- 1Institute of Psychiatry, Psychology and Neuroscience, Department of Basic and Clinical Neuroscience, Division of Neuroscience, King’s College London, London, United Kingdom
- 2King’s College Hospital NHS Foundation Trust, London, United Kingdom
- 3Department of Neurology, David Geffen School of Medicine, University of California, Los Angeles, Los Angeles, CA, United States
- 4Parkinson’s Disease Research, Education and Clinical Centers, Greater Los Angeles Veterans Affairs Medical Center, Los Angeles, CA, United States
- 5Faculty of Medicine, Transilvania University of Braşov, Brașov, Romania
- 6Department of Neurology, County Clinic Hospital, Brașov, Romania
- 7Department of Neurology, Neurosurgery and Medical Genetics, Federal State Autonomous Educational Institution of Higher Education “N.I. Pirogov Russian National Research Medical University” of the Ministry of Health of the Russian Federation, Moscow, Russia
- 8Department of Neurodegenerative Diseases, Federal State Budgetary Institution “Federal Center of Brain Research and Neurotechnologies” of the Federal Medical Biological Agency, Moscow, Russia
- 9Department of Neurology, University Hospital, Lund, Sweden
- 10Older Person’s Medical Clinic, Broadmeadow, NSW, Australia
- 11Center for Parkinson’s Disease and Movement Disorders, Manipal Hospital, Karnataka, India, Bangalore
- 12Parkinson’s Disease and Movement Disorders Clinic, Bangalore, Karnataka, India
- 13Department of Neurology, Nizam’s Institute of Medical Sciences, Autonomous University, Hyderabad, India
- 14Neurology Department, Medanta, Gurugram, India
We have recently published the notion of the “vitals” of Parkinson’s, a conglomeration of signs and symptoms, largely nonmotor, that must not be missed and yet often not considered in neurological consultations, with considerable societal and personal detrimental consequences. This “dashboard,” termed the Chaudhuri’s vitals of Parkinson’s, are summarized as 5 key vital symptoms or signs and comprise of (a) motor, (b) nonmotor, (c) visual, gut, and oral health, (d) bone health and falls, and finally (e) comorbidities, comedication, and dopamine agonist side effects, such as impulse control disorders. Additionally, not addressing the vitals also may reflect inadequate management strategies, leading to worsening quality of life and diminished wellness, a new concept for people with Parkinson’s. In this paper, we discuss possible, simple to use, and clinically relevant tests that can be used to monitor the status of these vitals, so that these can be incorporated into clinical practice. We also use the term Parkinson’s syndrome to describe Parkinson’s disease, as the term “disease” is now abandoned in many countries, such as the U.K., reflecting the heterogeneity of Parkinson’s, which is now considered by many as a syndrome.
Introduction
First described by James Parkinson’s in 1817 (1), Parkinson’s disease (PD), which should be regarded as a syndrome, is a progressive neurodegenerative condition, whose incidence has risen sharply worldwide, leading some authors to suggest we may be facing a Parkinson’s pandemic (2). Modern concepts of PD (3) extend beyond the well characterized motor symptoms to address nonmotor symptoms (NMS) (4), as well as to include the concept of wellness (5), the latter a marriage of motor, nonmotor, socio-cultural and socio-economic issues. Taking these into account, we recently proposed the concept of the “vitals” of Parkinson’s (6), which are comprised of the crucially important comorbid or related symptoms of PD. Vitals consist of well-established essential motor and nonmotor features of PD, but also include other health factors that are crucial to providing holistic care, encompassing patients’ safety and quality of life (QoL). Often motor and NMS can occupy an entire consultation, however we bring to attention and stress on the importance of three other vitals (Figure 1), which are often omitted by healthcare professionals and rarely reported by people with Parkinson’s (PwP). When these vitals are missed in clinical practice, it can lead to marked deterioration of patients’ wellness. According to our and our collaborators’ clinical experience across sites in the U.K., U.A.E., and Romania, we have seen limited usage of these vitals even in well-established PD clinics, with consequences such as poor absorption of levodopa (7), or fractures secondary to falls due to non-detection of osteoporosis (8), or potentially dangerous daytime sleepiness and sudden onset of sleep (9).
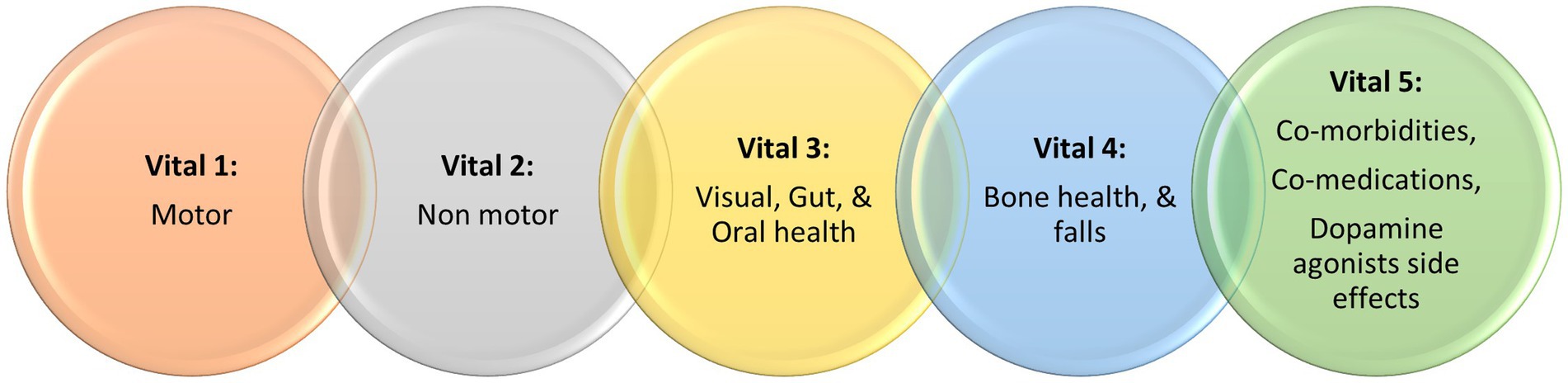
Figure 1. A summary of the Chaudhuri’s Dashboard of Vitals in Parkinson’s, adapted from Chaudhuri et al. (6).
Adopting the new “Chaudhuri’s Dashboard of Vitals in Parkinson’s” as a blueprint (Figure 1), in this review we discuss the tests that can be either be used or have the potential to be used once properly validated, in everyday clinical practice to assess these vitals. Some of clinical tools discussed are already in use for other conditions or clinical purposes, while others are more investigational at present. Figure 2 summarize the clinical tools we discuss in this review, divided into three categories according to the current applicability. It also includes useful clinical scales and questionnaires, which are beyond the scope of this review.
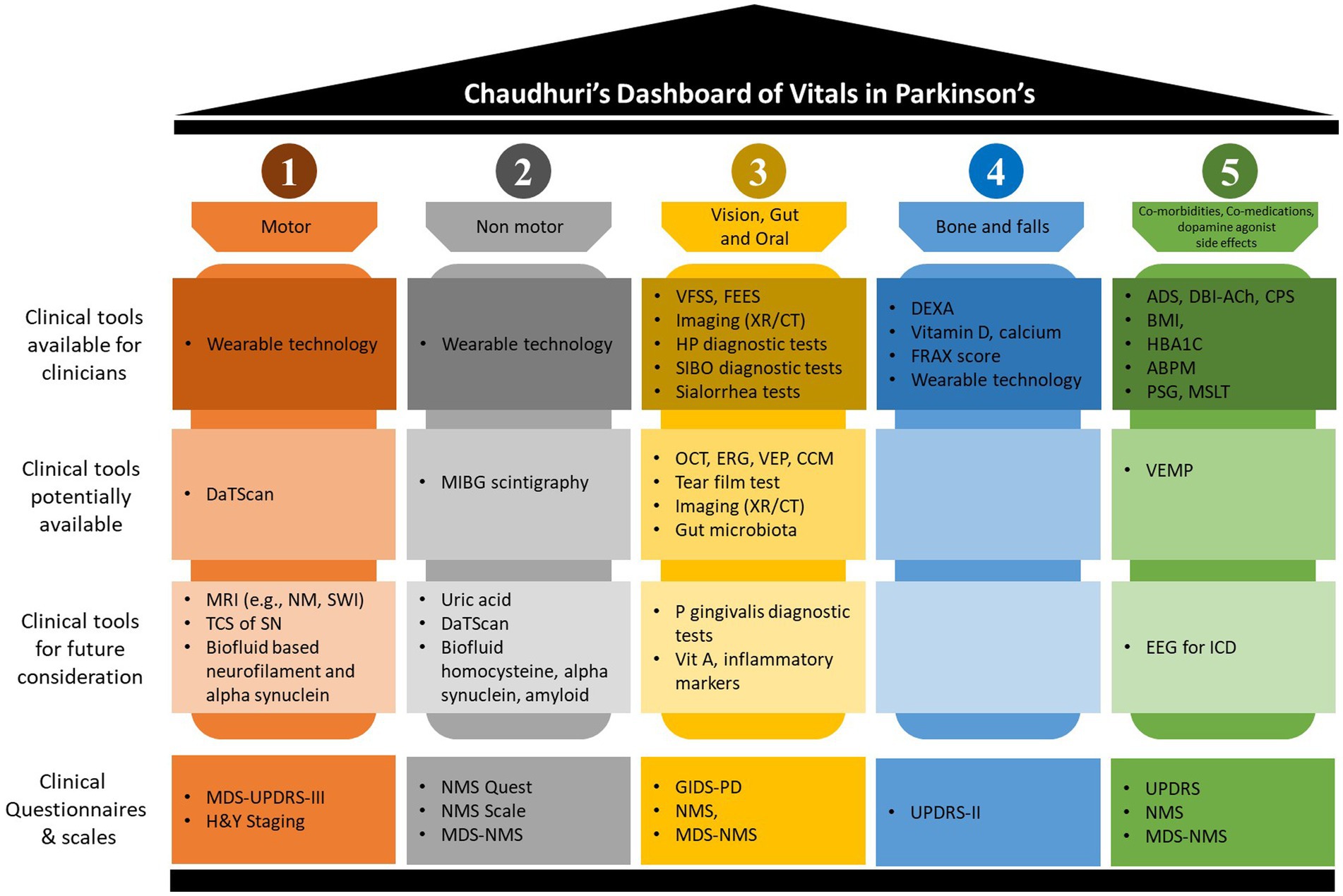
Figure 2. Chaudhuri’s Dashboard of Vitals clinical tools. This figure summarizes discussed clinical tools when applied to the Dashboard of Vitals in Parkinson’s disease. The figure splits the tools into four categories; (1) clinical tools available for clinicians, these are currently available tools with good evidence to application to real-clinical practice; (2) clinical tools potentially available, these are tools which may limited due to resources and should be considered with careful consideration depending on the clinical indications; (3) clinical tools for future consideration, these are tools which may be available or currently being developed but are not solely able to be applied without further evidence for their application in clinical practice; and (4) clinical questionnaires and scales, these are tools which allow for screening and progression monitoring in clinical practice. ABPM, ambulatory blood pressure monitor; ADS, anticholinergic drug scale; BMI, body mass index; CCM, corneal confocal microscopy; CPS, co-morbidities polypharmacy scale; CT, computerized tomography; DaTScan, presynaptic dopamine transporter single-photon emission computerized tomography; DBI-Ach, burden index anticholinergic component; DEXA, dual-energy X-ray absorptiometry; EEG, electroencephalogram; ERG, electroretinography; FEES, fibreoptic endoscopic evaluation of swallowing; FRAX, fracture risk assessment tool; GIDS, gastrointestinal dysfunction scale; H&Y, Hoehn and Yahr; HBA1C, glycated hemoglobin; HP, helicobacter pylori; ICD, impulsive control disorder; MDS, movement disorder society; MIBG, 123I-metaiodobenzylguandine; MRI, magnetic resonance imaging; MSLT, multiple sleep latency tests; NM, neuromelanin; NMS, nonmotor symptoms; OCT, optical coherence tomography; P gingivalis, porphyromonas gingivalis; PD, Parkinson’s disease; PSG, polysomnography; QUEST, questionnaire; SIBO, small intestinal bacterial overgrowth; SN, substantia nigra; SWI, susceptibility weighted imaging; TCS, transcranial sonography; UPDRS, unified Parkinson’s disease rating scale; VEMP, vestibular-evoked myogenic potential; VEP, visual evoked potential; VFSS, videofluoroscopic swallowing study; Vit, vitamin; XR, x-ray.
Dashboard vital: motor
The cardinal motor features of PD include bradykinesia, resting tremor, rigidity, and postural instability. Our understanding of PD has evolved to appreciate there are different motor subtypes, such as tremor-dominant (TD), postural instability and gait-disturbance (PIGD), akinetic-rigid (AR) subtypes, allowing for a more personalized approach in the clinical practice (10). These motor phenotypes can be differentiated through algorithms developed from the Unified Parkinson’s Disease Rating Scale (UPDRS) (11) or the revised Movement Disorder Society (MDS-UPDRS) (12), or with the use of specific motor symptoms scales such as the Modified Bradykinesia Rating Scale (13), alongside with the application of wearable technology (14). The motor subtypes are in fact characterized by a different progression, different NMS burden and different response to treatment, which might reflect a variable involvement of non-dopamine neurotransmitters (15–19).
Clinical tests for motor
Imaging-based clinical tools
Presynaptic dopamine transporter (DaT) single-photon emission computerized tomography (DaTScan) reflects the loss of dopaminergic neurons in the substantia nigra (SN), measured as caudate, putamen and striatum binding ratios (20). DaTScan is a validated tool used to support the diagnosis of Parkinson’s and parkinsonism and in PD, it shows dopamine depletion starting posteriorly from the putamen (21). In PD, DaTScan can also correlates with the laterality of symptoms (22), and some studies suggest that the striatal binding ratio has a negative correlation with motor scores (23–25). Additionally, the tracer uptake is more reduced in the AR and PIGD subtypes compared to TD one, supporting the hypothesis that dopamine depletion in the basal ganglia is the main mechanism for this specific subtypes and providing the evidence for a better levodopa response compared to TD one (26–28). Disease progression models also support its role in predicting motor disease progression (24, 29, 30). However, application of the DaTScan in real clinical practice is more difficult, given its cost and availability limitations, and more evidence needs to be provided on its role in predicting disease trajectory or its utility in management of dopamine-replacing treatments.
A promising tool to investigate the motor vital, despite still under investigation, is brain magnetic resonance imaging (MRI) scan paradigms. It has been shown in fact that specific sequences, such as neuromelanin or susceptibility-weighted imaging and diffusion imaging of the SN, represent a good biomarker of disease progression and correlate with motor symptom burden (31–35). However, this remains investigational and not widely available for clinical application.
Finally, given its practicality, transcranial sonography of the SN, should be taken into consideration for future possible clinical applications, being its usefulness not only confined to the differential diagnosis among PD and controls. It has in fact being demonstrated that the SN hyperechogenecity correlates to motor disability and gait impairment in PD (36). However, evidence in its role in monitoring the motor vital are still poor, it is operator dependent, and not widely available for clinical application.
Blood and tissue-based clinical tools
Neurofilament light chain (NfL) levels, a marker of neuronal injury, can be found increased in cerebrospinal fluid (CSF), serum, and plasma of PwP compared with controls, and correlate to motor phenotypes in PD (37–39). Specifically, plasma NfL levels are found to be higher in PIGD subtype than in TD subtype (40, 41), whilst serum NfL levels have shown to correlate with motor severity (42). CSF, serum, and plasma alpha-synuclein levels have been investigated in several studies for its mapping of motor features in PD, confirming its role as a potential clinical tool for assessing motor severity (43–46). We feel therefore these may be important markers to consider in future, once its validity is widely established, with focus on plasma and serum-based tests over CSF for clinician’s ease of application.
Other clinical tools
Wearable technology (WT) is widely used in research settings for motor monitoring, especially in the more advanced stages of PD, characterized by the presence of motor fluctuations and more severe sleep disturbances (47, 48). Given the ability of WT to provide objective and continuous monitoring, it has the potential to assist clinicians in their assessments of PwP. In fact, studies have provided supporting evidence over WTs ability to collect reliable daily measurements of motor features (49), together with information on the effectiveness of levodopa treatment (50). However, its clinical application is limited at present, given lack of standardization across the several possible WT available and in development (47). Therefore, clinicians may choose to use WT in addition to their clinical-based management strategies.
Dashboard vital: nonmotor
NMS in PD are ubiquitous, from prodromal to palliative stage (51). Based on data-driven analysis as well as observational case series-based data, a recent description of nonmotor subtypes or endophenotypes, such as Park-Cognitive and Park-Pain, has been suggested. These subtypes may be underpinned by specific dominant neurotransmitter dysfunctions, largely cholinergic, noradrenergic, and serotonergic, apart from the well-known dopaminergic one (3, 52, 53). A subtype-specific management has been proposed (53) and the nonmotor dashboard of vitals aims to reflect tests that can identify the different subtypes, to aid a dashboard-triggered personalized medicine.
Clinical tests for nonmotor
Imaging-based clinical tools
DaTScans abnormalities have been associated with multiple NMS (54), but it is unclear whether this measurement could be used to assess the overall burden of NMS in PD. This might be due to the predominantly non-dopaminergic nature of many NMS, therefore would not be captured by dopaminergic imaging alone (55).
123I-metaiodobenzylguandine (MIBG) scintigraphy can be used to evaluate the postganglionic cardiac autonomic denervation, typical of PD (56). Recent evidence has supported the use of MIBG scintigraphy for the evaluation of the burden of autonomic dysfunction, as measured by the wash-out rate which seems to correlate with severity of orthostatic hypotension (57). However, the results have not been replicated in other studies (58). MIBG use in NMS remains investigational at present and requires careful consideration.
Blood and tissue-based clinical tools
Serum uric acid (UA, urate) has been explored in several studies, given its potential neuroprotective effect in PD and its inverse association with the risk of developing PD (59–61). Lower UA levels have been correlated with experiencing higher NMS burden, whilst higher UA levels seem to be more protective (62–64). However, conflicting data exists for UA application as a measure of NMS, and as such, it’s use clinically is currently not appropriate without further large-scale studies (65–67).
Serum homocysteine (Hcy) has been posed as a gender-specific biomarker for males developing PD dementia (PDD) and for disease progression (68, 69). In a recent data-driven study, using ‘severe’ subtype (motor, non-motor and cognitive domains) had higher levels of serum Hcy and C-reactive protein (CRP) compared to other, less severe, subtypes (70). However, levodopa, the gold standard of therapy in PD, has been shown to increase Hcy levels (71–73) and so the real validity of Hcy measurement remains questionable.
Among CSF biomarkers, those associated with the overall burden of NMS include β-amyloid 1–42 (Aβ1–42) and α-synuclein (74, 75). In particular, CSF Aβ1–42 has been found to be a significant predictor of longitudinal increase in NMS severity over 2 years in a cohort of over 400 patients from the Parkinson’s Progression Marker Initiative (PPMI) (74). On the other hand, in a study involving 46 PwP, CSF α-synuclein was inversely correlated with the total score of the NMS Scale (NMSS) (75). However studies of CSF and plasma Aβ1–42 and α-synuclein remain inconsistent (76–80). Among other suggested markers, CSF and plasma NfL increase can also be associated with cognitive symptoms of PD (81, 82).
Other clinical tools
WT has been shown to be useful in the evaluation of NMS, specifically daytime somnolence, the presence of RBD (83) and nocturnal movements (84). Additionally, measures of bradykinesia and dyskinesia as collected through the Parkinson’s KinetiGraph (PKG), have showed to be associated with gastrointestinal problems (85).
Additionally, modern technologies can also help in the assessment of cognition in PD. Several computerized cognitive batteries are in fact available to screen and investigate cognitive impairment, which can be also useful for patients’ remote assessment and digital intervention such as cognitive training (65, 66).
Dashboard vital: visual, gut and oral health
Visual health
Visual abnormalities are particularly frequent in PwP, with an overall prevalence ranging from 78 to 82% (67, 86). Nevertheless, they are surprisingly often neglected in both clinical and research settings, leading to functional consequences, such as falls at night-time related to reduced night vision, road traffic accidents in PwP who drive, dry eyes causing ocular irritation and overall reduced QoL for patients (67, 87).
PwP suffer from a wide range of visual defects, from contrast insensitivity and reduced color discrimination, symptoms usually present since the early stages, to oculomotor disturbances, in particular convergence insufficiency and diplopia (88–90). The pathophysiology of visual abnormalities in PD is complex, and is in part due to the retinal dopamine depletion (91), the presence of a PD-related retinopathy (92, 93), alongside neuronal dysfunction in the visual cortex (94), and cortical or basal ganglia circuits dysfunction (95). Dry eyes can be caused by decreased blinking rate and decreased tear production due to autonomic dysfunction (96), while other symptoms can be related to the use of PD medication, such as the rare levodopa-induced ocular dyskinesia, iatrogenic hallucinations, or blurred vision induced by monoamine oxidase inhibitors and amantadine (97–99). Prolonged use of amantadine might also cause changes in corneal endothelium and oedema (100, 101).
Gut health
Gut health is strictly intertwined with the control of motor symptoms, NMS, and pathophysiology burden of the disease (102). Dysphagia, a crucial NMS in advanced PD, has significant impact on nutrition, hydration, and general health in PwP (7, 103) and is often not considered in earlier stages of PD. Constipation impacts the QoL and levodopa absorption in PwP (104). Additionally, intestinal pseudo-obstruction (paralytic ileus) can be one of the presentations of severe constipation in advanced PD, representing a medical emergency (105).
Another gut health-related factor impacting symptom control in PD is Helicobacter pylori (HP)-induced gastritis, which has an increased prevalence in PwP (104). Eradication of HP in PwP has been shown by several studies to improve levodopa response and motor performances (106), although a randomized placebo-controlled trial refutes these findings (107).
HP infection, coupled with decreased intestinal transit time, diminish the gastric acid production too, facilitating gut dysbiosis, including small intestinal bacterial overgrowth (SIBO) (108). SIBO is associated with poor motor function and could be a potential future target for disease modifying treatment (109). Other possible targets associated with gut dysbiosis, are the PD specific microbiome profiles (110, 111), whereby bacteria belonging to the Lachnospiraeceae family and faecalibactrium genus, are emerging as the most consistently altered gut microbiome in PwP (112).
Oral health
Due to the impairments caused by motor and NMS, PwP may have poor oral health and hygiene, with consequent increase of oral disease such as periodontitis and cavities (113), which, in turn, can increase the burden of some of the NMS (e.g., swallowing issues, pain, anxiety) and worsen QoL (114). Oral health may also play a role in the pathogenesis of PD, contributing via oral-dysbiosis, to induce systemic inflammation measurable, amongst others, with CRP (115–120).
Among oral bacteria, Porphyromonas gingivalis is associated with the presence of chronic periodontitis, and its toxic proteases, gingipains, and lipopolysaccharide, have been found in the blood of patients with PD (119), suggesting a possible pathological role in neurodegeneration (121, 122).
Sialorrhea, affecting up to 80% of PwP, often is the by-product of reduced swallowing, sealed lips from hypomimia, and poor awareness of drooling due to changes in sensation and posture (123, 124).
Clinical tests for visual, gut, and oral health
Visual health
Numerous studies have shown significant retinal nerve fiber layer thinning and macular volume reduction at optical coherence tomography (OCT) in PwP compared to controls (125–127), yet there remains no agreement on which retinal segments are the most affected (125). These findings explain symptoms such as decreased visual acuity and impaired contrast sensitivity, altered color perception, and seem to be associated with disease stage and severity (125–127).
An earlier biomarker, even with normal OCT findings, is the bioelectrical retinal dysfunction shown with the electroretinography (ERG) and measured via several parameters, such as alpha-wave, beta-wave, or oscillatory potentials amplitude (128–130). Similarly, visual evoked potentials (VEP), can be used to discriminate PD patients from controls in early disease stages, being a higher P100 latency the most consistent biomarker (131).
Corneal confocal microscopy (CCM), measuring parameters like corneal nerve branch density, corneal nerve fiber length, corneal total branch density, and corneal nerve fiber area, is a non-invasive tool to evaluate small nerve and autonomic fibers damage (132). Several studies have demonstrated the presence of corneal nerve fiber pathology in PwP than controls (133–135), whose extension have been shown to be associated with disease severity, and specifically motor, autonomic and cognitive dysfunction (135–137).
Tear film tests, such as Schirmer’s test or tear film breakup time associated with dry eyes, together with blink rate evaluated with specific cameras, have been demonstrated to be abnormal in PwP and to correlate with disease severity (96, 138, 139). Furthermore, initial findings on tears protein composition, have shown a significant increase of oligomeric alpha synuclein in both basal and, more largely, reflex tears in PwP compared to healthy controls, paving the way for a new potential approach to alpha synuclein-based biomarkers (140), which need further exploration for clinical application.
Since nyctalopia (night-blindness) is the earliest and most common symptom of hypovitaminosis A (141), and PD might be associated to low levels of specific vitamins such as vitamin D and B12 (142, 143), the possibility of reduced blood and/or CSF concentrations of vitamin A (retinol) should be investigated. This deficit could contribute to worsen PD-related visual symptoms at the most challenging time of the day for a PwP, and therefore we believe it should be taken into consideration, as potentially reversible. However, studies have failed to show a systemic reduction of vitamin A levels in PwP, being its concentration controlled by a rigorous homeostatic process, or of retinoic acid, retinol’s active metabolite, because of its high biochemical instability (144).
Gut health
Upper gastrointestinal symptoms screening has been proposed for evaluating swallowing and oropharyngeal dysfunction (145). Diagnostic tools for swallowing abnormalities can be extensive, and include videofluoroscopic swallow study (VFSS) for the oropharyngeal phase of swallowing, or the fiberoptic endoscopic evaluation of swallowing (FEES) for the pharyngeal phase of swallowing, with both VFSS and FEES having a role in the identification of aspiration, particularly significant in the advance stages (146). From a practical and pragmatic point of view a VFSS seems to be the most relevant.
Constipation is a clinical diagnosis although imaging such as abdominal XR and CT scans can be used to demonstrate its severity. Radiological findings of constipation can include evidence of fecal loading throughout the colon, luminal faecalomas, with or without reduction of luminal gas (147). Furthermore, abdominal XR or CT helps in the diagnosis of pseudo-obstruction showing dilation of the colon, with or without other associated radiological features such as a volvulus-sign and is strongly recommended in advanced PD cases, particularly when screening for intrajejunal levodopa infusion therapies (105).
HP diagnostic testing are numerous, including the urea breath test, the stool antigen test, serology, and polymerase chain reaction, and should also be used to confirm the absence of HP post-eradication therapy (148). Gut dysbiosis and local proinflammatory status can be measured through sampling using stool microbiota (112), while the hydrogen breath test specifically applies to the identification of SIBO (149).
Oral health
For the effective evaluation of oral health in PwP, current recommendations advise for regular review by a dentist, with particular attention to the presence of local inflammatory conditions such as periodontitis (150).
Systemic inflammatory markers, such as CRP, might have a role in early detection of inflammatory oral conditions in PD, together with other systemic inflammatory markers, e.g., WBC, IL-1alpha, IL-1beta, IL-17A and TNF-alpha (118–120).
To identify Porphyromonas gingivalis, the possibility of detecting gingipains in the bloodstream, particularly gingipain R1, and the analysis of the oral microbiota through a saliva sample, should be explored further (116, 117, 119).
A simple salivatory test consisting in an Enzyme-linked immunosorbent assay (ELISA)-based multi-marker test of proteins including matrix metalloproteinases (MMP8, MMP9, MMP2, MMP3), has shown promising results in early diagnosis of gingivitis and periodontitis (125), but this remains under investigation.
Objective measurement of sialorrhea is often difficult, however methodologies exist, including salivation collection (151), suctioning (152), swallowing counts (153), Lashley cup over the parotid duct (153), and dental cotton buds in the mouth (154). However, these objective tests are often time consuming, costly, and poorly practicable in clinical practice.
Dashboard vitals: bone health and falls
PwP are at increasing risk of developing osteoporosis, with women having a significantly higher risk than men with PD (155). In fact, the prevalence of osteoporosis has been observed in 91% of women and 61% of men with PD (155).
Osteoporosis is a reduction of bone mineral density (BMD) subsequently increasing the risk of fragility fractures (156). Several pathophysiological mechanisms seem to be implicated in the association between PD and osteoporosis and reduced BMD, and these include: female gender, vitamin D deficiency, reduced exposure to sunlight, low body weight, nutritional status, vitamin B12, folate deficiency and hyperhomocysteinemia, PD duration and severity, immobility, decreased muscle strength and other neuroendocrinal status (e.g., increased serum concentration of under-carboxylated osteocalcin) (155, 157).
Recent evidence has suggested that osteoporosis associated with PD might be caused by the dopaminergic degeneration itself, as well as by the treatment with levodopa, which could promote osteoclastogenesis and suppress bone formation, in association with elevated prolactin and following both gonadal steroid hormone-dependent and-independent metabolic pathways (158).
Considering the higher risk of falls due to postural and gait impairment in PwP (159–161), the presence of osteoporosis further increases the risk of fractures and subsequent hospitalization compared to age-matched individuals (8, 162).
Clinical tests for bone health and falls
Imaging-based clinical tools
To confirm the diagnosis of osteoporosis, a dual-energy X-ray absorptiometry (DEXA) at the hip is required: according to the World Health Organization classification, a BMD of 2.5 standard deviations (SD) below that of a young adult defines osteoporosis, while value on DEXA between 1 and 2.5 SD is considered as osteopenia (157). In our opinion, DEXA should be obligatory in PD patients over the age of 50.
Recently, other imaging techniques have been investigated in PD, such as distal radius DEXA, which could potentially optimize the detection of osteoporosis, especially in female PD patients (163), and the less invasive calcaneal quantitative ultrasound to assess bone quality, which has demonstrated good correlation with DEXA measurements and has been recently evaluated in a cohort of PwP (164).
Blood-based clinical tools
To promptly address and, when possible, prevent osteoporosis in PwP, several simple blood tests can be undertaken to get an overview of the calcium metabolism and other potential risk factors for osteoporosis. First and foremost, serum levels of 25-OH-vitamin D have been shown to directly correlate with BMD of the hip and lumbar spine in PD (155, 165, 166). Additionally, serum ionized calcium has also been found to correlate with BMD and to be significantly different between PwP and healthy controls (166).
Other clinical tools
The Fracture Risk Assessment Tool (FRAX) (167) helps predict the 10-year probability of hip fractures and major osteoporosis-related fractures, and has been used in several large studies exploring osteoporosis and fracture risks in the population (156). FRAX tool may require adjustment to have PD as an independent risk factor for fractures and as such, requires a modern adaptation to PwP (168, 169).
Wearable sensors have been shown to be reliable in PwP for detecting balance issues and predict risk of falls. WT can analyze gait and balance in real-time whilst providing feedback (e.g., visual, and auditory cues) for features of postural instability, such as freezing of gait. This feedback provided by WT can then reduce the risk of fall events from occurring, as such, WT can be a promising tool for rehabilitation and falls prevention (170, 171).
Dashboard vital: co-morbidities, co-medications, and dopamine agonists side effects
Several global epidemiological studies confirmed the present of several comorbidities in PwP, which can be grouped as cardiovascular (e.g., hypertension, heart failure), neuropsychiatric (e.g., depression, dementia), and others including diabetes mellitus, arthritis, and urinary symptoms (172–177). Advanced stages of PD are associated with balance issues and postural instability (178). Despite no evidence of direct involvement of the vestibular system in PD, a possible vestibular hypofunction could be considered in patients with dizziness but not orthostatic hypotension (179). The importance of appreciating PwP co-morbidities in their management has been highlighted by Schrag and colleagues (2022) in a case–control study, observing how certain co-morbidities and risk factors may reflect potential early extra-striatal and extracerebral pathophysiology in PwP (180).
Given the progressive nature of PD, co-medications, particularly in the advanced stages, represent a clinical challenge and expose patients to increased risk for drug interaction and hospitalization (181, 182). Additionally, the required increased dopaminergic stimulation in PD, in particularly when using dopamine agonists alone or in combination, might give emergence to significant side effects, such as severe daytime sleepiness (183) or impulse control disorders (ICDs) (184). ICDs and impulse control behaviors encompass a range of presentations including hypersexuality, gambling, binge eating, and dopamine dysregulation syndrome (185).
Clinical tests for co-morbidities, co-medications, and dopamine agonists side effects
Co-morbidities
Studies report that there is an association between diabetes and PD progression, with a greater motor burden and motor decline been demonstrated in PwP who have diabetes (186, 187). Non-diabetic hyperglycaemia, with recorded glycosylated hemoglobin (HBA1c) levels above 45 mmol/mol, is an independent predictor of quicker motor progression (188–190).
The MARK-PD Study evaluated the relationship between diabetes, HBA1c and serum NfL levels as markers of neuroaxonal damage in PwP, concluding that those with diabetes and PD had higher serum NfL and more cognitive impairment, with diabetes also associated with having a higher H&Y score (191, 192).
Weight variability is a common clinical finding in PwP and yet remains poorly understood (193). Unexplained weight loss has been reported across all stages of PD (193) and it has also been proposed as a prodromal feature (194). Monitoring the patient’s body mass index (BMI) can be a simple and effective way to prevent pathological weight loss leading to poorer prognosis and higher mortality (195, 196). Weight loss in PD has also been associated with faster cognitive decline, while presynaptic dopaminergic depletion in the right striatum may serve as a predictor of future weight changes (197).
Blood pressure fluctuations have been recognized in PD, due to the concomitant involvement of the autonomic system (198), which can present as postprandial hypotensive episodes during the day, as well as nocturnal hypotension, causing great limitation of QoL. These fluctuations can be measured using a 24-h ambulatory blood pressure monitoring device (199). Further assessment through the head-up tilt-test gives clinicians insight into the activation of the autonomic systemic compensatory mechanism in PwP (200).
Dizziness, in the absence of orthostatic hypotension, can be examined using the Vestibular Evoked Myogenic Potentials, to consider vestibular hypofunction as a cause (179). However, the clinical application of this remains under investigation.
Co-medications
Co-morbidities Polypharmacy Score (CPS) is a simple clinical tool providing a surrogate measure of co-morbidities burden, correlating with poor outcomes and mortality rates (201, 202). When assessing co-medications, particular attention should be given to the anticholinergic burden, especially in the older adults, considering the numerous medications that have anticholinergic properties, such as analgesics, antispasmodics, antiarrhythmics and anti-Parkinson’s (203). Anticholinergic burden has been linked to functional and cognitive decline in PwP, with possible contribution to overt dementia, and potential side effects including constipation, agitation, urinary retention, and delirium (203, 204). The use of anticholinergic indexes, such as Anticholinergic Drug Scale (ADS) and the Drug Burden Index - Anticholinergic Component (DBI-ACh), are useful clinical tool for such assessments, with ADS being shown to correlate with serum anticholinergic activity (203, 205, 206).
Dopamine agonist side effects
For ICDs, the use of electroencephalography to measure feedback-related negativity has been suggested to reflect the reward-processing of individuals, thus potentially representing a predictive clinical tool (207, 208).
Excessive daytime sleepiness (EDS) is a known side effect of dopamine agonists, with higher D2/D3 receptor agonism, such as Ropinirole and Pramipexole (183, 209, 210), and can be assessed with Multiple Sleep Latency Tests (MSLT) (211, 212). If EDS presents in the absence or withdrawal of dopamine agonists intake, then obstructive sleep apnoea should be suspected and polysomnography should be considered as further investigation (213, 214).
Conclusion
We suggest the use of ‘The Chaudhuri’s Dashboard of Vitals for Parkinson’s’ for the care of PwP. This tool can incorporate several clinical tests that might help clinicians with the screening, diagnosis, treatment, and monitoring of key and potentially health threatening factors. Until now, only two of the five vitals have been widely investigated in clinical practice, namely motor and nonmotor assessments. In this paper, we have worked to highlight the importance of three other vitals (Vital 3–5, Figure 1), which have an impact on not only the QoL for PwP, but also the disease progression and prognosis. Some clinical tests discussed in this paper are already available in either general or PD-related clinical practice, whilst others have the potential of future implementation, providing they are validated and are cost-effective for healthcare systems across the globe.
Author contributions
MQ, SR, LB, IS, CF-P, NT, and KR contributed toward the design and methodology. MQ, SR, LB, and KR contributed toward the formal analysis and first draft of the manuscript. All authors contributed toward the conceptualization, review, and editing of the manuscript for submission.
Conflict of interest
The authors declare that the research was conducted in the absence of any commercial or financial relationships that could be construed as a potential conflict of interest.
Publisher’s note
All claims expressed in this article are solely those of the authors and do not necessarily represent those of their affiliated organizations, or those of the publisher, the editors and the reviewers. Any product that may be evaluated in this article, or claim that may be made by its manufacturer, is not guaranteed or endorsed by the publisher.
References
1. Parkinson, J. An essay on the shaking palsy. 1817. J Neuropsychiatry Clin Neurosci. (2002) 14:223–36. doi: 10.1176/jnp.14.2.223
2. Dorsey, ER, Sherer, T, Okun, MS, and Bloem, BR. The emerging evidence of the Parkinson pandemic. J Parkinsons Dis. (2018) 8:S3–8. doi: 10.3233/JPD-181474
3. Titova, N, Padmakumar, C, Lewis, SJG, and Chaudhuri, KR. Parkinson’s: a syndrome rather than a disease? J Neural Transm. (2017) 124:907–14. doi: 10.1007/s00702-016-1667-6
4. Chaudhuri, KR, Healy, DG, and Schapira, AH. Non-motor symptoms of Parkinson’s disease: diagnosis and management. Lancet Neurol. (2006) 5:235–45. doi: 10.1016/S1474-4422(06)70373-8
5. Subramanian, I, Brindle, S, Perepezko, K, and Chaudhuri, KR. Wellness, sexual health, and nonmotor Parkinson’s. Int Rev Neurobiol, (2022) 162, 171–184. doi: 10.1016/bs.irn.2021
6. Chaudhuri, KR, Titova, N, Qamar, MA, Murășan, I, and Falup-Pecurariu, C. The dashboard vitals of Parkinson’s: not to be missed yet an unmet need. J Pers Med. (2022) 12:1994. doi: 10.3390/jpm12121994
7. Leta, V, Klingelhoefer, L, Longardner, K, Campagnolo, M, Levent, HÇ, Aureli, F, et al. Gastrointestinal barriers to levodopa transport and absorption in Parkinson’s disease. Eur J Neurol. (2023) 30:1465–80. doi: 10.1111/ene.15734
8. Raglione, LM, Sorbi, S, and Nacmias, B. Osteoporosis and Parkinson's disease. Clin Cases Miner Bone Metab. (2011) 8:16–8.
9. Yeung, EYH, and Cavanna, AE. Sleep attacks in patients with Parkinson's disease on dopaminergic medications: a systematic review. Mov Disord Clin Pract. (2014) 1:307–16. doi: 10.1002/mdc3.12063
10. Titova, N, and Chaudhuri, KR. Personalized medicine in Parkinson's disease: time to be precise. Mov Disord. (2017) 32:1147–54. doi: 10.1002/mds.27027
11. Palmer, JL, Coats, MA, Roe, CM, Hanko, SM, Xiong, C, and Morris, JC. Unified Parkinson's disease rating scale-motor exam: inter-rater reliability of advanced practice nurse and neurologist assessments. J Adv Nurs. (2010) 66:1382–7. doi: 10.1111/j.1365-2648.2010.05313.x
12. Goetz, CG, Tilley, BC, Shaftman, SR, Stebbins, GT, Fahn, S, Martinez-Martin, P, et al. Movement Disorder Society-sponsored revision of the unified Parkinson’s disease rating scale (MDS-UPDRS): scale presentation and clinimetric testing results. Mov Disord. (2008) 23:2129–70. doi: 10.1002/mds.22340
13. Kishore, A, Espay, AJ, Marras, C, al-Khairalla, T, Arenovich, T, Asante, A, et al. Unilateral versus bilateral tasks in early asymmetric Parkinson’s disease: differential effects on bradykinesia. Mov Disord. (2007) 22:328–33. doi: 10.1002/mds.21238
14. Prime, M, McKay, JL, Bay, AA, Hart, AR, Kim, C, Abraham, A, et al. Differentiating Parkinson disease subtypes using clinical balance measures. J Neurol Phys Ther. (2020) 44:34–41. doi: 10.1097/NPT.0000000000000297
15. Ren, J, Hua, P, Li, Y, Pan, C, Yan, L, Yu, C, et al. Comparison of three motor subtype classifications in de novo Parkinson’s disease patients. Front Neurol. (2020) 11:601225. doi: 10.3389/fneur.2020.601225
16. Campbell, MC, Myers, PS, Weigand, AJ, Foster, ER, Cairns, NJ, Jackson, JJ, et al. Parkinson disease clinical subtypes: key features & clinical milestones. Ann Clin Transl Neurol. (2020) 7:1272–83. doi: 10.1002/acn3.51102
17. Ren, J, Pan, C, Li, Y, Li, L, Hua, P, Xu, L, et al. Consistency and stability of motor subtype classifications in patients with de novo Parkinson’s disease. Front Neurosci. (2021) 15:637896. doi: 10.3389/fnins.2021.637896
18. Spiegel, J, Hellwig, D, Samnick, S, Jost, W, Möllers, MO, Fassbender, K, et al. Striatal FP-CIT uptake differs in the subtypes of early Parkinson’s disease. J Neural Transm. (2007) 114:331–5. doi: 10.1007/s00702-006-0518-2
19. Zaidel, A, Arkadir, D, Israel, Z, and Bergman, H. Akineto-rigid vs. tremor syndromes in parkinsonism. Curr Opin Neurol. (2009) 22:387–93. doi: 10.1097/WCO.0b013e32832d9d67
20. Brücke, T, and Brücke, C. Dopamine transporter (DAT) imaging in Parkinson’s disease and related disorders. J Neural Transm. (2022) 129:581–94. doi: 10.1007/s00702-021-02452-7
21. Calle, S, Dawood, L, Tripathee, NR, Cai, C, Kaur, H, Wan, DQ, et al. Identification of patterns of abnormalities seen on DaTscan™ SPECT imaging in patients with non-Parkinson’s movement disorders. RMI. (2019) 12:9–15. doi: 10.2147/RMI.S201890
22. Gayed, I, Joseph, U, Fanous, M, Wan, D, Schiess, M, Ondo, W, et al. The impact of DaTscan in the diagnosis of Parkinson disease. Clin Nucl Med. (2015) 40:390–3. doi: 10.1097/RLU.0000000000000766
23. Nakajima, A, Shimo, Y, Sekimoto, S, Kamagata, K, Jo, T, Oyama, G, et al. Dopamine transporter imaging predicts motor responsiveness to levodopa challenge in patients with Parkinson’s disease: a pilot study of DATSCAN for subthalamic deep brain stimulation. J Neurol Sci. (2018) 385:134–9. doi: 10.1016/j.jns.2017.12.030
24. Zhou, Y, Tinaz, S, and Tagare, HD. Robust bayesian analysis of early-stage Parkinson’s disease progression using DaTscan images. IEEE Trans Med Imaging. (2021) 40:549–61. doi: 10.1109/TMI.2020.3031478
25. Augimeri, A, Cherubini, A, Cascini, GL, Galea, D, Caligiuri, ME, Barbagallo, G, et al. CADA-computer-aided DaTSCAN analysis. EJNMMI Phys. (2016) 3:4. doi: 10.1186/s40658-016-0140-9
26. Lee, JW, Song, YS, Kim, H, Ku, BD, and Lee, WW. Alteration of tremor dominant and postural instability gait difficulty subtypes during the progression of Parkinson's disease: analysis of the PPMI cohort. Front Neurol. (2019) 10:471. doi: 10.3389/fneur.2019.00471
27. Mohl, B, Berman, BD, Shelton, E, and Tanabe, J. Levodopa response differs in Parkinson's motor subtypes: a task-based effective connectivity study. J Comp Neurol. (2017) 525:2192–201. doi: 10.1002/cne.24197
28. Bhattacharjee, S, Paramanandam, V, and Bhattacharya, A. Analysis of the effect of dopamine transporter scan on the diagnosis and Management in a Tertiary Neurology Center. Neurohospitalist. (2019) 9:144–50. doi: 10.1177/1941874419829293
29. Simuni, T, Siderowf, A, Lasch, S, Coffey, CS, Caspell-Garcia, C, Jennings, D, et al. Longitudinal change of clinical and biological measures in early Parkinson's disease: Parkinson's progression markers initiative cohort. Mov Disord. (2018) 33:771–82. doi: 10.1002/mds.27361
30. Adams, MP, Rahmim, A, and Tang, J. Improved motor outcome prediction in Parkinson's disease applying deep learning to DaTscan SPECT images. Comput Biol Med. (2021) 132:104312. doi: 10.1016/j.compbiomed.2021.104312
31. Gaurav, R, Yahia-Cherif, L, Pyatigorskaya, N, Mangone, G, Biondetti, E, Valabrègue, R, et al. Longitudinal changes in neuromelanin MRI signal in Parkinson's disease: a progression marker. Mov Disord. (2021) 36:1592–602. doi: 10.1002/mds.28531
32. Prasad, S, Stezin, A, Lenka, A, George, L, Saini, J, Yadav, R, et al. Three-dimensional neuromelanin-sensitive magnetic resonance imaging of the substantia nigra in Parkinson’s disease. Eur J Neurol. (2018) 25:680–6. doi: 10.1111/ene.13573
33. Cheng, Z, Zhang, J, He, N, Li, Y, Wen, Y, Xu, H, et al. Radiomic features of the nigrosome-1 region of the substantia nigra: using quantitative susceptibility mapping to assist the diagnosis of idiopathic Parkinson’s disease. Front Aging Neurosci. (2019) 11:167. doi: 10.3389/fnagi.2019.00167
34. Langkammer, C, Pirpamer, L, Seiler, S, Deistung, A, Schweser, F, Franthal, S, et al. Quantitative susceptibility mapping in Parkinson's disease. PLoS One. (2016) 11:e0162460. doi: 10.1371/journal.pone.0162460
35. Ofori, E, Pasternak, O, Planetta, PJ, Burciu, R, Snyder, A, Febo, M, et al. Increased free water in the substantia nigra of Parkinson's disease: a single-site and multi-site study. Neurobiol Aging. (2015) 36:1097–104. doi: 10.1016/j.neurobiolaging.2014.10.029
36. Zhu, S, Wang, Y, Jiang, Y, Gu, R, Zhong, M, Jiang, X, et al. Clinical features in Parkinson's disease patients with hyperechogenicity in substantia nigra: a cross-sectional study. Neuropsychiatr Dis Treat. (2022) 18:1593–601. doi: 10.2147/NDT.S374370
37. Hansson, O, Janelidze, S, Hall, S, Magdalinou, N, Lees, AJ, Andreasson, U, et al. Blood-based NfL: a biomarker for differential diagnosis of parkinsonian disorder. Neurology. (2017) 88:930–7. doi: 10.1212/WNL.0000000000003680
38. Marques, TM, van Rumund, A, Oeckl, P, Kuiperij, HB, Esselink, RAJ, Bloem, BR, et al. Serum NFL discriminates Parkinson disease from atypical parkinsonisms. Neurology. (2019) 92:e1479–86. doi: 10.1212/WNL.0000000000007179
39. Lin, CH, Li, CH, Yang, KC, Lin, FJ, Wu, CC, Chieh, JJ, et al. Blood NfL: a biomarker for disease severity and progression in Parkinson disease. Neurology. (2019) 93:e1104–11. doi: 10.1212/WNL.0000000000008088
40. Ng, ASL, Tan, YJ, Yong, ACW, Saffari, SE, Lu, Z, Ng, EY, et al. Utility of plasma neurofilament light as a diagnostic and prognostic biomarker of the postural instability gait disorder motor subtype in early Parkinson’s disease. Mol Neurodegener. (2020) 15:33. doi: 10.1186/s13024-020-00385-5
41. Bäckström, D, Linder, J, Jakobson Mo, S, Riklund, K, Zetterberg, H, Blennow, K, et al. NfL as a biomarker for neurodegeneration and survival in Parkinson disease. Neurology. (2020) 95:e827–38. doi: 10.1212/WNL.0000000000010084
42. Huang, Y, Huang, C, Zhang, Q, Shen, T, and Sun, J. Serum NFL discriminates Parkinson disease from essential tremor and reflect motor and cognition severity. BMC Neurol. (2022) 22:39. doi: 10.1186/s12883-022-02558-9
43. Vavougios, GD, Doskas, T, Kormas, C, Krogfelt, KA, Zarogiannis, SG, and Stefanis, L. Identification of a prospective early motor progression cluster of Parkinson's disease: data from the PPMI study. J Neurol Sci. (2018) 387:103–8. doi: 10.1016/j.jns.2018.01.025
44. Mollenhauer, B, Caspell-Garcia, CJ, Coffey, CS, Taylor, P, Singleton, A, Shaw, LM, et al. Longitudinal analyses of cerebrospinal fluid α-Synuclein in prodromal and early Parkinson's disease. Mov Disord. (2019) 34:1354–64. doi: 10.1002/mds.27806
45. Chang, C-W, Yang, SY, Yang, CC, Chang, CW, and Wu, YR. Plasma and serum alpha-synuclein as a biomarker of diagnosis in patients with Parkinson's disease. Front Neurol. (2020) 10:1388. doi: 10.3389/fneur.2019.01388
46. Foulds, PG, Diggle, P, Mitchell, JD, Parker, A, Hasegawa, M, Masuda-Suzukake, M, et al. A longitudinal study on α-synuclein in blood plasma as a biomarker for Parkinson's disease. Sci Rep. (2013) 3:2540. doi: 10.1038/srep02540
47. Barrachina-Fernández, M, Maitín, AM, Sánchez-Ávila, C, and Romero, JP. Wearable technology to detect motor fluctuations in Parkinson’s disease patients: current state and challenges. Sensors. (2021) 21:4188. doi: 10.3390/s21124188
48. Ossig, C, Antonini, A, Buhmann, C, Classen, J, Csoti, I, Falkenburger, B, et al. Wearable sensor-based objective assessment of motor symptoms in Parkinson’s disease. J Neural Transm. (2016) 123:57–64. doi: 10.1007/s00702-015-1439-8
49. Oyama, G, Burq, M, Hatano, T, Marks, WJ Jr, Kapur, R, Fernandez, J, et al. Analytical and clinical validity of wearable, multi-sensor technology for assessment of motor function in patients with Parkinson’s disease in Japan. Sci Rep. (2023) 13:3600. doi: 10.1038/s41598-023-29382-6
50. Ricci, M, Lazzaro, GD, Errico, V, Pisani, A, Giannini, F, and Saggio, G. The impact of wearable electronics in assessing the effectiveness of levodopa treatment in Parkinson’s disease. IEEE J Biomed Health Inform. (2022) 26:2920–8. doi: 10.1109/JBHI.2022.3160103
51. Schapira, AHV, Chaudhuri, KR, and Jenner, P. Non-motor features of Parkinson disease. Nat Rev Neurosci. (2017) 18:435–50. doi: 10.1038/nrn.2017.62
52. Sauerbier, A, Jenner, P, Todorova, A, and Chaudhuri, KR. Non motor subtypes and Parkinson's disease. Parkinsonism Relat Disord. (2016) 22:S41–6. doi: 10.1016/j.parkreldis.2015.09.027
53. Marras, C, Chaudhuri, KR, Titova, N, and Mestre, TA. Therapy of Parkinson’s disease subtypes. Neurotherapeutics. (2020) 17:1366–77. doi: 10.1007/s13311-020-00894-7
54. Liu, R, Umbach, DM, Tröster, AI, Huang, X, and Chen, H. Non-motor symptoms and striatal dopamine transporter binding in early Parkinson’s disease. Parkinsonism Relat Disord. (2020) 72:23–30. doi: 10.1016/j.parkreldis.2020.02.001
55. Chaudhuri, KR, and Schapira, AH. Non-motor symptoms of Parkinson’s disease: dopaminergic pathophysiology and treatment. Lancet Neurol. (2009) 8:464–74. doi: 10.1016/S1474-4422(09)70068-7
56. Skowronek, C, Zange, L, and Lipp, A. Cardiac 123I-MIBG scintigraphy in neurodegenerative Parkinson syndromes: performance and pitfalls in clinical practice. Front Neurol. (2019) 10:152. doi: 10.3389/fneur.2019.00152
57. Jeong, YJ, Jeong, JE, Cheon, SM, Yoon, BA, Kim, JW, and Kang, DY. Relationship between the washout rate of I-123 MIBG scans and autonomic function in Parkinson's disease. PLoS One. (2020) 15:e0229860. doi: 10.1371/journal.pone.0229860
58. Rocchi, C, Pierantozzi, M, Galati, S, Chiaravalloti, A, Pisani, V, Prosperetti, C, et al. Autonomic function tests and MIBG in Parkinson's disease: correlation to disease duration and motor symptoms. CNS Neurosci Ther. (2015) 21:727–32. doi: 10.1111/cns.12437
59. Schlesinger, I, and Schlesinger, N. Uric acid in Parkinson’s disease. Mov Disord. (2008) 23:1653–7. doi: 10.1002/mds.22139
60. Winquist, A, Steenland, K, and Shankar, A. Higher serum uric acid associated with decreased Parkinson's disease prevalence in a large community-based survey. Mov Disord. (2010) 25:932–6. doi: 10.1002/mds.23070
61. Jain, S, Ton, TG, Boudreau, RM, Yang, M, Thacker, EL, Studenski, S, et al. The risk of Parkinson disease associated with urate in a community-based cohort of older adults. Neuroepidemiology. (2011) 36:223–9. doi: 10.1159/000327748
62. Moccia, M, Picillo, M, Erro, R, Vitale, C, Longo, K, Amboni, M, et al. Presence and progression of non-motor symptoms in relation to uric acid in de novo Parkinson's disease. Eur J Neurol. (2015) 22:93–8. doi: 10.1111/ene.12533
63. van Wamelen, DJ, Taddei, RN, Calvano, A, Titova, N, Leta, V, Shtuchniy, I, et al. Serum uric acid levels and non-motor symptoms in Parkinson’s disease. J Parkinsons Dis. (2020) 10:1003–10. doi: 10.3233/JPD-201988
64. Shi, X, Zheng, J, Ma, J, Wang, Z, Sun, W, Li, M, et al. Low serum uric acid levels are associated with the nonmotor symptoms and brain gray matter volume in Parkinson’s disease. Neurol Sci. (2022) 43:1747–54. doi: 10.1007/s10072-021-05558-8
65. Aarsland, D, Batzu, L, Halliday, GM, Geurtsen, GJ, Ballard, C, Ray Chaudhuri, K, et al. Parkinson disease-associated cognitive impairment. Nat Rev Dis Primers. (2021) 7:47. doi: 10.1038/s41572-021-00280-3
66. Hanna-Pladdy, B, Enslein, A, Fray, M, Gajewski, BJ, Pahwa, R, and Lyons, KE. Utility of the NeuroTrax computerized battery for cognitive screening in Parkinson’s disease: comparison with the MMSE and the MoCA. Int J Neurosci. (2010) 120:538–43. doi: 10.3109/00207454.2010.496539
67. Sauerbier, A, and Ray Chaudhuri, K. Parkinson’s disease and vision. Basal Ganglia. (2013) 3:159–63. doi: 10.1016/j.baga.2013.05.002
68. Bakeberg, MC, Jefferson, A, Riley, M, Byrnes, M, Ghosh, S, Mastaglia, FL, et al. Elevated serum homocysteine levels have differential gender-specific associations with motor and cognitive states in Parkinson’s disease. Parkinson’s Dis. (2019) 2019:3124295. doi: 10.1155/2019/3124295
69. Sleeman, I, Lawson, RA, Yarnall, AJ, Duncan, GW, Johnston, F, Khoo, TK, et al. Urate and homocysteine: predicting motor and cognitive changes in newly diagnosed Parkinson's disease. J Parkinsons Dis. (2019) 9:351–9. doi: 10.3233/JPD-181535
70. Deng, X, Saffari, SE, Liu, N, Xiao, B, Allen, JC, Ng, SYE, et al. Biomarker characterization of clinical subtypes of Parkinson disease. NPJ Parkinsons Dis. (2022) 8:109. doi: 10.1038/s41531-022-00375-y
71. Müller, T, Jugel, C, Ehret, R, Ebersbach, G, Bengel, G, Muhlack, S, et al. Elevation of total homocysteine levels in patients with Parkinson’s disease treated with duodenal levodopa/carbidopa gel. J Neural Transm. (2011) 118:1329–33. doi: 10.1007/s00702-011-0614-9
72. Toth, C, Breithaupt, K, Ge, S, Duan, Y, Terris, JM, Thiessen, A, et al. Levodopa, methylmalonic acid, and neuropathy in idiopathic Parkinson disease. Ann Neurol. (2010) 68:28–36. doi: 10.1002/ana.22021
73. Ojo, OO, Oladipo, OO, Ojini, FI, Sanya, EO, Danesi, MA, and Okubadejo, NU. Plasma homocysteine level and its relationship to clinical profile in Parkinson's disease patients at the Lagos university teaching hospital. West Afr J Med. (2011) 30:319–24.
74. Simuni, T, Caspell-Garcia, C, Coffey, CS, Weintraub, D, Mollenhauer, B, Lasch, S, et al. Baseline prevalence and longitudinal evolution of non-motor symptoms in early Parkinson’s disease: the PPMI cohort. J Neurol Neurosurg Psychiatry. (2018) 89:78–88. doi: 10.1136/jnnp-2017-316213
75. Schirinzi, T, Sancesario, GM, di Lazzaro, G, Biticchi, B, Colona, VL, Mercuri, NB, et al. CSF α-synuclein inversely correlates with non-motor symptoms in a cohort of PD patients. Parkinsonism Relat Disord. (2019) 61:203–6. doi: 10.1016/j.parkreldis.2018.10.018
76. Lin, CH, Yang, SY, Horng, HE, Yang, CC, Chieh, JJ, Chen, HH, et al. Plasma biomarkers differentiate Parkinson’s disease from atypical parkinsonism syndromes. Front Aging Neurosci. (2018) 10:123. doi: 10.3389/fnagi.2018.00123
77. Chojdak-Łukasiewicz, J, Małodobra-Mazur, M, Zimny, A, Noga, L, and Paradowski, B. Plasma tau protein and Aβ42 level as markers of cognitive impairment in patients with Parkinson’s disease. Adv Clin Exp Med. (2020) 29:115–21. doi: 10.17219/acem/112058
78. Chen, Z, Li, G, and Liu, J. Autonomic dysfunction in Parkinson’s disease: implications for pathophysiology, diagnosis, and treatment. Neurobiol Dis. (2020) 134:104700. doi: 10.1016/j.nbd.2019.104700
79. Wang, YX, Zhao, J, Li, DK, Peng, F, Wang, Y, Yang, K, et al. Associations between cognitive impairment and motor dysfunction in Parkinson's disease. Brain Behav. (2017) 7:e00719. doi: 10.1002/brb3.719
80. Wang, L, Zhang, W, Liu, F, Mao, C, Liu, CF, Cheng, W, et al. Association of cerebrospinal fluid neurofilament heavy protein levels with clinical progression in patients with Parkinson disease. JAMA Netw Open. (2022) 5:–e2223821. doi: 10.1001/jamanetworkopen.2022.23821
81. Batzu, L, Rota, S, Hye, A, Heslegrave, A, Trivedi, D, Gibson, LL, et al. Plasma p-tau181, neurofilament light chain and association with cognition in Parkinson’s disease. NPJ Parkinsons Dis. (2022) 8:154. doi: 10.1038/s41531-022-00384-x
82. Sheng, ZH, Ma, LZ, Liu, JY, Ou, YN, Zhao, B, Ma, YH, et al. Cerebrospinal fluid neurofilament dynamic profiles predict cognitive progression in individuals with de novo Parkinson’s disease. Front Aging Neurosci. (2022) 14:1061096. doi: 10.3389/fnagi.2022.1061096
83. Ko, YF, Kuo, PH, Wang, CF, Chen, YJ, Chuang, PC, Li, SZ, et al. Quantification analysis of sleep based on smartwatch sensors for Parkinson’s disease. Biosensors. (2022) 12:74. doi: 10.3390/bios12020074
84. Zampogna, A, Manoni, A, Asci, F, Liguori, C, Irrera, F, and Suppa, A. Shedding light on nocturnal movements in Parkinson's disease: evidence from wearable technologies. Sensors. (2020) 20:5171. doi: 10.3390/s20185171
85. van Wamelen, DJ, Hota, S, Podlewska, A, Leta, V, Trivedi, D, Rizos, A, et al. Non-motor correlates of wrist-worn wearable sensor use in Parkinson’s disease: an exploratory analysis. NPJ Parkinson's Dis. (2019) 5:22. doi: 10.1038/s41531-019-0094-4
86. Borm, C, Visser, F, Werkmann, M, de Graaf, D, Putz, D, Seppi, K, et al. Seeing ophthalmologic problems in Parkinson disease: results of a visual impairment questionnaire. Neurology. (2020) 94:e1539–47. doi: 10.1212/WNL.0000000000009214
87. Ekker, MS, Janssen, S, Seppi, K, Poewe, W, de Vries, NM, Theelen, T, et al. Ocular and visual disorders in Parkinson's disease: common but frequently overlooked. Parkinsonism Relat Disord. (2017) 40:1–10. doi: 10.1016/j.parkreldis.2017.02.014
88. Diederich, NJ, Pieri, V, Hipp, G, Rufra, O, Blyth, S, and Vaillant, M. Discriminative power of different nonmotor signs in early Parkinson's disease. Mov Disord Clin Pract. (2010) 25:882–7. doi: 10.1002/mds.22963
89. Law, C, Chriqui, E, Kergoat, MJ, Leclerc, BS, Panisset, M, Irving, EL, et al. Prevalence of convergence insufficiency-type symptomatology in Parkinson’s disease. Can J Neurol Sci. (2017) 44:562–6. doi: 10.1017/cjn.2017.39
90. Bye, L, and Chaudhuri, K. Diplopia in Parkinson's disease: a clinical and optometric study In:. Movement disorders. Hoboken, NJ: Wiley-Liss Div John Wiley & Sons Inc (2007)
91. Ortuño-Lizarán, I, Sánchez-Sáez, X, Lax, P, Serrano, GE, Beach, TG, Adler, CH, et al. Dopaminergic retinal cell loss and visual dysfunction in Parkinson disease. Ann Neurol. (2020) 88:893–906. doi: 10.1002/ana.25897
92. Archibald, NK, Clarke, MP, Mosimann, UP, and Burn, DJ. The retina in Parkinson's disease. Brain. (2009) 132:1128–45. doi: 10.1093/brain/awp068
93. Hajee, ME, March, WF, Lazzaro, DR, Wolintz, AH, Shrier, EM, Glazman, S, et al. Inner retinal layer thinning in Parkinson disease. Arch Ophthalmol. (2009) 127:737–41. doi: 10.1001/archophthalmol.2009.106
94. Abe, Y, Kachi, T, Kato, T, Arahata, Y, Yamada, T, Washimi, Y, et al. Occipital hypoperfusion in Parkinson’s disease without dementia: correlation to impaired cortical visual processing. J Neurol Neurosurg Psychiatry. (2003) 74:419–22. doi: 10.1136/jnnp.74.4.419
95. Lepore, FE. Parkinson’s disease and diplopia. Neuroophthalmol. (2006) 30:37–40. doi: 10.1080/01658100600742838
96. Tamer, C, Melek, IM, Duman, T, and Oksüz, H. Tear film tests in Parkinson's disease patients. Ophthalmology. (2005) 112:1795–1795.e8. doi: 10.1016/j.ophtha.2005.04.025
97. Armstrong, RA. Visual symptoms in Parkinson's disease. Parkinsons Dis. (2011) 2011:908306. doi: 10.4061/2011/908306
98. Stowe, RL, Ives, N, Clarke, CE, van Hilten,, Ferreira, J, Hawker, RJ, et al. Dopamine agonist therapy in early Parkinson's disease. Cochrane Database Syst Rev. (2008) 2:Cd006564. doi: 10.1002/14651858.CD006564.pub2
99. Linazasoro, G, van Blercom, N, Lasa, A, Indakoetxea, B, and Ruiz, J. Levodopa-induced ocular dyskinesias in Parkinson's disease. Mov Disord. (2002) 17:186–7. doi: 10.1002/mds.10017
100. Buzzi, M, Giannaccare, G, Cennamo, M, Bernabei, F, Rothschild, PR, Vagge, A, et al. Ocular surface features in patients with Parkinson disease on and off treatment: a narrative review. Life. (2022) 12:2141. doi: 10.3390/life12122141
101. Daggumilli, S, Vanathi, M, Ganger, A, Goyal, V, and Tandon, R. Corneal evaluation in patients with parkinsonism on long-term amantadine therapy. Cornea. (2019) 38:1131–6. doi: 10.1097/ICO.0000000000001951
102. Yemula, N, Dietrich, C, Dostal, V, and Hornberger, M. Parkinson’s disease and the gut: symptoms, nutrition, and microbiota. J Parkinsons Dis. (2021) 11:1491–505. doi: 10.3233/JPD-212707
103. Kwon, M, and Lee, JH. Oro-pharyngeal dysphagia in Parkinson's disease and related movement disorders. J Mov Disord. (2019) 12:152–60. doi: 10.14802/jmd.19048
104. Nielsen, HH, Qiu, J, Friis, S, Wermuth, L, and Ritz, B. Treatment for Helicobacter pylori infection and risk of Parkinson's disease in Denmark. Eur J Neurol. (2012) 19:864–9. doi: 10.1111/j.1468-1331.2011.03643.x
105. Tateno, F, Sakakibara, R, Kishi, M, Ogawa, E, Yoshimatsu, Y, Takada, N, et al. Incidence of emergency intestinal Pseudo-obstruction in Parkinson's disease. J Am Geriatr Soc. (2011) 59:2373–5. doi: 10.1111/j.1532-5415.2011.03686.x
106. Lolekha, P, Sriphanom, T, and Vilaichone, R-K. Helicobacter pylori eradication improves motor fluctuations in advanced Parkinson’s disease patients: a prospective cohort study (HP-PD trial). PLoS One. (2021) 16:e0251042. doi: 10.1371/journal.pone.0251042
107. Tan, AH, Lim, SY, Mahadeva, S, Loke, MF, Tan, JY, Ang, BH, et al. Helicobacter pylori eradication in Parkinson’s disease: a randomized placebo-controlled trial. Mov Disord. (2020) 35:2250–60. doi: 10.1002/mds.28248
108. Dukowicz, AC, Lacy, BE, and Levine, GM. Small intestinal bacterial overgrowth: a comprehensive review. Gastroenterol Hepatol. (2007) 3:112–22.
109. Dănău, A, Dumitrescu, L, Lefter, A, Tulbă, D, and Popescu, BO. Small intestinal bacterial overgrowth as potential therapeutic target in Parkinson's disease. Int J Mol Sci. (2021) 22:11663. doi: 10.3390/ijms222111663
110. Leta, V, Ray Chaudhuri, K, Milner, O, Chung-Faye, G, Metta, V, Pariante, CM, et al. Neurogenic and anti-inflammatory effects of probiotics in Parkinson’s disease: a systematic review of preclinical and clinical evidence. Brain Behav Immun. (2021) 98:59–73. doi: 10.1016/j.bbi.2021.07.026
111. Boertien, JM, Pereira, PAB, Aho, VTE, and Scheperjans, F. Increasing comparability and utility of gut microbiome studies in Parkinson’s disease: a systematic review. J Parkinsons Dis. (2019) 9:S297–312. doi: 10.3233/JPD-191711
112. Romano, S, Savva, GM, Bedarf, JR, Charles, IG, Hildebrand, F, and Narbad, A. Meta-analysis of the Parkinson's disease gut microbiome suggests alterations linked to intestinal inflammation. Parkinson’s Dis. (2021) 7:27. doi: 10.1038/s41531-021-00156-z
113. Nakayama, Y, Washio, M, and Mori, M. Oral health conditions in patients with Parkinson’s disease. J Epidemiol. (2004) 14:143–50. doi: 10.2188/jea.14.143
114. Auffret, M, Meuric, V, Boyer, E, Bonnaure-Mallet, M, and Vérin, M. Oral health disorders in Parkinson’s disease: more than meets the eye. J Parkinsons Dis. (2021) 11:1507–35. doi: 10.3233/JPD-212605
115. Kaur, T, Uppoor, A, and Naik, D. Parkinson’s disease and periodontitis – the missing link? A review. Gerodontology. (2016) 33:434–8. doi: 10.1111/ger.12188
116. Fleury, V, Zekeridou, A, Lazarevic, V, Gaïa, N, Giannopoulou, C, Genton, L, et al. Oral dysbiosis and inflammation in Parkinson’s disease. J Parkinsons Dis. (2021) 11:619–31. doi: 10.3233/JPD-202459
117. Mihaila, D, Donegan, J, Barns, S, LaRocca, D, du, Q, Zheng, D, et al. The oral microbiome of early stage Parkinson’s disease and its relationship with functional measures of motor and non-motor function. PLoS One. (2019) 14:e0218252. doi: 10.1371/journal.pone.0218252
118. Lyra, P, Botelho, J, Machado, V, Rota, S, Walker, R, Staunton, J, et al. Self-reported periodontitis and C-reactive protein in Parkinson’s disease: a cross-sectional study of two American cohorts. NPJ Parkinsons Dis. (2022) 8:40. doi: 10.1038/s41531-022-00302-1
119. Adams, B, Nunes, JM, Page, MJ, Roberts, T, Carr, J, Nell, TA, et al. Parkinson’s disease: a systemic inflammatory disease accompanied by bacterial inflammagens. Front Aging Neurosci. (2019) 11:210. doi: 10.3389/fnagi.2019.00210
120. Botelho, J, Lyra, P, Proença, L, Godinho, C, Mendes, JJ, and Machado, V. Relationship between blood and standard biochemistry levels with periodontitis in Parkinson’s disease patients: data from the NHANES 2011-2012. J Pers Med. (2020) 10:69. doi: 10.3390/jpm10030069
121. Li, D, Ren, T, Li, H, Liao, G, and Zhang, X. Porphyromonas gingivalis: a key role in Parkinson’s disease with cognitive impairment? Front Neurol. (2022) 13:945523. doi: 10.3389/fneur.2022.945523
122. Feng, YK, Wu, QL, Peng, YW, Liang, FY, You, HJ, Feng, YW, et al. Oral P. gingivalis impairs gut permeability and mediates immune responses associated with neurodegeneration in LRRK2 R1441G mice. J Neuroinflammation. (2020) 17:347. doi: 10.1186/s12974-020-02027-5
123. van Wamelen, DJ, Leta, V, Johnson, J, Ocampo, CL, Podlewska, AM, Rukavina, K, et al. Drooling in Parkinson’s disease: prevalence and progression from the non-motor international longitudinal study. Dysphagia. (2020) 35:955–61. doi: 10.1007/s00455-020-10102-5
124. Meningaud, JP, Pitak-Arnnop, P, Chikhani, L, and Bertrand, JC. Drooling of saliva: a review of the etiology and management options. Oral Surg Oral Med Oral Pathol Oral Radiol Endod. (2006) 101:48–57. doi: 10.1016/j.tripleo.2005.08.018
125. Lee, JY, Ahn, J, Kim, TW, and Jeon, BS. Optical coherence tomography in Parkinson’s disease: is the retina a biomarker? J Parkinsons Dis. (2014) 4:197–204. doi: 10.3233/JPD-130306
126. Jiménez, B, Ascaso, FJ, Cristóbal, JA, and López del Val, J. Development of a prediction formula of Parkinson disease severity by optical coherence tomography. Mov Disord. (2014) 29:68–74. doi: 10.1002/mds.25747
127. Altintaş, Ö, Işeri, P, Özkan, B, and Çağlar, Y. Correlation between retinal morphological and functional findings and clinical severity in Parkinson’s disease. Doc Ophthalmol. (2008) 116:137–46. doi: 10.1007/s10633-007-9091-8
128. Nowacka, B, Lubiński, W, Honczarenko, K, Potemkowski, A, and Safranow, K. Bioelectrical function and structural assessment of the retina in patients with early stages of Parkinson's disease (PD). Doc Ophthalmol. (2015) 131:95–104. doi: 10.1007/s10633-015-9503-0
129. Burguera, JA, Vilela, C, Traba, A, Ameave, Y, and Vallet, M. The electroretinogram and visual evoked potentials in patients with Parkinson’s disease. Arch Neurobiol. (1990) 53:1–7.
130. Mello, LGM, Paraguay, IBB, Andrade, TS, Rocha, AAN, Barbosa, ER, Oyamada, MK, et al. Electroretinography reveals retinal dysfunction in Parkinson’s disease despite normal high-resolution optical coherence tomography findings. Parkinsonism Relat Disord. (2022) 101:90–5. doi: 10.1016/j.parkreldis.2022.06.018
131. He, SB, Liu, CY, Chen, LD, Ye, ZN, Zhang, YP, Tang, WG, et al. Meta-analysis of visual evoked potential and Parkinson's disease. Parkinsons Dis. (2018) 2018:3201308. doi: 10.1155/2018/3201308
132. Tavakoli, M, and Malik, RA. Corneal confocal microscopy: a novel non-invasive technique to quantify small fibre pathology in peripheral neuropathies. J Vis Exp. (2011) 47:2194. doi: 10.3791/2194
133. Kass-Iliyya, L, Javed, S, Gosal, D, Kobylecki, C, Marshall, A, Petropoulos, IN, et al. Small fiber neuropathy in Parkinson's disease: a clinical, pathological and corneal confocal microscopy study. Parkinsonism Relat Disord. (2015) 21:1454–60. doi: 10.1016/j.parkreldis.2015.10.019
134. Lim, SH, Ferdousi, M, Kalteniece, A, Kass-Iliyya, L, Petropoulos, IN, Malik, RA, et al. Corneal confocal microscopy detects small fibre neurodegeneration in Parkinson's disease using automated analysis. Sci Rep. (2020) 10:20147. doi: 10.1038/s41598-020-76768-x
135. Che, NN, Jiang, QH, Ding, GX, Chen, SY, Zhao, ZX, Li, X, et al. Corneal nerve fiber loss relates to cognitive impairment in patients with Parkinson's disease. NPJ Parkinsons Dis. (2021) 7:80. doi: 10.1038/s41531-021-00225-3
136. Che, NN, Chen, S, Jiang, QH, Chen, SY, Zhao, ZX, Li, X, et al. Corneal confocal microscopy differentiates patients with Parkinson's disease with and without autonomic involvement. NPJ Parkinsons Dis. (2022) 8:114. doi: 10.1038/s41531-022-00387-8
137. Lim, SH, Ferdousi, M, Kalteniece, A, Mahfoud, ZR, Petropoulos, IN, Malik, RA, et al. Corneal confocal microscopy identifies Parkinson's disease with more rapid motor progression. Mov Disord. (2021) 36:1927–34. doi: 10.1002/mds.28602
138. Söğütlü Sarı, E, Koçak, R, Yazıcı, A, Şahin, G, Çakmak, H, Kocatürk, T, et al. Tear osmolarity, break-up time and schirmer's scores in Parkinson's disease. Turk J Ophthalmol. (2015) 45:142–5. doi: 10.4274/tjo.46547
139. Aksoy, D, Ortak, H, Kurt, S, Cevik, E, and Cevik, B. Central corneal thickness and its relationship to Parkinson's disease severity. Can J Ophthalmol. (2014) 49:152–6. doi: 10.1016/j.jcjo.2013.12.010
140. Lew, M, Janga, S, Ju, Y, Feigenbaum, D, Besharat, A, Freire, D, et al. Biomarkers for Parkinson’s disease with reflex tears stratified by disease duration (S16.002). Neurology. (2022) 98:3750
141. Clifford, LJ, Turnbull, AMJ, and Denning, AM. Reversible night blindness – a reminder of the increasing importance of vitamin a deficiency in the developed world. J Optom. (2013) 6:173–4. doi: 10.1016/j.optom.2013.01.002
142. Fullard, ME, and Duda, JE. A review of the relationship between vitamin D and Parkinson disease symptoms. Front Neurol. (2020) 11:454. doi: 10.3389/fneur.2020.00454
143. Dietiker, C, Kim, S, Zhang, Y, and Christine, CW. Characterization of vitamin B12 supplementation and correlation with clinical outcomes in a large longitudinal study of early Parkinson’s disease. J Mov Disord. (2019) 12:91–6. doi: 10.14802/jmd.18049
144. Marie, A, Darricau, M, Touyarot, K, Parr-Brownlie, LC, and Bosch-Bouju, C. Role and mechanism of vitamin a metabolism in the pathophysiology of Parkinson’s disease. J Parkinsons Dis. (2021) 11:949–70. doi: 10.3233/JPD-212671
145. Srivanitchapoom, P, Pandey, S, and Hallett, M. Drooling in Parkinson's disease: a review. Parkinsonism Relat Disord. (2014) 20:1109–18. doi: 10.1016/j.parkreldis.2014.08.013
146. Cosentino, G, Avenali, M, Schindler, A, Pizzorni, N, Montomoli, C, Abbruzzese, G, et al. A multinational consensus on dysphagia in Parkinson's disease: screening, diagnosis and prognostic value. J Neurol. (2022) 269:1335–52. doi: 10.1007/s00415-021-10739-8
147. Alexander, SS. Imaging of constipation and its complications In: M Gyula, editor. Constipation. IntechOpen: Rijeka (2018)
148. Wang, YK, Kuo, FC, Liu, CJ, Wu, MC, Shih, HY, Wang, SS, et al. Diagnosis of Helicobacter pylori infection: current options and developments. World J Gastroenterol. (2015) 21:11221–35. doi: 10.3748/wjg.v21.i40.11221
149. Banik, GD, de, A, Som, S, Jana, S, Daschakraborty, SB, Chaudhuri, S, et al. Hydrogen sulphide in exhaled breath: a potential biomarker for small intestinal bacterial overgrowth in IBS. J Breath Res. (2016) 10:026010. doi: 10.1088/1752-7155/10/2/026010
150. Martimbianco, ALC, Prosdocimi, FC, Anauate-Netto, C, dos Santos, EM, Mendes, GD, and Fragoso, YD. Evidence-based recommendations for the oral health of patients with Parkinson’s disease. Neurol Ther. (2021) 10:391–400. doi: 10.1007/s40120-021-00237-4
151. Proulx, M, de Courval, FP, Wiseman, MA, and Panisset, M. Salivary production in Parkinson's disease. Mov Disord. (2005) 20:204–7. doi: 10.1002/mds.20189
152. Turk-Gonzales, M, and Odderson, IR. Quantitative reduction of saliva production with botulinum toxin type B injection into the salivary glands. Neurorehabil Neural Repair. (2005) 19:58–61. doi: 10.1177/1545968304273201
153. Marks, L, Turner, K, O'sullivan, J, Deighton, B, and Lees, A. Drooling in Parkinson’s disease: a novel speech and language therapy intervention. Int J Lang Commun Disord. (2001) 36:282–7. doi: 10.3109/13682820109177898
154. Ellies, M, Gottstein, U, Rohrbach-Volland, S, Arglebe, C, and Laskawi, R. Reduction of salivary flow with botulinum toxin: extended report on 33 patients with drooling, salivary fistulas, and sialadenitis. Laryngoscope. (2004) 114:1856–60. doi: 10.1097/00005537-200410000-00033
155. Invernizzi, M, Carda, S, Viscontini, GS, and Viscontini, GS. Osteoporosis in Parkinson's disease. Parkinsonism Relat Disord. (2009) 15:339–46. doi: 10.1016/j.parkreldis.2009.02.009
156. Dennison, EM, Compston, JE, Flahive, J, Siris, ES, Gehlbach, SH, Adachi, JD, et al. Effect of co-morbidities on fracture risk: findings from the global longitudinal study of osteoporosis in women (GLOW). Bone. (2012) 50:1288–93. doi: 10.1016/j.bone.2012.02.639
157. Metta, V, Sanchez, TC, and Padmakumar, C. Osteoporosis: a hidden nonmotor face of Parkinson's disease. Int Rev Neurobiol. (2017) 134:877–90. doi: 10.1016/bs.irn.2017.05.034
158. Handa, K, Kiyohara, S, Yamakawa, T, Ishikawa, K, Hosonuma, M, Sakai, N, et al. Bone loss caused by dopaminergic degeneration and levodopa treatment in Parkinson’s disease model mice. Sci Rep. (2019) 9:13768. doi: 10.1038/s41598-019-50336-4
159. Contreras, A, and Grandas, F. Risk of falls in Parkinson's disease: a cross-sectional study of 160 patients. Parkinsons Dis. (2012) 2012:362572. doi: 10.1155/2012/362572
160. Pelicioni, PHS, Menant, JC, Latt, MD, and Lord, SR. Falls in Parkinson's disease subtypes: risk factors, locations and circumstances. Int J Environ Res Public Health. (2019) 16:2216. doi: 10.3390/ijerph16122216
161. Harris, DM, Duckham, RL, Daly, RM, Abbott, G, Johnson, L, Rantalainen, T, et al. Development of a Parkinson’s disease specific falls questionnaire. BMC Geriatr. (2021) 21:614. doi: 10.1186/s12877-021-02555-6
162. COPPADIS Study GroupSantos García, D, de Deus Fonticoba, T, Cores, C, Suárez Castro, E, Hernández Vara, J, et al. Falls predict acute hospitalization in Parkinson’s disease. J Parkinsons Dis. (2023) 13:105–24. doi: 10.3233/JPD-212539
163. Tan, YJ, Lim, SY, Yong, VW, Choo, XY, Ng, YD, Sugumaran, K, et al. Osteoporosis in Parkinson's disease: relevance of distal radius dual-energy X-ray absorptiometry (DXA) and sarcopenia. J Clin Densitom. (2021) 24:351–61. doi: 10.1016/j.jocd.2020.07.001
164. Caplliure-Llopis, J, Escrivá, D, Navarro-Illana, E, Benlloch, M, de la Rubia Ortí, JE, and Barrios, C. Bone quality in patients with Parkinson’s disease determined by quantitative ultrasound (QUS) of the calcaneus: influence of sex differences. Int J Environ Res Public Health. (2022) 19:2804. doi: 10.3390/ijerph19052804
165. van den Bos, F, Speelman, AD, Nimwegen, M, Schouw, YT, Backx, FJG, Bloem, BR, et al. Bone mineral density and vitamin D status in Parkinson's disease patients. J Neurol. (2013) 260:754–60. doi: 10.1007/s00415-012-6697-x
166. Abou-Raya, S, Helmii, M, and Abou-Raya, A. Bone and mineral metabolism in older adults with Parkinson’s disease. Age Ageing. (2009) 38:675–80. doi: 10.1093/ageing/afp137
167. Shribman, S, Torsney, KM, Noyce, AJ, Giovannoni, G, Fearnley, J, and Dobson, R. A service development study of the assessment and management of fracture risk in Parkinson's disease. J Neurol. (2014) 261:1153–9. doi: 10.1007/s00415-014-7333-8
168. Lyell, V, Henderson, E, Devine, M, and Gregson, C. Assessment and management of fracture risk in patients with Parkinson's disease. Age Ageing. (2015) 44:34–41. doi: 10.1093/ageing/afu122
169. Schini, M, Bhatia, P, Shreef, H, Johansson, H, Harvey, NC, Lorentzon, M, et al. Increased fracture risk in Parkinson's disease – an exploration of mechanisms and consequences for fracture prediction with FRAX. Bone. (2023) 168:116651. doi: 10.1016/j.bone.2022.116651
170. Silva de Lima, AL, Smits, T, Darweesh, SKL, Valenti, G, Milosevic, M, Pijl, M, et al. Home-based monitoring of falls using wearable sensors in Parkinson’s disease. Mov Disord. (2020) 35:109–15. doi: 10.1002/mds.27830
171. Lu, R, Xu, Y, Li, X, Fan, Y, Zeng, W, Tan, Y, et al. Evaluation of wearable sensor devices in Parkinson’s disease: a review of current status and future prospects. Parkinsons Dis. (2020) 2020:4693019. doi: 10.1155/2020/4693019
172. Gil-Prieto, R, Pascual-Garcia, R, San-Roman-Montero, J, Martinez-Martin, P, Castrodeza-Sanz, J, and Gil-de-Miguel, A. Measuring the burden of hospitalization in patients with Parkinson’s disease in Spain. PLoS One. (2016) 11:e0151563. doi: 10.1371/journal.pone.0151563
173. Pohar, SL, and Allyson Jones, C. The burden of Parkinson disease (PD) and concomitant comorbidities. Arch Gerontol Geriatr. (2009) 49:317–21. doi: 10.1016/j.archger.2008.11.006
174. Wang, X, Zeng, F, Jin, WS, Zhu, C, Wang, QH, Bu, XL, et al. Comorbidity burden of patients with Parkinson's disease and parkinsonism between 2003 and 2012: a multicentre, nationwide, retrospective study in China. Sci Rep. (2017) 7:1671. doi: 10.1038/s41598-017-01795-0
175. Nuyen, J, Schellevis, FG, Satariano, WA, Spreeuwenberg, PM, Birkner, MD, van den Bos, GAM, et al. Comorbidity was associated with neurologic and psychiatric diseases: a general practice-based controlled study. J Clin Epidemiol. (2006) 59:1274–84. doi: 10.1016/j.jclinepi.2006.01.005
176. McLean, G, Hindle, JV, Guthrie, B, and Mercer, SW. Co-morbidity and polypharmacy in Parkinson’s disease: insights from a large Scottish primary care database. BMC Neurol. (2017) 17:126. doi: 10.1186/s12883-017-0904-4
177. Xu, K, Alnaji, N, Zhao, J, Bertoni, JM, Chen, LW, Bhatti, D, et al. Comorbid conditions in Parkinson’s disease: a population-based study of statewide Parkinson’s disease registry. Neuroepidemiology. (2018) 50:7–17. doi: 10.1159/000484410
178. Palakurthi, B, and Burugupally, SP. Postural instability in Parkinson's disease: a review. Brain Sci. (2019) 9:239. doi: 10.3390/brainsci9090239
179. Park, J-H, and Kang, SY. Dizziness in Parkinson’s disease patients is associated with vestibular function. Sci Rep. (2021) 11:18976. doi: 10.1038/s41598-021-98540-5
180. Schrag, A, Bohlken, J, Dammertz, L, Teipel, S, Hermann, W, Akmatov, MK, et al. Widening the Spectrum of risk factors, comorbidities, and prodromal features of Parkinson disease. JAMA Neurol. (2023) 80:161. doi: 10.1001/jamaneurol.2022.3902
181. Bhagavathula, AS, Tesfaye, W, Vidyasagar, K, and Fialova, D. Polypharmacy and hyperpolypharmacy in older individuals with Parkinson's disease: a systematic review and meta-analysis. Gerontology. (2022) 68:1081–90. doi: 10.1159/000521214
182. Giovannini, S, Laudisio, A, Navarini, L, Lo Monaco, MR, Ciaburri, M, Serafini, E, et al. Polypharmacy is a determinant of hospitalization in Parkinson’s disease. Eur Rev Med Pharmacol Sci. (2021) 25:4810–7. doi: 10.26355/eurrev_202107_26394
183. Frucht, S, Rogers, JD, Greene, PE, Gordon, MF, and Fahn, S. Falling asleep at the wheel: motor vehicle mishaps in persons taking pramipexole and ropinirole. Neurology. (1999) 52:1908–10. doi: 10.1212/WNL.52.9.1908
184. Borovac, JA. Side effects of a dopamine agonist therapy for Parkinson's disease: a mini-review of clinical pharmacology. Yale J Biol Med. (2016) 89:37–47.
185. Samuel, M, Rodriguez-Oroz, M, Antonini, A, Brotchie, JM, Ray Chaudhuri, K, Brown, RG, et al. Management of impulse control disorders in Parkinson's disease: controversies and future approaches. Mov Disord. (2015) 30:150–9. doi: 10.1002/mds.26099
186. Pagano, G, Polychronis, S, Wilson, H, Giordano, B, Ferrara, N, Niccolini, F, et al. Diabetes mellitus and Parkinson disease. Neurology. (2018) 90:e1654–62. doi: 10.1212/WNL.0000000000005475
187. Chohan, H, Senkevich, K, Patel, RK, Bestwick, JP, Jacobs, BM, Bandres Ciga, S, et al. Type 2 diabetes as a determinant of Parkinson's disease risk and progression. Mov Disord. (2021) 36:1420–9. doi: 10.1002/mds.28551
188. Markaki, I, Ntetsika, T, Sorjonen, K, and Svenningsson, P, for the BioPark Study Group. Euglycemia indicates favorable motor outcome in Parkinson's disease. Mov Disord. (2021) 36:1430–4. doi: 10.1002/mds.28545
189. Zittel, S, Uyar, M, Lezius, S, Gerloff, C, and Choe, CU. HbA1c and motor outcome in Parkinson's disease in the mark-PD study. Mov Disord. (2021) 36:1991–2. doi: 10.1002/mds.28689
190. Huxford, B, Haque, T, Joseph, AB, Simonet, C, Gallagher, D, Budu, C, et al. Parkinson's disease and type 2 diabetes: HbA1c is associated with motor and cognitive severity. Mov Disord. (2022) 37:427–8. doi: 10.1002/mds.28829
191. Uyar, M, Lezius, S, Buhmann, C, Pötter-Nerger, M, Schulz, R, Meier, S, et al. Diabetes, glycated hemoglobin (HbA1c), and neuroaxonal damage in Parkinson's disease (MARK-PD study). Mov Disord. (2022) 37:1299–304. doi: 10.1002/mds.29009
192. Vijiaratnam, N, Lawton, M, Real, R, Heslegrave, AJ, Guo, T, Athauda, D, et al. Diabetes and neuroaxonal damage in Parkinson’s disease. Mov Disord. (2022) 37:1568–9. doi: 10.1002/mds.29067
193. Kistner, A, Lhommée, E, and Krack, P. Mechanisms of body weight fluctuations in Parkinson's disease. Front Neurol. (2014) 5:84. doi: 10.3389/fneur.2014.00084
194. Logroscino, G, Sesso, HD, Paffenbarger, RS, and Lee, IM. Body mass index and risk of Parkinson's disease: a prospective cohort study. Am J Epidemiol. (2007) 166:1186–90. doi: 10.1093/aje/kwm211
195. van der Marck, MA, Dicke, HC, Uc, EY, Kentin, ZHA, Borm, GF, Bloem, BR, et al. Body mass index in Parkinson’s disease: a meta-analysis. Parkinsonism Relat Disord. (2012) 18:263–7. doi: 10.1016/j.parkreldis.2011.10.016
196. Yoon, SY, Heo, SJ, Lee, HJ, Shin, J, Kim, YW, Yang, SN, et al. Initial BMI and weight loss over time predict mortality in Parkinson disease. J Am Med Dir Assoc. (2022) 23:1719.e1–7. doi: 10.1016/j.jamda.2022.07.015
197. Urso, D, van Wamelen, DJ, Batzu, L, Leta, V, Staunton, J, Pineda-Pardo, JA, et al. Clinical trajectories and biomarkers for weight variability in early Parkinson’s disease. NPJ Parkinsons Dis. (2022) 8:95. doi: 10.1038/s41531-022-00362-3
198. Zesiewicz, TA, Baker, MJ, Wahba, M, and Hauser, RA. Autonomic nervous system dysfunction in Parkinson’s disease. Curr Treat Options Neurol. (2003) 5:149–60. doi: 10.1007/s11940-003-0005-0
199. Tsukamoto, T, Kitano, Y, and Kuno, S. Blood pressure fluctuation and hypertension in patients with Parkinson’s disease. Brain Behav. (2013) 3:710–4. doi: 10.1002/brb3.179
200. Kim, YH, Kim, Y, Yoon, J, Cho, YS, Kym, D, Hur, J, et al. Frontal lobe hemodynamics detected by functional near-infrared spectroscopy during head-up tilt table tests in patients with electrical burns. Front Hum Neurosci. (2022) 16:986230. doi: 10.3389/fnhum.2022.986230
201. Santos García, D, Suárez Castro, E, Expósito, I, de Deus, T, Tuñas, C, Aneiros, A, et al. Comorbid conditions associated with Parkinson's disease: a longitudinal and comparative study with Alzheimer disease and control subjects. J Neurol Sci. (2017) 373:210–5. doi: 10.1016/j.jns.2016.12.046
202. Stawicki, SP, Kalra, S, Jones, C, Justiniano, CF, Papadimos, TJ, Galwankar, SC, et al. Comorbidity polypharmacy score and its clinical utility: a pragmatic practitioner's perspective. J Emerg Trauma Shock. (2015) 8:224–31. doi: 10.4103/0974-2700.161658
203. Narayan, SW, Pearson, SA, Litchfield, M, le Couteur, DG, Buckley, N, McLachlan, AJ, et al. Anticholinergic medicines use among older adults before and after initiating dementia medicines. Br J Clin Pharmacol. (2019) 85:1957–63. doi: 10.1111/bcp.13976
204. Cochrane Dementia and Cognitive Improvement GroupTaylor-Rowan, M, Edwards, S, Noel-Storr, AH, McCleery, J, Myint, PK, et al. Anticholinergic burden (prognostic factor) for prediction of dementia or cognitive decline in older adults with no known cognitive syndrome. Cochrane Database Syst Rev. (2021) 2021:Cd013540. doi: 10.1002/14651858.CD013540.pub2
205. Sumbul-Sekerci, B, Bilgic, B, Pasin, O, Emre, M, and Hanagasi, HA. Anticholinergic burden, polypharmacy, and cognition in Parkinson's disease patients with mild cognitive impairment: a cross-sectional observational study. Dement Geriatr Cogn Disord. (2023) 51:386–95. doi: 10.1159/000526863
206. Carnahan, RM, Lund, BC, Perry, PJ, Pollock, BG, and Culp, KR. The anticholinergic drug scale as a measure of drug-related anticholinergic burden: associations with serum anticholinergic activity. J Clin Pharmacol. (2006) 46:1481–6. doi: 10.1177/0091270006292126
207. Marín-Lahoz, J, Martinez-Horta, S, Pagonabarraga, J, Horta-Barba, A, Aracil-Bolaños, I, Bejr-kasem, H, et al. Predicting impulse control disorders in Parkinson disease through incentive biomarkers. Ann Neurol. (2022) 92:974–84. doi: 10.1002/ana.26486
208. Weintraub, D, Posavi, M, Fontanillas, P, Tropea, TF, Mamikonyan, E, Suh, E, et al. Genetic prediction of impulse control disorders in Parkinson's disease. Ann Clin Transl Neurol. (2022) 9:936–49. doi: 10.1002/acn3.51569
209. Chaudhuri, KR, Pal, S, and Brefel-Courbon, C. 'Sleep attacks' or 'unintended sleep episodes' occur with dopamine agonists: is this a class effect? Drug Saf. (2002) 25:473–83. doi: 10.2165/00002018-200225070-00001
210. Hobson, DE, Lang, AE, Martin, WRW, Razmy, A, Rivest, J, and Fleming, J. Excessive daytime sleepiness and sudden-onset sleep in Parkinson disease: a survey by the Canadian movement disorders group. JAMA. (2002) 287:455–63. doi: 10.1001/jama.287.4.455
211. Poryazova, R, Benninger, D, Waldvogel, D, and Bassetti, CL. Excessive daytime sleepiness in Parkinson’s disease: characteristics and determinants. Eur Neurol. (2010) 63:129–35. doi: 10.1159/000276402
212. Ataide, M, Franco, CM, and Lins, OG. Daytime sleepiness and Parkinson's disease: the contribution of the multiple sleep latency test. Sleep Disord. (2014) 2014:767181. doi: 10.1155/2014/767181
213. Martinez-Ramirez, D, De Jesus, S, Walz, R, Cervantes-Arriaga, A, Peng-Chen, Z, Okun, MS, et al. A polysomnographic study of Parkinson’s disease sleep architecture. Parkinson’s Disease. (2015) 2015:570375. doi: 10.1155/2015/570375
Keywords: Parkinson’s disease, motor, nonmotor, vitals, dashboard, oral, gut, vision
Citation: Qamar MA, Rota S, Batzu L, Subramanian I, Falup-Pecurariu C, Titova N, Metta V, Murasan I, Odin P, Padmakumar C, Kukkle PL, Borgohain R, Kandadai RM, Goyal V and Chaudhuri KR (2023) Chaudhuri’s Dashboard of Vitals in Parkinson’s syndrome: an unmet need underpinned by real life clinical tests. Front. Neurol. 14:1174698. doi: 10.3389/fneur.2023.1174698
Edited by:
Roberta Balestrino, San Raffaele Scientific Institute (IRCCS), ItalyReviewed by:
Carlo Alberto Artusi, University of Turin, ItalyChien Tai Hong, Taipei Medical University, Taiwan
Copyright © 2023 Qamar, Rota, Batzu, Subramanian, Falup-Pecurariu, Titova, Metta, Murasan, Odin, Padmakumar, Kukkle, Borgohain, Kandadai, Goyal and Ray Chaudhuri. This is an open-access article distributed under the terms of the Creative Commons Attribution License (CC BY). The use, distribution or reproduction in other forums is permitted, provided the original author(s) and the copyright owner(s) are credited and that the original publication in this journal is cited, in accordance with accepted academic practice. No use, distribution or reproduction is permitted which does not comply with these terms.
*Correspondence: Mubasher A. Qamar, bXViYXNoZXIucWFtYXJAbmhzLm5ldA==; Silvia Rota, c2lsdmlhLnJvdGFAa2NsLmFjLnVr
†These authors share first authorship