- 1Department of Human Neurosciences, Sapienza University of Rome, Rome, Italy
- 2IRCCS Neuromed Institute, Pozzilli, Italy
- 3Department of Neurology, James J. and Joan A. Gardner Center for Parkinson’s Disease and Movement Disorders, University of Cincinnati, Cincinnati, OH, United States
- 4Department of Electronic Engineering, University of Rome Tor Vergata, Rome, Italy
- 5CRC – Centro Ricerca e Cura, Rome, Italy
- 6Department of Sense Organs, Otorhinolaryngology Section, Sapienza University of Rome, Rome, Italy
- 7IRCCS San Raffaele Pisana, Rome, Italy
Background: Stuttering is a childhood-onset neurodevelopmental disorder affecting speech fluency. The diagnosis and clinical management of stuttering is currently based on perceptual examination and clinical scales. Standardized techniques for acoustic analysis have prompted promising results for the objective assessment of dysfluency in people with stuttering (PWS).
Objective: We assessed objectively and automatically voice in stuttering, through artificial intelligence (i.e., the support vector machine – SVM classifier). We also investigated the age-related changes affecting voice in stutterers, and verified the relevance of specific speech tasks for the objective and automatic assessment of stuttering.
Methods: Fifty-three PWS (20 children, 33 younger adults) and 71 age−/gender-matched controls (31 children, 40 younger adults) were recruited. Clinical data were assessed through clinical scales. The voluntary and sustained emission of a vowel and two sentences were recorded through smartphones. Audio samples were analyzed using a dedicated machine-learning algorithm, the SVM to compare PWS and controls, both children and younger adults. The receiver operating characteristic (ROC) curves were calculated for a description of the accuracy, for all comparisons. The likelihood ratio (LR), was calculated for each PWS during all speech tasks, for clinical-instrumental correlations, by using an artificial neural network (ANN).
Results: Acoustic analysis based on machine-learning algorithm objectively and automatically discriminated between the overall cohort of PWS and controls with high accuracy (88%). Also, physiologic ageing crucially influenced stuttering as demonstrated by the high accuracy (92%) of machine-learning analysis when classifying children and younger adults PWS. The diagnostic accuracies achieved by machine-learning analysis were comparable for each speech task. The significant clinical-instrumental correlations between LRs and clinical scales supported the biological plausibility of our findings.
Conclusion: Acoustic analysis based on artificial intelligence (SVM) represents a reliable tool for the objective and automatic recognition of stuttering and its relationship with physiologic ageing. The accuracy of the automatic classification is high and independent of the speech task. Machine-learning analysis would help clinicians in the objective diagnosis and clinical management of stuttering. The digital collection of audio samples here achieved through smartphones would promote the future application of the technique in a telemedicine context (home environment).
1. Introduction
Stuttering is a persistent childhood-onset neurodevelopmental disorder affecting speech fluency (1), typically manifested in 30–48 months old children, and affecting 5%–8% of preschool children (M:F ratio = 1.5:1) (2). Clinically, people with stuttering (PWS) manifest “dysfluency,” namely the deterioration of speech fluency, with a variable association of involuntary, audible, or silent repetitions or prolongations of sounds, syllables, words, sentences, dysrhythmic phonation, such as blocks and prolongations, and broken words (1, 3). PWS may not be able to readily control the impaired vocalization and may also manifest involuntary movements and emotions including fear, embarrassment, or irritation (4–6). The complexity of stuttering behavior tends to change with age, and seems independent from sex, anxiety, or from its intrinsic clinical severity (7). Indeed, stuttering improves with physiologic ageing, thus reducing the prevalence of stuttering at 1% of younger adults starting from the age of 15 (M:F ratio = 4:1) (2, 8, 9). It has been suggested that functional changes in the phonatory apparatus occurring during puberty may play a role in the age-related reduction of stuttering (7). Stuttering is a complex and multifactorial neurodevelopmental disorder whose pathophysiologic mechanisms are supposed to rely on the impairment of several neural networks underlying speech, language, and emotional functions (8, 10).
Currently, the diagnosis of stuttering is based on neuropsychologic (i.e., perceptual) clinical examination with the aid of dedicated clinical scales for the assessment of additional developmental disorders (4, 11, 12). However, clinical scales are qualitative tools which rely on the examiner’s skills and experiences, thus potentially biasing the assessment’s accuracy. To overcome these limitations, as an objective tool for assessing stuttering several authors have adopted acoustic analysis and reported several changes in specific features (13–26). Previous acoustic analysis, however, did not allow to detect the severity of stuttering and monitor its progression. Hence, novel approaches are required for a thorough objective evaluation and assessment of dysfluencies in PWS, at different ages of life.
More recently, artificial intelligence has demonstrated its potential utility in the objective assessment of the human voice under several physiologic and neurologic conditions (27) including laryngeal dystonia, essential tremor, and Parkinson’s disease (28–34). To date, only preliminary data have been reported in PWS, by using machine-learning algorithms, thus showing promising but still preliminary and heterogeneous results. These studies applied automatic stuttering identification systems (ASIS) to a heterogenous dataset of audio samples without a thorough clinical characterization (35–37). Also, most of the previous studies have not considered the effect of ageing on acoustic features in stuttering. Despite a few clinical investigations of stuttering relative to ageing (38), a detailed data-driven analysis in different age-related groups in PWS has never been conducted, so far. Furthermore, none of the previous studies in the field have thoroughly assessed the detrimental effect of linguistic issues, namely worsening of speech in response to specific linguistic tasks. Finally, previous studies on machine-learning in stuttering have not used devices for audio recordings in an ecological scenario.
In the present study, we applied an acoustic analysis based on support vector machine (SVM) classifier to detect abnormal acoustic features in stuttering with the aim of helping clinicians in the automatic and objective classification of stuttering. For this purposes, a large cohort of PWS and controls underwent a thorough clinical investigation, including dedicated clinical scales. The second aim of the study was to assess the effect of ageing on stuttering. Therefore, our cohort included two age-related groups: children (7–12 years old), and young adults (15–30 years old). A further aim of the study was to verify the detrimental effect of linguistic issues on stuttering. Accordingly specific speech tasks have been recorded for each participant. Finally, we aimed to assess the usefulness of machine learning analysis for telemedicine purposes, by recording audio samples with commonly available devices, in an ecological scenario. The sensitivity, specificity, positive/negative predictive values, and accuracy of all diagnostic tests were assessed in detail. Furthermore, we calculated the area under the receiver operating characteristic (ROC) curves to verify the optimal diagnostic threshold as reflected by the associated criterion (Ass. Crit.) and Youden Index (YI). Finally, clinical scale outcomes were correlated with the likelihood ratios (LRs), continuous numerical values providing a measure of stuttering severity for each patient as calculated through feed-forward artificial neural network (ANN) analysis.
2. Materials and methods
2.1. Participants
We recruited a cohort of 53 people with stuttering (24 females, 29 males; mean age ± SD 16.7 ± 7.6 years, range 7–30) and a group of 71 age- and sex-matched controls (29 females, 44 males; mean age ± SD 16.2 ± 6.5 years, range 7–30). Participants or their legal guardians gave consent to the study, which was approved by the local Institutional Review Board (0026508/2019), following the Declaration of Helsinki. Depending on the age, PWS were included into two independent sex-matched subgroups: 20 children in prepubertal age (cPWS) (9 females, 11 males; mean age ± SD 9.1 ± 1.6 years, range 7–12), and 33 young adults in post-pubertal age (yPWS) (15 females, 18 males; mean age ± SD 21.3 ± 5.8 years, range 15–30). Similarly, 31 controls were included in the subgroup of children (cC) (12 females, 19 males; mean age ± SD 9.6 ± 1.5 years, range 7–12), and in the subgroup of 40 young adults (yC) (17 females, 23 males; mean age ± SD 21.1 ± 3.9 years, range 15–30). Participants were recruited at “Centro Ricerca e Cura Balbuzie – Disturbi del Linguaggio e dell’Apprendimento” in Rome, Italy. All participants were native Italian speakers, and non-smokers, and did not manifest cognitive or mood impairment, unilateral/bilateral hearing loss, respiratory disorders, and other disorders potentially affecting the vocal cords. None of the participants was taking drugs acting on the central nervous system at the time of the study. Demographic and anthropometric parameters were collected during the enrollment visit. Also, symptoms related to stuttering were scored using the Italian validated version of the Voice Handicap Index (VHI) (11, 39), the Stuttering Severity Instrument (SSI)-4 and the Stuttering Severity Scale (SSS) (4). In PWS and controls, cognitive function and mood were assessed using the Mini-Mental State Evaluation (MMSE) (40), the Frontal Assessment Battery (FAB) scale (41) and the Hamilton depression scale (HAM-D) (42). Participant demographic and clinical features are reported in Table 1.
2.1.1. Audio recordings and machine-learning analysis
The recording session started by asking participants to sit on a chair in the middle of a silent room, at home. By sending the written experimental paradigm via email, participants were instructed to handle and face a smartphone at about 30 cm from the mouth and then to speak with their usual voice intensity, pitch, and quality. For audio recordings, participants used smartphones currently available in the market (various brands including Apple®, Google®, Samsung®, Huawei®, Xiaomi®, and Asus®). For both patients and controls, the experimental design included a single recording session based on three separate speech tasks. The first speech task consisted of the sustained emission of the vowel/e/ for 5 s, whereas the second and third tasks consisted of the reading of samples of connected speech. More in detail, the second speech task was the following Italian phonetically balanced sentence: “Nella casa in riva al mare maria vide tre cani bianchi e neri” (s1) (29, 32), whereas the last speech task was a rich-in-occlusive-consonant Italian sentence: “Poichè la principessa non tornava al castello, la regina ordinò alle guardie di cercarla nal bosco” (s2), known to induce detrimental effects on stuttering. Audio recordings were collected according to a previously reported standardized procedure (29, 32, 43). To simplify the procedures of home-made audio recording, all participants were asked to save the audio tracks in mp4 format at the end of the recording session and then, to send audio tracks by encrypted e-mail to our institutional mail server, which was protected by password and accessible only by the authors. Lastly, a segmentation procedure was applied to separate each audio track into single recordings of speech samples (Audacity®) (29). The machine-learning analysis was based on specific and standardized algorithms, namely the SVM, in agreement with previous works by our group in the field (44–47). The classification analysis was based on SVM built with a linear kernel for binary classifications. Training of SVM classifier consisted of the first 30 most relevant features ranked by the CAE, in order to reduce the number of selected features needed to perform the classification and to reduce the probability of overfitting. A list of the first 30 features which represent functionals applied to audio LLDs – extracted from the vowel for the comparison between PWS and controls is reported in Table 2. A detailed discussion on technical issues concerning the analysis has been provided in Supplementary material 1. Also, the experimental paradigm is summarized in Figure 1 and Supplementary file 1.
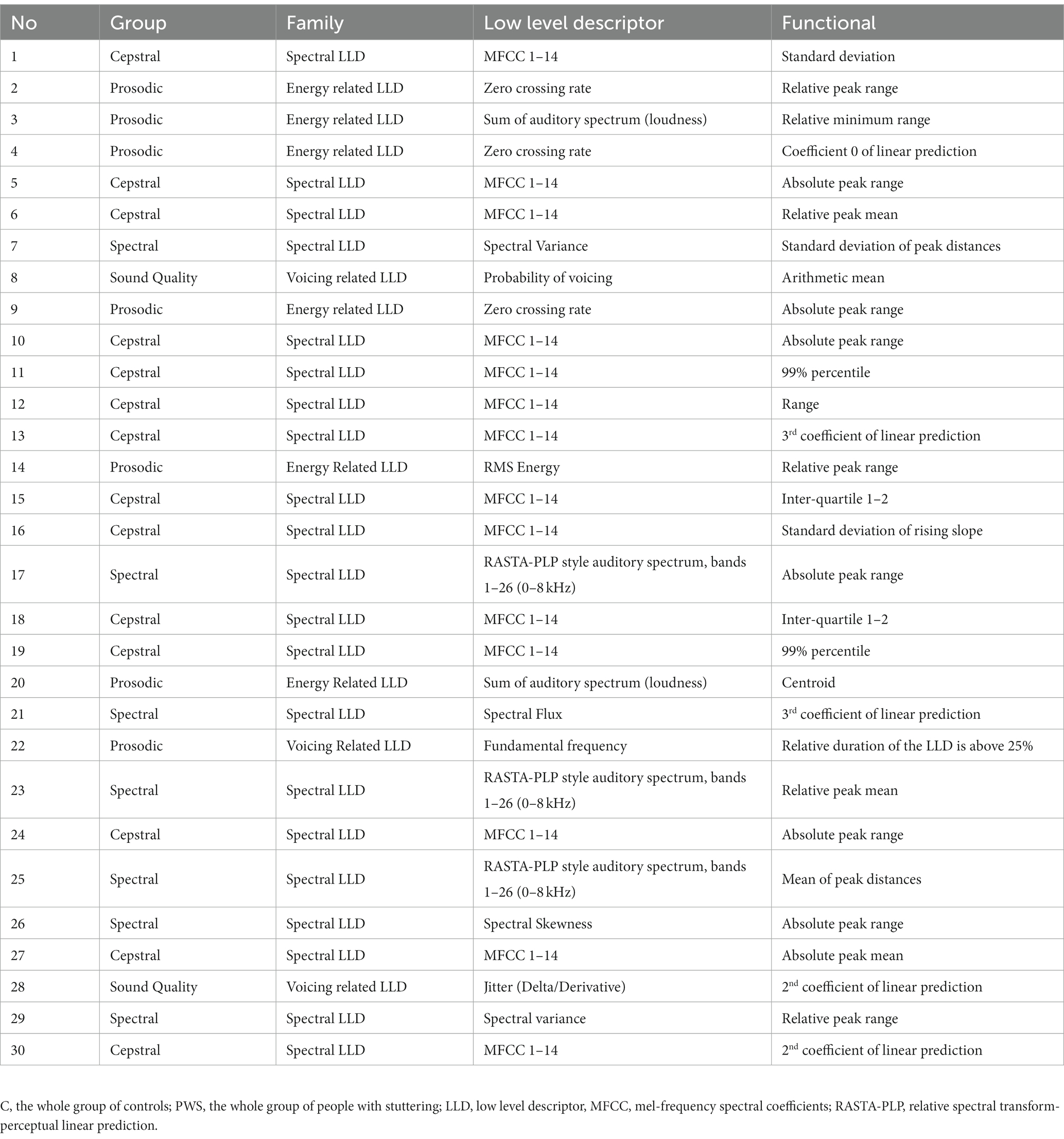
Table 2. List of the first 30 selected features for the comparison between C and PWS during the sustained emission of a vowel.
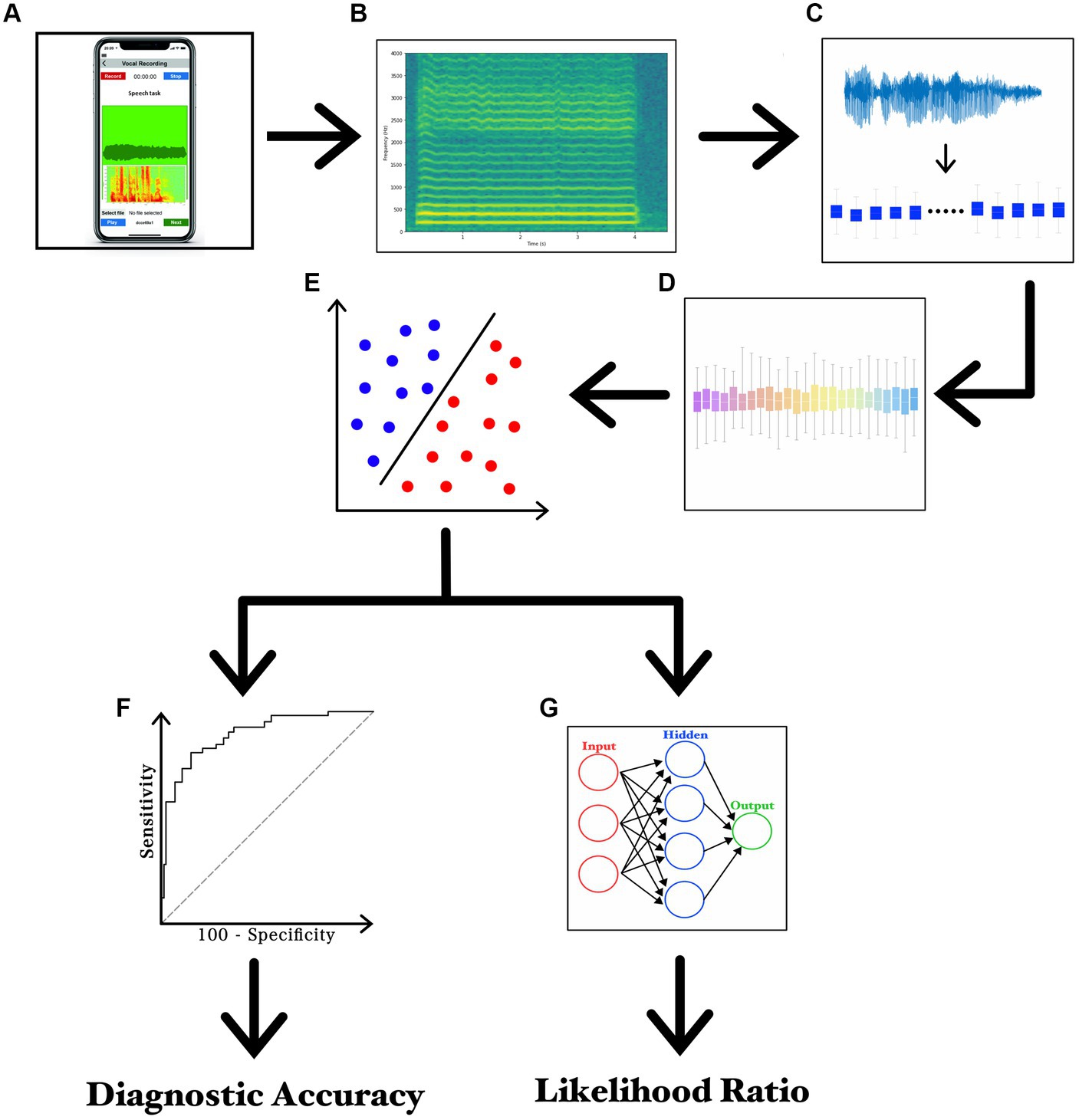
Figure 1. Experimental paradigm. (A) recording of voice samples using a smartphone; (B) narrow-band spectrogram of the acoustic voice signal; (C) feature extraction; (D) feature selection; (E) feature classification; (F) ROC curve analysis; and (G) LR values calculated through ANN.
2.2. Statistical analysis
The normality of the demographic and anthropometric variables in patients and controls was assessed using the Kolmogorov-Smirnov test. Mann–Whitney U test was used to compare demographic scores in patients and controls. ROC analyses were performed to identify the optimal diagnostic cut-off values of the support vector machine (SVM) (selected features), for discriminating between:
(1) controls vs. PWS during the sustained emission of the vowel and sentence s1;
(2) controls vs. PWS during the emission of sentences s1 and s2;
(3) yC vs. yPWS during the sustained emission of the vowel and sentence s1;
(4) cC vs. cPWS during the sustained emission of the vowel and sentence s1;
(5) cC vs. yC during the sustained emission of the vowel and sentence s1;
(6) cPWS vs. yPWS during the sustained emission of the vowel and sentence s1.
Cut-off values were calculated as the point of the curves with the highest Y.I. (sensitivity + specificity −1) to maximize the sensitivity and specificity of the diagnostic tests. The positive and negative predictive values were also calculated. According to standardized procedures (48), we compared the area under the curves (AUCs) in the ROC curves calculated from SMO (selected features) to verify the optimal test for discriminating within the subgroups. All ROC analyses were performed using MATLAB. Spearman’s rank correlation coefficient was used to assess correlations between clinical scores and LR values. p < 0.05 was considered statistically significant. Unless otherwise stated, all values are presented as mean ± standard deviation (SD). Statistical analyses were performed using Statistica version 10 (StatSoft, Inc) and MATLAB (Mathworks, Inc.).
3. Results
The Kolmogorov–Smirnov test showed that demographic (age) and anthropometric (weight and height) parameters were normally distributed in controls and people with stuttering (p < 0.05). Mann-Whitney U test showed comparable demographic, MMSE, FAB, and HAM-D scores in patients and controls (p > 0.05) (Table 1). The clinical assessment of stuttering was based on VHI, SSI-4, and SSS scales. AVHI mean ± SD score of 48.3 ± 22.32 is suggestive of moderate disease severity; an SSI-4 mean ± SD score of 20.5 ± 9.0 is indicative of a mild-to-moderate stuttering severity both in children and adults; An SSS mean ± SD score of 3.2 ± 1.7 is suggestive of mild disease severity (49). The recorded MMSE, FAB, and HAM-D scores in both PWS and controls suggest normal cognition and the absence of mood depression (All p values >0.05; Table 1).
3.1. Machine-learning analysis
When analyzing PWS versus controls during the sustained emission of the vowel, ROC curve analyses identified an optimal diagnostic threshold value of −0.24 (associated criterion) with Y.I. of 0.75. Furthermore, during the sustained emission of the sentence s1, ROC curve analyses identified an optimal diagnostic threshold value of 0.12 with a Y.I. of 0.66. Then, we compared the ROC curves obtained during the emission of the vowel and the sentence s1 showing comparable results (the difference between AUCs = 0.028, z = 0.660, SE = 0.042, and p = 0.51) (Figure 2A, Table 3).
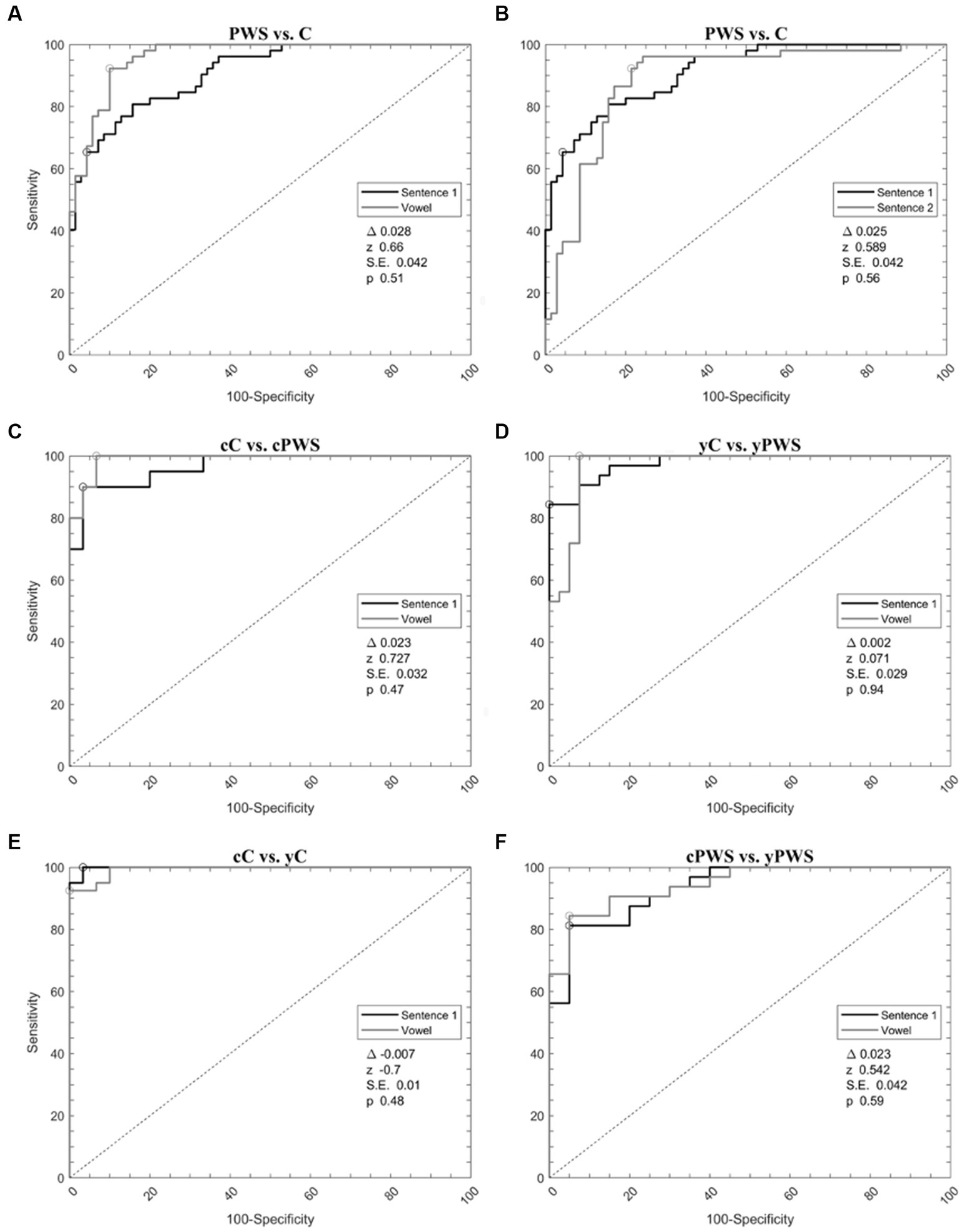
Figure 2. Support vector machine analysis of voice samples. Receiver operating characteristic curves calculated with a support vector machine to differentiate: (1) Controls and people with stuttering during the emission of vowel and sentence 1 (A); (2) Controls and people with stuttering during the emission of sentence s1 and s2 (B); (3) Younger adult controls and people with stuttering during the emission of vowel and sentence 1 (C); (4) Children controls and people with stuttering during the emission of vowel and sentence 1 (D); (5) Children and younger adult controls during the emission of vowel and sentence s1 (E); (6) Children and younger adult people with stuttering during the emission of vowel and sentence s1 (F). C, the whole group of controls; cC, controls in prepubertal age; yC, younger adult controls in postpubertal age; PWS, the whole group of people with stuttering; cPWS, children with stuttering in the prepubertal age; yPWS, younger adults with stuttering in the postpubertal age.
Machine-learning discriminated between PWS and controls during the emission of the sentence s1 and s2. When comparing the 30 most relevant selected features extracted from the emission of s2, ROC curve analyses identified an optimal diagnostic threshold value of −0.09 with Y.I. of 0.62. Then, we compared the ROC curves obtained during the emission of s1 and s2 showing comparable results (the difference between AUCs = 0.025, z = 0.589, SE = 0.042, and p = 0.56) (Figure 2B, Table 3).
Concerning the classification of cC vs. cPWS during the sustained emission of the vowel and sentence s1, for the emission of the vowel, ROC curve analyses identified an optimal diagnostic threshold value of 0.23 with YI of 0.94. Furthermore, during the sustained emission of the sentence s1, ROC curve analyses identified an optimal diagnostic threshold value of 0.06, with YI of 0.85. Then, we compared the ROC curves obtained during the emission of the vowel and sentence s1 showing comparable results (the difference between AUCs = 0.023, z = 0.727, SE = 0.032, and p = 0.47) (Figure 2C, Table 3).
The discrimination between yC vs. yPWS, during the sustained emission of the vowel and sentence s1 also disclosed high accuracy. When comparing the 30 related most relevant selected features extracted from the emission of the vowel, ROC curve analyses identified an optimal diagnostic threshold value of 0.66 (associated criterion), when applying discretization and 10-folds cross-validation (Y.I. = 0.80). Furthermore, when comparing 30 selected features extracted from the sustained emission of the sentence s1, ROC curve analyses identified an optimal diagnostic threshold value of 0.07, when applying discretization and 10-folds cross-validation (Y.I. = 0.81). Afterwards, we compared the ROC curves obtained during the emission of the vowel and sentence s1 showing comparable results (the difference between AUCs = 0.002, z = 0.071, SE = 0.029, and p = 0.94) (Figure 2D, Table 3).
When comparing cC and yC with sequential minimal optimization (SMO), we were able to obtain high results for both the emission of the vowel and sentence s1. For the emission of the vowel, ROC curve analyses identified an optimal diagnostic threshold value of 0.39 with Y.I. of 0.88. Furthermore, when comparing 30 selected features extracted from the sustained emission of the sentence s1, ROC curve analyses identified an optimal diagnostic threshold value of 0.43, when applying discretization and 10-folds cross-validation (Y.I. = 0.94). Then, we compared the ROC curves obtained during the emission of the vowel and sentence s1 showing comparable results (the difference between AUCs = −0.007, z = −0.700, SE = 0.010, p = 0.48) (Figure 2E, Table 3).
Finally, we discriminated between cPWS and yPWS with SMO. In this case, we were also able to obtain high results for both the emission of the vowel and sentence s1. For the emission of the vowel, ROC curve analyses identified an optimal diagnostic threshold value of 0.20 (associated criterion) with Y.I. of 0.86. Furthermore, during the sustained emission of the sentence s1, ROC curve analyses identified an optimal diagnostic threshold value of 0.24, with a Y.I. of 0.89. Then, we compared the ROC curves obtained during the emission of the vowel and sentence s1 showing comparable results (the difference between AUCs = 0.023, z = 0.542, SE = 0.042, and p = 0.59) (Figure 2F, Table 3).
3.2. Correlation analysis
In the group of PWS, the Spearman test disclosed a negative correlation between age and HAM-D (r = −0.35, p = 0.012), i.e., young PWS manifest a higher psychological burden of disease. Also, we found highly relevant positive correlations among scores at clinical scales for the multidimensional assessment of stuttering. More in detail, VHI significantly correlated with SSS (r = 0.95, p < 0.01) and SSI-4 (r = 0.97, p < 0.01), i.e., the higher impairment of stuttering, the greater the stuttering-related complaint. Lastly, SSS positively correlated with SSI-4 (r = 0.94, p < 0.01).
Concerning the clinical-instrumental correlations, we found a positive correlation between LRs collected in the overall group of PWS during the sustained emission of vowel/e/ as well as during the reading of sentences S1 and S2 and SSS (r = 0.31, p = 0.02, for the vowel; r = 0.30, p = 0.03, for S1; r = 0.44, p < 0.01, for S2), SSI-4 (r = 0.32, p = 0.02, for the vowel; r = 0.36, p < 0.01, for S1; r = 0.46, p < 0.01, for S2) and VHI (r = 0.32, p = 0.02, for the vowel; r = 0.35, p = 0.01, for S1; r = 0.43, p < 0.01, for S2). The higher the LR values attributed by machine-learning, the higher disability and severity of overall stuttering and voice symptoms. When considering subgroups of cPWS as well as yPWS, we reported a higher positive correlation between LRs calculated during the emission of speech tasks and scores at clinical scales (i.e., SSS, SSI-4, and VHI). More in detail, in cPWS and yPWS, LRs positively correlated with SSS during the emission of the vowel (r = 0.67, p < 0.01, and r = 0.43, p = 0.02, respectively), S1 (r = 0.53, p = 0.02, and r = 0.54, p < 0.01, respectively) and S2 (r = 0.67, p < 0.01, and r = 0.42, p = 0.02, respectively). Still, in cPWS and yPWS, LRs positively correlated with SSI-4 during the emission of the vowel (r = 0.63, p < 0.01, and r = 0.54, p < 0.01, respectively), S1 (r = 0.56, p = 0.02, and r = 0.51, p < 0.01, respectively) and S2 (r = 0.62, p < 0.01, and r = 0.42, p = 0.02, respectively). Lastly, in cPWS, LRs also positively correlated with VHI during the emission of the vowel (r = 0.64, p < 0.01, and r = 0.51, p < 0.01, respectively), S1 (r = 0.57, p = 0.02, and r = 0.50, p < 0.01, respectively) and S2 (r = 0.61, p < 0.01, and r = 0.41, p = 0.02, respectively).
4. Discussion
In this study, we objectively recognized people with stuttering by using artificial intelligence (i.e., the SVM algorithm). We identified relevant acoustic features altered in stuttering, achieving high classification accuracy in discriminating between PWS and controls. We also found a significant effect of ageing in modifying abnormal acoustic features reported in stuttering, as shown by high classification accuracy when discriminating between independent ageing groups, among PWS and controls. Our analysis was highly consistent and reliable as suggested by the lack of linguistic-related detrimental effects exerted by speech tasks on stuttering. Lastly, we found significant clinical-instrumental correlations pointing to the great medical relevance of our analysis. Overall, our findings support the role of machine learning in the objective recognition of specific voice disorders (50–52).
To collect homogeneous audio recordings in PWS and controls, we carefully controlled for several methodological factors. Participants were all native Italian speakers, non-smokers, and did not report pathologic conditions affecting voice emission, thus allowing for the exclusion of possible confounding factors. All young adults (e.g., both people with stuttering and controls) completed the pubertal development, thus excluding incomplete development of systems involved in voice emission. Patients with stuttering had similar demographic and anthropometric characteristics (e.g., height, weight, and BMI) as compared with controls, thus excluding confounding related to these physiologic factors. Subjects with cognitive impairment and mood disorders were excluded from the study cohort. Furthermore, since the worsening of dysfluencies during the day, we asked participants to record audio samples in the morning, when possible. The stuttering diagnosis was based on current international guidelines. As speech tasks, we selected the sustained emission of a vowel and sentences according to standardized procedures, respectively. All samples were recorded through smartphones currently available on the market and able to save audio tracks in the required file format. Corrupted recordings were excluded from the analysis.
4.1. Clinical evaluation In people with stuttering
Clinically, both cPWS and yPWS showed mild-to-moderate disease severity in terms of stuttering, and absent depression, thus confirming developmental stuttering. The mild-to-moderate stuttering severity measured in our sample of PWS is in line with the literature findings of a full recovery during the first four years of age reported in up to 75% of pre-schoolers with developmental stuttering (2, 53), thus showing a milder form of the disease once grown-up. We found that young PWS manifest a trend towards a higher psychological burden of disease, though without reaching statistical significance. Also, we found highly relevant positive correlations among scores at clinical scales for the multidimensional assessment of stuttering (VHI, SSS, and SSI-4), independently from the specific age groups.
4.2. Machine-learning analysis in people with stuttering
In this study, we objectively recognized PWS by acoustic analysis based on SVM. This finding is highlighted by the high reliability and accuracy of results achieved during the emission of speech tasks when classifying controls and PWS. As shown by the most relevant acoustic features selected by machine-learning, our methodology fits with previous studies based on spectral analysis, thus confirming the biological plausibility of our observations. It should be mentioned that all previous studies aiming at the objective analysis of stuttering were based on standardized spectral analysis and were able to find multiple abnormal acoustic features in stuttering (13–26). These investigations have certainly contributed to improve current knowledge of stuttering by reporting specific changes in acoustic features (13–26). However, standard acoustic analysis does not allow for dynamically combining selected features extracted from a large dataset, and it does not offer the opportunity to automatically learn and improve from experience (29, 32, 33). Therefore, other authors have applied automatic tools to detect speech impairment in the field of stuttering, for classification purposes. Historically, the first attempt to automatically and objectively detect stuttering through machine-learning comes from the automatic speech recognition (ASR) analysis, consisting of a preliminary process that converts audio signals to text, and then a second phase used to detect and identify linguistic abnormalities related to stuttering (54–56). The main limitation of this approach was the great number of errors. Therefore, authors have begun to apply machine-learning to acoustic features extracted from audio recordings in patients with stuttering, using several approaches including but not limited to the Mel frequency cepstral coefficients (MFCC), the ANN, the hidden Markov model (HMM), and finally the SVM (57–62). Despite the achievements in the objective analysis of stuttering, previous research in the field was characterized by several limitations. Machine-learning studies in stuttering have included small and heterogeneous cohorts of audio samples collected in a dataset of patients with stuttering. Also, the large part of datasets of audio recordings from stutterers lacks relevant clinical as well as anthropometric parameters which are known to be involved in voice emission. Also, it has been documented that PWS show difficulties in coordinating airflow, articulation, and resonance, and minor asynchronies have been found even during fluent speech (63). Hence, we believe that our study demonstrates the ability of acoustic analysis to objectively recognize PWS. The acoustic features selected by our classifier and used for further classification purposes correspond to those considered in previous seminal works in the field, using spectral and cepstral analysis (see also Table 2) (57–62). Moreover, our study showed for the first-time significant clinical-instrumental correlations: the higher the LR values attributed by machine-learning, the higher severity of symptoms in PWS. Hence, we demonstrated that the degree of voice changes in PWS correlates with disease severity, and finally, LR values can be considered reliable scores to express the severity of PWS.
Our machine-learning approach would further help in clarifying the pathophysiological underpinning of stuttering. Recent clinical and experimental observations have raised the hypothesis that stuttering reflects impaired sensorimotor integration in specific brain networks (64, 65), to the point that it may be even considered as a form of focal/segmental action dystonia (66, 67). Hence, we conjecture that future experimental investigations combining machine-learning analysis of voice with neurophysiological and neuroimaging techniques would help in better assessing voice-related changes in sensorimotor integration in PWS (34).
4.3. Effect of ageing in people with stuttering
Concerning the effect of ageing on acoustic features in stuttering, this is the first study to demonstrate a significant role of ageing in changing acoustic features known to be altered in stuttering as shown by previously unreported high accuracy in classifying children (i.e., 7–12 years) and younger adults (i.e., 15–30 years) among PWS and controls. The observation that machine-learning can achieve high classification accuracy when discriminating between cC and yC allows us to confirm and expand data reported in a previous publication by our groups on healthy controls in which we showed that objective acoustic analysis distinguished between younger and older adults, with a high level of accuracy (29). In this study, we demonstrated a similar effect of human ageing on acoustic features in PWS, as suggested by the high accuracy when classifying cPWS and yPWS. As previously demonstrated for healthy controls, similar age-related changes in physiological functions may explain our findings in PWS. More in detail, the physiological basis underlying our results is prominently linked to age-related changes in the phonatory apparatus, including the effects of hormone molecules during the transition from pre-pubertal to post-pubertal age. Still, we found significant clinical-instrumental correlations also in cPWS and yPWS: the greater the LR values, the higher severity of PWS. Interestingly, when classifying cC and cPWS as well as yC and yPWS we obtained higher results than those achieved during the comparison between PWS and the overall group of controls. These results confirm previous data reported by our group showing that the accuracy of the machine-learning algorithm tended to further improve when comparing the groups of subjects with a narrower age band (29).
4.4. Effect of speech task in people with stuttering
We observed similar classification accuracy for each speech task considered in the analysis, namely the sustained emission of the vowel and sentences. This issue could be possibly judged controversial since it is known that several types of dysfluencies in PWS, including involuntary, audible, or silent, repetitions or prolongations of sounds, syllables, words, sentences, dysrhythmic phonation, such as blocks, and prolongations tend to worsen in response to specific speech task (i.e., the detrimental effect of linguistic issues). However, as already reported for other disorders affecting voice, including laryngeal dystonia and Parkinson’s disease, we speculate that machine learning is able to detect subtle changes in acoustic features occurring even during a simple voice task (i.e., the sustained emission of a vowel). This finding is of great relevance since it testifies the transveral applicability of machine learning analysis among different languages. With the present study we showed the wide applicability of our analysis for telemedicine purposes, as shown by the results achieved using smartphone-recorded audio samples, in an ecologic scenario. The importance of the application of telemedicine to research on stuttering has been previously suggested, although with some technical limitations (68), which have been resolved in the present study. It is well-known that telemedicine and telepractice can be used as complementary therapeutic methods for neurologic diseases in general (69), and for stuttering in particular (68), hence we are confident that our study will lay the foundation for future therapeutic efforts in this field.
5. Conclusion
We achieved high classification accuracy when discriminating between PWS and controls. We also demonstrated a significant effect of ageing, as shown by high accuracy when discriminating between children and younger controls as well as PWS. Furthermore, we showed that our analysis was highly consistent and reliable as suggested by the lack of linguistic-related detrimental effects on stuttering. In addition, we demonstrated the applicability of our analysis for telemedicine purposes. Lastly, we found significant clinical-instrumental correlations pointing to machine-learning analysis as a consistent and reliable tool to objectively diagnose stuttering. To date, therapeutic strategies for stuttering mostly rely on non-pharmacological approaches based on speech therapy or on stuttering devices, which alter the voice frequency or slow the rate of speech using auditory feedback (53). Within this clinical and therapeutic context, we believe that acoustic analysis could be highly useful for checking and monitoring PWS over time (e.g., before and after a given therapeutic approach). This aspect is valuable and innovative, due to the significant lack of studies on the objective assessment of treatment outcomes in PWS. Ultimately, we are confident that future studies using machine-learning techniques and automated acoustic analysis, may further help clinicians in the classification and management of stuttering as well as other neurodevelopmental disorders of speech production (53).
Data availability statement
The original contributions presented in the study are included in the article/Supplementary material, further inquiries can be directed to the corresponding author.
Ethics statement
The studies involving human participants were reviewed and approved by Institutional Review Board (0026508/2019). Written informed consent to participate in this study was provided by the participants’ legal guardian/next of kin.
Author contributions
FA and LM: organization, execution of the study design, writing of the first draft, review, and critique. AS and GS: conception, organization of the study design, review, and critique. EM, PDL, and MP, execution of the study design, writing of the first draft, review, and critique. LL, GR, FDG, and DT: review and critique. GC: conception, organization of the study design, review, and critique. All authors contributed to the article and approved the submitted version.
Conflict of interest
LM has received honoraria from the International Association of Parkinsonism and Related Disorders (IAPRD) Society for social media and web support and a grant contribution from the International Parkinson Disease Movement Disorders Society for the Unified Tremor Rating Scale Validation Program.
The remaining authors declare that the research was conducted in the absence of any commercial or financial relationships that could be construed as a potential conflict of interest.
The reviewer FM declared a past collaboration with the authors LM at the time of the review.
Publisher’s note
All claims expressed in this article are solely those of the authors and do not necessarily represent those of their affiliated organizations, or those of the publisher, the editors and the reviewers. Any product that may be evaluated in this article, or claim that may be made by its manufacturer, is not guaranteed or endorsed by the publisher.
Supplementary material
The Supplementary material for this article can be found online at: https://www.frontiersin.org/articles/10.3389/fneur.2023.1169707/full#supplementary-material
References
1. Büchel, C, and Sommer, M. What causes stuttering? PLoS Biol. (2004) 2:E46. doi: 10.1371/journal.pbio.0020046
2. Yairi, E. Defining stuttering for research purposes. J Fluen Disord. (2013) 38:294–8. doi: 10.1016/j.jfludis.2013.05.001
3. Karniol, R. Stuttering, language, and cognition: a review and a model of stuttering as suprasegmental sentence plan alignment (SPA). Psychol Bull. (1995) 117:104–24. doi: 10.1037/0033-2909.117.1.104
4. Ambrose, NG, Yairi, E, Loucks, TM, Seery, CH, and Throneburg, R. Relation of motor, linguistic and temperament factors in epidemiologic subtypes of persistent and recovered stuttering: initial findings. J Fluen Disord. (2015) 45:12–26. doi: 10.1016/j.jfludis.2015.05.004
5. Martin, RR, and Haroldson, SK. Stuttering identification: standard definition and moment of stuttering. J Speech Hear Res. (1981) 24:59–63. doi: 10.1044/jshr.2401.59
6. Wingate, ME. A standard definition of stuttering. J Speech Hear Disord. (1964) 29:484–9. doi: 10.1044/jshd.2904.484
7. O’Brian, S, Jones, M, Packman, A, Onslow, M, Menzies, R, Lowe, R, et al. The complexity of stuttering behavior in adults and adolescents: relationship to age, severity, mental health, impact of stuttering, and behavioral treatment outcome. J Speech Lang Hear Res. (2022) 65:2446–58. doi: 10.1044/2022_JSLHR-21-00452
8. Smith, A, and Weber, C. How stuttering develops: the multifactorial dynamic pathways theory. J Speech Lang Hear Res. (2017) 60:2483–505. doi: 10.1044/2017_JSLHR-S-16-0343
9. Yairi, E, and Ambrose, N. Epidemiology of stuttering: 21st century advances. J Fluen Disord. (2013) 38:66–87. doi: 10.1016/j.jfludis.2012.11.002
10. Sokolowski, HM, and Levine, B. Common neural substrates of diverse neurodevelopmental disorders. Brain. (2022) 146:438–47. doi: 10.1093/brain/awac387
11. Schindler, A, Ottaviani, F, Mozzanica, F, Bachmann, C, Favero, E, Schettino, I, et al. Cross-cultural adaptation and validation of the voice handicap index into Italian. J Voice. (2010) 24:708–14. doi: 10.1016/j.jvoice.2009.05.006
12. Yaruss, JS, and Quesal, RW. Overall assessment of the Speaker’s experience of stuttering (OASES): documenting multiple outcomes in stuttering treatment. J Fluen Disord. (2006) 31:90–115. doi: 10.1016/j.jfludis.2006.02.002
13. Adams, MR, and Ramig, P. Vocal characteristics of normal speakers and stutterers during choral reading. J Speech Hear Res. (1980) 23:457–69. doi: 10.1044/jshr.2302.457
14. Andrews, G, Howie, PM, Dozsa, M, and Guitar, BE. Stuttering: speech pattern characteristics under fluency-inducing conditions. J Speech Hear Res. (1982) 25:208–16. doi: 10.1044/jshr.2502.208
15. Bakhtiar, M, Zhang, C, and Sze, KS. Impaired processing speed in categorical perception: speech perception of children who stutter. PLoS One. (2019) 14:e0216124. doi: 10.1371/journal.pone.0216124
16. Bakker, K, and Brutten, GJ. Speech-related reaction times of stutterers and nonstutterers: diagnostic implications. J Speech Hear Disord. (1990) 55:295–9. doi: 10.1044/jshd.5502.295
17. Brejon Teitler, N, Ferré, S, and Dailly, C. Specific subtype of fluency disorder affecting French speaking children: A phonological analysis. J Fluen Disord. (2016) 50:33–43. doi: 10.1016/j.jfludis.2016.09.002
18. Dayalu, VN, Guntupalli, VK, Kalinowski, J, Stuart, A, Saltuklaroglu, T, and Rastatter, MP. Effect of continuous speech and non-speech signals on stuttering frequency in adults who stutter. Logoped Phoniatr Vocol. (2011) 36:121–7. doi: 10.3109/14015439.2011.562535
19. Healey, EC, and Gutkin, B. Analysis of stutterers’ voice onset times and fundamental frequency contours during fluency. J Speech Hear Res. (1984) 27:219–25. doi: 10.1044/jshr.2702.219
20. Maruthy, S, Feng, Y, and Max, L. Spectral coefficient analyses of word-initial stop consonant productions suggest similar anticipatory Coarticulation for stuttering and nonstuttering adults. Lang Speech. (2018) 61:31–42. doi: 10.1177/0023830917695853
21. Max, L, and Gracco, VL. Coordination of oral and laryngeal movements in the perceptually fluent speech of adults who stutter. J Speech Lang Hear Res. (2005) 48:524–42. doi: 10.1044/1092-4388(2005/036)
22. McFarlane, SC, and Shipley, KG. Latency of vocalization onset for stutterers and nonstutterers under conditions of auditory and visual cueing. J Speech Hear Disord. (1981) 46:307–12. doi: 10.1044/jshd.4603.307
23. McLean-Muse, A, Larson, CR, and Gregory, HH. Stutterers’ and nonstutterers’ voice fundamental frequency changes in response to auditory stimuli. J Speech Hear Res. (1988) 31:549–55. doi: 10.1044/jshr.3104.549
24. Metz, DE, Samar, VJ, and Sacco, PR. Acoustic analysis of stutterers’ fluent speech before and after therapy. J Speech Hear Res. (1983) 26:531–6. doi: 10.1044/jshr.2604.531
25. Peters, HF, Hulstijn, W, and Starkweather, CW. Acoustic and physiological reaction times of stutterers and nonstutterers. J Speech Hear Res. (1989) 32:668–80. doi: 10.1044/jshr.3203.668
26. Prosek, RA, and Runyan, CM. Temporal characteristics related to the discrimination of stutterers’ and nonstutterers’ speech samples. J Speech Hear Res. (1982) 25:29–33. doi: 10.1044/jshr.2501.29
27. Saggio, G, and Costantini, G. Worldwide healthy adult voice baseline parameters: A comprehensive review. J Voice. (2022) 36:637–49. doi: 10.1016/j.jvoice.2020.08.028
28. Asci, F, Costantini, G, Saggio, G, and Suppa, A. Fostering voice objective analysis in patients with movement disorders. Mov Disord. (2021) 36:1041. doi: 10.1002/mds.28537
29. Asci, F, Costantini, G, Di Leo, P, Zampogna, A, Ruoppolo, G, Berardelli, A, et al. Machine-learning analysis of voice samples recorded through smartphones: the combined effect of ageing and gender. Sensors (Basel). (2020) 20:5022. doi: 10.3390/s20185022
30. Hlavnička, J, Tykalová, T, Ulmanová, O, Dušek, P, Horáková, D, Růžička, E, et al. Characterizing vocal tremor in progressive neurological diseases via automated acoustic analyses. Clin Neurophysiol. (2020) 131:1155–65. doi: 10.1016/j.clinph.2020.02.005
31. Rusz, J, Tykalova, T, Ramig, LO, and Tripoliti, E. Guidelines for speech recording and acoustic analyses in Dysarthrias of movement disorders. Mov Disord. (2021) 36:803–14. doi: 10.1002/mds.28465
32. Suppa, A, Asci, F, Saggio, G, Marsili, L, Casali, D, Zarezadeh, Z, et al. Voice analysis in adductor spasmodic dysphonia: objective diagnosis and response to botulinum toxin. Parkinsonism Relat Disord. (2020) 73:23–30. doi: 10.1016/j.parkreldis.2020.03.012
33. Suppa, A, Asci, F, Saggio, G, Di Leo, P, Zarezadeh, Z, Ferrazzano, G, et al. Voice analysis with machine learning: one step closer to an objective diagnosis of essential tremor. Mov Disord. (2021) 36:1401–10. doi: 10.1002/mds.28508
34. Suppa, A, Marsili, L, Giovannelli, F, Di Stasio, F, Rocchi, L, Upadhyay, N, et al. Abnormal motor cortex excitability during linguistic tasks in adductor-type spasmodic dysphonia. Eur J Neurosci. (2015) 42:2051–60. doi: 10.1111/ejn.12977
35. Pruett, DG, Shaw, DM, Chen, HH, Petty, LE, Polikowsky, HG, Kraft, SJ, et al. Identifying developmental stuttering and associated comorbidities in electronic health records and creating a phenome risk classifier. J Fluen Disord. (2021) 68:105847. doi: 10.1016/j.jfludis.2021.105847
36. Qiao, J, Wang, Z, Zhao, G, Huo, Y, Herder, CL, Sikora, CO, et al. Functional neural circuits that underlie developmental stuttering. PLoS One. (2017) 12:e0179255. doi: 10.1371/journal.pone.0179255
37. Shakeel, A, Sheikh, MS, Hirsch, F, and Ouni, S. Machine learning for stuttering identification: review, challenges and future directions. Neurocomputing. (2022) 514:385–402. doi: 10.1016/j.neucom.2022.10.015
38. Mailend, ML, Maas, E, Beeson, PM, Story, BH, and Forster, KI. Examining speech motor planning difficulties in apraxia of speech and aphasia via the sequential production of phonetically similar words. Cogn Neuropsychol. (2021) 38:72–87. doi: 10.1080/02643294.2020.1847059
39. Jacobson, BH, Johnson, A, Grywalski, C, Silbergleit, A, Jacobson, G, Benninger, MS, et al. The voice handicap index (VHI). Am J Speech Lang Pathol. (1997) 6:66–70. doi: 10.1044/1058-0360.0603.66
40. Folstein, MF, Folstein, SE, and McHugh, PR. “Mini-mental state”. A practical method for grading the cognitive state of patients for the clinician. J Psychiatr Res. (1975) 12:189–98. doi: 10.1016/0022-3956(75)90026-6
41. Dubois, B, Slachevsky, A, Litvan, I, and Pillon, B. The FAB: a frontal assessment battery at bedside. Neurology. (2000) 55:1621–6. doi: 10.1212/WNL.55.11.1621
42. Hamilton, M. A rating scale for depression. J Neurol Neurosurg Psychiatry. (1960) 23:56–62. doi: 10.1136/jnnp.23.1.56
43. Suppa, A, Costantini, G, Asci, F, Di Leo, P, Al-Wardat, MS, Di Lazzaro, G, et al. Voice in Parkinson’s disease: a machine learning study. Front Neurol. (2022) 13:831428. doi: 10.3389/fneur.2022.831428
44. E, A In: M Press, editor. Introduction to machine learning. Cambridge, Mass: Alpaydın E. (2010).
45. Eyben, F WM, and Schuller, B. (2010). Opensmile: the Munich versatile and fast open-source audio feature extractor. In: Press A, editorl. Proceedings of the international conference on multimedia. Firenze, Italy. p. 1459.
46. Russell, SJ, Norvig, P, and Davis, E. Artificial intelligence: a modern approach. 3rd ed. Upper Saddle River, NJ, Prentice Hall. (2010).
47. Specht, DF. A general regression neural network. IEEE Trans Neural Netw. (1991) 2:568–76. doi: 10.1109/72.97934
48. DeLong, ER, DeLong, DM, and Clarke-Pearson, DL. Comparing the areas under two or more correlated receiver operating characteristic curves: a nonparametric approach. Biometrics. (1988) 44:837–45. doi: 10.2307/2531595
50. Cesarini, V, Casiddu, N, Porfirione, C, Massazza, G, Saggio, G, and Costantini, G. (2021). A machine learning-based voice analysis for the detection of dysphagia biomarkers. MetroInd4 0&IoT. 407–411.
51. Costantini, G, Dr, VC, Robotti, C, Benazzo, M, Pietrantonio, F, Di Girolamo, S, et al. Deep learning and machine learning-based voice analysis for the detection of COVID-19: A proposal and comparison of architectures. Knowl Based Syst. (2022) 253:109539. doi: 10.1016/j.knosys.2022.109539
52. Amato, F, Fasani, M., Raffaelli, G., Cesarini, V., Olmo, G., Di Lorenzo, N., and Saggio, G. (2022). Obesity and gastro-esophageal reflux voice disorders: a machine learning approach. IEEE international symposium on medical measurements and applications (MeMeA) Messina, Italy. p. 1–6.
54. Alharbi, S, Hasan, M., Simons, A. J. H., Simons, A., Brumfitt, B., and Green, P. (2018). A lightly supervised approach to detect stuttering in children’s speech. Proceedings of Interspeech. Hyderabad, India. ISCA; p. 3433–7.
55. Alharbi, S HM, Simons, AJ, Brumfitt, S, and Green, P. (2017). Detecting stuttering events in transcripts of children’s speech. New York: Springer. 217–228.
56. LR, Heeman PA, Mc Millin, A, and Yaruss, JS. (2016). Using clinician annotations to improve automatic speech recognition of stuttered speech. Proceedings of the Annual Conference of the International Speech Communication Association, Interspeech. 2651–5.
57. Ravikumar, K, Rajagopal, R, and Nagaraj, H. An approach for objective assessment of stuttered speech using MFCC. DSP J. (2009) 9:19.
58. Howell, P, Sackin, S, and Glenn, K. Development of a two-stage procedure for the automatic recognition of dysfluencies in the speech of children who stutter: II. ANN recognition of repetitions and prolongations with supplied word segment markers. J Speech Lang Hear Res. (1997) 40:1085–96. doi: 10.1044/jslhr.4005.1085
59. Nöth, E, Niemann, H, Haderlein, T, Decher, M, Eysholdt, U, Rosanowski, F., et al. Automatic stuttering recognition using hidden Markov models. Proc. 6th International Conference on Spoken Language Processing (ICSLP 2000). (2000). 4:65–68. doi: 10.21437/ICSLP.2000-752
60. Geetha, YVPK, Ashok, R, and Ravindra, SK. Classification of childhood disfluencies using neural networks. J Fluen Disord. (2000) 25:99–117. doi: 10.1016/S0094-730X(99)00029-7
61. Tan T-S, H-L, Ariff, AK, Ting, C-M, and Salleh, S-H. (2007). Application of Malay speech technology in Malay speech therapy assistance tools. 2007 International Conference on Intelligent and Advanced Systems; Kuala Lumpur.
62. Ravikumar, KRB, Rajagopal, R, and Nagaraj, H. Automatic detection of syllable repetition in read speech for objective assessment of stuttered disfluencies. Proc World Acad Sci Eng Technol. (2008) 36:270–3.
63. Van Riper, CEL. Speech correction: an introduction to speech pathology and audiology. 7th ed. Prentice-Hall, Englewood Cliffs: Prentice-Hall (1984). 284 p.
64. Slis, A, Savariaux, C, Perrier, P, and Garnier, M. Rhythmic tapping difficulties in adults who stutter: A deficit in beat perception, motor execution, or sensorimotor integration? PLoS One. (2023) 18:e0276691. doi: 10.1371/journal.pone.0276691
65. Abu-Zhaya, R, Goffman, L, Brosseau-Lapré, F, Roepke, E, and Seidl, A. The effect of somatosensory input on word recognition in typical children and those with speech sound disorder. J Speech Lang Hear Res. (2023) 66:84–97. doi: 10.1044/2022_JSLHR-22-00226
66. Kiziltan, G, and Akalin, MA. Stuttering may be a type of action dystonia. Mov Disord. (1996) 11:278–82. doi: 10.1002/mds.870110311
67. Alm, PA. Stuttering and the basal ganglia circuits: a critical review of possible relations. J Commun Disord. (2004) 37:325–69. doi: 10.1016/j.jcomdis.2004.03.001
68. Bayati, B, and Ayatollahi, H. Comprehensive review of factors influencing the use of Telepractice in stuttering treatment. Healthc Inform Res. (2021) 27:57–66. doi: 10.4258/hir.2021.27.1.57
Keywords: stuttering, machine-learning, telemedicine, home environment, acoustic analysis
Citation: Asci F, Marsili L, Suppa A, Saggio G, Michetti E, Di Leo P, Patera M, Longo L, Ruoppolo G, Del Gado F, Tomaiuoli D and Costantini G (2023) Acoustic analysis in stuttering: a machine-learning study. Front. Neurol. 14:1169707. doi: 10.3389/fneur.2023.1169707
Edited by:
Katerina Markopoulou, NorthShore University HealthSystem, United StatesReviewed by:
Carlo Alberto Artusi, University of Turin, ItalyMammarella Fulvio, San Camillo-Forlanini Hospital, Italy
Copyright © 2023 Asci, Marsili, Suppa, Saggio, Michetti, Di Leo, Patera, Longo, Ruoppolo, Del Gado, Tomaiuoli and Costantini. This is an open-access article distributed under the terms of the Creative Commons Attribution License (CC BY). The use, distribution or reproduction in other forums is permitted, provided the original author(s) and the copyright owner(s) are credited and that the original publication in this journal is cited, in accordance with accepted academic practice. No use, distribution or reproduction is permitted which does not comply with these terms.
*Correspondence: Giovanni Costantini, Y29zdGFudGluaUB1bmlyb21hMi5pdA==
†These authors have contributed equally to this work