aSAH patients with POP and non-POP in the internal cohort. The median length of hospital stay in patients with POP was 20 days, which was significantly higher than the 13 days in patients with non-POP (P < 0.001).
Selected predictors
Out of 34 independent variables, 6 potential predictors were selected by using LASSO regression analysis in the training set (Figure 2), including GCS, MVT, albumin, CRP, smoking, and DIC. A final model was created using multivariable logistic regression analysis based on six predictors screened from LASSO regression analysis (Table 4). In multivariable logistic regression, the influence of these six independent variables on the dependent variable POP was statistically significant (P < 0.05).
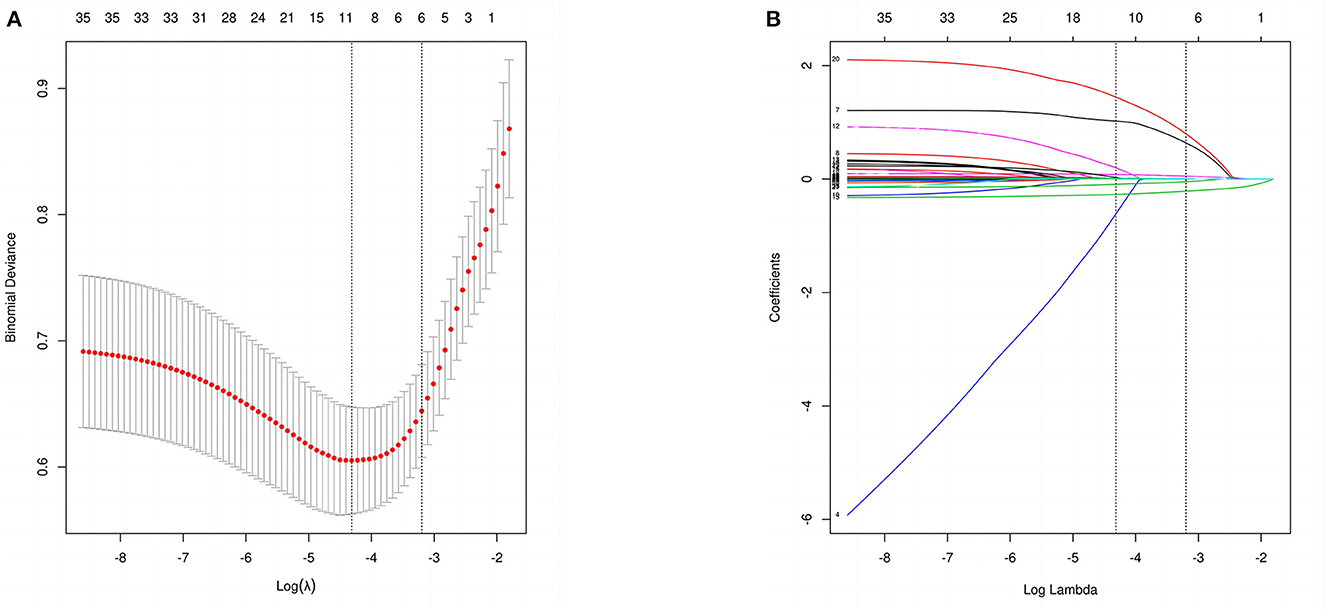
Figure 2. Perioperative variable selection using a LASSO logistic regression model. (A) The minimum criteria (lambda.min) and 1 SE of the minimum criteria (lambda.1se) were used to depict the optimal values with dotted vertical lines. (B) LASSO coefficient profile of 34 variables. The coefficient profile is plotted according to the logarithmic sequence. To determine the optimal predictors of the model, five-fold cross-validation with minimum criteria was used, resulting in six features with nonzero coefficients.
Construction and validation of the nomogram
The nomogram presented in Figure 3 could predict the likelihood of POP in patients with aSAH. The model considers several factors, with GCS having the greatest effect on the development of POP, followed by MVT, albumin, CRP, smoking, and DCI. The total score of these independent prognostic factors was positively correlated with the patient's risk of POP.
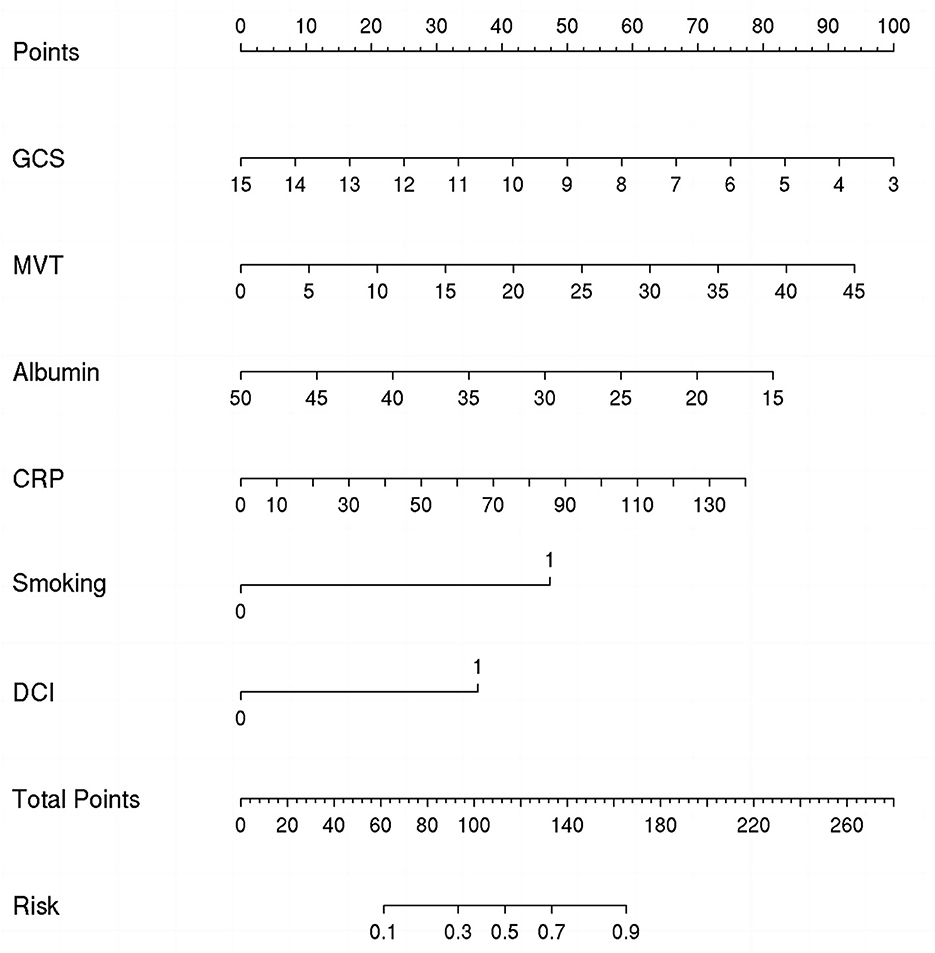
Figure 3. Nomogram for predicting the risk of postoperative pneumonia (POP). Points were assigned for Glasgow Coma Scale (GCS), mechanical ventilation time (MVT), albumin, C-reactive protein (CRP), smoking, and delayed cerebral ischemia (DCI). The total score obtained by adding up the scores of all individual variables is used to find the appropriate position on the “Risk of POP” axis to determine the patient's individual risk of POP.
ROC curve analysis was conducted to evaluate the nomogram model's discrimination performance. The AUC value was 0.914 (95% CI: 0.887–0.942, Figure 4A) in the training set and 0.856 (95% CI: 0.776–0.936, Figure 4B) in the internal validation set. We further assessed the model's performance using an external validation set which yielded an AUC of 0.851 (95% CI: 0.751–0.952, Figure 4C), indicating the good discrimination performance of the nomogram.
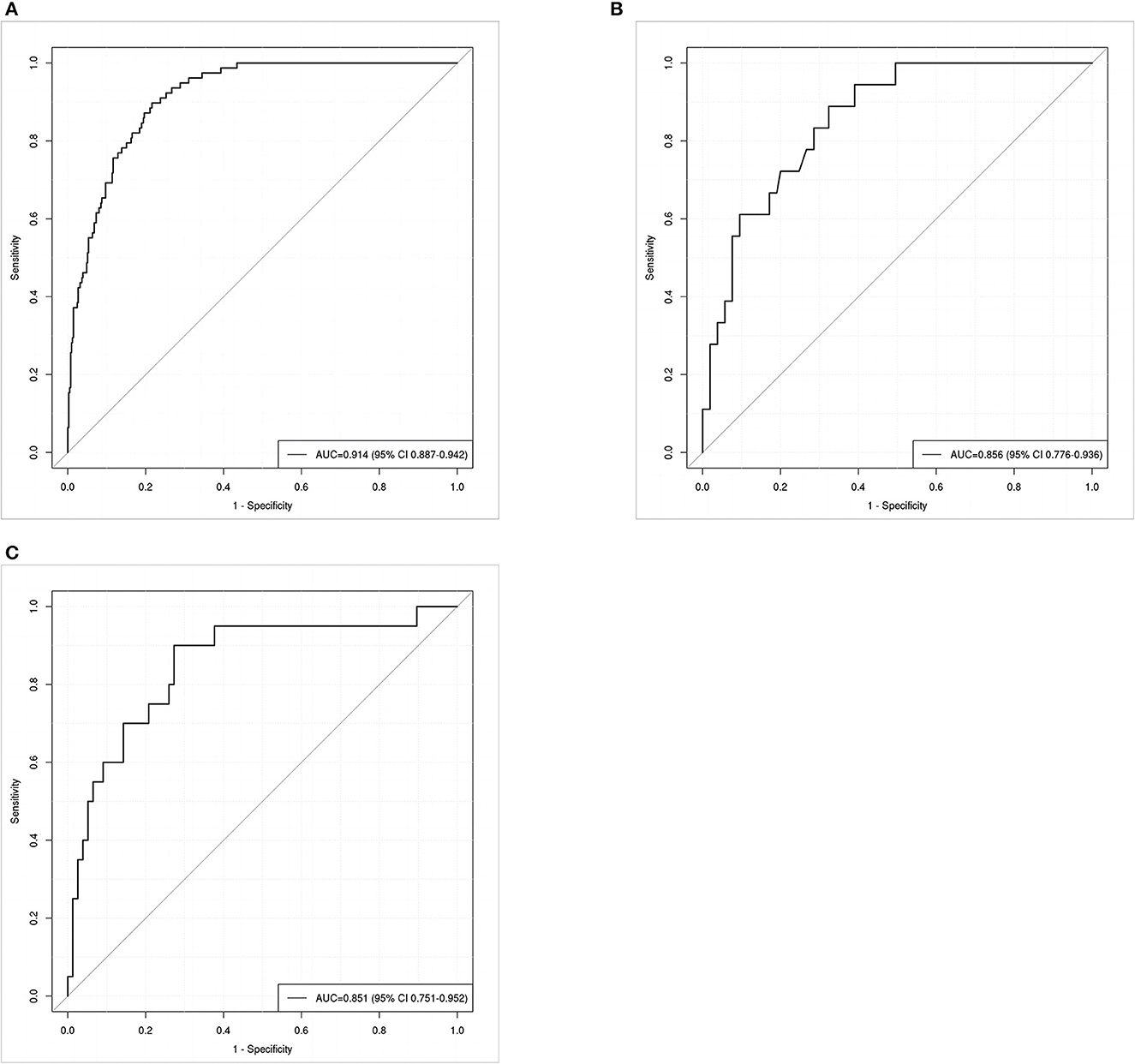
Figure 4. Receiver operating characteristic (ROC) curves for predicting postoperative pneumonia in aneurysmal subarachnoid hemorrhage patients in the training set (A), internal validation set (B), and external validation set (C). The area under the receiver operating characteristic curve (AUC) of the training set (A), internal validation set (B), and external validation set (C) was 0.914, 0.856, and 0.851, respectively.
A calibration plot was constructed by using 1,000 bootstrap resamples to assess the nomogram's calibration performance. The results showed an optimal agreement between the predicted and observed outcomes in the training set (Figure 5A), the internal validation set (Figure 5B), and the external validation set (Figure 5C). The mean absolute error values for the three calibration plots were 0.039, 0.032, and 0.046, respectively. To quantify the performance of calibration, we utilized the Brier score. The results showed that the Brier scores were 0.084 in the training set, 0.098 in the internal validation set, and 0.143 in the external validation set. Additionally, we conducted a DCA to evaluate the clinical benefit of the nomogram. As illustrated in Figures 6A–C, decision curve analyses of the training set, the internal validation set, and the external validation set demonstrated a net clinical benefit.
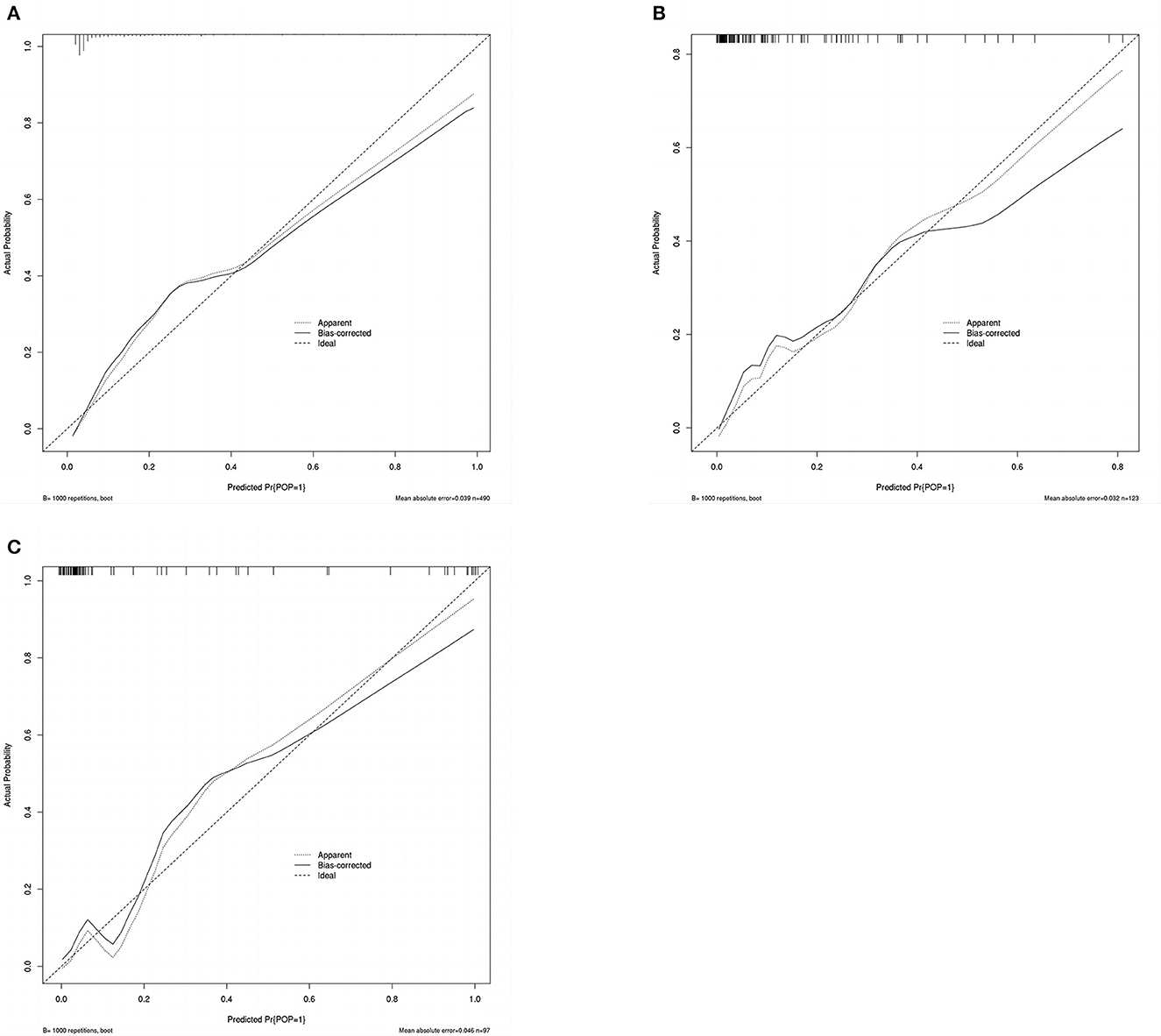
Figure 5. Calibration plot of the nomogram in the training set (A), internal validation set (B), and external validation set (C). Predictions generated from the model are plotted against actual patient outcomes. The 45-degree line represents the perfect model calibration. The dotted line (apparent) indicates calibration when the model is applied to each set, and the solid line (bias-corrected) indicates calibration when the model is applied to the bootstrap set.
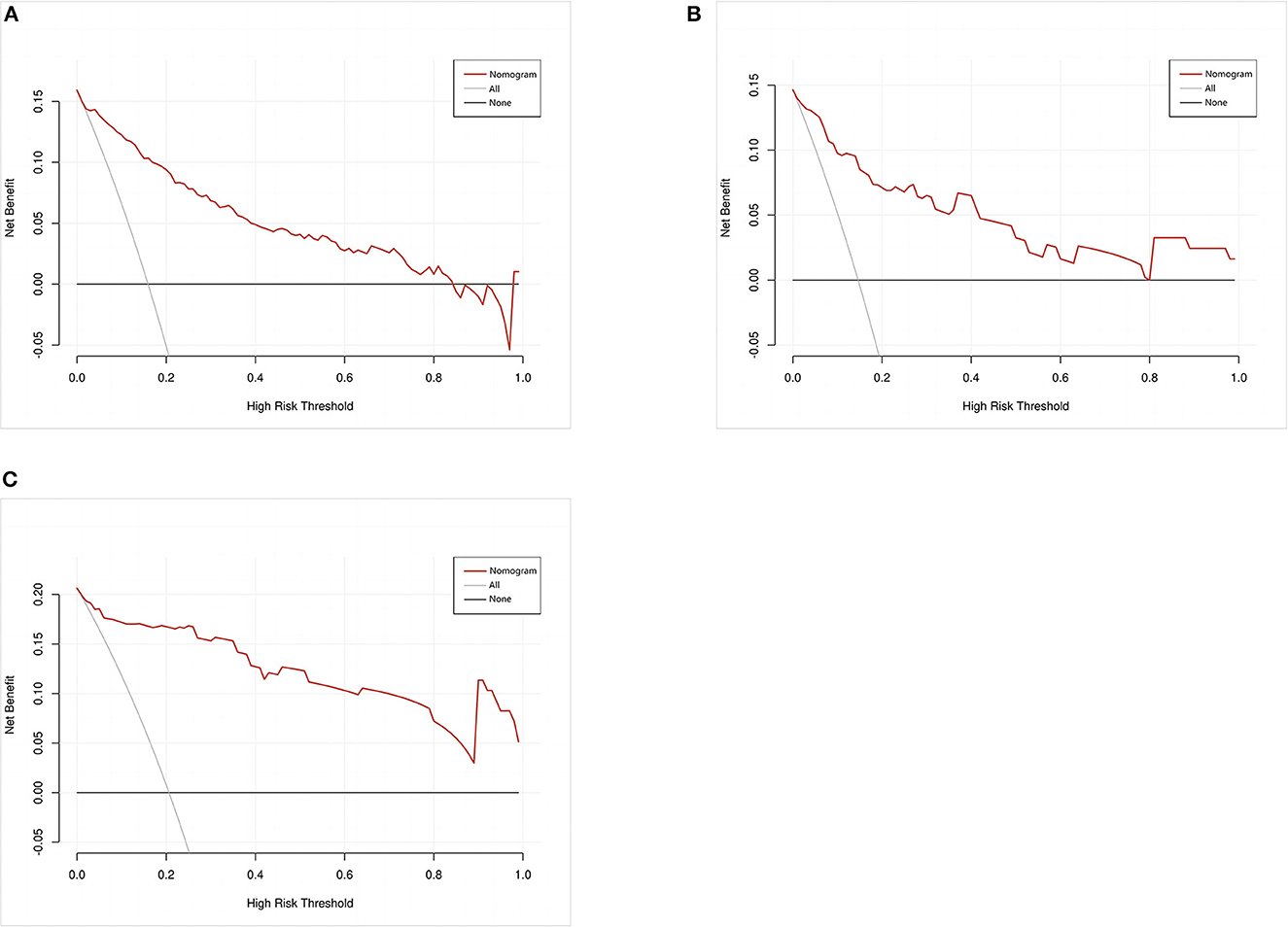
Figure 6. Decision curve analysis of the nomogram in the training set (A), internal validation set (B), and external validation set (C). The red line displays the net benefit of our model. The gray line assumes that all patients develop postoperative pneumonia (POP). The black line assumes that no patients develop POP.
Discussion
In this observational study, GCS, MVT, albumin, CRP, smoking, and DCI were identified as independent predictors of POP in patients with aSAH. Using these six variables, we constructed a nomogram to assess the risk of POP in this patient population. During internal validation, we successfully demonstrated that the model exhibited both discriminative and well-calibrated performance. Although there may be differences between the internal database and the external database due to race, time of admission and discharge of patients, and confounding factors, external validation confirmed its satisfactory accuracy and generalizability.
This study analyzed the incidence of POP in patients with aSAH from the internal cohort and found it to be ~5.66% lower than that reported in most previous cases (7–11, 21), attributed to the exclusion of patients with pneumonia before operation and advancements in surgical techniques and perioperative care.
Our study found that GCS had the greatest impact on the development of POP according to the nomogram. Patients who developed POP had significantly lower GCS scores compared to those who did not develop POP (P < 0.01), which could be attributed to lower GCS scores being associated with decreased consciousness, limb movement disorders, and prolonged bedridden periods, which can result in stroke-associated pneumonia (SAP) (22). Additionally, GCS is a reliable indicator of unfavorable neurological outcomes (23).
Herein, we found that MVT was an independent risk factor for POP (P = 0.001, OR = 1.108, 95% CI: 1.044–1.079). Previous research has demonstrated that prompt extubation could significantly decrease the likelihood of POP. A study conducted by Vera Urquiza et al. (21) discovered that extubation after 6 h was an independent risk factor for pneumonia following cardiac surgery (P = 0.005, OR = 15.81, 95% CI: 2.2–110.7). In a study by Savardekar et al. (11), it was found that tracheal intubation lasting over 48 h increased the risk of POP in patients undergoing microsurgical clipping of ruptured intracranial aneurysms (P = 0.041, OR = 6.638, 95% CI: 1.08–40.8) likely due to the association between long-term intubation and ventilator-associated pneumonia (24). Therefore, daily sedation interruption and assessment of readiness to extubate can reduce the risk of ventilator-associated pneumonia and prevent pneumonia in these patients.
Consistent with the literature, albumin was a major predictor for POP in aSAH patients (9). Furthermore, other studies have demonstrated that albumin therapy can improve organ function and reduce complications in aSAH patients, potentially leading to better outcomes (25–27). This finding may be attributed to the fact that low levels of albumin in the blood can cause a decrease in plasma osmotic pressure, leading to pulmonary interstitial edema. Additionally, hypoalbuminemia can weaken the immune system of patients, increasing their susceptibility to pulmonary infections (28).
CRP is a well-known biomarker of systemic inflammation (29). An increasing body of evidence suggests that CRP can predict various postoperative infections (30–32). Our study also indicated that monitoring serum CRP levels can be useful in predicting POP in patients with aSAH.
Moreover, we found that smoking was identified as an independent risk factor for POP in aSAH patients. Similarly, it is reportedly a risk factor for pneumonia after lung cancer surgery (33), attributed to the inhibitory effect of smoking on the innate immune system. Previous reviews have suggested that quitting smoking after early-stage lung cancer can improve the prognosis (34). Therefore, quitting smoking may also provide benefits for aSAH patients.
Our study found that DCI was independently associated with the development of POP in patients with aSAH. However, further investigation into the temporal course of DCI and its relationship with the development of POP is necessary to establish causation. It is highly conceivable that causation is not a linear process but rather a result of intricate interactions between dynamic systems (35). On the one hand, infection is a known cause of systemic inflammation, which can lead to an increase in platelets (thrombocytosis) (36), white blood cells (leukocytosis), and the release of inflammatory cytokines—specifically interleukin-6 (37). All these factors have been linked to the development of DCI (38–41). On the other hand, DCI has been associated with lower levels of consciousness, low GCS score, and prolonged bedridden periods, which leads to stroke-associated pneumonia (SAP) (22).
Several limitations of this study should be acknowledged. First, the study's retrospective nature introduces the possibility of confounding factors influencing the results. Although the findings have been validated in another database, conducting a large prospective controlled trial and validating several different external datasets are crucial to validate the reported results. Second, several risk factors reported by previous studies were not involved in this study, such as WFNS grade (12) and high LDH (8). Third, patients were not followed up after discharge, indicating the need for longer-term studies to validate our findings. Fourth, in external cohort inclusion, it is difficult to find out whether patients have undergone surgery due to the lack of data. Therefore, the operation was not taken as the inclusion criteria for enrolling an external cohort. Furthermore, due to the limited information in the external database, we cannot exclude the external cohort using exactly the same criteria as the internal cohort. Not applying the same inclusion and exclusion criteria was also a factor affecting the results.
Conclusion
Our findings corroborate that GCS, MVT, albumin, CRP, smoking, and DCI are important predictors of postoperative infection and enable physicians to make informed decisions and implement appropriate clinical interventions to reduce the risk of POP. Overall, our nomogram allows for accurate and straightforward prediction of pulmonary infection occurrence in this patient population.
Data availability statement
The raw data supporting the conclusions of this article will be made available by the authors, without undue reservation.
Ethics statement
The studies involving human participants were reviewed and approved by the Ethics Committee in Clinical Research (ECCR) of the First Affiliated Hospital of Wenzhou Medical University. The acceptance number of our review is KY2023-R079. Informed consent was not required in this study because the data collected did not contain personal identifiers.
Author contributions
CC made substantial contributions to the conception and design of this study and agreed to be accountable for all aspects of the study in ensuring that questions related to the accuracy or integrity of any part of the study were appropriately investigated and resolved. XJ participated in drafting and revising the manuscript and in acquiring, collecting, analyzing, and interpreting the data. SW, CZ, and SY participated in collecting and interpreting the data. LL and SX participated in editing the final draft and confirmed the authenticity of the data. All authors have agreed to submit the article to the current journal, approved the final version for publication, and have taken accountability for all aspects of the article.
Funding
The authors gratefully acknowledge the financial support by the General Program (Key Program, Major Research Plan) of the National Natural Science Foundation of China under grant no. 81470225 as well as the Basic Science Foundation of Wenzhou City under Project no. Y2020003.
Acknowledgments
All authors appreciate the participation of the patients and their families.
Conflict of interest
The authors declare that the research was conducted in the absence of any commercial or financial relationships that could be construed as a potential conflict of interest.
Publisher's note
All claims expressed in this article are solely those of the authors and do not necessarily represent those of their affiliated organizations, or those of the publisher, the editors and the reviewers. Any product that may be evaluated in this article, or claim that may be made by its manufacturer, is not guaranteed or endorsed by the publisher.
References
1. Collaborators GBDS. Global, regional, and national burden of stroke and its risk factors, 1990-2019: a systematic analysis for the Global Burden of Disease Study 2019. Lancet Neurol. (2021) 20:795–820.
2. Ma Q, Li R, Wang L, Yin P, Wang Y, Yan C, et al. Temporal trend and attributable risk factors of stroke burden in China, 1990-2019: an analysis for the Global Burden of Disease Study 2019. Lancet Public Health. (2021) 6:e897–906. doi: 10.1016/S2468-2667(21)00228-0
3. Tu WJ Wang LD Special Special writing group of China stroke surveillance R. China stroke surveillance report 2021. Mil Med Res. (2023) 10:33. doi: 10.1186/s40779-023-00463-x
4. Chaudhry SR, Shafique S, Sajjad S, Hanggi D, Muhammad S. Janus faced HMGB1 and post-aneurysmal subarachnoid hemorrhage (aSAH) inflammation. Int J Mol Sci. (2022) 23:19. doi: 10.3390/ijms231911216
5. de Rooij NK, Linn FH, van der Plas JA, Algra A, Rinkel GJ. Incidence of subarachnoid haemorrhage: a systematic review with emphasis on region, age, gender and time trends. J Neurol Neurosurg Psychiatry. (2007) 78:1365–72. doi: 10.1136/jnnp.2007.117655
6. van Gijn J, Kerr RS, Rinkel GJ. Subarachnoid haemorrhage. Lancet. (2007) 369:306–18. doi: 10.1016/S0140-6736(07)60153-6
7. Alaraj A, Hussein AE, Esfahani DR, Amin-Hanjani S, Aletich VA, Charbel FT. Reducing length of stay in aneurysmal subarachnoid hemorrhage: A three year institutional experience. J Clin Neurosci. (2017) 42:66–70. doi: 10.1016/j.jocn.2017.03.049
8. Ding CY, Peng L, Lin YX Yu LH, Wang DL, Kang DZ. Elevated Lactate Dehydrogenase Level Predicts Postoperative Pneumonia in Patients with Aneurysmal Subarachnoid Hemorrhage. World Neurosurg. (2019) 129:e821–e30. doi: 10.1016/j.wneu.2019.06.041
9. Zhang X, Zhang S, Wang C, Liu R, Li A. High neutrophil-to-albumin ratio predicts postoperative pneumonia in aneurysmal subarachnoid hemorrhage. Front Neurol. (2022) 13:840858. doi: 10.3389/fneur.2022.840858
10. Li R, Lin F, Chen Y, Lu J, Han H, Yan D, et al. In-hospital complication-related risk factors for discharge and 90-day outcomes in patients with aneurysmal subarachnoid hemorrhage after surgical clipping and endovascular coiling: a propensity score-matched analysis. J Neurosurg. (2021) 2:1–12. doi: 10.3171/2021.10.JNS211484
11. Savardekar A, Gyurmey T, Agarwal R, Podder S, Mohindra S, Gupta SK, et al. Incidence, risk factors, and outcome of postoperative pneumonia after microsurgical clipping of ruptured intracranial aneurysms. Surg Neurol Int. (2013) 4:24. doi: 10.4103/2152-7806.107894
12. Yuan K, Li R, Zhao Y, Wang K, Lin F, Lu J, et al. Pre-operative predictors for post-operative pneumonia in aneurysmal subarachnoid hemorrhage after surgical clipping and endovascular coiling: a single-center retrospective study. Front Neurol. (2022) 13:893516. doi: 10.3389/fneur.2022.893516
13. Balachandran VP, Gonen M, Smith JJ, DeMatteo RP. Nomograms in oncology: more than meets the eye. Lancet Oncol. (2015) 16:e173–80. doi: 10.1016/S1470-2045(14)71116-7
14. Johnson A, Bulgarelli L, Pollard T, Horng S, Celi L. A, Mark R. MIMIC-IV (version 22) PhysioNet. (2023).
15. Goldberger A, Amaral L, Glass L, Hausdorff J, Ivanov PC, Mark R. PhysioBank, PhysioToolkit, and PhysioNet: components of a new research resource for complex physiologic signals. Circulation. 1(2021) 1:e215–e220. doi: 10.1161/01.CIR.101.23.e215
16. Smith CJ, Kishore AK, Vail A, Chamorro A, Garau J, Hopkins SJ, et al. Diagnosis of stroke-associated pneumonia: recommendations from the pneumonia in stroke consensus group. Stroke. (2015) 46:2335–40. doi: 10.1161/STROKEAHA.115.009617
17. Nam KW, Kwon HM, Lim JS, Han MK, Lee YS. Clinical relevance of abnormal neuroimaging findings and long-term risk of stroke recurrence. Eur J Neurol. (2017) 24:1348–54. doi: 10.1111/ene.13391
18. Nam KW, Kim TJ, Lee JS, Kwon HM, Lee YS, Ko SB, et al. High neutrophil-to-lymphocyte ratio predicts stroke-associated pneumonia. Stroke. (2018) 49:1886–92. doi: 10.1161/STROKEAHA.118.021228
19. Liu J, Huang J, Ma S, Wang K. Incorporating group correlations in genome-wide association studies using smoothed group Lasso. Biostatistics. (2013) 14:205–19. doi: 10.1093/biostatistics/kxs034
20. Hijazi Z, Oldgren J, Lindback J, Alexander JH, Connolly SJ, Eikelboom JW, et al. The novel biomarker-based ABC (age, biomarkers, clinical history)-bleeding risk score for patients with atrial fibrillation: a derivation and validation study. Lancet. (2016) 387:2302–11. doi: 10.1016/S0140-6736(16)00741-8
21. Vera Urquiza R, Bucio Reta ER, Berrios Barcenas EA, Choreno Machain T. Risk factors for the development of postoperative pneumonia after cardiac surgery. Arch Cardiol Mex. (2016) 86:203–7. doi: 10.1016/j.acmx.2015.12.005
22. Liu DD, Chu SF, Chen C, Yang PF, Chen NH, He X. Research progress in stroke-induced immunodepression syndrome (SIDS) and stroke-associated pneumonia (SAP). Neurochem Int. (2018) 114:42–54. doi: 10.1016/j.neuint.2018.01.002
23. Cavallo C, Safavi-Abbasi S, Kalani MYS, Gandhi S, Sun H, Oppenlander ME, et al. Pulmonary complications after spontaneous aneurysmal subarachnoid hemorrhage: experience from barrow neurological institute. World Neurosurg. (2018) 119:e366–e73. doi: 10.1016/j.wneu.2018.07.166
24. Oliveira J, Zagalo C, Cavaco-Silva P. Prevention of ventilator-associated pneumonia. Rev Port Pneumol. (2014) 20:152–61. doi: 10.1016/j.rppneu.2014.01.002
25. Dubois MJ, Orellana-Jimenez C, Melot C, De Backer D, Berre J, Leeman M, et al. Albumin administration improves organ function in critically ill hypoalbuminemic patients: a prospective, randomized, controlled, pilot study. Crit Care Med. (2006) 34:2536–40. doi: 10.1097/01.CCM.0000239119.57544.0C
26. Vincent JL, Dubois MJ, Navickis RJ, Wilkes MM. Hypoalbuminemia in acute illness: is there a rationale for intervention? A meta-analysis of cohort studies and controlled trials. Ann Surg. (2003) 237:319–34. doi: 10.1097/01.SLA.0000055547.93484.87
27. Suarez JI, Martin RH, Calvillo E, Dillon C, Bershad EM, Macdonald RL, et al. The albumin in subarachnoid hemorrhage (ALISAH) multicenter pilot clinical trial: safety and neurologic outcomes. Stroke. (2012) 43:683–90. doi: 10.1161/STROKEAHA.111.633958
28. Li S, Su J, Sui Q, Wang G. A nomogram for predicting postoperative pulmonary infection in esophageal cancer patients. BMC Pulm Med. (2021) 21:283. doi: 10.1186/s12890-021-01656-7
29. Kunutsor SK, Laukkanen JA. Serum C-reactive protein-to-albumin ratio is a potential risk indicator for pneumonia: findings from a prospective cohort study. Respir Med. (2022) 199:106894. doi: 10.1016/j.rmed.2022.106894
30. Richter F, Mehdorn AS, Fedders T, Reichert B, Egberts JH, Becker T, et al. C-Reactive protein as predictor for infectious complications after robotic and open esophagectomies. J Clin Med. (2022) 11:19. doi: 10.3390/jcm11195654
31. Fakler JK, Grafe A, Dinger J, Josten C, Aust G. Perioperative risk factors in patients with a femoral neck fracture - influence of 25-hydroxyvitamin D and C-reactive protein on postoperative medical complications and 1-year mortality. BMC Musculoskelet Disord. (2016) 17:51. doi: 10.1186/s12891-016-0906-1
32. Barbic J, Ivic D, Alkhamis T, Drenjancevic D, Ivic J, Harsanji-Drenjancevic I, et al. Kinetics of changes in serum concentrations of procalcitonin, interleukin-6, and C- reactive protein after elective abdominal surgery. Can it be used to detect postoperative complications? Coll Antropol. (2013) 37:195–201.
33. Lugg ST, Agostini PJ, Tikka T, Kerr A, Adams K, Bishay E, et al. Long-term impact of developing a postoperative pulmonary complication after lung surgery. Thorax. (2016) 71:171–6. doi: 10.1136/thoraxjnl-2015-207697
34. Parsons A, Daley A, Begh R, Aveyard P. Influence of smoking cessation after diagnosis of early stage lung cancer on prognosis: systematic review of observational studies with meta-analysis. BMJ. (2010) 340:b5569. doi: 10.1136/bmj.b5569
35. Foreman PM, Chua M, Harrigan MR, Fisher WS. 3rd, Vyas NA, Lipsky RH, et al. Association of nosocomial infections with delayed cerebral ischemia in aneurysmal subarachnoid hemorrhage. J Neurosurg. (2016) 125:1383–9. doi: 10.3171/2015.10.JNS151959
36. Griesshammer M, Bangerter M, Sauer T, Wennauer R, Bergmann L, Heimpel H. Aetiology and clinical significance of thrombocytosis: analysis of 732 patients with an elevated platelet count. J Intern Med. (1999) 245:295–300. doi: 10.1046/j.1365-2796.1999.00452.x
37. Oda S, Hirasawa H, Shiga H, Nakanishi K, Matsuda K, Nakamua M. Sequential measurement of IL-6 blood levels in patients with systemic inflammatory response syndrome (SIRS)/sepsis. Cytokine. (2005) 29:169–75. doi: 10.1016/j.cyto.2004.10.010
38. Dhar R, Diringer MN. The burden of the systemic inflammatory response predicts vasospasm and outcome after subarachnoid hemorrhage. Neurocrit Care. (2008) 8:404–12. doi: 10.1007/s12028-008-9054-2
39. Kasius KM, Frijns CJ, Algra A, Rinkel GJ. Association of platelet and leukocyte counts with delayed cerebral ischemia in aneurysmal subarachnoid hemorrhage. Cerebrovasc Dis. (2010) 29:576–83. doi: 10.1159/000306645
40. McMahon CJ, Hopkins S, Vail A, King AT, Smith D, Illingworth KJ, et al. Inflammation as a predictor for delayed cerebral ischemia after aneurysmal subarachnoid haemorrhage. J Neurointerv Surg. (2013) 5:512–7. doi: 10.1136/neurintsurg-2012-010386
Keywords: postoperative pneumonia, aneurysmal subarachnoid hemorrhage, Medical Information Mart for Intensive Care, nomogram, external validation
Citation: Jin X, Wang S, Zhang C, Yang S, Lou L, Xu S and Cai C (2023) Development and external validation of a nomogram for predicting postoperative pneumonia in aneurysmal subarachnoid hemorrhage. Front. Neurol. 14:1251570. doi: 10.3389/fneur.2023.1251570
Received: 01 July 2023; Accepted: 07 August 2023;
Published: 04 September 2023.
Edited by:
Wen-Jun Tu, Chinese Academy of Medical Sciences and Peking Union Medical College, ChinaReviewed by:
Qin Genggeng, Southern Medical University, ChinaZilong He, Southern Medical University, China
Kaibin Huang, Southern Medical University, China
Copyright © 2023 Jin, Wang, Zhang, Yang, Lou, Xu and Cai. This is an open-access article distributed under the terms of the Creative Commons Attribution License (CC BY). The use, distribution or reproduction in other forums is permitted, provided the original author(s) and the copyright owner(s) are credited and that the original publication in this journal is cited, in accordance with accepted academic practice. No use, distribution or reproduction is permitted which does not comply with these terms.
*Correspondence: Chang Cai, V1pGWTIwMTdAMTYzLkNPTQ==