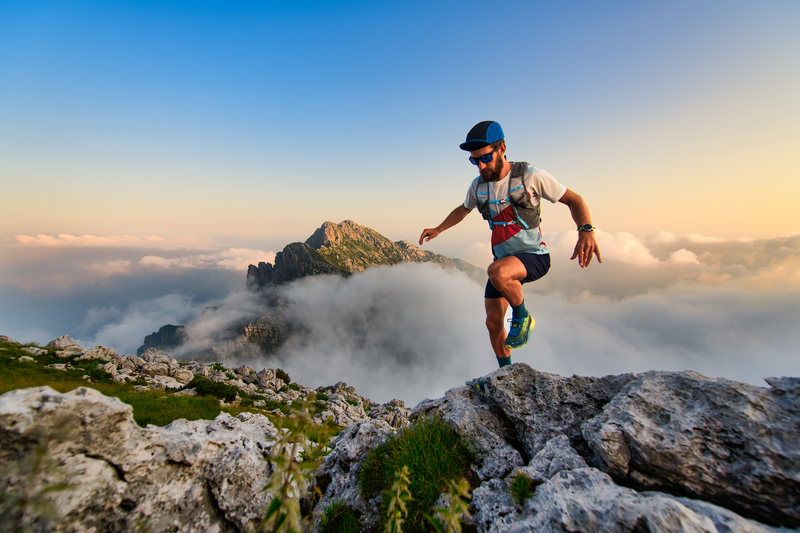
94% of researchers rate our articles as excellent or good
Learn more about the work of our research integrity team to safeguard the quality of each article we publish.
Find out more
ORIGINAL RESEARCH article
Front. Neurol. , 13 March 2023
Sec. Epilepsy
Volume 14 - 2023 | https://doi.org/10.3389/fneur.2023.1147576
This article is part of the Research Topic The Mechanism of Sudden Unexpected Death in Epilepsy and the Specific Forensic Diagnostic Indicators in Sudden Death with a Negative Autopsy View all 10 articles
Introduction: Previous case-control studies of sudden unexpected death in epilepsy (SUDEP) patients failed to identify ECG features (peri-ictal heart rate, heart rate variability, corrected QT interval, postictal heart rate recovery, and cardiac rhythm) predictive of SUDEP risk. This implied a need to derive novel metrics to assess SUDEP risk from ECG.
Methods: We applied Single Spectrum Analysis and Independent Component Analysis (SSA-ICA) to remove artifact from ECG recordings. Then cross-frequency phase-phase coupling (PPC) was applied to a 20-s mid-seizure window and a contour of −3 dB coupling strength was determined. The contour centroid polar coordinates, amplitude (alpha) and angle (theta), were calculated. Association of alpha and theta with SUDEP was assessed and a logistic classifier for alpha was constructed.
Results: Alpha was higher in SUDEP patients, compared to non-SUDEP patients (p < 0.001). Theta showed no significant difference between patient populations. The receiver operating characteristic (ROC) of a logistic classifier for alpha resulted in an area under the ROC curve (AUC) of 94% and correctly classified two test SUDEP patients.
Discussion: This study develops a novel metric alpha, which highlights non-linear interactions between two rhythms in the ECG, and is predictive of SUDEP risk.
SUDEP is the sudden death of a person with epilepsy without known cause, which typically occurs after a convulsive seizure in sleep and accounts for 1 in 5 cases of epilepsy-related mortality (1–3). SUDEP often follows a generalized tonic-clonic seizure (GTCS) and results from brainstem dysfunction that impairs arousal, respiration and cardiac processes, where brainstem dysfunction may be related to suppression of activity due to spreading depolarization or other mechanisms (4–8).
Risk factors for SUDEP include increased frequency or recent history of seizure, especially tonic-clonic seizures, sub-therapeutic anti-seizure medication (ASM) levels, and lack of supervision during sleep (4). A third of epilepsy patients are not fully controlled by ASMs and many suffer ASM-related adverse effects (9). A biomarker for SUDEP could alert clinicians to recommend nocturnal monitoring or alternative treatments such as neuromodulation therapy or surgical resection (10).
Analysis of electrocardiogram (ECG) recordings may provide a biomarker of SUDEP [e.g., ventricular conduction abnormalities (11) or decreased heart rate variability (12)] but is limited by muscle-induced artifact among other issues (11–13). Single Spectrum Analysis and Independent Component Analysis (SSA-ICA) have been shown to remove artifacts from electroencephalogram (EEG) signals with simulated artifacts (14). Cross-frequency Phase-Phase Coupling (PPC) reduces sensitivity to high-amplitude noise and has been shown to be physiologically relevant for analysis of neural signals (15).
The objective of our study was to use SSA-ICA to remove artifacts from ictal ECG recordings, and assess PPC features to predict SUDEP risk. The metrics alpha and theta were derived from the PPC of a 20 s mid-seizure window.
Our series included 9 definite-SUDEP (16) patients (sudden, unexpected death of a patient without relevant comorbidities, in which postmortem examination, including toxicology, does not reveal a cause of death other than epilepsy) and 12 non-SUDEP patients with drug-resistant focal (temporal or extratemporal lobe) epilepsy, undergoing presurgical evaluation. Patients were not on ASMs at the time of their epilepsy monitoring unit (EMU) EEG recording. The ECG recordings were acquired using the Natus/Xltek EEG system, 2-lead recordings, active electrode placed in left supraclavicular region, reference electrode on left mastoid. Apart from their definite-SUDEP designation, the following data were not available in this retrospective study: simultaneous video EEG, sleep/wakefulness states, other medications, MRI findings, or non-epilepsy medical history.
Two of the definite-SUDEP patients who succumbed to SUDEP more than 8 years after their last simultaneous EEG and ECG recordings were reserved as test cases (patients 20, 21); the remaining SUDEP patients died within 3 years of their last available recordings. Patients categorized as non-SUDEP did not die within 10 years of their last available recordings. Concurrent EEG and ECG recordings were obtained from the patients through the consortium formed by the Toronto Western Hospital, the New York University (NYU) Comprehensive Epilepsy Center, and the Phramongkutklao Royal Army Hospital (Table 1). Ictal (seizure) durations were identified from EEG scalp electrode recordings by board-certified neurologists/electroencephalographers.
The institutional review boards of the consortium approved the study protocol and all patients gave informed consent.
A 4-step process was used (Figure 1): (1) Applying SSA-ICA to the ictal ECG; (2) Using PPC to generate a comodulogram; (3) Analyzing the −3 dB contour of coupling power to extract the centroid in polar coordinates; (4) Training a logistic classifier for risk assessment. Computations were performed on the Niagara supercomputer at the SciNet HPC Consortium using Python 3.9.
Figure 1. (A) Block diagram of ECG data analysis. (B) Sample ictal ECG. (C) Phase-phase comodulogram (PPC) feature extraction: polar coordinates alpha and theta of the contour centroid (red).
Recordings were down sampled to the lowest sample rate in the dataset, 200 Hz. We applied SSA-ICA to remove artifacts, using a 250 samples (1.25 s) window size, decomposed into 15 components. Since a residual DC-level, or drift, in the signal after the application of SSA-ICA presents as low-frequency artifact, we applied a 0.5 Hz high-pass filter to prevent ECG “baseline wander” from appearing as strongly phase-coupled artifacts in our PPC (17).
A comodulogram representing the PPC of frequencies was calculated from the phase values of a continuous wavelet transformation using a morlet wavelet with center frequency of 0.8125 Hz. Phase values were compared by phase locking value (PLV) at each combination of frequencies between 0.1 and 6 Hz, using 0.1 Hz steps, where the PLV is an established measure of phase coherence (18). We used a time-averaged n:m PLV (19) for values of n, m = 1, 2, 3 … 30, where m > n. The comodulogram indicates maximum coupling (1.0) when two frequencies within the same signal are phase-locked with respect to the quotient m/n, and no coupling (0.0) when there is no phase coherence between them. SSA-ICA was applied to the entire recording, and PPC analysis was performed for a 20 s window at the midpoint of the seizure, as identified by the electroencephalographer.
The region of interest (ROI) for further analysis was determined by selecting the lowest-frequency contour formed by the −3 dB threshold of maximum coupling power and determining the half-power point contour using the −3 dB threshold of the local maximum coupling within the region where the centroid (α, θ) of this ROI is the first moment of area in polar coordinates, based on Hall (20).
Binary logistic regression was used to assess SUDEP risk of the seizures. Propensity scores obtained from training set alpha values were used to create a validation receiver operating characteristic (ROC) curve by varying the classification threshold from 0 to 1. An optimal propensity score threshold was selected for accuracy. Patients were then classified as SUDEP vs. non-SUDEP based on the classification of the majority of their seizures.
Alpha was significantly elevated in SUDEP compared to non-SUDEP patients (p < 0.0001), while there were no significant differences for theta (p = 0.6050). Figure 2 shows both the estimation statistics and the per-patient box plots. Estimation statistics were used to plot the overall difference between SUDEP and non-SUDEP populations. The use of estimation statistics decreases overreliance on p-values alone (21), and explicitly shows the effect size, range of results, and number of data points. Reported p-values use the Wilcoxon rank-sum test (22). Box plots show per-patient results, with one column per patient, and one data point seizure. All measures resulted in wide ranges of results on a per-patient basis, attributable to differences between seizures of a given patient. Figure 3 presents results separated by seizure type. The most significant differences are in Figure 3A, the alpha metric for GTCS (p<0.0001). The theta metric was insignificant for both types of seizures.
Figure 2. Estimation statistics of both generalized and non-generalized seizures, comparing mid-seizure 20s windows for SUDEP vs. non-SUDEP populations. Population sizes indicated by Ns (number of seizures), and associated Np (number of patients). Alpha metric (A) and theta metric (B) of the contour centroid. Blue dots represent seizures from non-SUDEP patients. Orange dots represent seizures from SUDEP patients. Pink horizontal bars are mean values by patient.
Figure 3. Estimation statistics for alpha and theta metrics, by two seizure types (GTCS and non-GTCS). Patient number is consistent across all figures, however not all patients have both types of seizures. Ns is number of seizures, Np is number of patients. Blue dots represent seizures from non-SUDEP patients. Orange dots represent seizures from SUDEP patients. Pink horizontal bars are mean values by patient. (A) Alpha for GTCS only. (B) Theta for GTCS only. (C) Alpha for non-GTCS only. (D) Theta for non-GTCS only.
The two SUDEP patients with recordings most distant from death were reserved for testing a classifier (patients 20, 21). Figure 4 compares alpha and theta measures for GTCS in this test set against those of non-SUDEP patients. The results are consistent with those of the training set of SUDEP GTCS seizures, where alpha (p < 0.002), but not theta, was significant.
Figure 4. Estimation statistics for (A) alpha and (B) theta metrics for GTCS of two SUDEP patients reserved for testing, compared to those of non-SUDEP patients; (C) alpha and (D) theta metrics for non-GTCS of SUDEP patient reserved for testing (patient 21), compared to non-SUDEP patients. Patient number is consistent across all figures. Ns is number of seizures, Np is number of patients. Blue dots represent seizures from non-SUDEP patients. Green dots represent seizures from SUDEP patients reserved for testing. Pink horizontal bars are mean values by patient.
For clinical application, a logistic classifier was trained on alpha for all the GTCS. The resulting ROC curve of training data had an area under the curve (AUC) of 94% (Figure 5). When optimizing for accuracy, the resulting F1 score is 80%, with a classifier threshold of 0.305. Each seizure was categorized as SUDEP (1.0) or non-SUDEP (0.0) relative to this threshold, and the mean patient classification scores are plotted. Error bars reflect standard deviation of each population. Both test patients are classified as SUDEP by this system.
Figure 5. Logistic classifier trained on alpha determined from PPCs of GTCS. (A) ROC curve of training data: Accuracy optimized point (red), used to determine classifier decision threshold. (B) Training seizure propensity scores by patient; blue dots are non-SUDEP, orange dots are SUDEP patients. (C) Mean training patient classification scores, with standard deviation shown. (D) Mean patient classification scores for GTCS of two eventual SUDEP patients not included in training set.
We describe a previously unreported PPC-based ECG metric to assess SUDEP risk using patients' GTCS features. Clinical application of this method requires only a 20-s mid-seizure ECG window for PPC analysis and alpha estimation. This study assesses a patient's risk of SUDEP by logistic classification using the alpha metrics of the majority of their seizures.
Two SUDEP test patients were withheld from classifier training. Ictal ECG recordings from the test patients 20 and 21 were acquired 10 and 8 years prior to SUDEP, respectively. Both patients resulted in mean classification scores of 0.75 (Figure 5D), thereby correctly assessed as high risk of SUDEP.
A 2010 matched-pair case-control study evaluated an extensive set of ECG features for SUDEP prediction (23). The study investigated features including peri-ictal heart rate (HR), heart rate variability (HRV), corrected QT interval (QTc), and postictal HR recovery, concluding these were not significant predictors of SUDEP. We therefore aimed to develop a novel ECG metric not derived from existing features to successfully assess SUDEP risk.
Surges et al. suggest that ECG features of SUDEP (maximal ictal HR, postictal HR recovery) identified by Nei et al. (24) can be attributed to a higher prevalence of secondarily GTCS in SUDEP patients (23, 25, 26). There were significant differences in HR and HRV between GTCS and non-GTCS, however a GTCS-only subpopulation was not investigated. A comparison between these parameters and the metrics developed in this study would be beneficial for a larger population of low-artifact ictal ECG recordings.
The assessment developed in this study controls for seizure type by considering only GTCS. The alpha metric in this study stands as a novel ECG feature, without derivation from previously reported ECG metrics. The alpha metric highlights non-linear interactions between different rhythms in the ECG. The set of ECG features used in cardiac investigations should be expanded to include this metric. Our results were obtained in ictal states only, due to limitations of the dataset in this retrospective study. Prospective studies will include sufficient pre- and post-ictal data, and explore the alpha metric in the context of the cardiac syncytium.
We chose a logistic regression to produce a propensity score (27) for each seizure from the cohorts available for this study (Figure 5B). Propensity scores can overcome an inability to pair-match in observational studies. The optimal propensity score threshold was used to categorize patients as SUDEP, or non-SUDEP, based on the classification of the majority of their seizures.
SSA-ICA was successful in decreasing artifacts throughout the recording. ECG artifacts often overlapped with frequency ranges of interest and other blind source separation methods such as ensemble empirical mode decomposition (EEMD) were less suitable under these conditions. Phase-amplitude coupling (28), where the phase of a low frequency rhythm modulates the amplitude of a higher frequency rhythm, has been used in the development of EEG-based biomarkers (29). However, PPC may be more appropriate for ECG-based biomarkers as the phases of cardiac waves are of particular interest and more robust measures than their absolute amplitudes. Measures of phase-phase coherence such as PLV are used to detect synchronization in noisy systems (19). PLV measures the cyclic relative phases between two signals. Higher artifact recordings necessitated lengthier time windows for strong coherence results. This study used a 20-s time-averaged PLV, which resulted in higher-contrast comodulograms and clearer −3 dB thresholds than were obtained for shorter durations. An artifact-tolerant measure based on PPC would enhance investigations of other cardiac-related unexplained death syndromes, such as sudden infant death syndrome (SIDS) (30), among others, and may provide an accessible measure of seizure severity.
A natural extension of this work is the application of this methodology to pre-ictal, post-ictal (29, 31) and inter-ictal ECG recordings in patients with epilepsy. Further, investigation of the PPC comodulograms could isolate the effect of waveform variability. Also, a multivariate approach incorporating EEG, respiration, and ECG would improve the risk assessment of SUDEP. A detailed analysis of the alpha metric would clarify its relationship with cardiac function. Analysis of a larger cohort of SUDEP patients would enhance the statistical power of these conclusions and more rapidly lead to clinical application of this work.
The data analyzed in this study is subject to the following licenses/restrictions: The anonymized datasets used in this study are available upon request. They are not publicly available due to institutional restrictions associated with the original data acquisition protocols. Requests to access these datasets should be directed to BB, YmVyai5iYXJkYWtqaWFuQHV0b3JvbnRvLmNh.
The studies involving human participants were reviewed and approved by the Ethics Committees associated with the Toronto Western Hospital, the New York University (NYU) Comprehensive Epilepsy Center, and the Phramongkutklao Royal Army Hospital. Written informed consent to participate in this study was provided by the participants' legal guardian/next of kin.
All authors listed have made a substantial, direct, and intellectual contribution to the work and approved it for publication.
BB acknowledges grant support by the Natural Sciences and Engineering Research Council of Canada (NSERC), and EpLink—the Epilepsy Research Program of the Ontario Brain Institute. The Ontario Brain Institute is an independent non-profit corporation, funded partially by the Ontario Government. The opinions, results and conclusions are those of the authors and no endorsement by the Ontario Brain Institute is intended or should be inferred. Computations were performed on the Niagara supercomputer at the SciNet HPC Consortium. SciNet was funded by the Canada Foundation for Innovation; the Government of Ontario; Ontario Research Fund—Research Excellence; and the University of Toronto. Finding A Cure for Epilepsy and Seizures (FACES) supported the collection and processing of NYU Langone Epilepsy Center data.
The authors declare that the research was conducted in the absence of any commercial or financial relationships that could be construed as a potential conflict of interest.
All claims expressed in this article are solely those of the authors and do not necessarily represent those of their affiliated organizations, or those of the publisher, the editors and the reviewers. Any product that may be evaluated in this article, or claim that may be made by its manufacturer, is not guaranteed or endorsed by the publisher.
The Supplementary Material for this article can be found online at: https://www.frontiersin.org/articles/10.3389/fneur.2023.1147576/full#supplementary-material
1. Lhatoo SD, Faulkner HJ, Dembny K, Trippick K, Johnson C, Bird J. An electroclinical case-control study of sudden unexpected death in epilepsy. Ann Neurol. (2010) 68:787–96. doi: 10.1002/ana.22101
2. Verducci C, Friedman D, Donner E, Laze J, Devinsky O. SUDEP classification: discordances between forensic investigators and epileptologists. Epilepsia. (2020) 61:173–8. doi: 10.1111/epi.16712
3. Devinsky O. Sudden, unexpected death in epilepsy. N Engl J Med. (2011) 365:1801–11. doi: 10.1056/NEJMra1010481
4. Devinsky O, Hesdorffer DC, Thurman DJ, Lhatoo S, Richerson G. Sudden unexpected death in epilepsy: epidemiology, mechanisms, and prevention. Lancet Neurol. (2016) 15:1075–88. doi: 10.1016/S1474-4422(16)30158-2
5. Bozorgi A, Lhatoo SD. Seizures, cerebral shutdown, and SUDEP: SUDEP - a perfect storm. Epilepsy Curr. (2013) 13:236–40. doi: 10.5698/1535-7597-13.5.236
6. Salam MT, Montandon G, Genov R, Devinsky O, del Campo M, Carlen PL. Mortality with brainstem seizures from focal 4-aminopyridinewn, epi recurrent hippocampal seizures. Epilepsia. (2017) 58:1637–44. doi: 10.1111/epi.13846
7. Lertwittayanon W, Devinsky O, Carlen PL. Cardiorespiratory depression from brainstem seizure activity in freely moving rats. Neurobiol Dis. (2020) 134:104628. doi: 10.1016/j.nbd.2019.104628
8. Aiba I, Noebels JL. Spreading depolarization in the brainstem mediates sudden cardiorespiratory arrest in mouse SUDEP models. Sci Transl Med. (2015) 7:282ra46. doi: 10.1126/scitranslmed.aaa4050
9. Tang F, Hartz AMS, Bauer B. Drug-resistant epilepsy: multiple hypotheses, few answers. Front Neurol. (2017) 8:301. doi: 10.3389/fneur.2017.00301
10. Al-Otaibi FA, Hamani C, Lozano AM. Neuromodulation in epilepsy. Neurosurgery. (2011) 69:957–79. doi: 10.1227/NEU.0b013e31822b30cd
11. Chyou JY, Friedman D, Cerrone M, Slater W, Guo Y, Taupin D, et al. Electrocardiographic features of sudden unexpected death in epilepsy. Epilepsia. (2016) 57:135–9. doi: 10.1111/epi.13411
12. Sivathamboo S, Friedman D, Laze J, Nightscales R, Chen Z, Kuhlmann L, et al. Association of short-term heart rate variability and sudden unexpected death in epilepsy. Neurology. (2021) 97:2357–67. doi: 10.1212/WNL.0000000000012946
13. DeGiorgio CM, Curtis A, Hertling D, Moseley B. Sudden unexpected death in epilepsy: risk factors, biomarkers, and prevention. Acta Neurol Scand. (2019) 139:220–30. doi: 10.1111/ane.13049
14. Cheng J, Li L, Li C, Liu Y, Liu A, Qian R, et al. Remove diverse artifacts simultaneously from a single-channel EEG based on SSA and ICA: a semi-simulated study. IEEE Access. (2019) 7:276–89. doi: 10.1109/ACCESS.2019.2915564
15. Varela F, Lachaux JP, Rodriguez E, Martinerie J. The brainweb: phase synchronization and large-scale integration. Nat Rev Neurosci. (2001) 2:229–39. doi: 10.1038/35067550
16. Devinsky O, Bundock E, Hesdorffer D, Donner E, Moseley B, Cihan E, et al. Resolving ambiguities in SUDEP classification. Epilepsia. (2018) 59:1220–33. doi: 10.1111/epi.14195
17. Zhao Z, Chen Y. A new method for removal of baseline wander and power line interference in ECG signals. Int Conf Mach Learn Cybern. (2006) 4342–7. doi: 10.1109/ICMLC.2006.259082
18. Lechaux JP, Rodriguez E, Martinerie J, Varela FJ. Measuring phase synchrony in brain signals. Hum Brain Mapp. (1999) 8:192–208. doi: 10.1002/(SICI)1097-0193(1999)8:4<194::AID-HBM4>3.0.CO;2-C
19. Tass P, Rosenblum MG, Weule J, Kurths J, Pikovsky A, Volkmann J. Detection of n: m phase locking from noisy data: application to magnetoencephalography. Phys Rev Lett. (1998) 81:3291–4. doi: 10.1103/PhysRevLett.81.3291
20. Hall JK. Algorithms and programs for the rapid computation of area and center of mass. Comput Geosci. (1976) 1:203–5. doi: 10.1016/0098-3004(76)90008-X
21. Ho J, Tumkaya T, Aryal S, Choi H, Claridge-Chang A. Moving beyond P values: data analysis with estimation statistics. Nat Methods. (2019) 16:565–6. doi: 10.1038/s41592-019-0470-3
22. Wilcoxon F. Individual comparisons by ranking methods. Biometrics Bull. (1945) 1:80–3. doi: 10.2307/3001968
23. Surges R, Adjei P, Kallis C, Erhuero J, Scott CA, Bell GS, et al. Pathologic cardiac repolarization in pharmacoresistant epilepsy and its potential role in sudden unexpected death in epilepsy: a case-control study. Epilepsia. (2010) 51:233–42. doi: 10.1111/j.1528-1167.2009.02330.x
24. Nei M, Ho RT, Abou-Khalil BW, Drislane FW, Liporace J, Romeo A, et al. EEG and ECG in sudden unexplained death in epilepsy. Epilepsia. (2004) 45:338–45. doi: 10.1111/j.0013-9580.2004.05503.x
25. Langan Y, Nashef L, Sander JW. Case-control study of SUDEP. Neurology. (2005) 64:113–3. doi: 10.1212/01.WNL.0000156352.61328.CB
26. Tomson T, Nashef L, Ryvlin P. Sudden unexpected death in epilepsy: current knowledge and future directions. Lancet Neurol. (2008) 7:1021–31. doi: 10.1016/S1474-4422(08)70202-3
27. Streiner DL. Statistics commentary series: commentary No. 30: propensity scores. J Clin Psychopharmacol. (2019) 39:991. doi: 10.1097/JCP.0000000000000991
28. Tort ABL, Komorowski R, Eichenbaum H, Kopell N. Measuring phase-amplitude coupling between neuronal oscillations of different frequencies. J Neurophysiol. (2010) 104:1195–210. doi: 10.1152/jn.00106.2010
29. Grigorovsky V, Jacobs D, Breton VL, Tufa U, Lucasius C, Del Campo JM, et al. Delta-gamma phase-amplitude coupling as a biomarker of postictal generalized EEG suppression. Brain Commun. (2020) 2:182. doi: 10.1093/braincomms/fcaa182
30. Ioakeimidis NS, Papamitsou T, Meditskou S, Iakovidou-Kritsi Z. Sudden infant death syndrome due to long QT syndrome: a brief review of the genetic substrate and prevalence. J Biol Res Thessaloniki. (2017) 24:6. doi: 10.1186/s40709-017-0063-1
Keywords: epilepsy, sudden unexpected death in epilepsy, cross-frequency coupling, ECG, signal processing, risk assessment, non-linear interaction in cardiac rhythms
Citation: Gravitis AC, Tufa U, Zukotynski K, Streiner DL, Friedman D, Laze J, Chinvarun Y, Devinsky O, Wennberg R, Carlen PL and Bardakjian BL (2023) Ictal ECG-based assessment of sudden unexpected death in epilepsy. Front. Neurol. 14:1147576. doi: 10.3389/fneur.2023.1147576
Received: 18 January 2023; Accepted: 21 February 2023;
Published: 13 March 2023.
Edited by:
Likun Wang, Affiliated Hospital of Guizhou Medical University, ChinaReviewed by:
Josef Halamek, Institute of Scientific Instruments (ASCR), CzechiaCopyright © 2023 Gravitis, Tufa, Zukotynski, Streiner, Friedman, Laze, Chinvarun, Devinsky, Wennberg, Carlen and Bardakjian. This is an open-access article distributed under the terms of the Creative Commons Attribution License (CC BY). The use, distribution or reproduction in other forums is permitted, provided the original author(s) and the copyright owner(s) are credited and that the original publication in this journal is cited, in accordance with accepted academic practice. No use, distribution or reproduction is permitted which does not comply with these terms.
*Correspondence: Berj L. Bardakjian, YmVyai5iYXJkYWtqaWFuQHV0b3JvbnRvLmNh
Disclaimer: All claims expressed in this article are solely those of the authors and do not necessarily represent those of their affiliated organizations, or those of the publisher, the editors and the reviewers. Any product that may be evaluated in this article or claim that may be made by its manufacturer is not guaranteed or endorsed by the publisher.
Research integrity at Frontiers
Learn more about the work of our research integrity team to safeguard the quality of each article we publish.