- 1Department of Intensive Care Unit, The Second Affiliated Hospital of Nanchang University, Nanchang, China
- 2School of Nursing, Nanchang University, Nanchang, China
- 3Department of Post Anesthesia Care Unit, The Second Affiliated Hospital of Nanchang University, Nanchang, China
Objectives: To identify and compare published models that use related factors to predict the risk of intracranial hemorrhage (ICH) in acute ischemic stroke patients receiving intravenous alteplase treatment.
Methods: Risk prediction models for ICH in acute ischemic stroke patients receiving intravenous alteplase treatment were collected from PubMed, Embase, Web of Science, and the Cochrane Library up to April 7, 2023. A meta-analysis was performed using Stata 13.0, and the included models were evaluated using the Prediction Model Risk of Bias Assessment Tool (PROBAST).
Results: A total of 656 references were screened, resulting in 13 studies being included. Among these, one was a prospective cohort study. Ten studies used internal validation; five studies used external validation, with two of them using both. The area under the receiver operating characteristic (ROC) curve for subjects reported in the models ranged from 0.68 to 0.985. Common predictors in the prediction models include National Institutes of Health Stroke Scale (NIHSS) (OR = 1.17, 95% CI 1.09–1.25, p < 0.0001), glucose (OR = 1.54, 95% CI 1.09–2.17, p < 0.05), and advanced age (OR = 1.50, 95% CI 1.15–1.94, p < 0.05), and the meta-analysis shows that these are independent risk factors. After PROBAST evaluation, all studies were assessed as having a high risk of bias but a low risk of applicability concerns.
Conclusion: This study systematically reviews available evidence on risk prediction models for ICH in acute ischemic stroke patients receiving intravenous alteplase treatment. Few models have been externally validated, while the majority demonstrate significant discriminative power.
Introduction
According to the latest global stroke data, approximately 12.2 million new stroke cases and 101 million existing stroke patients are reported worldwide each year, making it the second leading cause of death globally (1, 2). Ischemic strokes account for about 62.4–82.6% of these cases (1, 3). Revascularization methods for patients with acute ischemic stroke (AIS) include intravenous thrombolysis, intra-arterial thrombolysis, endovascular thrombectomy, and bridging thrombolytic therapy with endovascular thrombectomy (4, 5). The American Stroke Association (ASA) recommends intravenous thrombolysis with alteplase within 4.5 h of AIS onset as the first-line treatment (6). Alteplase is currently the only approved intravenous medication for AIS (7). However, its use can lead to complications such as hemorrhage, vascular edema, and seizures (8, 9). Intracranial hemorrhage (ICH) is the most severe complication, potentially resulting in prolonged hospital stays, increased medical costs, and a higher likelihood of disability and death (10). The one-year mortality rate associated of ICH patients is reported to be 52.2% (11). Therefore, the early recognition and management of ICH are crucial for AIS patients receiving intravenous alteplase. Numerous models for predicting ICH risk in AIS patients treated with intravenous alteplase have been developed worldwide. The purpose of this study is to thoroughly review and systematically summarize and compare these risk prediction models for ICH (including asymptomatic and symptomatic intracranial hemorrhage), aiming to enhance their construction and application in the management of AIS patients treated with intravenous alteplase.
Methods and analysis
This research protocol is registered in the International Prospective Register of Systematic Reviews (PROSPERO) with the registration number CRD42023415649.
Search strategy
A literature search was conducted in the following databases: PubMed, Embase, Web of Science, and the Cochrane Library. The search terms included “ischemic stroke,” “intracranial hemorrhage,” “alteplase,” and “factor.” Our complete search string can be found in Supplementary material S1. The search deadline was April 7, 2023.
Eligibility criteria
The inclusion criteria for literature are: (1) type of research, either cohort study or case–control study; (2) participants, patients treated with alteplase after an acute ischemic stroke (AIS) who experienced intracranial hemorrhage, including asymptomatic and symptomatic intracranial hemorrhage; (3) studies providing relevant content on model construction and validation. The exclusion criteria are: (1) lack of practicality of predictive factors in clinical practice; (2) inability to obtain the full text; (3) duplicate publications.
Literature screening and data extraction
Two researchers independently screened the literature and conducted cross-checks. Disagreements were resolved by consulting a third researcher. Information from the included literature was extracted regarding basic information, model performance, model composition, etc., as shown in Tables 1, 2.
Statistical analysis
A meta-analysis was performed using Stata (version 13.0). The relationship between risk factors and ICH in patients with AIS receiving intravenous alteplase treatment was explored through the odds ratio (OR) and corresponding 95% confidence interval (CI). Heterogeneity between studies was detected using the Q-test, and appropriate effect models were selected. A sensitivity analysis was conducted by sequentially excluding studies. If Begg’s test and/or Egger’s test (p < 0.05) indicated publication bias, the trim-and-fill method was employed for reassessment.
Bias risk assessment
Two researchers used the Prediction Model Risk of Bias Assessment Tool (PROBAST) (24) to evaluate the included study’s bias risk and consulted a third researcher in cases of disagreement. PROBAST evaluates the risk of bias and the applicability of the model across four domains: participants, predictors, outcomes, and analysis.
Predictive performance
The predictive performance of the model was evaluated through discrimination and calibration. The discrimination index is the Area Under the Curve (AUC), with an AUC ≥ 0.7 indicating good discrimination of the model, and externally tested AUC being more convincing. Calibration indicators include the Hosmer-Lemeshow test and calibration plots. A model is considered well-fitted if the Hosmer-Lemeshow test yields a p-value >0.05, or if the calibration plot’s slope is close to 1.
Results
Screening process and results
The researchers initially identified 657 studies. After screening, 13 studies (10, 12–23) were included in the final analysis. Details are provided in Figure 1.
General information on included studies
A total of thirteen risk prediction models for intracranial hemorrhage (ICH) in patients with acute ischemic stroke (AIS) receiving intravenous alteplase treatment were included. Of these, three were conducted in multiple countries (12, 13, 15), six in China (10, 19–23), two in Italy (17, 18), one in America (14), and one in Thailand (16). Regarding the type of research, one was a prospective cohort study (10), and the others were retrospective. The earliest included publication year was 2008 (12), with four articles published in the past three years (10, 21–23). All studies defined participants as patients treated with alteplase for AIS; six specified participants as 18 years or older (10, 17, 20–23), demonstrating high homogeneity in the studies (Table 1).
Modeling and verification
In these studies, the modeling sample size ranged from 131 to 13,908, with the incidence of ICH varying from 3.97 to 21.25%. For modeling methods, one study (23) employed machine learning (ML), while the remaining 12 studies (10, 12–22) used single-factor analysis combined with logistic regression. Regarding model validation, two studies (14, 22) used both internal and external validation, seven studies (13, 16, 17, 19–21, 23) used internal validation, and three studies (10, 15, 18) used only external validation (Table 1).
Predictors and assignment
The number of predictive factors in the models varied, with the maximum being 10 (17) and the minimum three (25). Common predictors of ICH in AIS patients treated with intravenous alteplase were NIHSS (n = 13), glucose (n = 7), and advanced age (n = 6). For risk factor assignment, 12 studies (10, 12–22) utilized OR values or logistic regression β coefficients to assign weights to predictors. One study (23) used machine learning for weight allocation, as detailed in Table 2.
Meta-analysis for risk factors
A meta-analysis was conducted for NIHSS, glucose, and advanced age. Some studies did not provide effective OR values and 95% CIs, hence they were excluded from the meta-analysis. The results indicated that NIHSS, glucose, and advanced age are independent risk factors for ICH in AIS patients treated with intravenous alteplase (Table 3).
Nine studies (10, 13, 14, 16, 18–22) examined the impact of NIHSS on ICH in patients treated with intravenous alteplase post-AIS (heterogeneity: p = 0.001, I2 = 84.2%). There was a significant difference between the groups (95% CI: 1.09–1.25, p < 0.0001; Figure 2). A subgroup meta-analysis based on sample size was conducted due to significant differences in sample sizes included. The meta-analysis revealed NIHSS as an independent risk factor for ICH in all patient subgroups (Table 4). Sensitivity analysis indicated that excluding any study did not significantly alter the meta-analysis results (Figure 3). Begg’s test (p < 0.05) and Egger’s test (p < 0.05) suggested publication bias (Figures 4A,B). The trim-and-fill method was used to correct for publication bias. After three iterations using the linear method, the adjusted OR remained significant (OR = 1.096, 95% CI 1.013–1.185, p < 0.05), indicating the reliability of the results (Table 4).
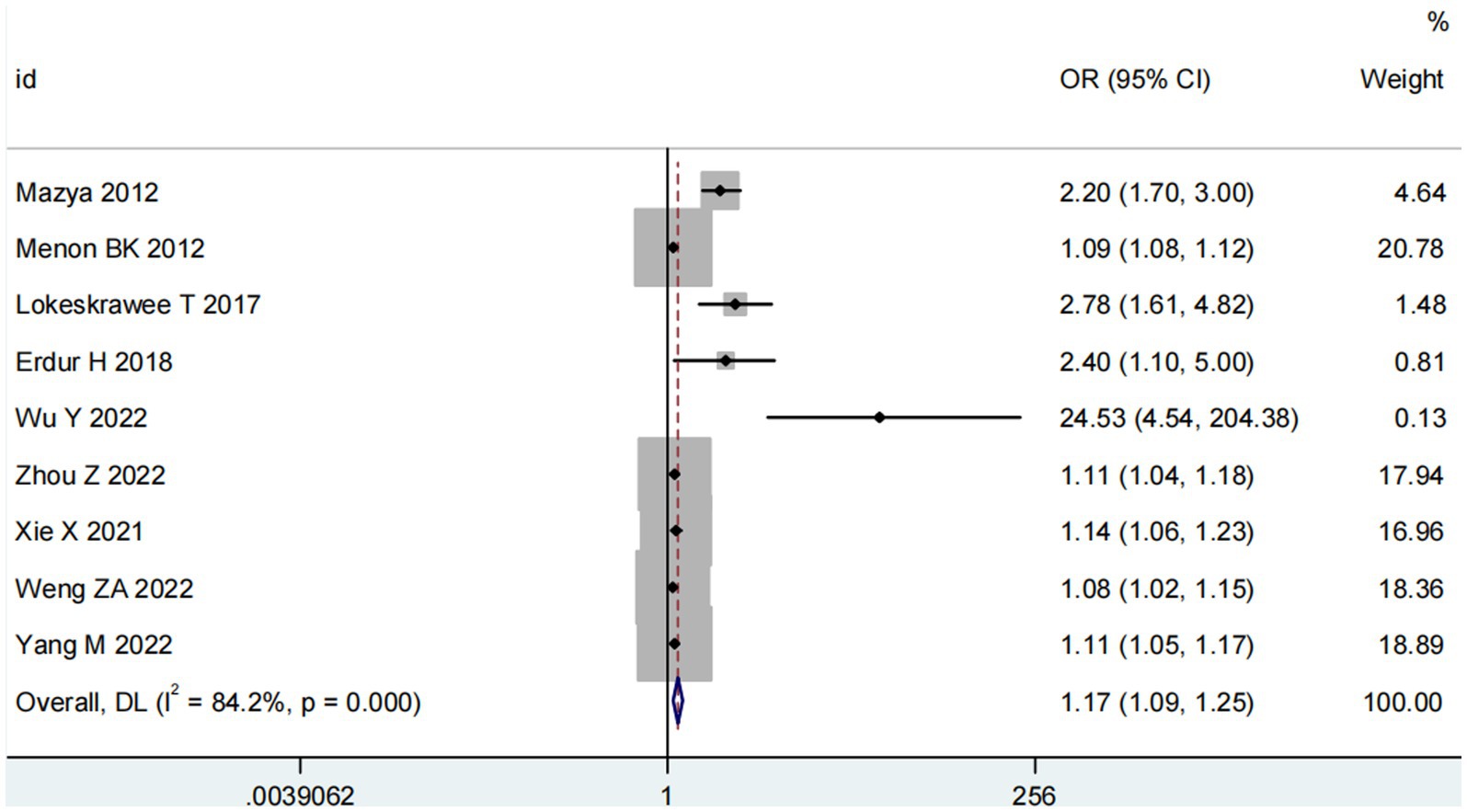
Figure 2. Meta-analysis of NIHSS on ICH in patients receiving intravenous alteplase therapy after AIS.
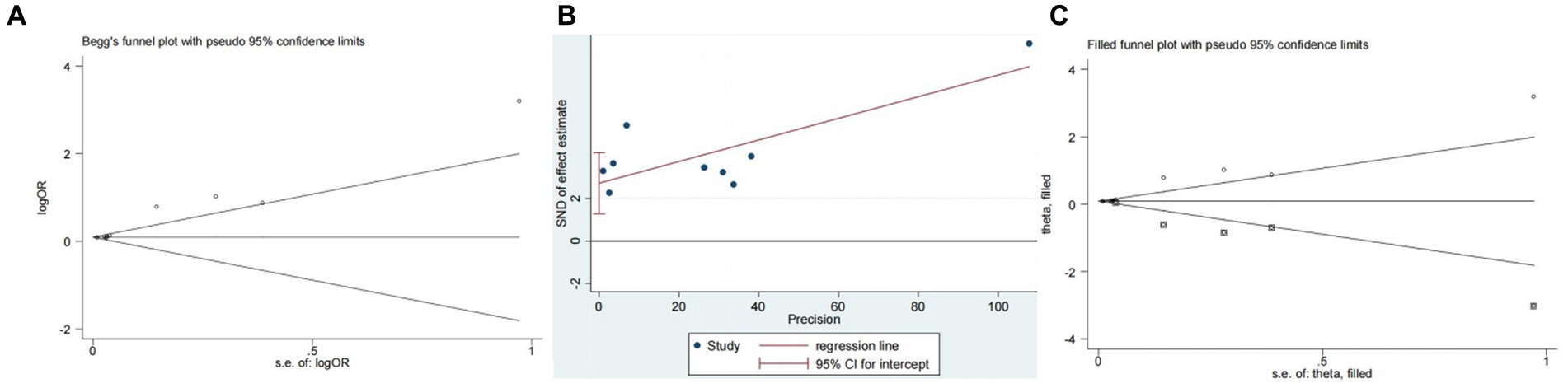
Figure 4. Plots for publication bias test in meta-analysis for the association between NIHSS and ICH. (A) Begg’s funnel plot; (B) Egger’s publication bias plot; (C) Filled funnel plot.
Four studies (13, 14, 18, 20) explored the impact of glucose on ICH in patients receiving intravenous alteplase post-AIS (heterogeneity: p = 0.001, I2 = 93.9%). A significant difference was observed between groups (95% CI: 1.09–2.17, p < 0.05; Figure 5). Sensitivity analysis confirmed the robustness of the result (Figure 6). Begg’s test (p > 0.05) and Egger’s test (p > 0.05) showed no publication bias (Figures 7A,B).
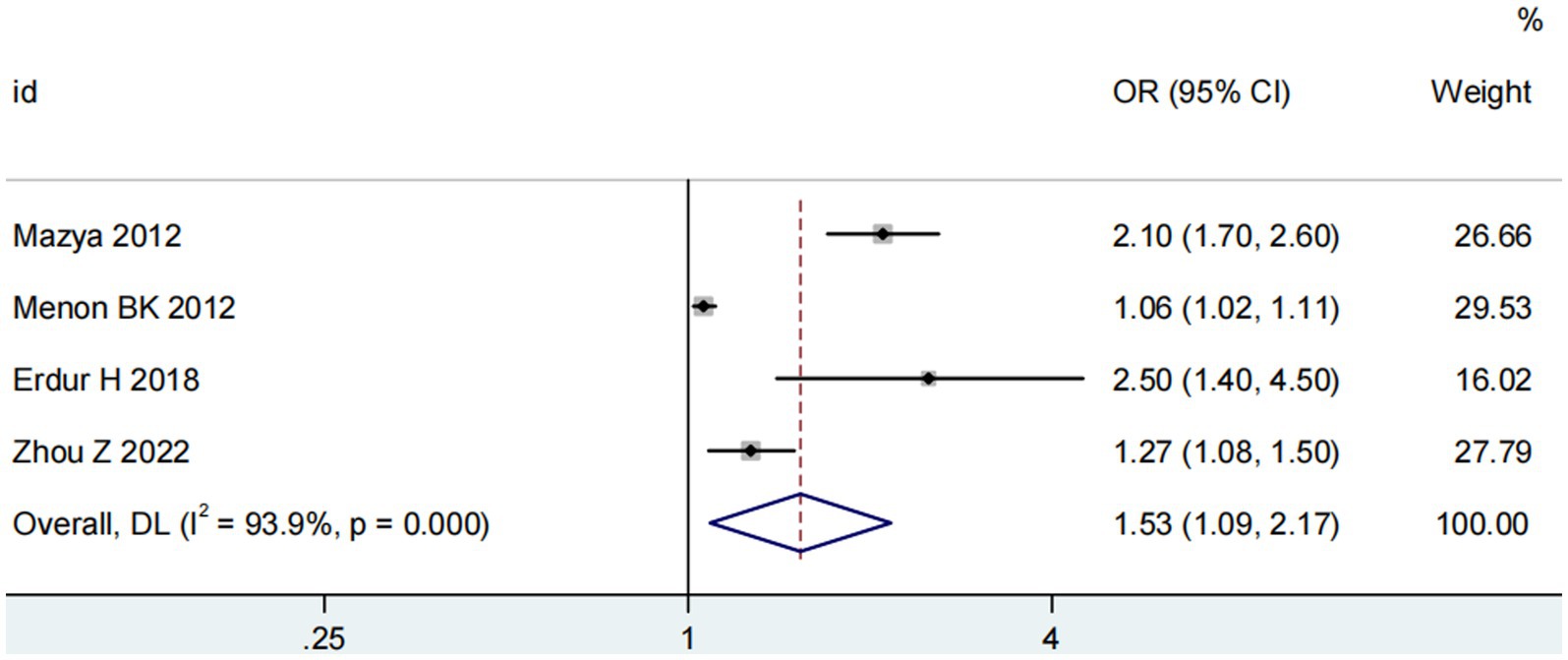
Figure 5. Meta-analysis of glucose on ICH in patients receiving intravenous alteplase therapy after AIS.
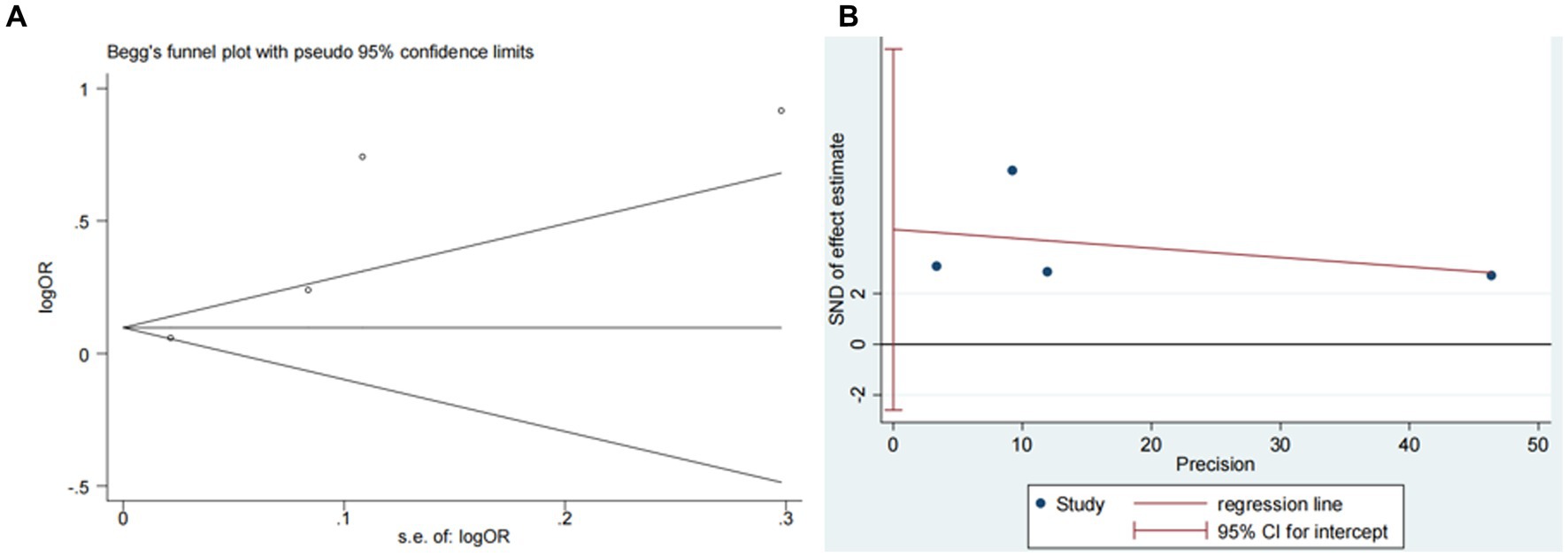
Figure 7. Plots for publication bias test in meta-analysis for the association between glucose and ICH. (A) Begg’s funnel plot; (B) Egger’s publication bias plot.
Three studies (13, 14, 18) assessed the impact of advanced age on ICH in patients treated with intravenous alteplase post-AIS (heterogeneity: p = 0.005, I2 = 80.9%). The difference was statistically significant (95% CI: 1.15–1.94, p < 0.05; Figure 8). Sensitivity analysis showed that excluding any study did not change the results (Figure 9). Begg’s test (p > 0.05) and Egger’s test (p > 0.05) indicated no significant publication bias (Figures 10A,B).
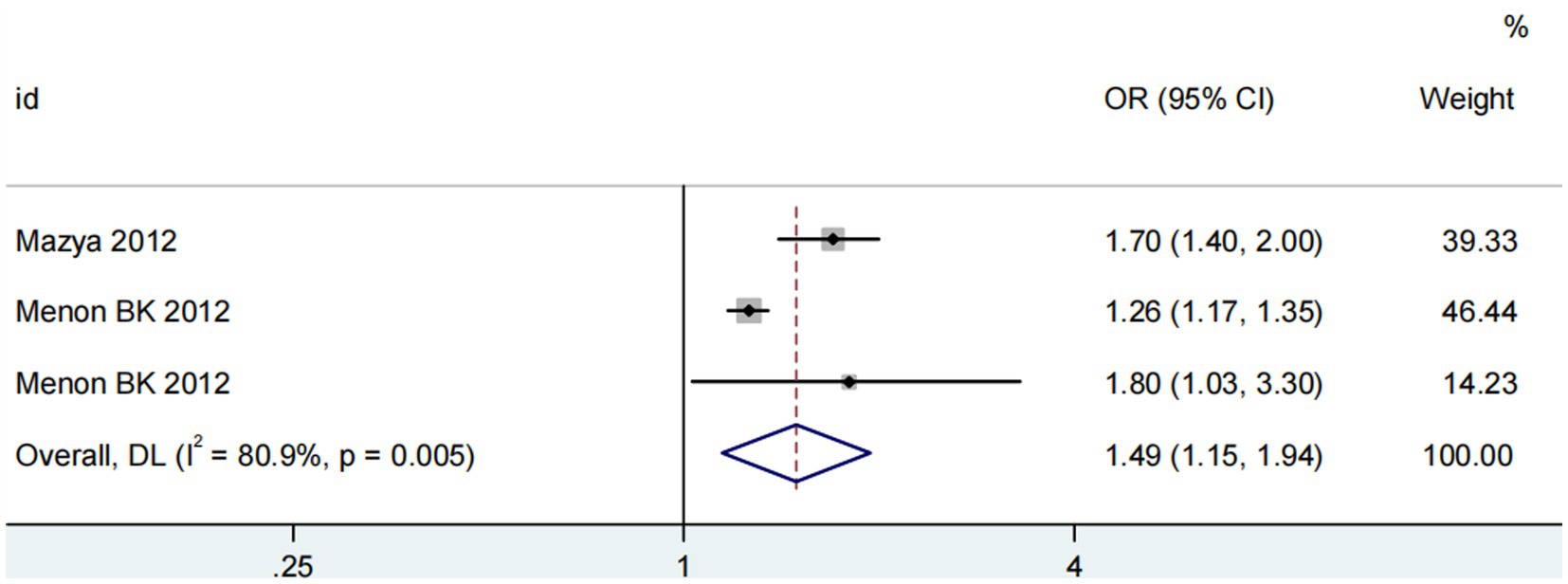
Figure 8. Meta-analysis of advanced age on ICH in patients receiving intravenous alteplase therapy after AIS.
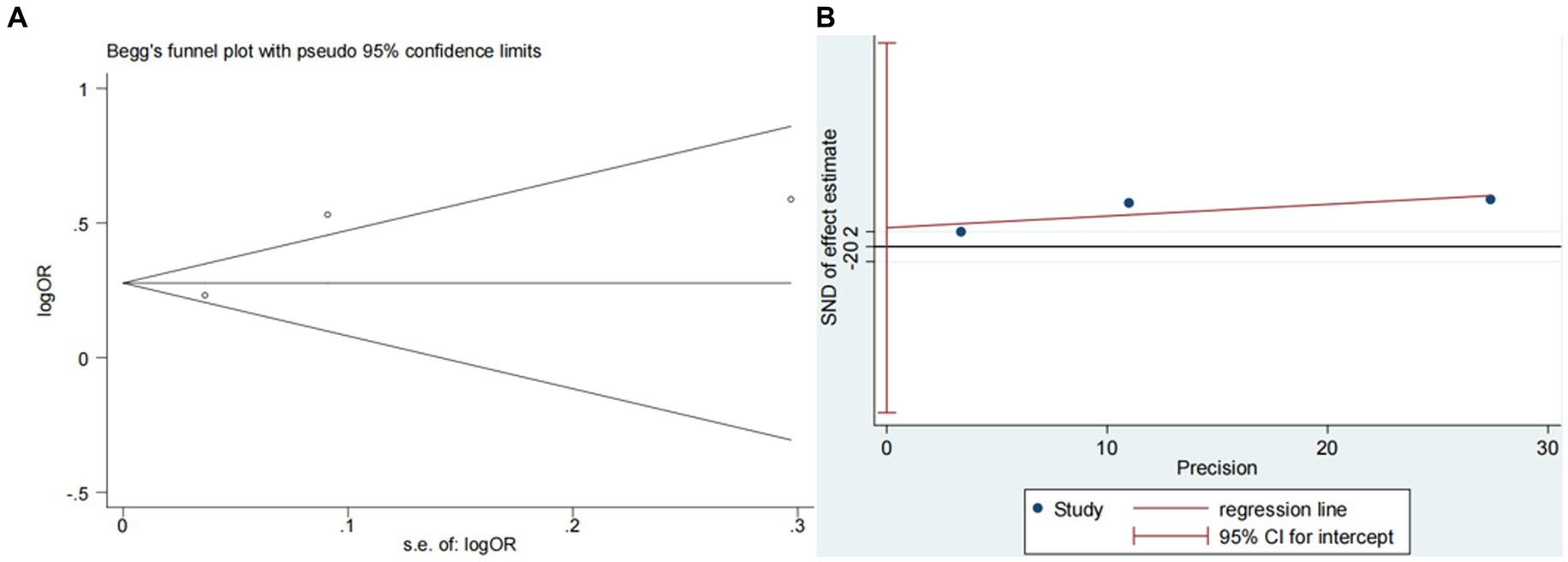
Figure 10. Plots for publication bias test in meta-analysis for the association between advanced age and ICH. (A) Begg’s funnel plot; (B) Egger’s publication bias plot.
Methodological quality evaluation
Among the thirteen pieces of literature included, the bias risk assessment results indicated a high risk for all, primarily due to participant selection and statistical analysis aspects. However, the applicability evaluation results for all the studies were considered low-risk (Table 5).
Predictive performance evaluation
Regarding discrimination, four studies reported AUCs less than 0.7 during model establishment or validation, suggesting suboptimal model performance (12–14, 17). Strbian et al. (15, 18) reported a modeling AUC of 0.77. Five studies (16, 19–21, 23) achieved AUCs greater than 0.7 in both modeling and internal validation. Wu et al. constructed models with AUCs exceeding 0.95 (19), and two studies (10, 22) reported AUCs greater than 0.7 in both modeling and external validation. For calibration, six studies (10, 13–17) used the Hosmer-Lemeshow test with all results indicating well-fitting models (p > 0.05). The calibration slopes in two studies (19, 22) were close to 1, and the model by Zhou et al. (20) showed good performance by both criteria. Based on these two indicators, the model by Weng ZA et al. (22) is considered to have exceptional performance.
Discussion
Overall, the development of risk prediction models for intracranial hemorrhage (ICH) in patients with acute ischemic stroke (AIS) receiving intravenous alteplase treatment is still in its early stages. The research spans a considerable time frame, with a primary focus on America, Thailand, Italy, and China. Most of the models lack external validation and have not yet been implemented clinically.
Discussion on overall bias risk
The risk of bias in prediction models is closely associated with participants, predictors, outcomes, and analysis. All 13 articles included in our study exhibited a high risk of bias (10, 12–23). According to PROBAST, data from prospective cohort studies are considered more reliable than those from retrospective cohort studies (24). However, our study included only one prospective cohort study (10). PROBAST also stipulates that to avoid overfitting, the modeling sample size should include more than 20 events per variable (EPV), and the validation sample size should comprise at least 100 subjects (26). Seven studies did not meet this standard, increasing the risk of model bias (10, 15, 19–23). Regarding the treatment of independent variables, several studies (12–14, 16–20) simplified continuous variables into categorical ones, reducing information efficiency and potentially lowering model performance. As for missing data, three studies (12, 13, 18) failed to address this issue, while the others (10, 14–17, 19–23) excluded samples with missing data. Most studies (10, 12–22) selected factors through uni-variate analysis, and five (13, 15, 17, 18, 21) modified the significance level in this analysis. However, uni-variate analysis can overlook col-linearity between independent variables, leading to the selection of inappropriate factors. Therefore, the Transparent Reporting of a Multivariate Prediction Model for Individual Prognosis or Diagnosis (TRIPOD) recommends adjusting the significance level of uni-variate analysis or employing stepwise regression (27). In terms of model performance, ten studies (10, 13–17, 19, 20, 22, 23) reported both discrimination and calibration. Discrimination is measured by the Area Under Curve (AUC). Calibration is typically assessed using Hosmer-Lemeshow tests and calibration plots, though six studies only used Hosmer-Lemeshow tests (10, 13–17). The p-value in Hosmer-Lemeshow tests does not fully represent model calibration (27); calibration plots are recommended. Two studies (19, 22) utilized calibration plots, and one study (20) used both methods. Model performance may display optimistic bias due to over-fitting, underscoring the need for effective validation methods. Seven studies (13, 16, 17, 19–21, 23) only conducted internal validation, with four (13, 17, 20, 23) using the less efficient split-sample method. It is advised to use bootstrap sampling or cross-validation for internal validation; two studies (16, 21) used bootstrap sampling, and one (19) combined randomized splitting with bootstrap sampling. PROBAST (24) indicates that the absence of external validation in predictive model development leads to a high overall bias risk. Only five studies (10, 14, 15, 18, 22) employed external validation, but one (15) did not report the AUC for external validation. Regarding model applicability: All studies defined participants as patients treated with alteplase for AIS, aligning with our study design. Additionally, the definition, assessment, and timing of evaluating predictive factors in all models are consistent with our study. Lastly, the determination of ICH in all included studies matches our study, confirming the excellent overall applicability of the studies included (10, 12–23).
Prediction factor analysis
The models included in this study encompass a variety of predictive factors, including general data, disease-related information, biochemical indicators, and imaging results. While each model comprises different predictive factors, common elements are present. Notably, NIHSS, glucose, and advanced age were found to be strongly associated with intracranial hemorrhage (ICH) in patients with acute ischemic stroke (AIS) receiving intravenous alteplase treatment. Meta-analysis confirms that these factors are independent risk factors, aligning with the findings of numerous related studies (28–30). The National Institutes of Health Stroke Scale (NIHSS) is widely used for assessing stroke severity and is endorsed by the American Stroke Association (ASA) guidelines as an effective tool for emergency departments to evaluate stroke severity (31, 32). All models included in our study featured NIHSS, and meta-analysis validated that a higher NIHSS score is an independent risk factor for ICH. Whiteley WN et al. conducted a meta-analysis of 55 articles on ICH risk factors in AIS patients treated with intravenous alteplase, concluding that a higher NIHSS score is a risk factor for ICH (33). Teekaput et al.’s retrospective study on 725 AIS patients who received alteplase treatment demonstrated that the incidence of ICH in patients with a higher baseline NIHSS was 1.9 times that of the control group (11). Patients with AIS and a high baseline NIHSS typically have larger infarct areas and more extensive vascular damage, increasing their susceptibility to ICH following alteplase treatment (32, 34). Therefore, careful consideration of treatment methods, enhanced monitoring, and proactive intervention are crucial for patients with high baseline NIHSS scores to effectively prevent ICH. Glucose is another independent predictor of ICH, consistent with previous research findings. Hyperglycemia is common in the acute phase of AIS, often resulting from stress-induced increases in cortisol and catecholamine levels following ischemic injury. Elevated glucose levels are associated with stroke severity and adverse outcomes (35, 36). Advanced age is also a significant predictor. Dong S. et al.’s meta-analysis of 25 cohort studies established that advanced age is an independent predictor of ICH in AIS patients (37). This is particularly relevant for patients over 80 years old receiving alteplase treatment (38). As patients age, their overall physical condition deteriorates, the prevalence of cardiovascular diseases rises, blood vessel elasticity decreases, and brain parenchyma undergoes degenerative changes, all of which contribute to a higher risk of ICH after thrombolysis (38, 39).
Advantages and limitations
Advantages
(1) This study systematically integrates recent risk prediction models for intracranial hemorrhage (ICH) in patients with acute ischemic stroke (AIS) receiving intravenous alteplase treatment, emphasizing the modeling method, model performance, predictive factors, and factor assignment. (2) It employs PROBAST to evaluate the quality of these models, analyze risk sources, and provide a reference for future research. (3) The study enhances the reliability of its conclusions by supplementing quantitative analysis through meta-analysis.
Limitations
(1) The study is limited to literature published in English. (2) There are variations in the study populations and the standards for defining ICH among the included studies. (3) Some models lack validation, necessitating further research to verify their applicability.
Conclusion
In summary, there has been a steady increase in the number of risk prediction models for ICH in AIS patients treated with intravenous alteplase. However, the performance of these models varies. The 13 models included in this study present a high risk of bias due to statistical analysis issues, but they generally exhibit a low risk regarding overall applicability, which is beneficial for the early screening of high-risk patients. Due to the high overall bias risk in the included studies, it is not advisable to directly apply these predictive models in clinical practice. Medical professionals should aim to facilitate the application and generalization of existing models through external validation involving multiple centers and large samples or conduct large-sample prospective research to develop new predictive models in accordance with TRIPOD and PROBAST guidelines.
Data availability statement
The original contributions presented in the study are included in the article/Supplementary material, further inquiries can be directed to the corresponding author.
Author contributions
YH and PT conceived the study idea, designed the study, conducted database searches, and wrote the manuscript. CY and CZ interpreted the data and provided additional relevant information. QZ and YH analyzed the quality of each included study and confirmed the analysis results. DL and PT oversaw the methodology, and reviewed and revised the manuscript. All authors have read and approved the final manuscript.
Funding
This research was funded by the Jiangxi Province Education Department’s project (GJJ210183). No commercial entities were involved. The funding played no role in influencing the design and execution of the study, the collection, management, analysis, and interpretation of the data, the preparation, review, or approval of the manuscript, or the decision to submit the manuscript for publication.
Conflict of interest
The authors declare that the research was conducted in the absence of any commercial or financial relationships that could be construed as a potential conflict of interest.
Publisher’s note
All claims expressed in this article are solely those of the authors and do not necessarily represent those of their affiliated organizations, or those of the publisher, the editors and the reviewers. Any product that may be evaluated in this article, or claim that may be made by its manufacturer, is not guaranteed or endorsed by the publisher.
Supplementary material
The Supplementary material for this article can be found online at: https://www.frontiersin.org/articles/10.3389/fneur.2023.1224658/full#supplementary-material
Supplementary Material 1 | Complete search strategy.
Supplementary Material 2 | Subgroup meta-analysis of NIHSS on ICH in patients receiving intravenous alteplase therapy after AIS.
References
1. GBD 2019 Stroke Collaborators. Global, regional, and national burden of stroke and its risk factors, 1990-2019: a systematic analysis for the global burden of disease study 2019. Lancet Neurol. (2021) 20:795–820. doi: 10.1016/S1474-4422(21)00252-0
2. Feigin, VL, Brainin, M, Norrving, B, Martins, S, Sacco, RL, Hacke, W, et al. World stroke organization (WSO): global stroke fact sheet 2022. Int J Stroke. (2022) 17:18–29. doi: 10.1177/17474930211065917
3. Wang, YJ, Li, ZX, Gu, HQ, Zhai, Y, Zhou, Q, Jiang, Y, et al. China stroke statistics: an update on the 2019 report from the National Center for healthcare quality Management in Neurological Diseases, China National Clinical Research Center for neurological diseases, the Chinese Stroke Association, National Center for chronic and non-communicable disease control and prevention, Chinese Center for Disease Control and Prevention and institute for global neuroscience and stroke collaborations. Stroke Vasc Neurol. (2022) 7:415–50. doi: 10.1136/svn-2021-001374
4. Zubair, AS, and Sheth, KN. Emergency Care of Patients with acute ischemic stroke. Neurol Clin. (2021) 39:391–404. doi: 10.1016/j.ncl.2021.02.001
5. Herpich, F, and Rincon, F. Management of Acute Ischemic Stroke. Crit Care Med. (2022) 48:1654–63. doi: 10.1097/CCM.0000000000004597
6. Powers, WJ, Rabinstein, AA, Ackerson, T, Adeoye, OM, Bambakidis, NC, Becker, K, et al. Guidelines for the early Management of Patients with Acute Ischemic Stroke: 2019 update to the 2018 guidelines for the early Management of Acute Ischemic Stroke: a guideline for healthcare professionals from the American Heart Association/American Stroke Association. Stroke. (2019) 50:e344–418. doi: 10.1161/STR.0000000000000211
7. Tsivgoulis, G, Katsanos, AH, Sandset, EC, Turc, G, Nguyen, TN, Bivard, A, et al. Thrombolysis for acute ischaemic stroke: current status and future perspectives. Lancet Neurol. (2023) 22:418–29. doi: 10.1016/S1474-4422(22)00519-1
8. Brondani, R, de Almeida, AG, Cherubini, PA, Secchi, TL, de Oliveira, MA, Martins, SCO, et al. Risk factors for epilepsy after thrombolysis for ischemic stroke: a cohort study. Front Neurol. (2020) 10:1256. doi: 10.3389/fneur.2019.01256
9. Zhong, CS, Beharry, J, Salazar, D, Smith, K, Withington, S, Campbell, BCV, et al. Routine use of Tenecteplase for thrombolysis in acute ischemic stroke. Stroke. (2021) 52:1087–90. doi: 10.1161/STROKEAHA.120.030859
10. Yang, M, Zhong, W, Zou, W, Peng, J, and Tang, X. A novel nomogram to predict hemorrhagic transformation in ischemic stroke patients after intravenous thrombolysis. Front Neurol. (2022) 13:913442. doi: 10.3389/fneur.2022.913442
11. Fekete, KE, Héja, M, Márton, S, Tóth, J, Harman, A, Horváth, L, et al. Predictors and long-term outcome of intracranial hemorrhage after thrombolytic therapy for acute ischemic stroke-a prospective single-center study. Front Neurol. (2023) 14:1080046. doi: 10.3389/fneur.2023.1080046
12. Cucchiara, B, Tanne, D, Levine, SR, Demchuk, AM, and Kasner, S. A risk score to predict intracranial hemorrhage after recombinant tissue plasminogen activator for acute ischemic stroke. J Stroke Cerebrovasc Dis. (2008) 17:331–3. doi: 10.1016/j.jstrokecerebrovasdis.2008.03.012
13. Mazya, M, Egido, JA, Ford, GA, Lees, KR, Mikulik, R, Toni, D, et al. Predicting the risk of symptomatic intracerebral hemorrhage in ischemic stroke treated with intravenous alteplase: safe implementation of treatments in stroke (SITS) symptomatic intracerebral hemorrhage risk score. Stroke. (2012) 43:1524–31. doi: 10.1161/STROKEAHA.111.644815
14. Menon, BK, Saver, JL, Prabhakaran, S, Reeves, M, Liang, L, Olson, DM, et al. Risk score for intracranial hemorrhage in patients with acute ischemic stroke treated with intravenous tissue-type plasminogen activator. Stroke. (2012) 43:2293–9. doi: 10.1161/STROKEAHA.112.660415
15. Strbian, D, Engelter, S, Michel, P, Meretoja, A, Sekoranja, L, Ahlhelm, FJ, et al. Symptomatic intracranial hemorrhage after stroke thrombolysis: the SEDAN score. Ann Neurol. (2012) 71:634–41. doi: 10.1002/ana.23546
16. Lokeskrawee, T, Muengtaweepongsa, S, Patumanond, J, Tiamkao, S, Thamangraksat, T, Phankhian, P, et al. Prediction of symptomatic intracranial hemorrhage after intravenous thrombolysis in acute ischemic stroke: the symptomatic intracranial hemorrhage score. J Stroke Cerebrovasc Dis. (2017) 26:2622–9. doi: 10.1016/j.jstrokecerebrovasdis
17. Cappellari, M, Turcato, G, Forlivesi, S, Zivelonghi, C, Bovi, P, Bonetti, B, et al. STARTING-SICH nomogram to predict symptomatic intracerebral hemorrhage after intravenous thrombolysis for stroke. Stroke. (2018) 49:397–404. doi: 10.1161/STROKEAHA.117.018427
18. Erdur, H, Polymeris, A, Grittner, U, Scheitz, JF, Tütüncü, S, Seiffge, DJ, et al. A score for risk of thrombolysis-associated hemorrhage including pretreatment with statins. Front Neurol. (2018) 9:74. doi: 10.3389/fneur.2018.00074
19. Wu, Y, Chen, H, Liu, X, Cai, X, Kong, Y, Wang, H, et al. A new nomogram for individualized prediction of the probability of hemorrhagic transformation after intravenous thrombolysis for ischemic stroke patients. BMC Neurol. (2020) 20:426. doi: 10.1186/s12883-020-02002-w
20. Zhou, Z, Yin, X, Niu, Q, Liang, S, Mu, C, and Zhang, Y. Risk factors and a nomogram for predicting intracranial hemorrhage in stroke patients undergoing thrombolysis. Neuropsychiatr Dis Treat. (2020) 16:1189–97. doi: 10.2147/NDT.S250648
21. Xie, X, Yang, J, Ren, L, Hu, S, Lian, W, Xiao, J, et al. Nomogram to predict symptomatic intracranial hemorrhage after intravenous thrombolysis in acute ischemic stroke in Asian population. Curr Neurovasc Res. (2021) 18:543–51. doi: 10.2174/1567202619666211223150907
22. Weng, ZA, Huang, XX, Deng, D, Yang, ZG, Li, SY, Zang, JK, et al. A new nomogram for predicting the risk of intracranial hemorrhage in acute ischemic stroke patients after intravenous thrombolysis. Front Neurol. (2022) 13:774654. doi: 10.3389/fneur.2022.774654
23. Xu, Y, Li, X, Wu, D, Zhang, Z, and Jiang, A. Machine learning-based model for prediction of hemorrhage transformation in acute ischemic stroke after Alteplase. Front Neurol. (2022) 13:897903. doi: 10.3389/fneur.2022.897903
24. Wolff, RF, Moons, KGM, Riley, RD, Whiting, PF, Westwood, M, Collins, GS, et al. PROBAST: a tool to assess the risk of Bias and applicability of prediction model studies. Ann Intern Med. (2019) 170:51–8. doi: 10.7326/M18-1376
25. Moons, KGM, Wolff, RF, Riley, RD, Whiting, PF, Westwood, M, Collins, GS, et al. PROBAST: a tool to assess risk of Bias and applicability of prediction model studies: explanation and elaboration. Ann Intern Med. (2019) 170:W1–W33. doi: 10.7326/M18-1377
26. Collins, GS, Reitsma, JB, Altman, DG, and Moons, KG. Transparent reporting of a multivariable prediction model for individual prognosis or diagnosis (TRIPOD): the TRIPOD statement. BMJ. (2015) 350:g 7594. doi: 10.1136/bmj.g7594
27. van der Ende, NAM, Kremers, FCC, van der Steen, W, Venema, E, Kappelhof, M, Majoie, CBLM, et al. Symptomatic intracranial hemorrhage after endovascular stroke treatment: external validation of prediction models. Stroke. (2023) 54:476–87. doi: 10.1161/STROKEAHA.122.040065
28. Jiang, Y, Liu, N, Han, J, Li, Y, Spencer, P, Vodovoz, SJ, et al. Diabetes mellitus/Poststroke hyperglycemia: a detrimental factor for tPA thrombolytic stroke therapy. Transl Stroke Res. (2021) 12:416–27. doi: 10.1007/s12975-020-00872-3
29. Guo, Y, Yang, Y, Zhou, M, and He, L. Risk factors of haemorrhagic transformation for acute ischaemic stroke in Chinese patients receiving intravenous recombinant tissue plasminogen activator: a systematic review and meta-analysis. Stroke Vasc Neurol. (2018) 3:203–8. doi: 10.1136/svn-2018-000141
30. Cheng, Z, Geng, X, Rajah, GB, Gao, J, Ma, L, Li, F, et al. NIHSS consciousness score combined with ASPECTS is a favorable predictor of functional outcome post endovascular recanalization in stroke patients. Aging Dis. (2021) 12:415–24. doi: 10.14336/AD.2020.0709
31. Kwah, LK, and Diong, J. National Institutes of Health stroke scale (NIHSS). J Physiother. (2014) 60:61. doi: 10.1016/j.jphys.2013.12.012
32. Whiteley, WN, Slot, KB, Fernandes, P, Sandercock, P, and Wardlaw, J. Risk factors for intracranial hemorrhage in acute ischemic stroke patients treated with recombinant tissue plasminogen activator: a systematic review and meta-analysis of 55 studies. Stroke. (2012) 43:2904–9. doi: 10.1161/STROKEAHA.112.665331
33. Teekaput, C, Thiankhaw, K, Tanprawate, S, Teekaput, K, and Chai-Adisaksopha, C. Outcomes of asymptomatic recombinant tissue plasminogen activator associated intracranial hemorrhage. PLoS One. (2022) 17:e0272257. doi: 10.1371/journal.pone.0272257
34. Lu, Y, Ma, X, Zhou, X, and Wang, Y. The association between serum glucose to potassium ratio on admission and short-term mortality in ischemic stroke patients. Sci Rep. (2022) 12:8233. doi: 10.1038/s41598-022-12393-0
35. Dungan, KM, Braithwaite, SS, and Preiser, JC. Stress hyperglycaemia. Lancet. (2009) 373:1798–807. doi: 10.1016/S0140-6736(09)60553-5
36. Dong, S, Yu, C, Wu, Q, Xia, H, Xu, J, Gong, K, et al. Predictors of symptomatic intracranial hemorrhage after endovascular Thrombectomy in acute ischemic stroke: a systematic review and meta-analysis. Cerebrovasc Dis. (2022) 52:363–75. doi: 10.1159/000527193
37. Mione, G, Ducrocq, X, Thilly, N, Lacour, JC, Vespignani, H, and Richard, S. Outcome of intravenous recombinant tissue plasminogen activator for acute ischemic stroke in patients aged over 80 years. Geriatr Gerontol Int. (2016) 16:843–9. doi: 10.1111/ggi.12565
38. Tong, X, George, MG, Yang, Q, and Gillespie, C. Predictors of in-hospital death and symptomatic intracranial hemorrhage in patients with acute ischemic stroke treated with thrombolytic therapy: Paul Coverdell acute stroke registry 2008-2012. Int J Stroke. (2014) 9:728–34. doi: 10.1111/ijs.1215
Keywords: ischemic stroke, tissue plasminogen activator, intracranial hemorrhage, models, systematic review
Citation: Hua Y, Yan C, Zhou C, Zheng Q, Li D and Tu P (2024) Risk prediction models for intracranial hemorrhage in acute ischemic stroke patients receiving intravenous alteplase treatment: a systematic review. Front. Neurol. 14:1224658. doi: 10.3389/fneur.2023.1224658
Edited by:
Slaven Pikija, University Hospital Salzburg, AustriaReviewed by:
László Horváth, University of Debrecen, HungaryQinJian Sun, Shandong University, China
Copyright © 2024 Hua, Yan, Zhou, Zheng, Li and Tu. This is an open-access article distributed under the terms of the Creative Commons Attribution License (CC BY). The use, distribution or reproduction in other forums is permitted, provided the original author(s) and the copyright owner(s) are credited and that the original publication in this journal is cited, in accordance with accepted academic practice. No use, distribution or reproduction is permitted which does not comply with these terms.
*Correspondence: Ping Tu, VHVwaW5nb29AMTI2LmNvbQ==