- 1Te Herenga Waka – Victoria University of Wellington, Wellington, New Zealand
- 2TBI Network, Auckland University of Technology, Auckland, New Zealand
- 3University of Otago, Dunedin, New Zealand
- 4Massey University, Auckland, New Zealand
Objective: A latent disease explanation cannot exclusively explain post-concussion symptoms after mild traumatic brain injury (mTBI). Network analysis offers an alternative form of explanation for relationships between symptoms. The study aimed to apply network analysis to post-concussion symptoms in two different mTBI cohorts; an acute treatment-seeking sample and a sample 10 years post-mTBI.
Method: The treatment-seeking sample (n = 258) were on average 6 weeks post-injury; the 10 year post mTBI sample (n = 193) was derived from a population-based incidence and outcomes study (BIONIC). Network analysis was completed on post-concussion symptoms measured using the Rivermead Post-Concussion Questionnaire.
Results: In the treatment-seeking sample, frustration, blurred vision, and concentration difficulties were central to the network. These symptoms remained central in the 10 year post mTBI sample. A Network Comparison Test revealed evidence of a difference in network structure across the two samples (p = 0.045). However, the only symptoms that showed significant differences in strength centrality across samples were irritability and restlessness.
Conclusion: The current findings suggest that frustration, blurred vision and concentration difficulties may have an influential role in the experience and maintenance of post-concussion symptoms. The impact of these symptoms may remain stable over time. Targeting and prioritising the management of these symptoms may be beneficial for mTBI rehabilitation.
Introduction
There is a growing shift in recognising the frequency, persistence, and impact of post-concussion symptoms following mild traumatic brain injury (mTBI). The previously held belief that mTBI is predominately an acute event, with only a small minority of individuals experiencing persisting symptoms, is no longer supported (1–4). A substantial proportion of people can experience post-concussion symptoms that can persist for several months and sometimes even years (4–7). The implications of persistent post-concussion symptoms can be profound resulting in high disability, lower quality of life and increased use of healthcare resources (8–10). Thus, a better understanding of the aetiology of these symptoms is needed to inform intervention and prevention.
Historically, the term “post-concussion syndrome” has been used to conceptualise and explain the persistence of post-concussion symptoms after mTBI (11). However, the use of this term has been contentious (12). ‘Syndrome’ implies that the resultant symptoms are explained by a single disease entity, in this case, mTBI; yet this explanation has not been supported empirically (3, 13, 14). One problem is that post-concussion symptoms do not always cluster in the same and predictable manner (15, 16). This is not consistent with the symptoms truly representing a specific, cohesive, and predictable syndrome. In addition, post-concussion symptoms tend to be positively correlated (i.e., people who have worse headaches tend also to be more likely to experience concentration impairments, and blurred vision, etc.) (17). The severity of mTBI also does not appear to be a consistently strong predictor of post-concussion symptoms (18). Several studies have found a limited association between mTBI severity indices (such as loss of consciousness and post-traumatic amnesia) and acute or chronic post-concussion symptoms (19–21). In addition, research comparing the severity of post-concussion symptoms and functional outcomes based on the presence of acute intracranial abnormalities has been remarkably mixed (22). Although the term post-concussion symptoms might suggest otherwise, these symptoms are also not specific to TBI and frequently occur following trauma (23, 24), as well as in healthy adults and children (9, 25).
Finally, it is generally agreed that many factors contribute to the development and maintenance of post-concussion symptoms (3, 14). This includes the biological effects of the TBI, psychological and psychosocial factors, chronic pain, pre-injury vulnerabilities, demographic factors and personality characteristics (26–30). However, none of these factors has emerged as a latent common cause (3, 31). Instead, current conceptualisations assert that post-concussion symptoms are multifactorial in causation in accordance with a biopsychosocial framework (14, 18, 28, 32). Thus, a latent model or common cause theory for post-concussion symptoms is inconsistent with current understandings of the aetiology of post-concussion symptoms and prevailing biopsychosocial conceptualisations.
Recently, a new psychometric approach has become popular in psychology that provides an alternative to latent variable (common factor) explanations for symptom covariance. This is the network perspective (33). In network theory, symptoms can be conceptualised as nodes which can be connected by edges. Edges can be directed (representing directional causal effects) or undirected (where the direction of causality, if any, is unknown). The network perspective provides a new form of explanation for relationships observed between sets of symptoms: Rather than just being caused by an underlying disease entity, specific symptoms may have causal effects on one another (33, 34). There have been calls to apply network analysis to post-concussion symptoms (31). This approach would suggest that post-concussion symptoms co-occur because they are strongly interrelated, activating, amplifying, and mutually reinforcing, not because they arise from a common latent disease entity (31). A network approach may provide an explanation as to why a set of variables are correlated with one another. Thus, adopting a network perspective could lead to new insights into our understanding of the development and maintenance of post-concussion symptoms. This approach makes it possible to examine the architecture of post-concussion symptoms and identify symptoms that are more central and strongly interconnected (31). This has the potential to provide initial targets for treatment and rehabilitation. That is, focusing on one or two symptoms that have a high degree of centrality within the network may dampen or even ameliorate other post-concussion symptoms. This has the potential to result in more effective and less labour-intensive treatment.
Two recent studies have used networks to estimate relationships among common post-concussion symptoms in uninjured adolescents at baseline (before commencing the sports season) (35, 36). These studies found that feeling more emotional and dizziness were central baseline symptoms in adolescents with a history of mental health difficulties (35) and in adolescents with attention deficit hyperactivity disorder (36). Recently, Preszler et al. (37) applied network analysis to post-concussion symptoms in 326 adolescents recruited from a concussion speciality clinic (≤28 days post-injury); post-concussion symptoms were assessed using the Post-Concussion Symptom Scale (PCSS). Dizziness and sadness were the most central symptoms (37). A further study, used this approach, in high school athletes with suspected sports-related concussion also using the PCSS. Difficulty concentrating was the most central and influential symptom in the network (38).
In summary, there appears to be value in applying network analysis to understand the relationships between symptoms that are commonly evident after mTBI. However, existing published studies have produced divergent networks in relatively narrow populations limiting their generalisability. To the best of our knowledge, there is limited research applying this approach to civilian, community-based adult mTBI samples. Although a network analysis could be of value to various aspects of mTBI recovery, understanding the centrality of symptoms within a network of post-concussion symptoms in individuals seeking treatment could help clinicians target treatment efforts to mitigate the development of persistent symptoms. In addition, applying network analysis to individuals with historical mTBI (i.e., 10 years ago) could provide novel insights into the interrelationships among these symptoms over time. These are the overall objectives of the current study. Specifically, this study aimed to (i) estimate networks of post-concussion symptoms in a sample of adult participants seeking treatment for mTBI early after injury and a sample of participants who experienced mTBI 10 years prior, (ii) determine which symptoms are most central (i.e., strongly connected to other symptoms) in each sample network to identify key areas for intervention, and (iii) explore differences in symptom network between in the two samples to examine change in post-concussion symptom networks over time.
Materials and methods
Treatment seeking mTBI: this sample consisted of data collected as part of two studies using similar prospective observational methods. Participants were recruited from outpatient clinics providing rehabilitation services for mTBI across both the North and South Islands of New Zealand between February 2019 and October 2021, obtained independently across two different studies with similar recruitment methods and inclusion criteria (39, 40). All participating clinics were funded by New Zealand’s government-funded injury insurance scheme. At recruitment sites, eligible participants (n = 337) were approached by a clinician from the outpatient clinic within 3 months of entry into the service and invited to participate. Eligibility criteria for participants were: (1) aged 16 years or older, (2) sustained an mTBI according to World Health Organization Neurotrauma Taskforce criteria (19), (3) were less than three months post-injury at enrolment, (4) were fluent in English, and (5) had no prior neurological condition or severe unstable medical condition, including a past history of severe traumatic brain injury. Eligible and consenting participants completed questionnaires via REDCap (41), a secure web-based platform (n = 252), by mailed questionnaires (n = 5), or by telephone (n = 1). 64 participants did not complete the assessment and 15 withdrew from the study. Ethical approvals were received from New Zealand’s National Health and Disability Ethics Committee (ref 18/CEN/79) and the Auckland University of Technology Ethics Committee (ref 20/32).
10 year post-mTBI: this sample came from a population-based incidence and outcomes study (BIONIC (42)) which registered all cases of TBI that occurred in Hamilton city (urban) and the Waikato District (rural) region of New Zealand between 01/03/10 and 28/02/2011. Participants were recruited through a wide range of strategies including schools and sports clubs, GPs, allied health professionals as well as from the hospital. The original study included people of all ages and TBI severities (n = 1,369). All people eligible for the study were invited to complete a series of assessments at baseline (within 2 weeks of injury), one month, six months, one, four, eight, and 10 years after their injury. For the purposes of this analysis, data for adults (who were 16 years or older at the time of the 10 year follow-up assessment) who experienced an mTBI and who completed the Rivermead Post Concussion Symptoms Questionnaire ten years after their injury were included in this analysis. At 10 years following injury eligible participants (n = 404) who indicated an interest in further research were contacted via each participant’s preferred mode of contact (e.g., phone, text, mail, email or via social media). Participants were sent a participant information sheet/consent form and were asked to contact the research team if they wanted to participate. Following an expression of interest, participants were sent a link to an online questionnaire using the REDCap database. 140 participants were not able to be contacted, 47 did not complete the study measures, 13 declined to participate and 4 did not participate for unknown reasons.
Measures
For the mTBI treatment-seeking sample and the 10 year-post mTBI sample a range of measures were administered. In this study, the relevant measure was the 16-item Rivermead Post Concussion Symptom Questionnaire (43) (Cronbach’s alpha = 0.90). Each item specified a symptom (e.g., “headaches,” “nausea and/or vomiting”), and participants were asked to rate their experience in the previous 24 h. These response options were 0 = not experienced at all; 1 = no more of a problem than before injury; 2 = a mild problem; 3 = a moderate problem; 4 = a severe problem. In line with recommended practice “No more of a problem” was rescored as 0 rather than 1 (43).
Data analysis
In the mTBI treatment-seeking sample 0.4% of data points were missing among participants and 1% of data points were missing in the 10 year post-mTBI sample. Parameter selection in networks was conducted using the EBICglasso algorithm, which selects edges using a graphical lasso (34). Pairwise complete observations were used when calculating the input correlation matrix for the EBICglasso algorithm. The tuning parameter for the lasso is chosen using the Extended Bayesian Information Criterion, which in turn has a tuning parameter gamma (which we left at its default of 0.5).
Network parameters were estimated via full information maximum likelihood using the psychonetrics (44) and qgraph packages (45). The only variables in the network models were the 16 symptoms in the Rivermead Post Concussion Symptom Questionnaire. Full information maximum likelihood permits the inclusion of cases and variables with missing data but has the limitation of assuming the variables have a multivariate normal distribution. Taken literally, this assumption is breached because the original data stems from ordered-categorical items (whereas the multivariate normal distribution is continuous). We also completed a version of our analyses using diagonally weighted least squares estimation, which does not permit missing data but does not assume multivariate normality and is thus more robust to the use of ordered-categorical data. The results for these analyses were similar to those presented here and can be found in the Supplementary material. As is the case for any statistical method, network analyses can be vulnerable to sampling error and imperfect replicability. Some recent findings suggest that networks can be especially vulnerable to poor replicability (46). One heuristic for assessing the replicability of networks is the “case drop” bootstrap method, where a subset of cases is randomly dropped from a sample, edge weights and centrality are recalculated, and then correlated with the edge weight and centrality estimates from the full sample. If the findings are relatively stable, dropping a small proportion of cases should result in edge and centrality estimates in the bootstrap sample that remain strongly correlated with those in the full sample. Such bootstrapping can be conducted repeatedly, with differing proportions of cases excluded. We adopted this approach to assess the stability of network edges and centrality by using the case-drop strategy in the bootnet package (34). The Fruchterman-Reingold algorithm was used for network plotting (47), resulting in a plot where the distance between nodes corresponds approximately to the strength of their connections. All analyses were conducted in R (R Core Team), version 4.2.1 (48). Skewness and kurtosis statistics were calculated using the moments package (49).
Results
A summary of the demographic characteristics of the treatment-seeking mTBI and 10 year post-mTBI samples (at 10 year follow-up) is presented in Table 1.
Descriptive statistics
Descriptive statistics for the RPQ for each sample are displayed in Table 2. Symptoms were positively correlated across participants, with a median correlation between symptom pairs of 0.40 (min = 0.11, max = 0.75) in the mTBI treatment-seeking group and 0.37 (min = 0.10, max = 0.70) in the 10 year post mTBI group.
Network analysis
mTBI treatment seeking sample
The estimated network for the treatment-seeking sample is displayed in Figure 1. In the figure, thicker edges represent stronger connections. Importantly, the edges in this network are partial correlations, which means that they represent relationships between pairs of items while controlling all remaining nodes in the network. This means that they more plausibly represent causal effects between symptom pairs than would zero-order correlations (although the directions of any effects remain unknown, and it remains possible that third variables outside the network could produce spurious relationships). Because the edges have been subject to a regularisation process via LASSO during which some edges were removed, there is evidence in favour of the existence of each included edge in the population (even the relatively small edges).
Several interesting features are apparent in the network for the treatment-seeking group. First, items cluster to some extent into “communities”: For example, the mood symptoms of depressed mood, frustration and irritability are clearly connected. So too are the cognitive symptoms of forgetfulness, poor concentration, and “taking longer to think.” Visual disturbances (i.e., blurred vision and double vision) also clustered strongly together, alongside nausea and dizziness; and there was a cluster of more general physical symptoms (i.e., headache, light and noise sensitivity).
Second, almost all of the edges are positive. The strongest edges in the network included are those between blurred vision and double vision, dizziness and nausea, concentration difficulties and taking longer to think, frustration and depression, frustration and irritability, noise sensitivity and light sensitivity, and sleep disturbances and restlessness. Third, the network is of moderate density: Of a possible (16*15)/2 = 120 edges between items, the EBICglasso selected 75 edges, while the remaining 45 were constrained to zero, and are not displayed in the network plot.
Strength centrality plot. A strength centrality plot was also generated (see Figure 2). The strength centrality of each node is the sum of its connections (i.e., partial correlations) to other nodes. These were then converted to z-scores for easier interpretation. The centrality plot for the treatment-seeking sample suggested that the most central symptom was frustration, followed by blurred vision, poor concentration, and taking longer to think. The least central symptom was irritability, although the centrality estimates were not drastically different across items.
Network stability. The stability analyses indicated that (across 1,000 simulations), randomly dropping up to 59.3% of cases resulted in edge weights that retained a correlation of at least 0.7 with those in the original sample. The same percentage (59.3%) could be dropped while retaining a correlation of 0.7 between the strength estimates in the original sample and those in the trimmed sample. This suggests relatively good stability (Epskamp et al. (34) suggest that these percentages should preferably be above 50%).
Fit of network model. The covariance matrix between items implied by the network model was a relatively strong fit to the sample covariance matrix. A root mean square error of approximation (RMSEA) of 0.00 indicated relatively essential no error relative to model complexity; an RMSEA of less than 0.05 would typically be considered to indicate good fit (50). The comparative fit index (CFI) of 1.00 indicated extremely good fit relative to an independence model (a CFI of greater than 0.95 would typically be considered to indicate good fit). The chi-square statistic of χ2(45) = 43.85, p = 0.52 indicated that a null hypothesis of perfect fit in the population could not be rejected. Importantly, these fit statistics are vulnerable to overfitting due to the fact that they were calculated using the same data used to select parameters and fit the model.
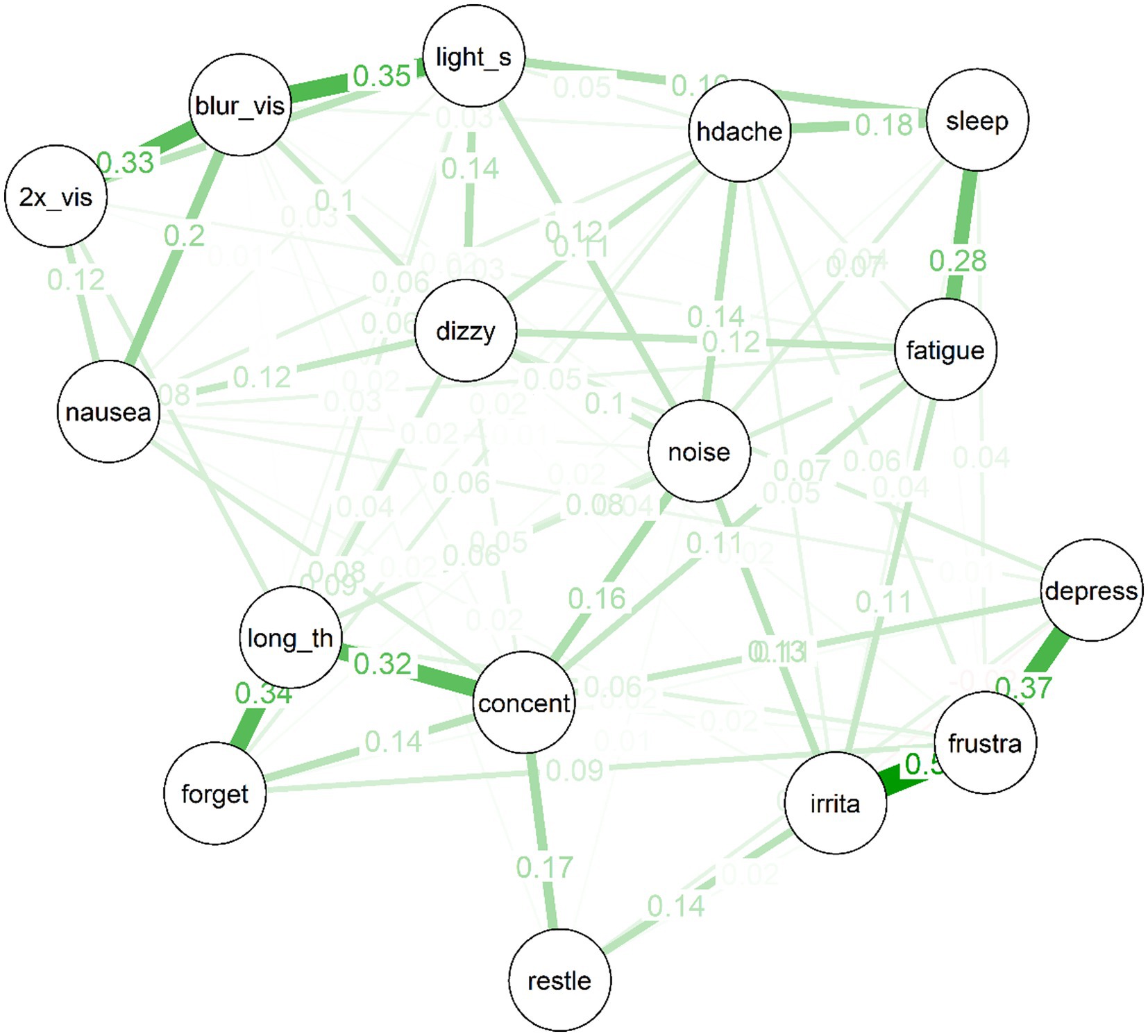
Figure 3. Partial correlation network for 10 year post-mTBI sample, the width, and saturation of edges are proportional to their strength.
10 years post mTBI
The edges for the network of post-concussive symptoms for the 10 year post mTBI sample were selected via the EBICglasso algorithm. Edges were then estimated using full information maximum likelihood.
Several interesting features are apparent in the network for the 10 year post-mTBI sample (See Figure 3). As was the case for the treatment-seeking sample, items cluster to some extent into similar “communities”: For example, the mood symptoms of depressed mood, frustration and irritability are strongly connected. So too are the cognitive symptoms of forgetfulness, poor concentration, and “taking longer to think.” There is also a cluster of physical symptoms (e.g., double vision, nausea, and light sensitivity) clustered around “blurred vision.” Second, there are no negative (red) partial correlations in the network. Third, the network is moderately sparse: Of a possible (16*15)/2 = 120 edges between items, the EBICglasso selected 77 edges, while the remaining 43 were constrained to zero, and are not displayed in the network plot.
Strength centrality plot. The strength centrality plot (see Figure 4) suggested that the three most central symptoms for the 10 year post mTBI sample were poor concentration. Frustration and blurred vision. These nodes were also the most central in Study 1 (albeit in a different order). The least central symptom (by some margin) was restlessness (the strength centrality of each node is the sum of its connections (i.e., partial correlations) to other nodes. These were then converted to z-scores for easier interpretation).
Network stability. Stability analyses indicated that (across 1,000 simulations), randomly dropping up to 35.9% of cases resulted in edge weights and strength centrality estimates that each retained a correlation of at least 0.7 with those in the original sample. This suggests relatively moderate stability, a phenomenon presumably in large part to the constrained sample size.
Fit of network model. The covariance matrix between items implied by the network model was a relatively strong fit to the sample covariance matrix. The RMSEA of 0.016 indicated relatively low error relative to model complexity, while the comparative fit index (CFI) of 1.0 indicated extremely good fit relative to an independence model. The chi-square statistic of χ2(43) = 45.06, p = 0.39 indicated that a null hypothesis of perfect fit in the population could not be rejected. These fit statistics are nevertheless vulnerable to overfitting due to the fact that they were calculated using the same data used to select parameters and fit the model.
Network comparison
The Network Comparison Test package in R (51) permits comparing network structure, edge strength, and global strength across networks. In a brief exploratory analysis we, therefore, compared the networks of the treatment-seeking and 10 year post mTBI samples. We found evidence of a difference in network structure across the two samples (p = 0.045). We found no evidence that the global strength (i.e., sum of absolute values of all edges) was different across groups: Sum treatment seeking = 7.08, sum 10 year post mTBI = 7.28, S = 0.197, p = 0.524. The only individual symptoms for which there was a significant difference in strength centrality between the two groups was irritability (p = 0.046, being higher in the 10 year post mTBI) and restlessness (p = 0.033, being higher in the 10 year post mTBI). For just a small minority of specific edges (16), there was a significant difference in edge weight between the treatment-seeking and 10 year post mTBI samples.
Discussion
The overall objective of the current study was to apply network analysis to post-concussion symptoms after mTBI. To achieve this, two civilian samples were used: individuals seeking treatment for mTBI who were on average 6 weeks post-injury, and individuals who had experienced mTBI 10 years prior. The latter sample provides a unique opportunity to examine the course of post-concussion symptoms in the longer term, and also explore possible differences in post-concussion symptom networks over time. Network analyses in both samples had good network fit and adequate network stability indicating the suitability of applying this approach to post-concussion symptoms as measured with the RPQ. There have been recent calls to use network analysis with post-concussion symptoms because these symptoms are not exclusively driven by a latent disease entity, but are also strongly interrelated, activating, amplifying, and mutually reinforcing (31, 37, 38). Our findings support the application of this approach to post-concussion symptoms after mTBI.
Network analysis identifies symptoms that are central to the network. A symptom can display high centrality due to being a common cause of many other symptoms or being affected by many other symptoms. Symptom centrality provides a preliminary basis for hypothesis-generation regarding symptoms which may be fruitful targets for clinical intervention given the widespread effect they have on the entire network of symptoms. In the treatment-seeking sample, the most central symptoms were frustration, followed by blurred vision, poor concentration, and taking longer to think. Interestingly, when considering the network of post-concussion symptoms in the 10 year post-injury sample the symptoms central to the network were consistent with the treatment-seeking sample, although the order of centrality differed. In this cohort poor concentration was most central followed by frustration and blurred vision. The possible role of each of these symptoms on the network of post-concussion symptoms will now be discussed.
First, in regards to frustration, there is a wealth of evidence that acute psychological distress contributes to the development and maintenance of post-concussion symptoms (3, 28, 29, 52). This distress may be precipitated by the pathophysiology of mTBI influencing emotion centres within the cerebral cortex (i.e., limbic system, prefrontal cortex), as well as challenges adjusting to the ongoing effects of the injury and its implications on functioning (53–55). This distress may also be higher in those with a pre-injury mental health diagnosis, a robust prognostic risk factor for poorer recovery after mTBI (3, 13, 56). Our results demonstrate the relationship between frustration and many other post-concussions symptoms; that is, when frustration is heightened other post-concussion symptoms are also likely to be experienced as more severe. In addition, the central role of frustration on the network of post-concussion symptoms was still evident in the 10 year post-injury sample which suggests that these relationships may persist over time. As our study adopts a cross-sectional design, it is not possible to infer the directionality of the relationship between frustration and post-concussion symptoms within the network. However, it could be speculated that a bidirectional relationship exists where frustration influences symptoms, i.e., concentration difficulties and the presence of post-concussion symptoms increases the intensity of frustration, which is then reinforced over time.
The finding of blurred vision as a central symptom within the treatment-seeking sample and 10 year post-injury sample is intriguing. The visual system has expansive anatomy and physiology throughout the brain (57). The large vision network requires efficient neural interconnections and processing from multiple areas of the brain including frontal and posterior cerebral cortices, cranial nerves, and axonal interconnections (58). It is therefore not surprising that the visual system may be particularly vulnerable to the effects of brain injury (59). In regards to mTBI, vision system impairments have been identified in the first 2 weeks following injury (60) as well as in those with prolonged recovery (e.g., up to 12 months) (61, 62). Our findings provide initial evidence of the possible consequential impact of visual disturbances, more specifically blurred vision, on other post-concussion symptoms possibly contributing to their severity and persistence over time. Again, the directionality of these relationships cannot be confidently inferred in this study, but there is evidence in the literature to suggest that these associations could be bidirectional. Studies have found that blurred or double vision contributes to fatigue, concentration and reading difficulties (63, 64), whereas attentional and executive dysfunction can cause visual disturbances (65). Network analysis illustrates the role these symptoms have within the entire network of post-concussion symptoms. Our findings in conjunction with the existing literature thus suggest that from a rehabilitation perspective, when individuals are seeking treatment for mTBI there may be benefit in targeting blurred vision given the widespread effect this symptom may have on the entire network of symptoms. Currently, findings pertaining to the efficacy of treatments of visual disturbances is mixed (66, 67). However, these findings support calls for ongoing research specifically into visual rehabilitation in mTBI given the possible influential effect that difficulties in this area have on the constellation of post-concussion symptoms.
Finally, concentration difficulties also emerged as a central symptom in the network analysis and was the most central symptom in the 10 year post-injury sample. The significant role of cognitive difficulties on mTBI long-term health outcomes was highlighted in a systematic review of prognostic models of mTBI. Silverberg et al. (3) found that early-post injury neuropsychological functioning was one of the most robust prognostic factors of mTBI outcomes. Although “neuropsychological functioning” was not defined, this may well comprise concentration difficulties given the fundamental role that this cognitive process has on wider cognitive functioning. These sequalae may be associated with the acute pathophysiology of mTBI (68, 69). In addition, concentration difficulty is a post-concussion symptom highly susceptible to the impact of other difficulties such as pain, fatigue, neurological symptoms, as well as mental distress (70). This may account for its central role within the post-concussion symptoms network.
In summary, the findings of the current study suggest that poor concentration, frustration and blurred vision may have an influential role on post-concussion symptoms, as well as their presence over time given their centrality within the network of symptoms. Not only were these symptoms highly central when individuals are approximately 6 weeks post-injury, but also even when individuals are 10 years post-injury. This finding provides initial unique insights to aid our understanding of how post-concussion symptoms develops and how they may be maintained over time. From a rehabilitation perspective, if future evidence confirms that frustration, concentration difficulties and blurred vision are central due to having causal effects on other symptoms, prioritising the treatment of these three symptoms may trigger the greatest reduction in overall post-concussion symptoms. Finally, the interplay between these symptoms over time may offer valuable insights into the distinct recovery pathways experienced after mTBI and the factors influencing participation in rehabilitation.
Currently, the application of network analysis to post-concussion symptoms has produced mixed and inconsistent findings. In accordance with our results, Goodwin et al. (38) found that difficulties concentrating were the most central symptom in high school athletes with suspected sports-related concussion. Additionally, Iverson et al. (35) found that “feeling more emotional” was a central baseline symptom in adolescents with ADHD; however, dizziness was also central which was also found in Preszler et al. (37) network analysis of adolescents recruited from a concussion speciality clinic. It is difficult to make direct inferences between our findings and the current evidence given the relatively narrow populations used. Differences in the centrality of symptoms may be indicative of the unique characteristics of the sample being analysed. The use of network analysis in post-concussion symptoms is in its infancy and future research is needed to examine this approach. It may be the case that a “one size fits all” network is not evident across the spectrum of mTBI, and differing networks may be revealed across different cohorts of individuals who have experienced a mTBI. For example, a post-concussion symptoms network for adolescents with mTBI may be different from a network of post-concussion symptoms for adults. One limitation of network analysis, is that its flexibility and complexity may mean that estimated networks are vulnerable to poor replicability (46). One strength of this paper is that we have used two different samples of individuals who have experienced a mTBI and yet the centrality in symptoms was very consistent. Although further replication is required, it does provide initial support for the findings of the role of these symptoms in the development and maintenance of post-concussion symptoms over time.
It is interesting when comparing the overall network of symptoms between the treatment-seeking sample to those who experienced their mTBI 10 years earlier, the centrality of symptoms remained fairly consistent with significant differences found only in irritability and restlessness. This finding suggests that the relationship between post-concussion symptoms may remain, for the most part, somewhat constant over time. However, it is currently not possible to infer the direct role that mTBI has had on the network of symptoms or these relationships. An ongoing challenge faced in our understanding of post-concussion symptoms is that all symptoms are non-specific and it is therefore difficult to tease out the causal effects of injury vs. non-injury factors (18). This highlights an important limitation that must be considered when interpreting the results of this study. Several non-symptom variables were absent from the network that could influence the relationship between symptoms, e.g., age, gender, concussion and physical health history. More specifically, it may be the case that certain individuals, have pre-existing ‘vulnerable’ networks where the strength of the relationship between certain symptoms are already evident and thus continue to persist over time. It may also be that the relationship between symptoms, or the centrality of symptoms changes as a consequence of a mTBI and these changes persist overtime. These changes may also be maintained by other non-injury-related factors (14). One of these factors that is particularly worthy of highlighting is presence of a pre-injury psychiatric diagnosis given the impact this could have on the network of symptoms. Pre-injury mental health conditions are robust predictors of mTBI outcomes, affecting incidence (71), severity (72), and duration of symptoms (73, 74). In support of the potential confounding role this factor may have on the interrelationship between symptoms, Fonda et al. (75) recently applied network analysis to post-concussion symptoms and comorbid psychiatric conditions in veterans and service members. Exploratory factor analysis was used to identify and define nodes to include in the network analysis based on a range of self-report measures. In this study, mTBI military count was included in the network analysis and was found to be the least influential node in the network. The authors also compared networks between those that had sustained a mTBI (n = 322) and those that had not (n = 297), and found that comparable network structures were evident between the two groups. It was consequently concluded that the pattern of symptoms prevalent in the sample may be largely independent of mTBI and psychiatric conditions may be the most influential factor in their development and maintenance. Thus, inclusion of potential confounding variables, such as pre-injury psychiatric conditions in future research using network analysis is essential to ensure that an understanding of all the factors, not just mTBI, that influence the network of post-concussion symptoms is understood.
The current study is also limited in that a single self-report tool was used to ascertain post-concussion symptom severity and thus post-concussion symptom severity was ascertained by using a single item that assesses symptom severity in the past 24 h. Bias in symptom reporting, as well as constraints imposed by a single self-report measure, could impact the validity of the data. Recently, Preszler et al. (37) included clinical assessments of vestibular and oculomotor functioning in their network analysis of post-concussion symptoms. In addition, the time constraint of the measure used could impact the accurate attainment of overall symptom experience. Future research would benefit from including data on post-concussion symptoms gathered from multiple methodologies, i.e., cognitive test scores or structured interviews of psychological disorders, as well as over an extended period of time. Specific information pertaining to the injury characteristics of these samples (i.e., Glasgow Coma Scale (GCS), duration of post-traumatic amnesia (PTA) or loss of consciousness (LOC), presence of intracranial injury) was not available. Given the implications these could have on symptom manifestation and severity, future research should include these variables when conducting network analyses. The dataset for both samples was only moderate in size, although the bootstrap analyses supported the stability of the results despite the sample sizes. Future studies could benefit from applying the Monte Carlo method for sample size determination for network analyses recently developed by Constantin et al. (76). Finally, it is important to bear in mind the characteristics of the samples and the implications this could have on the generalisability of the findings. The treatment-seeking sample had a higher rate of females with the most common cause of injury being either hit by an object or a fall. This is not consistent with the characteristics of mTBI more generally (42) and may therefore represent a unique cohort who are needing treatment. In lieu of this, the study findings should be treated cautiously and future research which addresses these limitations, is needed to ensure replicability of these results.
In conclusion, network analysis applied to post-concussion symptoms is a promising approach and may provide novel insights into our understanding of post-concussion symptoms and potentially aid in prioritisation and rehabilitation of these symptoms. We found that in a mTBI treatment seeking sample frustration, blurred vision and concentration difficulties were the most central symptoms within the network. These symptoms were also most central in those who were 10 years post mTBI. We also found few significant differences when the two networks were compared, suggesting that the relationship between symptoms within this network may remain somewhat constant over time. However, the use of network analysis in post-concussion symptoms is currently limited and future research is needed to replicate the current findings, compare networks with different cohorts of individuals who have experienced mTBI, as well as examine the direct role that mTBI has within the network.
Data availability statement
The raw data supporting the conclusions of this article will be made available by the authors, without undue reservation.
Ethics statement
Ethical approval for the treatment seeking sample were received from New Zealand’s National Health and Disability Ethics Committee (ref 18/CEN/79) and the Auckland University of Technology Ethics Committee (ref 20/32). For the 10 year post-mTBI sample ethical approval was received Health and Disability Ethics Committee (ref 19NTB166) and Auckland University of Technology Ethics Committee AUTEC 9 (ref 19/408). The patients/participants provided their written informed consent to participate in this study.
Author contributions
JF, DS, AT, and MW drafted and designed the study. MW designed and executed the statistical analyses. JF, DS, and AT collected data for the study. JF wrote an initial manuscript draft. DS, AT, and MW revised and approved the final manuscript. All authors contributed to the article and approved the submitted version.
Funding
This research was supported by grants from the Health Research Council of NZ (treatment-seeking sample: ref. 18/046; 20/041) and a Rutherford discovery fellowship administered by the Royal Society Tē Apārangi (10 year post-injury sample).
Conflict of interest
The authors declare that the research was conducted in the absence of any commercial or financial relationships that could be construed as a potential conflict of interest.
Publisher’s note
All claims expressed in this article are solely those of the authors and do not necessarily represent those of their affiliated organizations, or those of the publisher, the editors and the reviewers. Any product that may be evaluated in this article, or claim that may be made by its manufacturer, is not guaranteed or endorsed by the publisher.
Supplementary material
The Supplementary material for this article can be found online at: https://www.frontiersin.org/articles/10.3389/fneur.2023.1226367/full#supplementary-material
References
1. Nelson, LD, Temkin, NR, Dikmen, S, Barber, J, Giacino, JT, Yuh, E, et al. Recovery after mild traumatic brain injury in patients presenting to US level I trauma centers. JAMA Neurol. (2019) 76:1049–59. doi: 10.1001/jamaneurol.2019.1313
2. Lagacé-Legendre, C, Boucher, V, Robert, S, Tardif, PA, Ouellet, MC, de Guise, E, et al. Persistent Postconcussion symptoms: an expert consensus-based definition using the Delphi method. J Head Trauma Rehabil. (2020) 36:96–102. doi: 10.1097/HTR.0000000000000613
3. Silverberg, ND, Gardner, AJ, Brubacher, JR, Panenka, WJ, Li, JJ, and Iverson, GL. Systematic review of multivariable prognostic models for mild traumatic brain injury. J Neurotrauma. (2015) 32:517–26. doi: 10.1089/neu.2014.3600
4. Theadom, A, Parag, V, Dowell, T, McPherson, K, Starkey, N, Barker-Collo, S, et al. Persistent problems 1 year after mild traumatic brain injury: a longitudinal population study in New Zealand. Br J Gen Pract. (2015) 66:e16–23. doi: 10.3399/bjgp16X683161
5. Voormolen, DC, Cnossen, MC, Polinder, S, von Steinbuechel, N, Vos, PE, and Haagsma, JA. Divergent classification methods of post-concussion syndrome after mild traumatic brain injury: prevalence rates, risk factors, and functional outcome. J Neurotrauma. (2018) 35:1233–41. doi: 10.1089/neu.2017.5257
6. Mehrolhassani, N, Movahedi, M, Nazemi-Rafi, M, and Mirafzal, A. Persistence of post-concussion symptoms in patients with mild traumatic brain injury and no psychiatric history in the emergency department. Brain Inj. (2020) 34:1350–7. doi: 10.1080/02699052.2020.1802659
7. Machamer, J, Temkin, N, Dikmen, S, Nelson, LD, Barber, J, Hwang, P, et al. Symptom frequency and persistence in the first year after traumatic brain injury: a TRACK-TBI study. J Neurotrauma. (2022) 39:358–70. doi: 10.1089/neu.2021.0348
8. Theadom, A, Barker-Collo, S, Jones, K, Kahan, M, Te Ao, B, McPherson, K, et al. Work limitations 4 years after mild traumatic brain injury: a cohort study. Arch Phys Med Rehabil. (2017) 98:1560–6. doi: 10.1016/j.apmr.2017.01.010
9. Voormolen, DC, Polinder, S, von Steinbuechel, N, Vos, PE, Cnossen, MC, and Haagsma, JA. The association between post-concussion symptoms and health-related quality of life in patients with mild traumatic brain injury. Injury. (2019) 50:1068–74. doi: 10.1016/j.injury.2018.12.002
10. Chiang, CC, Guo, SE, Huang, KC, Lee, BO, and Fan, JY. Trajectories and associated factors of quality of life, global outcome, and post-concussion symptoms in the first year following mild traumatic brain injury. Qual Life Res. (2015) 25:2009–19. doi: 10.1007/s11136-015-1215-0
11. Ryan, LM, and Warden, DL. Post concussion syndrome. Int Rev Psychiatry. (2003) 15:310–6. doi: 10.1080/09540260310001606692
12. Broshek, DK, Pardini, JE, and Herring, SA. Persisting symptoms after concussion: time for a paradigm shift. PMR J. (2022) 14:1509–13. doi: 10.1002/pmrj.12884
13. Meares, S, Shores, EA, Taylor, AJ, Batchelor, J, Bryant, RA, Baguley, IJ, et al. Mild traumatic brain injury does not predict acute postconcussion syndrome. J Neurol Neurosurg Psychiatry. (2008) 79:300–6. doi: 10.1136/jnnp.2007.126565
14. Polinder, S, Cnossen, MC, Real, RG, Covic, A, Gorbunova, A, Voormolen, DC, et al. A multidimensional approach to post-concussion symptoms in mild traumatic brain injury. Front Neurol. (2018) 9:1113. doi: 10.3389/fneur.2018.01113
15. Arciniegas, DB, Anderson, CA, Topkoff, J, and McAllister, TW. Mild traumatic brain injury: a neuropsychiatric approach to diagnosis, evaluation, and treatment. Neuropsychiatr Dis Treat. (2005) 1:311–27. doi: 10.2147/ndt.s12160156
16. Katz, DI, Cohen, SI, and Alexander, MP. Mild traumatic brain injury. Handb Clin Neurol. (2015) 127:131–56. doi: 10.1016/B978-0-444-52892-6.00009-X
17. Eyres, S, Carey, A, Gilworth, G, Neumann, V, and Tennant, A. Construct validity and reliability of the Rivermead post-concussion symptoms questionnaire. Clin Rehabil. (2005) 19:878–87. doi: 10.1191/0269215505cr905oa
18. Silverberg, ND, and Iverson, GL. Etiology of the post-concussion syndrome: physiogenesis and psychogenesis revisited. NeuroRehabilitation. (2011) 29:317–29. doi: 10.3233/NRE-2011-0708
19. Carroll, L, Cassidy, JD, Peloso, P, Borg, J, Von Holst, H, Holm, L, et al. Prognosis for mild traumatic brain injury: results of the WHO collaborating Centre task force on mild traumatic brain injury. J Rehabil Med. (2004) 36:84–105. doi: 10.1080/16501960410023859
20. Hellstrøm, T, Westlye, LT, Sigurdardottir, S, Brunborg, C, Soberg, HL, Holthe, Ø, et al. Longitudinal changes in brain morphology from 4 weeks to 12 months after mild traumatic brain injury: associations with cognitive functions and clinical variables. Brain Inj. (2017) 31:674–85. doi: 10.1080/02699052.2017.1283537
21. Jacobs, B, Beems, T, Stulemeijer, M, van Vugt, AB, van der Vliet, TM, Borm, GF, et al. Outcome prediction in mild traumatic brain injury: age and clinical variables are stronger predictors than CT abnormalities. J Neurotrauma. (2010) 27:655–68. doi: 10.1089/neu.2009.1059
22. Karr, JE, Iverson, GL, Williams, MW, Huang, SJ, and Yang, CC. Complicated versus uncomplicated mild traumatic brain injuries: a comparison of psychological, cognitive, and post-concussion symptom outcomes. J Clin Exp Neuropsychol. (2020) 42:1049–58. doi: 10.1080/13803395.2020.1841118
23. Smith-Seemiller, L, Fow, NR, Kant, R, and Franzen, MD. Presence of post-concussion syndrome symptoms in patients with chronic pain vs mild traumatic brain injury. Brain Inj. (2003) 17:199–206. doi: 10.1080/0269905021000030823
24. Balalla, S, Krägeloh, C, Medvedev, O, and Siegert, R. Is the Rivermead post-concussion symptoms questionnaire a reliable and valid measure to assess long-term symptoms in traumatic brain injury and orthopedic injury patients? A novel investigation using Rasch analysis. Neurotrauma Rep. (2020) 1:63–72. doi: 10.1089/neur.2020.0017
25. Iverson, GL, and Lange, RT. Examination of postconcussion-like symptoms in a healthy sample. Appl Neuropsychol. (2003) 10:137–44. doi: 10.1207/S15324826AN1003_02
26. Cnossen, MC, Winkler, EA, Yue, JK, Okonkwo, DO, Valadka, AB, Steyerberg, EW, et al. Development of a prediction model for post-concussive symptoms following mild traumatic brain injury: a TRACK-TBI pilot study. J Neurotrauma. (2017) 34:2396–409. doi: 10.1089/neu.2016.4819
27. Young, G. Thirty complexities and controversies in mild traumatic brain injury and persistent post-concussion syndrome: a roadmap for research and practice. Psychol Injury Law. (2020) 13:427–51. doi: 10.1007/s12207-020-09395-6
28. Wäljas, M, Iverson, GL, Lange, RT, Hakulinen, U, Dastidar, P, Huhtala, H, et al. A prospective biopsychosocial study of the persistent post-concussion symptoms following mild traumatic brain injury. J Neurotrauma. (2015) 32:534–47. doi: 10.1089/neu.2014.3339
29. Faulkner, JW, and Snell, DL. A framework for understanding the contribution of psychosocial factors in biopsychosocial explanatory models of persistent postconcussion symptoms. Phys Ther. (2023) 103:pzac156. doi: 10.1093/ptj/pzac156
30. Skandsen, T, Stenberg, J, Follestad, T, Karaliute, M, Saksvik, SB, Einarsen, CE, et al. Personal factors associated with postconcussion symptoms 3 months after mild traumatic brain injury. Arch Phys Med Rehabil. (2021) 102:1102–12. doi: 10.1016/j.apmr.2020.10.106
31. Iverson, GL. Network analysis and precision rehabilitation for the post-concussion syndrome. Front Neurol. (2019) 10:489. doi: 10.3389/fneur.2019.00489
32. Register-Mihalik, JK, DeFreese, JD, Callahan, CE, and Carneiro, K. Utilizing the biopsychosocial model in concussion treatment: post-traumatic headache and beyond. Curr Pain Headache Rep. (2020) 24:1–7. doi: 10.1007/s11916-020-00870-y
33. Borsboom, D, Deserno, MK, Rhemtulla, M, Epskamp, S, Fried, EI, McNally, RJ, et al. Network analysis of multivariate data in psychological science. Nat Rev Methods Primers. (2021) 1:58. doi: 10.1038/s43586-021-00055-w
34. Epskamp, S, Borsboom, D, and Fried, EI. Estimating psychological networks and their accuracy: a tutorial paper. Behav Res Methods. (2018) 50:195–212. doi: 10.3758/s13428-017-0862-1
35. Iverson, GL, Jones, PJ, Karr, JE, Maxwell, B, Zafonte, R, Berkner, PD, et al. Architecture of physical, cognitive, and emotional symptoms at preseason baseline in adolescent student athletes with a history of mental health problems. Front Neurol. (2020a) 11:175. doi: 10.3389/fneur.2020.00175
36. Iverson, GL, Jones, PJ, Karr, JE, Maxwell, B, Zafonte, R, Berkner, PD, et al. Network structure of physical, cognitive, and emotional symptoms at preseason baseline in student athletes with attention-deficit/hyperactivity disorder. Arch Clin Neuropsychol. (2020b) 35:1109–22. doi: 10.1093/arclin/acaa030
37. Preszler, J, Manderino, L, Fazio-Sumrok, V, Eagle, SR, Holland, C, Collins, MW, et al. Multidomain concussion symptoms in adolescents: a network analysis. Applied neuropsychology. Child. (2022) Advanced online publication. 1–10. doi: 10.1080/21622965.2022.2099742
38. Goodwin, GJ, Salva, C, Woodyatt, JJ, Maietta, JE, Kuwabara, HC, Ross, S, et al. A-13 characterizing the network structure of post-concussion symptoms. Arch Clin Neuropsychol. (2022) 37:1248. doi: 10.1093/arclin/acac060.013
39. Faulkner, JW, Snell, DL, Theadom, A, Mahon, S, and Barker-Collo, S. The role of psychological flexibility in recovery following mild traumatic brain injury. Rehabil Psychol. (2021) 66:479–90. doi: 10.1037/rep0000406
40. Snell, DL, Faulkner, JW, Williman, JA, Silverberg, ND, Theadom, A, Surgenor, LJ, et al. Fear avoidance and return to work after mild traumatic brain injury. Brain Inj. (2023) 37:541–50. doi: 10.1080/02699052.2023.2180663
41. Harris, PA, Taylor, R, Thielke, R, Payne, J, Gonzalez, N, and Conde, JG. Research electronic data capture (REDCap)—a metadata-driven methodology and workflow process for providing translational research informatics support. J Biomed Inform. (2009) 42:377–81. doi: 10.1016/j.jbi.2008.08.010
42. Feigin, VL, Theadom, A, Barker-Collo, S, Starkey, NJ, McPherson, K, Kahan, M, et al. Incidence of traumatic brain injury in New Zealand: a population-based study. Lancet Neurol. (2013) 12:53–64. doi: 10.1016/S1474-4422(12)70262-4
43. King, NS, Crawford, S, Wenden, FJ, Moss, NE, and Wade, DT. The Rivermead post concussion symptoms questionnaire: a measure of symptoms commonly experienced after head injury and its reliability. J Neurol. (1995) 242:587–92. doi: 10.1007/BF00868811
45. Epskamp, S, Cramer, AOJ, Waldorp, LJ, Schmittmann, VD, and Borsboom, D. Qgraph: network visualizations of relationships in psychometric data. J Stat Softw. (2012) 48:1–18. doi: 10.18637/jss.v048.i04
46. Forbes, MK, Wright, AG, Markon, KE, and Krueger, RF. Evidence that psychopathology symptom networks have limited replicability. J Abnorm Psychol. (2017) 126:969–88. doi: 10.1037/abn0000276
47. Fruchterman, TM, and Reingold, EM. Graph drawing by force-directed placement. Software: practice and experience. Behav Res. (1991) 21:1129–64.
48. R Core Team. R: A language and environment for statistical computing [software]. Vienna, Austria: R Foundation for Statistical Computing; (2022) Available at: https://www.R-project.org/
49. Komsta, L, and Novometsky, F. Moments: moments, cumulants, skewness, kurtosis and related tests [software]. (2022). Available at: https://CRAN.R-project.org/package=moments
50. Hu, L, and Bentler, PM. Cutoff criteria for fit indexes in covariance structure analysis: conventional criteria versus new alternatives. Struct Equ Modeling. (1999) 6:1–55. doi: 10.1080/10705519909540118
51. Van Borkulo, CD, Van Bork, R, Boschloo, L, Kossakowski, JJ, Tio, P, Schoevers, RA, et al. Comparing network structures on three aspects: a permutation test. Psychol Methods. (2022). doi: 10.1037/met0000476
52. Ponsford, JL, Nguyen, S, Downing, M, Bosch, M, McKenzie, JE, Turner, S, et al. Factors associated with persistent post-concussion symptoms following mild traumatic brain injury in adults. J Rehabil Med. (2019) 51:32–9. doi: 10.2340/16501977-2492
53. Broshek, DK, De Marco, AP, and Freeman, JR. A review of post-concussion syndrome and psychological factors associated with concussion. Brain Inj. (2015) 29:228–37. doi: 10.3109/02699052.2014.974674
54. van der Horn, HJ, Out, ML, de Koning, ME, Mayer, AR, Spikman, JM, Sommer, IE, et al. An integrated perspective linking physiological and psychological consequences of mild traumatic brain injury. J Neurol. (2020) 267:2497–506. doi: 10.1007/s00415-019-09335-8
55. Mayer, AR, Mannell, MV, Ling, J, Gasparovic, C, and Yeo, RA. Functional connectivity in mild traumatic brain injury. Hum Brain Mapp. (2011) 32:1825–35. doi: 10.1002/hbm.21151
56. Ponsford, J, Cameron, P, Fitzgerald, M, Grant, M, Mikocka-Walus, A, and Schönberger, M. Predictors of postconcussive symptoms 3 months after mild traumatic brain injury. Neuropsychology. (2012) 26:304–13. doi: 10.1037/a0027888
57. Singman, EL. Automating the assessment of visual dysfunction after traumatic brain injury. Med Instrum. (2013) 1:3. doi: 10.7243/2052-6962-1-3
58. Kelts, EA. Traumatic brain injury and visual dysfunction: a limited overview. NeuroRehabilitation. (2010) 27:223–9. doi: 10.3233/NRE-2010-0601
59. Sen, N. An insight into the vision impairment following traumatic brain injury. Neurochem Int. (2017) 111:103–7. doi: 10.1016/j.neuint.2017.01.019
60. Mullen, SJ, Yücel, YH, Cusimano, M, Schweizer, TA, Oentoro, A, and Gupta, N. Saccadic eye movements in mild traumatic brain injury: a pilot study OPEN ACCESS. Can J Neurol Sci. (2014) 41:58–65. doi: 10.1017/S0317167100016279
61. Heitger, MH, Jones, RD, Macleod, AD, Snell, DL, Frampton, CM, and Anderson, TJ. Impaired eye movements in post-concussion syndrome indicate suboptimal brain function beyond the influence of depression, malingering or intellectual ability. Brain. (2009) 132:2850–70. doi: 10.1093/brain/awp181
62. Riggs, RV, Andrews, K, Roberts, P, and Gilewski, M. Visual deficit interventions in adult stroke and brain injury: a systematic review. Am J Phys Med Rehabil. (2007) 86:853–60. doi: 10.1097/PHM.0b013e318151f907
63. Ciuffreda, KJ, Han, Y, Kapoor, N, and Ficarra, AP. Oculomotor rehabilitation for reading in acquired brain injury. NeuroRehabilitation. (2006) 21:9–21. doi: 10.3233/NRE-2006-21103
64. Thiagarajan, P, Ciuffreda, KJ, Capo-Aponte, JE, Ludlam, DP, and Kapoor, N. Oculomotor neurorehabilitation for reading in mild traumatic brain injury (mTBI): an integrative approach. NeuroRehabilitation. (2014) 34:129–46. doi: 10.3233/NRE-131025
65. Suh, M, Kolster, R, Sarkar, R, Mc Candliss, B, and Ghajar, J, Cognitive and Neurobiological Research Consortium. Deficits in predictive smooth pursuit after mild traumatic brain injury. Neurosci Lett. (2006) 401:108–13. doi: 10.1016/j.neulet.2006.02.074
66. Barton, JJ, and Ranalli, PJ. Vision therapy: ocular motor training in mild traumatic brain injury. Ann Neurol. (2020) 88:453–61. doi: 10.1002/ana.25820
67. Simpson-Jones, ME, and Hunt, AW. Vision rehabilitation interventions following mild traumatic brain injury: a scoping review. Disabil Rehabil. (2019) 41:2206–22. doi: 10.1080/09638288.2018.1460407
68. Croall, ID, Cowie, CJ, He, J, Peel, A, Wood, J, Aribisala, BS, et al. White matter correlates of cognitive dysfunction after mild traumatic brain injury. Neurology. (2014) 83:494–501. doi: 10.1212/WNL.0000000000000666
69. de Freitas Cardoso, MG, Faleiro, RM, De Paula, JJ, Kummer, A, Caramelli, P, Teixeira, AL, et al. Cognitive impairment following acute mild traumatic brain injury. Front Neurol. (2019) 10:198. doi: 10.3389/fneur.2019.00198
70. Clarke, LA, Genat, RC, and Anderson, JF. Long-term cognitive complaint and post-concussive symptoms following mild traumatic brain injury: the role of cognitive and affective factors. Brain Inj. (2012) 26:298–307. doi: 10.3109/02699052.2012.654588
71. Legarreta, AD, Brett, BL, Solomon, GS, and Zuckerman, SL. The role of family and personal psychiatric history in postconcussion syndrome following sport-related concussion: a story of compounding risk. J Neurosurg Pediatr. (2018) 22:238–43. doi: 10.3171/2018.3.PEDS1850
72. Karr, JE, Iverson, GL, Huang, SJ, Silverberg, ND, and Yang, CC. Perceived change in physical, cognitive, and emotional symptoms after mild traumatic brain injury in patients with pre-injury anxiety or depression. J Neurotrauma. (2020) 37:1183–9. doi: 10.1089/neu.2019.6834
73. Langer, LK, Alavinia, SM, Lawrence, DW, Munce, SE, Kam, A, Tam, A, et al. Prediction of risk of prolonged post-concussion symptoms: derivation and validation of the TRICORDRR (Toronto Rehabilitation Institute concussion outcome determination and rehab recommendations) score. PLoS Med. (2021) 18:e1003652. doi: 10.1371/journal.pmed.1003652
74. Booker, J, Sinha, S, Choudhari, K, Dawson, J, and Singh, R. Description of the predictors of persistent post-concussion symptoms and disability after mild traumatic brain injury: the SHEFBIT cohort. Br J Neurosurg. (2019) 33:367–75. doi: 10.1080/02688697.2019.1598542
75. Fonda, JR, Crowe, ML, Levin, LK, Jagger-Rickels, A, Marx, BP, Milberg, WP, et al. Network analysis of mild traumatic brain injury, persistent neurobehavioral and psychiatric symptoms, and functional disability among recent-era United States veterans. J Trauma Stress. (2022) 35:1546–58. doi: 10.1002/jts.22860
76. Constantin, M, Schuurman, NK, and Vermunt, J. A general Monte Carlo method for sample size analysis in the context of network models. PsyArXiv; (2021) Available at: https://psyarxiv.com/j5v7u/
Keywords: mild traumatic brain injury, post-concussion symptoms, network analysis, outcomes, concussion
Citation: Faulkner JW, Theadom A, Snell DL and Williams MN (2023) Network analysis applied to post-concussion symptoms in two mild traumatic brain injury samples. Front. Neurol. 14:1226367. doi: 10.3389/fneur.2023.1226367
Edited by:
Nada Andelic, University of Oslo, NorwayReviewed by:
Michael Johnathan Charles Bray, Johns Hopkins University, United StatesEmilie Isager Howe, Oslo University Hospital, Norway
Marina Zeldovich, University Medical Center Göttingen, Germany
Copyright © 2023 Faulkner, Theadom, Snell and Williams. This is an open-access article distributed under the terms of the Creative Commons Attribution License (CC BY). The use, distribution or reproduction in other forums is permitted, provided the original author(s) and the copyright owner(s) are credited and that the original publication in this journal is cited, in accordance with accepted academic practice. No use, distribution or reproduction is permitted which does not comply with these terms.
*Correspondence: Josh W. Faulkner, josh.faulkner@vuw.ac.nz
†ORCID: Josh W. Faulkner, https://orcid.org/0000-0001-9555-4628
Alice Theadom, https://orcid.org/0000-0003-0351-6216
Deborah L. Snell, https://orcid.org/0000-0003-1664-0603