- 1School of Sports Medicine and Rehabilitation, Beijing Sport University, Beijing, China
- 2Linfen Central Hospital, Linfen, Shanxi, China
Background: This study aimed to observe the effects of six different types of AI rehabilitation techniques (RR, IR, RT, RT + VR, VR and BCI) on upper limb shoulder-elbow and wrist motor function, overall upper limb function (grip, grasp, pinch and gross motor) and daily living ability in subjects with stroke. Direct and indirect comparisons were drawn to conclude which AI rehabilitation techniques were most effective in improving the above functions.
Methods: From establishment to 5 September 2022, we systematically searched PubMed, EMBASE, the Cochrane Library, Web of Science, CNKI, VIP and Wanfang. Only randomized controlled trials (RCTs) that met the inclusion criteria were included. The risk of bias in studies was evaluated using the Cochrane Collaborative Risk of Bias Assessment Tool. A cumulative ranking analysis by SUCRA was performed to compare the effectiveness of different AI rehabilitation techniques for patients with stroke and upper limb dysfunction.
Results: We included 101 publications involving 4,702 subjects. According to the results of the SUCRA curves, RT + VR (SUCRA = 84.8%, 74.1%, 99.6%) was most effective in improving FMA-UE-Distal, FMA-UE-Proximal and ARAT function for subjects with upper limb dysfunction and stroke, respectively. IR (SUCRA = 70.5%) ranked highest in improving FMA-UE-Total with upper limb motor function amongst subjects with stroke. The BCI (SUCRA = 73.6%) also had the most significant advantage in improving their MBI daily living ability.
Conclusions: The network meta-analysis (NMA) results and SUCRA rankings suggest RT + VR appears to have a greater advantage compared with other interventions in improving upper limb motor function amongst subjects with stroke in FMA-UE-Proximal and FMA-UE-Distal and ARAT. Similarly, IR had shown the most significant advantage over other interventions in improving the FMA-UE-Total upper limb motor function score of subjects with stroke. The BCI also had the most significant advantage in improving their MBI daily living ability. Future studies should consider and report on key patient characteristics, such as stroke severity, degree of upper limb impairment, and treatment intensity/frequency and duration.
Systematic review registration: www.crd.york.ac.uk/prospero/#recordDetail, identifier: CRD42022337776.
Introduction
Globally, stroke is the leading cause of disability in adults, often resulting in symptoms such as muscle weakness, sensory deficits, spasticity, balance problems, reduced dexterity, communication difficulties and cognitive impairment (1). Evidence shows that 40% of people with a stroke still have upper limb impairment, which can lead to limited movement (2–4). Meanwhile, only 5 to 20% of stroke survivors recover full upper limb function, 25% recover partial upper limb function, and 60% have a complete loss of upper limb function (5). Consequently, reduced motor function of the upper limb (e.g., reaching and grasping) can have a significant negative impact on the ability to perform activities of daily living (ADLs) (e.g., eating, dressing and washing) (6).
One study found changes in the affected upper limbs were usually more apparent than in the affected lower limbs (7), including functional limitations in the affected arms and slow, uncoordinated movements of the hands (8, 9). Another study found that subjects with strokes had difficulty performing reaching tasks and movement when manipulating objects due to changes in timing and coordination as well as abnormal postural adjustments (10, 11) or were unable to control grip and fingertip strength (12, 13). Due to a combination of physical, cognitive and perceptual problems, those who have suffered strokes often have difficulty participating in family, work and community life and performing ADLs such as feeding, dressing and grooming (14).
Functional performance of the affected upper limbs can be improved if the subject with stroke has adequate opportunities for exercise. Different techniques and methods can be used in rehabilitation management (e.g., physiotherapy, occupational therapy, conductive education, splinting, pharmacotherapy and surgery) and specific techniques (e.g., neurodevelopmental therapy (NDT) or constraint-induced movement therapy (CIMT) (15–19). However, no strong evidence exists about successful treatment using any of these techniques or methods.
With the rapid development of rehabilitation management technology, artificial intelligence (AI) technology, represented by rehabilitation robots (RT), has received widespread attention from medical researchers (20). AI is defined as the study of disciplines that enable computers to simulate human thought processes and intelligent behaviors (such as learning, reasoning, thinking, planning, etc.). This study mainly includes assessing the principles by which computers are manufactured to replicate and realize human brain intelligence and can achieve higher-level applications (21). Meanwhile, the upper limb RT is a medical robot that facilitates the recovery of upper limb function by driving the patient through repetitive upper limb movement training with mechanical assistance (22). Additionally, brain-computer interface (BCI) electrical stimulation training is a new method of central neurological intervention that collects signals from the patient's brain during motor imagery tasks, converts them into computer commands, and applies electrical stimulation to the paralyzed limb. This enables the establishment of a “central-peripheral-central” closed-loop rehabilitation training model that promotes central re-modeling and peripheral control, thereby facilitating the recovery of motor function (23, 24).
Remote rehabilitation (RR) is a rehabilitation model that uses Internet communication technology to achieve inter-temporal treatment between medical workers and patients, which is convenient, fast and without time and space boundaries, and supports the continued rehabilitation training of patients after discharge from hospital (25). Intelligent rehabilitation (IR) is a new type of intelligent biofeedback therapy device which uses two-dimensional virtual games as biofeedback to conduct interactive training with patients through visual, auditory and tactile forms. IR can be used to assess and train patients' manual motor and sensory functions and to rehabilitate people with cognitive impairment (26). Similarly, virtual reality (VR) technology is an effective tool for stroke rehabilitation, using computers to generate a virtual environment that simulates reality and uses a variety of sensing devices to “immerse” the user in that environment, enabling the user to interact naturally with the virtual environment (27). Combining the characteristics of the AI technologies mentioned above reveals that BCI, RR and VR share common ground regarding training characteristics. Moreover, IR contains the virtual interactive scenarios found in VR technology. However, the training principles of RR, RT and BCI are different. RT emphasizes mechanical assistance for hemiplegic upper limbs, BCI emphasizes central neural integration, based on central integration and peripheral control to assist rehabilitation training, and RR emphasizes online 5G technology to provide online rehabilitation guidance for home rehabilitation patients. Non-invasive VR, on the other hand, detects the thought activity of the brain through a non-implantable device, and the signal is substantially attenuated as it passes through the skull, resulting in low signal intensity and accuracy. Invasive BCIs require the implantation of electrodes into the cerebral cortex to enable interaction and thus have sufficiently precise and risky properties.
Researchers Erosy and Iyigun demonstrated that virtual and real boxing training significantly restored motor function in the hemiplegic upper limbs of subjects with stroke, which supports the effectiveness of virtual boxing training (28). Rodríguez-Hernández et al. showed that VR was more effective than traditional rehabilitation methods in improving stroke patients' quality of life (29). Also, another study found that combining traditional physical fitness with VR technology increased patients' interest in rehabilitation and made them more engaged, leading to better clinical outcomes (30). Previous research confirms that BCI combined with other treatments such as robotic orthoses, mobile robots, VR devices and functional electrical stimulation (FES) effectively improve limb function in subjects with stroke (31). Another study confirmed that RR technology, based on large data platforms, can enable patients to access quality rehabilitation medical services at home, provide long-term rehabilitation support for patients and their families, reduce the gap between in- and out-patients and facilitate function recovery as well as the continuity of rehabilitation treatment (32).
However, the aforementioned studies all investigated the effect of a single AI technique on upper limb function amongst subjects with strokes. Network meta analysis (NMA) may provide a way to address this issue. In randomized controlled trials (RCTs), a quantitative summary of the “network of evidence” is achieved by combining the direct and indirect effects of three or more interventions compared with the same comparative intervention (usually a control or no-treatment intervention) (33). This is also referred to as a multiple treatment comparison (34). In this way, NMA can quantitatively combine evidence on the effectiveness of interventions directly compared in the same RCTs (direct comparison) and interventions from different RCTs with a common comparator (indirect comparison) (33).
At present, most of the meta-analyses at home and abroad investigating AI rehabilitation focus on the single RR and the effects of BCI, VR and RT on the upper limb function and motor function of subjects with stroke. There are few reports on NMA analysis of various AI techniques used in rehabilitating the upper limb function of subjects with stroke (35–38). There is only one NMA analysis on the use of upper limb RT in upper limb motor function in subjects with stroke. The study observed its effect on upper limb function in subjects with stroke by drawing multiple comparisons among different models of upper limb RT. Its indirect comparison showed that none of the types of upper limb RT were better or worse than any other RT, nor did it provide clear evidence to support the choice of a specific type of robotic device to facilitate arm recovery (39).
The present NMA analysis integrates all new AI technologies based on previous studies. Therefore, this study aimed to provide a systematic overview of current RCTs of different modalities of AI techniques and assess their relative effectiveness using an NMA. We aimed to assess the relative influence of different modalities of AI techniques on ADLs, hand/arm function and overall upper limb motor function amongst subjects with strokes and explore the safety of these techniques.
Methods
Study enrollment and reporting
The protocol was based on the preferred reporting items for systematic reviews and meta-analyses protocols (PRISMA guidelines 2020) (40). PRISMA extension statements were used to ensure that all aspects of methods and results were reported (41). The protocol is registered in PROSPERO [registration number CRD42022337776 (https://www.Crd.york.ac.uk/prospero/#recordDetails)].
Search strategy
The study was conducted using PubMed, Embase, Web of Science, the Cochrane Library and CNKI, Wanfang and VIP databases in English and Chinese. A comprehensive and reproducible literature search was undertaken up to September 2022. We developed a search strategy for a combination of thematic terms and free terminology based on the Population, Intervention, Comparison, Outcome, Study Design (PICOS) principles. The specific search protocol included various medical topics and free-text terms related to stroke, cerebrovascular disease, upper limb and hand dysfunction and AI to obtain a broad range of literature for further analysis. PubMed is used as an example, and the specific search strategy is provided in Supplementary Table S1.
Inclusion criteria
The inclusion criteria were as follows: (1) Population: the diagnostic criteria for stroke were met by the Classification of Cerebrovascular Diseases, and a diagnosis of cerebral infarction or cerebral hemorrhage was made by cranial CT or MRI (2, 42, 43). Intervention: six AI technologies (RT, BCI, RR, IR, VR, RT + VR) were used as intervention methods, alone or in combination with artificial intelligence rehabilitation; (3) Comparison: the control group received only conventional rehabilitation or any of the above intervention groups; (4) Outcome: primary outcome: FMA-UE-Total, secondary outcome: FMA-UE-Distal, FMA-UE-Proximal, ARAT and MBI; (5) Study design: only RCTs were included in this study.
Exclusion criteria
The exclusion criteria were as follows: (1) other neurological disorders; (2) no accurate diagnosis or inconsistent with the included diagnosis; (3) no outcome indicators or inconsistent with the study indicators; 4) interventions inconsistent with the inclusion criteria; (5) duplicate published studies or incomplete study data even after contacting the authors; and (6) systematic reviews, meta-analyses, theoretical studies, expert reviews, animal experiments, conference reports, economic analyses or case reports.
Study selection
EndNote (version X20, Clarivate, Philadelphia, Pennsylvania, USA) was used to process the search records. Two reviewers (JL and CW) independently screened the titles and abstracts against the developed inclusion and exclusion criteria. This was followed by reading the full text to exclude documents that did not meet the inclusion criteria. Finally, the two authors identified the remaining literature for inclusion. During this process, any discrepancies were discussed and resolved by the third author (YZ).
Data extraction and quality assessment
We completed the data extraction using Microsoft Excel. The data extraction was strictly based on author(s), year of publication, specific information about treatment and control groups and primary and secondary outcome indicators. Any disagreements between the two reviewers (LZ and YZ) were judged by a third reviewer (P.Z. Zhang). Two reviewers (YZ and CW) assessed the potential risk of bias in each study by independently using the Cochrane Risk of Bias Tool (44). We assessed seven areas: random sequence generation, allocation concealment, blinding of participants and personnel, blinding of outcome assessment, incomplete outcome data, selective outcome reporting and other possible biases. Each item was rated as unknown, low, or high risk of bias. The assessment was performed in Review Manager (version 5.3). The reviewer discrepancies were also addressed through discussions with a third reviewer (PZ) (45).
Statistical analysis
When trials used the same testing procedure (e.g., Barthel Index), we calculated the mean difference (MD) and the corresponding 95% confidence interval (CI). We calculated the standardized mean difference (SMD) using the 95% CI if various outcome measures were used for a given endpoint. For dichotomous endpoints, we determined the index of risk difference (RD) using the 95% CI.
We generated visual forest plots for all direct and indirect comparisons and compiled a relative ranking of each intervention based on the surface under the cumulative ranking line (SUCRA) (46). The SUCRA value calculates the percentage efficacy of each individual intervention compared with the “ideal” treatment. We performed all statistical analyses using the software STATA MP version 16.0 (47).
The network meta-analysis was based on a frequency approach, with weighted least squares for multiple regression with random effects. The method allows full consideration of multi-arm studies and includes restricted maximum likelihood estimates (48).
To test the possible assumptions of the transitivity hypothesis, we assessed global inconsistency utilizing consistency and inconsistency models (48, 49). Transitivity implies no systematic differences between the individual arms of the studies. At the local level, we used a node-splitting approach (48, 50). In addition to quantitative testing, we also qualitatively validated test descriptions that contained significant effect modifiers.
In the network diagram, we assessed the risk of bias between trials for each dimension as a study-level covariate along three dimensions (randomized sequences, hidden and blinded for randomized sequences).
Results
Results of study identification and selection
We included a total of 33,306 studies using the original search terms and 73 original studies in combination with the manual search. After screening for duplicates by de-duplication, we obtained 25,770 original papers. Following this, we obtained 18,387 original papers by applying the inclusion and exclusion criteria combined with abstract reading. After reading the full texts, we obtained 91 original papers (including 8,700 deleted for study purposes, 1,599 deleted for study subjects, 2,141 deleted for intervention methods, 783 deleted for outcome indicators, 1,799 deleted for non-RCTs, 345 deleted for data format discrepancies, 2,660 deleted for duplicate publications and 259 deleted for other reasons). Finally, we included 101 studies (Among them, 91 were filtered and 10 were manually searched according to the purpose of the study) in the risk assessment and NMA. The process of selection of the eligible studies was shown in Figure 1.
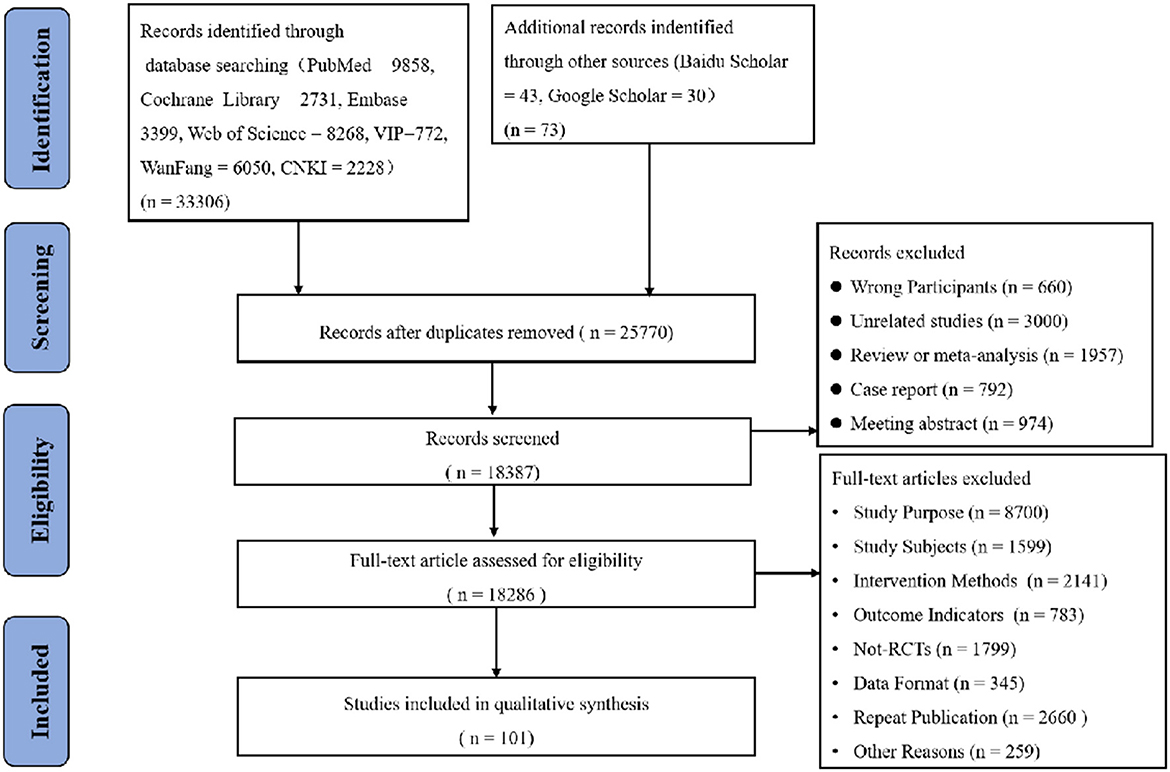
Figure 1. Flow diagram of eligible studies selection process. CNKI, China national knowledge infrastructure; WanFang knowledge servise platform; VIP, Chinese sceintific journals database; n, number of publications.
Characteristics of the included studies
We eventually included 101 RCTs, with 2,390 participants in the experimental group (EG) and 2,312 in the control group (CG). Of the 101 studies included, 44 were published in Chinese and 57 in English. The publication period was from 2008 to 2022. The primary outcome indicator was FMA-UE-Total, and the secondary indicators were FMA-UE-Distal, FMA-UE-Proximal, Modified Barthel Index (MBI) and ARAT. Table 1 details the essential characteristics of the included studies.
Quality assessment of the included studies
We assessed the risk of bias for each study using the Cochrane Risk of Bias Tool. All studies included RCTs that reported the random sequence generation and allocation concealment with a low risk of bias. Almost two-thirds of the studies were shown to be at low risk in both the blinding of participants and personnel and the blinding of outcome assessment. Of these, only 10% of the studies in the blinding of participants and personnel showed high risk, and 10% showed unclear risk. In the blinding of outcome assessment, ~ 20% of the studies showed high risk, and 20% showed unclear risk. Of the incomplete outcome data, ~ 70% showed unclear risk, 5% showed low risk and 25% showed high risk. In the selective reporting, ~ 15% showed low risk, 60% showed unclear risk and 25% showed high risk. Of the other biases, ~ 35% showed low risk, 30% showed unclear risk and 30% showed high risk. Figure 2 contains detailed information on the risk of bias.
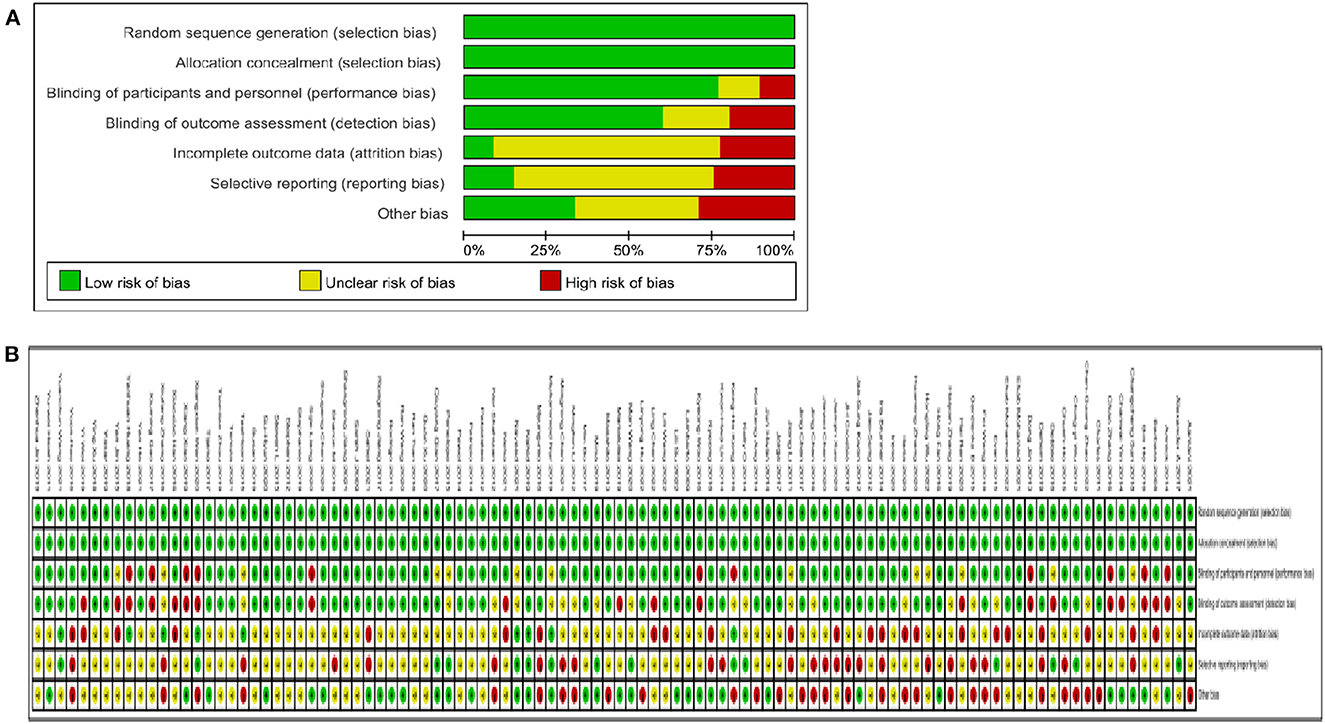
Figure 2. Quality assessment of selected studies by the cochrane risk of bias tool. (A) Risl of bias graph: review authors judgments about each risk of bias item presents as percentages across all included studies. (B) Risk of bias summary: review authors judgements about each risk of bias item for each included study.
Results of the network meta-analysis
Evidence network diagram
A total of 101 studies, 4,702 subjects were included in this study involving six interventions (RT, BCI, RR, IR, VR, RT + VR). A total of 71 studies were included in the network evidence map of the FMA-UE-Total involving the following interventions: CT, RT, BCI, RR, IR, VR, and RT + VR. We included a total of 49 studies in the MBI network evidence map involving interventions such as CT, RT, BCI, RR, IR, VR, and RT + VR. We included 22 studies in the ARAT network evidence map involving CT, RT, BCI, RR, IR, VR, and RT + VR interventions. Moreover, we included a total of 26 studies in the FMA-UE-Proximal network evidence map involving interventions such as CT, RT, BCI, RR, IR, VR, and RT + VR, and we included 28 studies in the network evidence map for the FMA-UE-Distal, involving interventions such as CT, RT, BCI, RR, IR, VR, and RT + VR. Figures 3A–E shows the details of the NMA map.
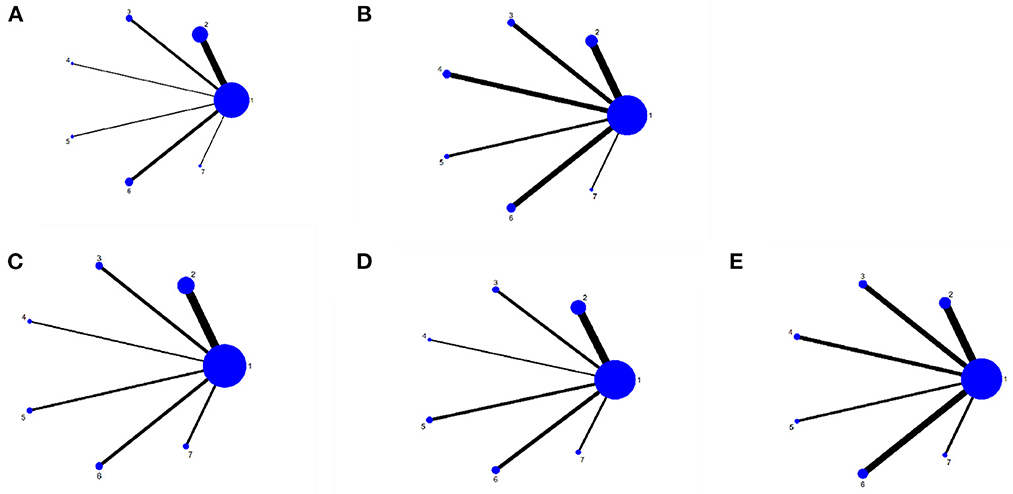
Figure 3. Network meta-analysis diagrams of eligible comparisons. (A) FMA-UE-Total, (B) MBI, (C) FMA-UE-Proximol, (D) FMA-UE-Distal, (E) FMA-UE-ARAT. Width of the lines is proportional to the number of trial. Size of every circle is proportional to the number of randomly assignes participants (sample size). 1, conventional training (CT); 2, Robot training (RT); 3, Brain-computer interface (BCI); 4, Remote rehabilitation (RR); 5, Intelligent rehabilitation (IR); 6, Virtual reality (VR); 7, Robot training + virtual reality (RT+VR).
Primary outcome
FMA-UE-total
We included 71 studies in the FMA-UE-Total in the comparison (51–66, 69–80, 87, 88, 91, 92, 147). As the network evidence map in this study did not form a closed loop, indirect comparisons and inconsistency tests could not be performed (148). However, P > 0.05 in the consistency test indicated excellent consistency and stability of the studies.
The NMA results showed that all interventions were not statistically significant, indicating that AI rehabilitation techniques did not significantly improve upper limb motor function amongst subjects with strokes (see Table 2). Figure 4A shows the SUCRA rankings for all treatments. Based on the results of the SUCRA analysis, IR [(SMD = 0.02, 95%CI = (−0.40, 0.43)] (SUCRA, 70.5%) was the most effective intervention for improving upper limb motor function amongst subjects with strokes, followed by BCI [(SMD = 0.001, 95%CI = (−0.46, 0.46)] (SUCRA, 69.5%); RT + VR [(SMD = 0.06, 95%CI = (−0.39, 0.51)] (SUCRA, 65.9%); VR [(SMD = 0.06, 95%CI = (−0.36, 0.48)] (SUCRA, 58.1%); RR [(SMD = 0.07, 95%CI = (−0.30, 0.45)] (SUCRA, 45.6%); RT [(SMD = 0.05, 95%CI = (−0.09, 0.20)] (SUCRA, 28.0%) and CT (SUCRA, 12.3%).
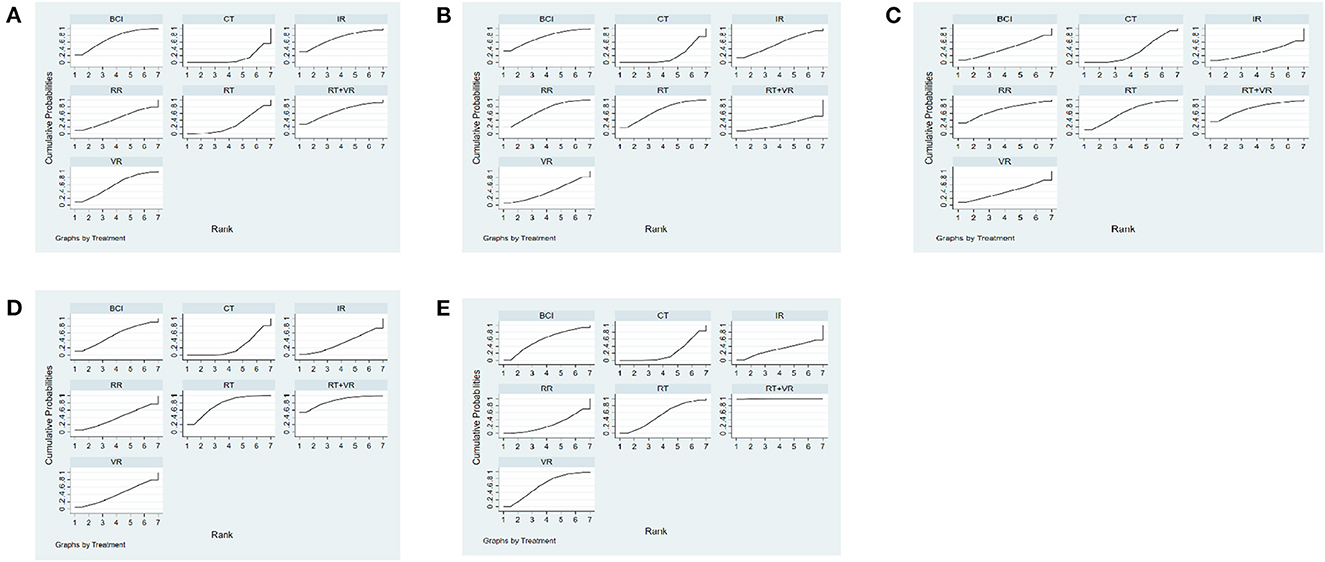
Figure 4. Cumulative probability ranking curve of different interventions. (A) FMA-UE-Total, (B) MBI, (C) FMA-UE-Proximal, (D) FMA-UE-Distal, (E) FMA-UE-ARAT. The fuller the area under the curve, the more effective it is. 1, conventional training (CT); 2, Robot training (RT); 3, Brain-computer interface (BCI); 4, Remote rehabilitation (RR); 5, Intelligent rehabilitation (IR); 6, Virtual reality (VR); 7, Robot training + virtual reality (RT+VR).
Secondary outcome
Modified barthel index
A total of 49 studies were included in the comparison of the MBI. As the network evidence map in this study did not form a closed loop, inconsistency tests could not be performed (148). However, P > 0.05 in the consistency test indicated excellent consistency and stability of the studies.
The NMA results showed no significant differences between the interventions for both direct and indirect comparisons, indicating that different modalities of AI technology had no significant effect on improving MBI function in subjects with stroke (see Table 3). Figure 4B shows the SUCRA rankings for all treatments. According to the results of the SUCRA analysis, BCI [(SMD = 0.03, 95%CI = (−0.24, 0.29)] (SUCRA, 73.6%) was the most effective intervention for improving quality of daily life amongst subjects with strokes, followed by RR [(SMD = 0.001, 95%CI = (−0.22, 0.23)] (SUCRA, 68.1%); RT [(SMD = 0.04, 95%CI = (−0.23, 0.31)] (SUCRA, 67.8%); IR [(SMD = 0.05, 95%CI = (−0.25, 0.36)] (SUCRA, 54.6%); VR [(SMD = 0.09, 95%CI = (−0.34, 0.51)] (SUCRA, 41.1%); RT + VR [(SMD = −0.03, 95%CI = (−0.39, 0.34)] (SUCRA, 26.5%) and CT (SUCRA, 18.4%).
FMA-UE-proximal
We included 26 studies in the FMA-UE-Proximal comparison. As the network evidence map in this study did not form a closed loop, inconsistency tests could not be performed (148). However, P > 0.05 in the consistency test indicates excellent consistency and stability of the studies.
The NMA study showed there were significant differences in direct comparisons between VR + RT [(SMD = 0.43, 95%CI = (0.01, 0.85)], RT [(SMD = 0.32, 95%CI = (0.07, 0.59)] and CT. There were no significant differences in direct and indirect comparisons between the other interventions. This suggests VR + RT and RT effectively improve motor function of the upper limb shoulder and elbow joints amongst subjects with strokes (see Table 4). Figure 4C shows the SUCRA rankings for all treatments. Based on the results of the SUCRA analysis, RT + VR (SUCRA, 84.8%) was the most effective intervention for improving shoulder and elbow joint motor function amongst subjects with strokes, followed by RT (SUCRA, 75.5%), BCI [(SMD = 0.10, 95%CI = (−0.49, 0.69)] (SUCRA, 54.7%); VR [(SMD = 0.01, 95%CI = (−0.62, 0.63)] (SUCRA, 39.6%); RR [(SMD = 0.04, 95%CI = (−0.57, 0.65)] (SUCRA, 38.9%), IR [(SMD = 0.05, 95%CI = (−0.36, 0.45)] (SUCRA, 34.2%) and CT (SUCRA, 22.3%).
FMA-UE-Distal
We included 28 studies in the FMA-UE-Distal comparison. As the network evidence map in this study did not form a closed loop, inconsistency tests could not be performed. However, P > 0.05 in the consistency test indicates excellent consistency and stability of the studies.
The NMA results showed no significant differences between the interventions, compared directly and indirectly, suggesting different modalities of AI techniques did not significantly influence the improvement of wrist joint motor function in the upper limbs of subjects with stroke (see Table 5). Figure 4D shows the SUCRA rankings for all treatments. Based on the results of the SUCRA analysis, RT + VR [(SMD = 0.03, 95%CI = (−0.47, 0.52)] (SUCRA, 74.1%) was probably the most effective intervention for improving wrist joint motor function amongst subjects with strokes, followed by RR [(SMD = 0.06, 95%CI = (−0.35, 0.46)] (SUCRA, 70.1%); RT [(SMD = 0.11, 95%CI = (−0.29, 0.51)] (SUCRA, 63.8%); BCI [(SMD = 0.02, 95%CI = (−0.52, 0.55)] (SUCRA, 40.8%); VR [(SMD = −0.001, 95%CI = (−0.41, 0.41)] (SUCRA, 38.5%); CT [(SMD = 0.05, 95%CI = (−0.33, 0.44)] (SUCRA, 32.5%) and IR (SUCRA, 30.4%).
Action research arm test
A total of 22 studies were included in the ARAT comparison (60, 81, 82, 131, 133, 139, 142, 144, 149). As the network evidence map in this study did not form a closed loop, inconsistency tests could not be performed (148). However, P > 0.05 in the consistency test indicated excellent consistency and stability of the studies.
The NMA results showed significant differences in all interventions compared to CT, suggesting RT + VR [(SMD = 0.73, 95%CI = (0.20, 1.26)], VR [(SMD = 0.73, 95%CI = (0.14, 1.32)], BCI [(SMD = 0.78, 95%CI = (0.25, 1.31)], RT [(SMD = 0.93, 95%CI = (0.17, 1.70)], IR [(SMD = 0.92, 95%CI = (0.36, 1.48)] and RR [(SMD = 0.91, 95%CI = (0.44, 1.39)] were effective in improving ARAT function in subject with stroke (see Table 6). Figure 4E shows the SUCRA rankings for all treatments. Based on the results of the SUCRA analysis, RT + VR (SUCRA, 99.6%) was the most effective intervention for improving hand function amongst subjects with strokes, followed by VR (SUCRA, 60.9%), BCI (SUCRA, 57.7%), RT (SUCRA, 51.9%), IR (SUCRA, 30.1%), RR (SUCRA, 27.0%) and CT (SUCRA, 22.8%).
Presence of adverse effects
A network meta-analysis of adverse reactions could not be completed further as all included studies did not report adverse reactions.
Publication bias and consistency assessment
We constructed a comparative corrected funnel plot of the main results of FMA-UE-Total for evaluation via Stata/MP 16.0. Figure 5 shows the funnel plots show a symmetrical distribution, indicating limited publication bias in this study.
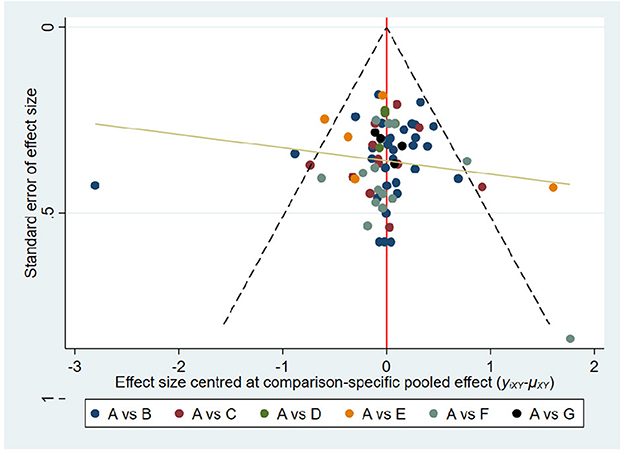
Figure 5. Comparison-adjusted funnel plots. FMA-UE total. The fuller the area under the curve, the more effective it is. A, conventional training (CT); B, Robot training (RT); C, Brain-computer interface (BCI); D, Remote rehabilitation (RR); E, Intelligent rehabilitation (IR); F, Virtual reality (VR); G, Robot training + virtual reality (RT+VR).
Discussion
We conducted a systematic evaluation by NMA analysis, including 101 studies involving 4,702 subjects. The results of the NMA analysis showed that, in ARAT, there were significant differences in direct and indirect comparisons of RT + VR with each of the other interventions. In the FMA-UE-Proximal, there were significant differences in direct comparisons between RT + VR and RT vs. CT. Meanwhile, there were no significant differences between direct and indirect comparisons for each of the other interventions. However, in FMA-UE-Total, FMA-UE-Distal and MBI, there were no significant differences in direct and indirect comparisons between the interventions. Overall, there were no significant adverse effects in any of the studies, indicating the strong reliability and safety of the results.
Our NMA analyses may provide new and valuable insights into using different modalities of AI technology in the functional rehabilitation of the upper limb among subjects with strokes. We believe these analyses may be seen as complementary to previous systematic reviews on this topic.
In this study, we compared the effects of different AI techniques on FMA-UE-Proximal amongst subjects with strokes. The analysis showed that RT + VR [SMD = 0.26; 95% CI (−0.26, 0.78)] was the best treatment for improving overall outcomes in improving wrist and shoulder joint motor function in the upper limbs of subjects with strokes. RT + VR (SUCRA = 84.8%) was also the most effective treatment, according to the SUCRA results. Our NMA results also found a significant difference between RT + VR and RT in improving wrist joint motion amongst subjects with strokes compared with CT. In contrast, a single RCT by Chen and Jiang showed no significant difference between RT + VR in improving wrist motion amongst subjects with strokes (113, 150). Chen and Jiang further mentioned that, due to the absence of other forms of hand function training with RT + VR, the upper limb RT device often left the wrist and hand in a relatively fixed position compared with conventional exercise therapy. The improvement in hand function was not significant when compared with conventional rehabilitation. To some degree, this is inconsistent with the findings of this study. Some studies using RT technology to observe its effect on the function of the upper limb wrist and shoulder elbow joint in subjects with stroke have shown that upper limb RT can effectively improve the wrist and shoulder elbow joint function of the upper limb of subjects with stroke (64). Another large study showed that VR technology can also effectively improve the wrist motor function of the upper limbs of subjects with stroke (151). This also indirectly confirms that the use of RT combined with VR may be more advantageous, such as the NMA results indicated in this study, which showed that RT + VR is the best treatment to improve the function of upper limbs, shoulders, elbows and wrists in subjects with strokes. At the same time, the search at home and abroad found that most scholars did not separately evaluate the three sub-terms of shoulder joint, wrist joint and elbow joint in FMA-UE to observe the effect of RT, VR and RT + VR technology on the motor function of the above three joints in subjects with stroke. The meta-analysis did not classify the three sub-terms of FMA-UE, so it was not possible to observe the effects of RT and VR technology on the motor function of the above three joints in subjects with stroke by meta-synthesis (37, 152).
The present study, in contrast, is based on a multiple comparison NMA analysis and provides a summary of previous studies. Therefore, the results of this study may be somewhat more convincing than the above studies. Similarly, RT significantly improved wrist motor function in the upper limbs of subjects with strokes, consistent with the findings from Kwon et al. (111). Moreover, the results combined with the NMA analysis further suggest that RT + VR [SMD = 0.43; 95% CI (0.01, 0.85)] may be the optimal treatment in terms of improving wrist motor function amongst subject with strokes. On the one hand, our NMA also found that direct and indirect comparisons between studies showed no significant differences in the FMA-UE-Total and FMA-UE-Distal comparisons. In FMA-UE-Distal, IR ranked first out of seven different AI techniques (SUCRA = 70.5%), reflecting that it was the optimal treatment. In the FMA-UE-Total, RT + VR [SMD = 0.26; 95% CI (−0.07, 0.60)] ranked first out of seven different AI techniques (SUCRA = 74.1%), reflecting that it was the optimal treatment. We speculate that the reason for this lack of significant difference may be related to the inconsistent duration of disease (acute, sub-acute and chronic), varying age ranges, and inconsistent Brunnstrom motor function staging and balance in the subjects included in the original study (60, 72, 86, 88, 109).
The ARAT assesses changes in limb function, including the ability to manage objects with different physical characteristics. It is a valid and applicable assessment of changes in upper limb motor function following the onset of a stroke. It involves 19 items divided into four sub-scales: grip, grip strength, pinch and gross motor (153, 154). In this study, our NMA results suggest that RT + VR [SMD = 0.91; 95% CI (0.44, 1.39)] was most effective in improving ARAT in subjects with upper limb dysfunction after a stroke. According to the SUCRA results, RT + VR (SUCRA = 99.6%) was the most effective treatment. Simultaneously, there was a significant difference between RT + VR in improving the motor function of the upper limbs of ARAT amongst subjects with strokes compared with other interventions. However, some high-quality meta-analyses and multi-center RCTs have provided mixed conclusions.
One of the Cochrane meta-analyses showed a significant improvement in upper extremity ARAT function in subjects with stroke who received an upper extremity RT intervention, with significant changes in arm function and no significant difference in arm strength (155). A multi-center large RCT from Lancet also showed that upper extremity RT training was ineffective in improving upper limb ARAT function in subjects with stroke (156). Although there was no statistically significant difference in the results, there was a clear trend toward improvement at 3 and 6 months, with the authors suggesting that the reason for the absence of a difference may be related to wear and tear on the upper extremity RT training apparatus, participant adherence, and attrition rates (156). A multi-center study of VR came to the same conclusion as Lancet, showing that VR did not show significant between-group differences in improving ARAT in subjects with strokes compared with CT (157). Meanwhile, the combined use of VR technology and the upper limb rehabilitation robot allows the two to complement each other, thus effectively improving the ARAT motor function of the upper limbs amongst subjects with strokes (82). In contrast, this study is based on multiple comparative NMA studies, summarizing previous studies' shortcomings through direct and indirect comparisons. Therefore, the reliability of the results of this study is somewhat convincing.
The MBI consists of 10 items, including eating, bathing, grooming, dressing, stooling, urinating, toileting, transferring, walking and ascending and descending stairs and is often used to assess basic ADLs amongst subjects with strokes (96, 158). The results of the NMA by Li et al. (36) found that different modalities of BCI improved upper limb motor function and ADLs amongst subjects with strokes, with BCI and an FES as the driving device having the best effect (36). Our NMA analysis found that direct and indirect comparisons between studies showed no significant differences in improving the quality of daily life amongst subjects with strokes. At the same time, BCI [SMD = 0.18; 95% CI (−0.03, 0.39)] ranked best among seven different AI techniques (SUCRA = 73.6), reflecting that it was the most effective treatment. In conjunction with the study by Li et al., the BCI technique effectively improved the ability of subjects with strokes to perform daily living activities (36). Their findings are not consistent with those of our NMA study. We speculate that this may be related to the age, duration of disease, location of symptoms and functional recovery of the subjects included in the study (95, 159, 160).
This study provides an innovative, systematic integration of various AI rehabilitation techniques for direct and indirect comparison to establish which AI rehabilitation techniques were most effective in improving upper limb function in subjects with stroke. Based on clinical considerations, this study concluded that RT + VR had a significant advantage in improving shoulder and wrist joint motor function and ARAT in subjects with stroke, and IR and BCI had a significant advantage in improving upper limb motor function and MBI in FMA. Therefore, based on the evidence from this study, RT + VR, IR and BCI techniques can be recommended as the preferred treatment method for upper limb functional rehabilitation in subjects with stroke, which also provides evidence-based information for using and promoting AI rehabilitation techniques in clinical practice. Follow-up studies should provide more precise and personalized treatment protocols based on key characteristics of subjects with stroke, such as the severity of a stroke and the degree of upper limb impairment, as well as the intensity, frequency and duration of treatment, depending on their different clinical characteristics and degree of impairment. In terms of methodology, researchers also need to better describe interventions (both tailored and individualized) and ensure that the implementation and delivery of interventions are accurately documented, with attention to symptom reduction, independence and function. There should also be reporting on barriers to implementation and measuring the potential impact and harm of AI technologies.
Strengths and limitations
First, our study included 101 studies and 4,702 patients, indicating a large sample size. Moreover, we involved seven treatment interventions and assessed the impact of the interventions in six ways to provide more comprehensive evidence-based recommendations. Second, most of the systematic reviews and meta-analyses of AI rehabilitation have assessed the effects of single RT, VR and BCI on upper limb function amongst subjects with strokes, with only one network meta-analysis reporting a study of different types of upper limb RT on upper limb function amongst subjects with strokes. We conducted the first NMA of different modalities of AI on upper limb function amongst subjects with strokes, providing the initial basis for further detailed studies in this area (39). This study also had limitations, including the following: (1) Many studies did not specifically report on randomization methods, allocation concealment and reliability of outcomes. The different treatment durations, frequencies and protocols included in the studies may have increased clinical heterogeneity. (2) Most original studies used semi-quantitative scales to assess shoulder-elbow and wrist joint motor function and total upper limb motor function scores and ADLs amongst subjects with strokes and did not use more objective and quantitative indicators. In subsequent studies, we must further use a combination of subjective and objective indicators to assess the improvement in their overall function. (3) We have created network diagrams that clearly illustrate the direct comparisons made in this domain; however, there were no closed loops in the network geometry. This led to our analysis not being an NMA or multiple treatment comparison (MTC) in the strictest sense but rather an adjusted indirect treatment comparison (ITC) belonging to the genus NMA.
Conclusion
The results of our NMA and SUCRA rankings suggest RT + VR appears to have an advantage over other interventions in improving upper limb motor function amongst subjects with stroke with FMA-UE-Proximal, FMA-UE-Distal and ARAT. Similarly, IR had shown the most significant advantage over other interventions in improving the FMA-UE-Total upper limb motor function score of subjects with stroke. The BCI also had the most significant advantage in improving their MBI daily living ability. In addition, it is worth noting that future studies should consider and report key patient characteristics such as stroke severity and degree of upper limb impairment, as well as treatment intensity, frequency and duration. Future meta-analyses should consider sub-group analyses based on the duration of the subject's illness and intervention and their gender to comprehensively explore the impact of different AI modalities and techniques on subjects with stroke from different populations.
Data availability statement
The original contributions presented in the study are included in the article/Supplementary material, further inquiries can be directed to the corresponding author.
Author contributions
Conceptualization: YZ and CW. Methodology and software: CW and JL. Validation, investigation, and data management: YZ and LZ. Formal analysis and visualization: LZ. Resources: YZ. Writing—original draft preparation: YZ, CW, and PZ. Writing—review and editing: YZ, CW, JL, LZ, and PZ. Supervision and funding acquisition: PZ. Project management: YZ and PZ. All authors contributed to the article and approved the submitted version.
Funding
This study was supported by the National Key Research and Development Program of China (2022YFC2010201) and Fundamental Research Funds for the Central Universities (2020045).
Acknowledgments
We are sincerely grateful to all members of our team and reviewers for their valuable comments.
Conflict of interest
The authors declare that the research was conducted in the absence of any commercial or financial relationships that could be construed as a potential conflict of interest.
Publisher's note
All claims expressed in this article are solely those of the authors and do not necessarily represent those of their affiliated organizations, or those of the publisher, the editors and the reviewers. Any product that may be evaluated in this article, or claim that may be made by its manufacturer, is not guaranteed or endorsed by the publisher.
Supplementary material
The Supplementary Material for this article can be found online at: https://www.frontiersin.org/articles/10.3389/fneur.2023.1125172/full#supplementary-material
Abbreviations
AI, artificial intelligence; CT, control treatment; RT, rehabilitation robots; BCI, brain - computer interface; VR, virtual reality; RR, remote rehabilitation; RT + VR, rehabilitation robots + virtual reality; RCTs, randomized controlled trials; ARAT, action research arm test; NMA, network meta-analysis; ADL, activity of daily life; NDT, neurodevelopmental therapy; CIMT, constraint induced movement therapy; FES, functional electronic stimulation; EG, experimental group; CG, control group; FMA - UE, Fugl-Meyer assessment upper extremity; MBI, modified barthel Index; OT, occupational therapy; MTC, multiple treatment comparison; ITC, indirect treatment comparison.
References
1. Langhorne P, Bernhardt J, Kwakkel G. Stroke rehabilitation. Lancet. (2011) 377:1693–702. doi: 10.1016/S0140-6736(11)60325-5
2. Persson HC, Parziali M, Danielsson A, Sunnerhagen KS. Outcome and upper extremity function within 72 hours after first occasion of stroke in an unselected population at a stroke unit. A part of the SALGOT study. BMC Neurol. (2012) 12:162. doi: 10.1186/1471-2377-12-162
3. Broeks JG, Lankhorst GJ, Rumping K, Prevo AJ. The long-term outcome of arm function after stroke: Results of a follow-up study. Disabil Rehabil. (1999) 21:357–64. doi: 10.1080/096382899297459
4. Nakayama H, Jørgensen HS, Raaschou HO, Olsen TS. Recovery of upper extremity function in stroke patients: the copenhagen stroke study. Arch Phys Med Rehabil. (1994) 75:394–8. doi: 10.1016/0003-9993(94)90161-9
5. Feigin VL, Lawes CM, Bennett DA, Anderson CS. Stroke epidemiology: A review of population-based studies of incidence, prevalence, and case-fatality in the late 20th century. Lancet Neurol. (2003) 2:43–53. doi: 10.1016/S1474-4422(03)00266-7
6. Corbetta D, Sirtori V, Castellini G, Moja L, Gatti R. Constraint-induced movement therapy for upper extremities in people with stroke. Cochrane Database Syst Rev. (2015) 2015:D4433. doi: 10.1002/14651858.CD004433.pub3
7. Wiklund LM, Uvebrant P. Hemiplegic cerebral palsy: Correlation between CT morphology and clinical findings. Dev Med Child Neurol. (1991) 33:512–23. doi: 10.1111/j.1469-8749.1991.tb14916.x
8. Pagliano E, Andreucci E, Bono R, Semorile C, Brollo L, Fedrizzi E. Evolution of upper limb function in children with congenital hemiplegia. Neurol Sci. (2001) 22:371–5. doi: 10.1007/s100720100067
9. Chin LF, Hayward KS, Brauer S. Upper limb use differs among people with varied upper limb impairment levels early post-stroke: a single-site, cross-sectional, observational study. Top Stroke Rehabil. (2020) 27:224–35. doi: 10.1080/10749357.2019.1690796
10. Hung YC, Charles J, Gordon AM. Bimanual coordination during a goal-directed task in children with hemiplegic cerebral palsy. Dev Med Child Neurol. (2004) 46:746–53. doi: 10.1111/j.1469-8749.2004.tb00994.x
11. Steenbergen B, Van J. Control of prehension in hemiparetic cerebral palsy: Similarities and differences between the IPSI- and contra-lesional sides of the body. Dev Med Child Neurol. (2004) 46:325–32. doi: 10.1111/j.1469-8749.2004.tb00493.x
12. Duff SV, Gordon AM. Learning of grasp control in children with hemiplegic cerebral palsy. Dev Med Child Neurol. (2003) 45:746–57. doi: 10.1111/j.1469-8749.2003.tb00884.x
13. Gordon AM, Lewis SR, Eliasson AC, Duff SV. Object release under varying task constraints in children with hemiplegic cerebral palsy. Dev Med Child Neurol. (2003) 45:240–8. doi: 10.1111/j.1469-8749.2003.tb00338.x
14. Sköld A, Josephsson S, Eliasson AC. Performing bimanual activities: the experiences of young persons with hemiplegic cerebral palsy. Am J Occup Ther. (2004) 58:416–25. doi: 10.5014/ajot.58.4.416
15. Sakzewski L, Ziviani J, Boyd R. Systematic review and meta-analysis of therapeutic management of upper-limb dysfunction in children with congenital hemiplegia. Pediatrics. (2009) 123:e1111–22. doi: 10.1542/peds.2008-3335
16. Helgøy KV, Bonsaksen T, Røykenes K. Research-based education in undergraduate occupational therapy and physiotherapy education programmes: a scoping review. Bmc Med Educ. (2022) 22:358. doi: 10.1186/s12909-022-03354-2
17. Lewis KJ, Ross L, Coppieters MW, Vicenzino B, Schmid AB. Education, night splinting and exercise versus usual care on recovery and conversion to surgery for people awaiting carpal tunnel surgery: a protocol for a randomised controlled trial. BMJ Open. (2016) 6:e12053. doi: 10.1136/bmjopen-2016-012053
18. Zhu MH, Shi MF, Shen YP, Jin M, Gu XD, Fu JM. Effect of occupational therapy on the quality of life of stroke patients. Chin J Phys Med Rehabil. (2009) 31:124–6. doi: 10.3760/cma.j.issn.0254-1424.2009.02.019
19. Li T, Zhang F, Zhang HC Si XY, Liu YR. Effectiveness of the conductive educational approach added to rehabilitation training for stroke patients. Journal of Nursing Science. (2022) 37:1–4. doi: 10.3870/j.issn.1001-4152.2022.17.001
20. Chen KY, Liu XY Ji X, Zhang H, Li T. Research Progress on the Application of Artificial Intelligence inRehabilitation Medicine in China. JACTA ACADEMIAE MEDICINAE SINICAE. (2021) 43:773–84. doi: 10.3881/j.issn.1000-503X.13926
21. Liu R. Application progress of artificial intelligence technology inrehabilitation and chronic disease management and auxiliary diagnosis. China Medical Engineering. (2021) 29:53–5. doi: 10.19338/j.issn.1672-2019.2021.07.013
22. Mazzoleni S, Duret C, Grosmaire AG, Battini E. Combining upper limb robotic rehabilitation with other therapeutic approaches after stroke: current status, rationale, and challenges. Biomed Res Int. (2017) 2017:8905637. doi: 10.1155/2017/8905637
23. Biasiucci A, Leeb R, Iturrate I, Perdikis S, Al-Khodairy A, Corbet T, et al. Brain-actuated functional electrical stimulation elicits lasting arm motor recovery after stroke. Nat Commun. (2018) 9:2421. doi: 10.1038/s41467-018-04673-z
24. Jia J. Central-peripheral-central closed-loop rehabilitation: a new concept of functional rehabilitation of the hand after stroke. Chin J Rehab Med. (2016) 31:1180–2. doi: 10.3969/j.issn.1001-1242.2016.11.001
25. Brennan DM, Mawson S, Brownsell S. Telerehabilitation: enabling the remote delivery of healthcare, rehabilitation, and self management. Stud Health Technol Inform. (2009) 145:231–48. doi: 10.3233/978-1-60750-018-6-231
26. Wu QY, Nie JY. Application of an intelligent motor feedback training system in the training of hand function and activities of daily living in stroke patients with hemiplegia. Chin J Rehab Med. (2012) 27:167–9. doi: 10.3969/j.issn.1001-1242.2012.02.018
27. Zheng CJ, Yang YW, Xia WG, Hua Q, Zhang YP, Chen YJ. Effect of virtual scenario-based interactive training combined with occupational therapy on the functional recovery of upper limbs in stroke patients. Chin J Phys Med Rehabil. (2014) 36:360–2. doi: 10.3760/cma.j.issn.0254-1424.2014.05.011
28. Ersoy C, Iyigun G. Boxing training in patients with stroke causes improvement of upper extremity, balance, and cognitive functions but should it be applied as virtual or real? Top Stroke Rehabil. (2021) 28:112–26. doi: 10.1080/10749357.2020.1783918
29. Rodríguez-Hernández M, Criado-Álvarez JJ, Corregidor-Sánchez AI, Martín-Conty JL, Mohedano-Moriano A, Polonio-López B. Effects of virtual reality-based therapy on quality of life of patients with subacute stroke: a three-month follow-up randomized controlled trial. Int J Environ Res Public Health. (2021) 18:2810. doi: 10.3390/ijerph18062810
30. Choi YH, Paik NJ. Mobile game-based virtual reality program for upper extremity stroke rehabilitation. J Vis Exp. (2018) 133:e56241. doi: 10.3791/56241
31. Camargo-Vargas D, Callejas-Cuervo M, Mazzoleni S. Brain-Computer interfaces systems for upper and lower limb rehabilitation: a systematic review. Sensors. (2021) 21:4312. doi: 10.3390/s21134312
32. Giansanti D. The social robot in rehabilitation and assistance: what is the future? Healthcare. (2021) 9:244. doi: 10.3390/healthcare9030244
33. Mills EJ, Bansback N, Ghement I, Thorlund K, Kelly S, Puhan MA, et al. Multiple treatment comparison meta-analyses: a step forward into complexity. Clin Epidemiol. (2011) 3:193–202. doi: 10.2147/CLEP.S16526
34. Mills EJ, Thorlund K, Ioannidis JP. Demystifying trial networks and network meta-analysis. BMJ. (2013) 346:f2914. doi: 10.1136/bmj.f2914
35. He XY, Ma QQ, Zhai YK. Cui FFg, Lu YY, Chen HT. A meta-analysis of the impact of tele-rehabilitation on the rehabilitation function of stroke patients. Chin J Rehab Med. (2020) 35:1466–71. doi: 10.3969/j.issn.1001-1242.2020.12.011
36. Li LL Yu Y, Jia YQ, Huang HQ. Effect of brain-computer interface on upper-limb motor function after stroke: a meta-analysis. Chin Rehab Theor Prac. (2021) 27:765–73. doi: 10.3969/j.issn.1006?9771.2021.07.005
37. Zhong C, He HC, Huang SS. Ma Rui, Wang JJ, He CQ. The effectiveness of virtual reality therapy in relieving upper limb hemiparesis after stroke: a meta-analysis of randomized and controlled trials. Chin J Phys Med Rehabil. (2019) 41:463–8. doi: 10.3760/cma.j.issn.0254-1424.2019.06.017
38. Zhu C E, Yu B, Chen W H, Zhang W. Systematic evaluation of robot-assisted training to improve upper extremity functional impairment in stroke patients. Chin J Rehab Med. (2016) 31:786–9. doi: 10.3969/j.issn.1001-1242.2016.07.015
39. Mehrholz J, Pollock A, Pohl M, Kugler J, Elsner B. Systematic review with network meta-analysis of randomized controlled trials of robotic-assisted arm training for improving activities of daily living and upper limb function after stroke. J Neuroeng Rehabil. (2020) 17:83. doi: 10.1186/s12984-020-00715-0
40. Page MJ, McKenzie JE, Bossuyt PM, Boutron I, Hoffmann TC, Mulrow CD, et al. The PRISMA 2020 statement: an updated guideline for reporting systematic reviews. BMJ. (2021) 372:n71. doi: 10.1136/bmj.n71
41. Welch V, Petticrew M, Petkovic J, Moher D, Waters E, White H, et al. Extending the PRISMA statement to equity-focused systematic reviews (PRISMA-E 2012): explanation and elaboration. Int J Equity Health. (2015) 14:92. doi: 10.1186/s12939-015-0219-2
42. Feng S, Tang M, Huang G, Wang J, He S, Liu D, et al. EMG biofeedback combined with rehabilitation training may be the best physical therapy for improving upper limb motor function and relieving pain in patients with the post-stroke shoulder-hand syndrome: a Bayesian network meta-analysis. Front Neurol. (2022) 13:1056156. doi: 10.3389/fneur.2022.1056156
43. Cho KH, Song WK. Effects of two different robot-assisted arm training on upper limb motor function and kinematics in chronic stroke survivors: a randomized controlled trial. Top Stroke Rehabil. (2021) 28:241–50. doi: 10.1080/10749357.2020.1804699
44. Savović J, Weeks L, Sterne JA, Turner L, Altman DG, Moher D, et al. Evaluation of the Cochrane Collaboration's tool for assessing the risk of bias in randomized trials: focus groups, online survey, proposed recommendations and their implementation. Syst Rev. (2014) 3:37. doi: 10.1186/2046-4053-3-37
45. Sterne J, Savović J, Page MJ, Elbers RG, Blencowe NS, Boutron I, et al. RoB 2: a revised tool for assessing risk of bias in randomised trials. BMJ. (2019) 366:l4898. doi: 10.1136/bmj.l4898
46. Salanti G, Ades AE, Ioannidis JP. Graphical methods and numerical summaries for presenting results from multiple-treatment meta-analysis: AN overview and tutorial. J Clin Epidemiol. (2011) 64:163–71. doi: 10.1016/j.jclinepi.2010.03.016
47. Chaimani A, Salanti G. Visualizing assumptions and results in network meta-analysis: the network graphs package. Stata J. (2015) 15:905–50. doi: 10.1177/1536867X1501500402
48. White IR, Barrett JK, Jackson D, Higgins JP. Consistency and inconsistency in network meta-analysis: model estimation using multivariate meta-regression. Res Synth Methods. (2012) 3:111–25. doi: 10.1002/jrsm.1045
49. Kiefer C, Sturtz S, Bender R. Indirect comparisons and network meta-analyses. Dtsch Arztebl Int. (2015) 112:803–8. doi: 10.3238/arztebl.2015.0803
50. Dias S, Welton NJ, Caldwell DM, Ades AE. Checking consistency in mixed treatment comparison meta-analysis. Stat Med. (2010) 29:932–44. doi: 10.1002/sim.3767
51. Su LL, Fang XY, Lin L, Li HY. Effects of upper limb robot-assisted training on cognition and upper limb motor function for subacute stroke patients. Chin J Rehab Theor Prac. (2022) 28:508–14. doi: 10.3969/j.issn.1006-9771.2022.05.003
52. Taravati S, Capaci K, Uzumcugil H, Tanigor G. Evaluation of an upper limb robotic rehabilitation program on motor functions, quality of life, cognition, and emotional status in patients with stroke: a randomized controlled study. Neurol Sci. (2022) 43:1177–88. doi: 10.1007/s10072-021-05431-8
53. Sale P, Franceschini M, Mazzoleni S, Palma E, Agosti M, Posteraro F. Effects of upper limb robot-assisted therapy on motor recovery in subacute stroke patients. J Neuroeng Rehabil. (2014) 11:104. doi: 10.1186/1743-0003-11-104
54. He Y, Zhang T. Effect of robot-assisted training on upper-limb function for stroke patients. Chin J Rehab Theor Prac. (2021) 27:797–801. doi: 10.3969/j.issn.1006-9771.2021.07.010
55. Burgar CG, Lum PS, Scremin AM, Garber SL, Van der Loos HF, Kenney D, et al. Robot-assisted upper-limb therapy in acute rehabilitation setting following stroke: department of veterans affairs multisite clinical trial. J Rehabil Res Dev. (2011) 48:445–58. doi: 10.1682/JRRD.2010.04.0062
56. de Araújo RC, Junior FL, Rocha DN, Sono TS, Pinotti M. Effects of intensive arm training with an electromechanical orthosis in chronic stroke patients: A preliminary study. Arch Phys Med Rehabil. (2011) 92:1746–53. doi: 10.1016/j.apmr.2011.05.021
57. Housman SJ, Scott KM, Reinkensmeyer DJ, A. randomized controlled trial of gravity-supported, computer-enhanced arm exercise for individuals with severe hemiparesis. Neurorehabil Neural Repair. (2009) 23:505–14. doi: 10.1177/1545968308331148
58. Hsieh YW, Wu CY, Liao WW, Lin KC, Wu KY, Lee CY. Effects of treatment intensity in upper limb robot-assisted therapy for chronic stroke: a pilot randomized controlled trial. Neurorehabil Neural Repair. (2011) 25:503–11. doi: 10.1177/1545968310394871
59. Page SJ, Hill V, White S. Portable upper extremity robotics is as efficacious as upper extremity rehabilitative therapy: a randomized controlled pilot trial. Clin Rehabil. (2013) 27:494–503. doi: 10.1177/0269215512464795
60. Timmermans AA, Lemmens RJ, Monfrance M, Geers RP, Bakx W, Smeets RJ, et al. Effects of task-oriented robot training on arm function, activity, and quality of life in chronic stroke patients: a randomized controlled trial. J Neuroeng Rehabil. (2014) 11:1–12. doi: 10.1186/1743-0003-11-45
61. Budhota A, Chua K, Hussain A, Kager S, Cherpin A, Contu S, et al. Robotic assisted upper limb training post stroke: a randomized control trial using combinatory approach toward reducing workforce demands. Front Neurol. (2021) 12:622014. doi: 10.3389/fneur.2021.622014
62. Zhang HY, Wu FC Li JH, Liao ZP. Effect of upper limb rehabilitation robot assisted training on upper limb function of stroke survivors. Chin J Sports Med. (2019) 38:859–63. doi: 10.16038/j.1000-6710.2019.10.006
63. Sun CC, Wang CF, Ding XJ, Guo D, Han XM, Du JY. Effects of assistant training of upper-limb rehabilitation robot on upper-limb motor of hemiple functiongic stroke patients. Chin J Rehab Med. (2018) 33:1162–7. doi: 10.3969/j.issn.1001-1242.2018.10.006
64. Tomić TJ, Savić AM, Vidaković AS, Rodić SZ, Isaković MS, Rodríguez-de-Pablo C, et al. Arm Assist robotic system versus matched conventional therapy for poststroke upper limb rehabilitation: a randomized clinical trial. Biomed Res Int. (2017) 2017:7659893. doi: 10.1155/2017/7659893
65. Conroy SS, Wittenberg GF, Krebs HI, Zhan M, Bever CT, Whitall J. Robot-assisted arm training in chronic stroke: addition of transition-to-task practice. Neurorehabil Neural Repair. (2019) 33:751–61. doi: 10.1177/1545968319862558
66. Fan H, Wu YF, Dong XQ, Feng L. Robots for the rehabilitation of upper limb motor function after stroke. Chin J Phys Med Rehabil. (2016) 38:104–7. doi: 10.3760/cma.j.issn.0254-1424.2016.02.006
67. Lee KW, Kim SB, Lee JH, Lee SJ, Wan Yoo S. Effect of upper extremity robot-assisted exercise on spasticity in stroke patients. Ann Rehabil Med. (2016) 40:961–71. doi: 10.5535/arm.2016.40.6.961
68. Villafañe JH, Taveggia G, Galeri S, Bissolotti L, Mullè C, Imperio G, et al. Efficacy of short-term robot-assisted rehabilitation in patients with hand paralysis after stroke: a randomized clinical trial. Hand. (2018) 13:95–102. doi: 10.1177/1558944717692096
69. Zhang C, Liu Xn, Hou ZG, Peng L, Yang H, Peng L, et al. Effects of upper limb robot-assisted therapy on motor function and activities of daily living in patients with convalescent stroke. Chin J Rehab Theor Prac. (2016) 22:1365–70. doi: 10.3969/j.issn.1006-9771.2016.12.001
70. Zhang XF, Gao XM, Zhao N, Zhang L, Ge SP, Zhang YM, et al. Effect of upper limb rehabilitation robot training on the recovery of upper limb function in stroke patients with hemiplegia. Chin J Phys Med Rehabil. (2016) 38:180–2. doi: 10.3760/cma.j.issn.0254-1424.2016.03.005
71. He B, Zhang C, Liu X. Effects of upper limb robot-assisted therapy on motor recovery in patients with acute stroke. Chin J Rehab Theor Prac. (2016) 22:688–92. doi: 10.3969/j.issn.1006-9771.2016.06.014
72. Singh N, Saini M, Kumar N, Srivastava M, Mehndiratta A. Evidence of neuroplasticity with robotic hand exoskeleton for post-stroke rehabilitation: a randomized controlled trial. J Neuroeng Rehabil. (2021) 18:1–15. doi: 10.1186/s12984-021-00867-7
73. Jiang S, You H, Zhao W, Zhang M. Effects of short-term upper limb robot-assisted therapy on the rehabilitation of sub-acute stroke patients. Technol Health Care. (2021) 29:295–303. doi: 10.3233/THC-202127
74. Gandolfi M, Valè N, Dimitrova EK, Mazzoleni S, Battini E, Filippetti M, et al. Effectiveness of robot-assisted upper limb training on spasticity, function and muscle activity in chronic stroke patients treated with botulinum toxin: A randomized Single-Blinded controlled trial. Front Neurol. (2019) 10:41. doi: 10.3389/fneur.2019.00041
75. Dehem S, Gilliaux M, Stoquart G, Detrembleur C, Jacquemin G, Palumbo S, et al. Effectiveness of upper-limb robotic-assisted therapy in the early rehabilitation phase after stroke: a single-blind, randomised, controlled trial. Ann Phys Rehabil Med. (2019) 62:313–20. doi: 10.1016/j.rehab.2019.04.002
76. Carpinella I, Lencioni T, Bowman T, Bertoni R, Turolla A, Ferrarin M, et al. Effects of robot therapy on upper body kinematics and arm function in persons post stroke: a pilot randomized controlled trial. J Neuroeng Rehabil. (2020) 17:1–19. doi: 10.1186/s12984-020-0646-1
77. Xu Q, Li C, Pan Y, Li W, Jia T, Li Z, et al. Impact of smart force feedback rehabilitation robot training on upper limb motor function in the subacute stage of stroke. NeuroRehabilitation. (2020) 47:209–15. doi: 10.3233/NRE-203130
78. Huang Y, Lai WP, Qian Q, Hu X, Tam EW, Zheng Y. Translation of robot-assisted rehabilitation to clinical service: a comparison of the rehabilitation effectiveness of EMG-driven robot hand assisted upper limb training in practical clinical service and in clinical trial with laboratory configuration for chronic stroke. Biomed Eng Online. (2018) 17:1–17. doi: 10.1186/s12938-018-0516-2
79. Susanto EA, Tong RK, Ockenfeld C, Ho NS. Efficacy of robot-assisted fingers training in chronic stroke survivors: a pilot randomized-controlled trial. J Neuroeng Rehabil. (2015) 12:1–9. doi: 10.1186/s12984-015-0033-5
80. Wu CY, Yang CL, Chuang LL, Lin KC, Chen HC, Chen MD, et al. Effect of therapist-based versus robot-assisted bilateral arm training on motor control, functional performance, and quality of life after chronic stroke: a clinical trial. Phys Ther. (2012) 92:1006–16. doi: 10.2522/ptj.20110282
81. Abd El-Kafy EM, Alshehri MA, El-Fiky AA-R, Guermazi MA, Mahmoud HM. The effect of robot-mediated virtual reality gaming on upper limb spasticity poststroke: a randomized-controlled trial. Games Health J. (2022) 11:93–103. doi: 10.1089/g4h.2021.0197
82. Hu YL, Xiao YH, Hua YP, Lu AM, Lu HD, Huang Y, et al. Effect of virtual reality technology combined with upper limb rehabilitation robot on functional recovery of upper limb in stroke patients. J Integ Trad Chin Western Med. (2022) 32:537–9. doi: 10.3969/j.issn.1005-4561.2022.06.012
83. Chen M, Pangdong R, et al. Effect of robot combined with virtual reality technology on upper limb function of hemiplegia after stroke. Continu Med Educ China. (2021).
84. Wang Y, Zhu M. Effect of virtual reality combined with upper limb robot training system on upper limb function recovery in stroke. Modern Chinese Doctor. (2021).
85. Gueye T, Dedkova M, Rogalewicz V, Grunerova-Lippertova M, Angerova Y. Early post-stroke rehabilitation for upper limb motor function using virtual reality and exoskeleton: equally efficient in older patients. Neurol Neurochir Pol. (2021) 55:91–6. doi: 10.5603/PJNNS.a2020.0096
86. Rand D, Weingarden H, Weiss R, Yacoby A, Reif S, Malka R, et al. Self-training to improve UE function at the chronic stage post-stroke: a pilot randomized controlled trial. Disabil Rehabil. (2017) 39:1541–8. doi: 10.1080/09638288.2016.1239766
87. Wang LL, Zhang Y, Wang CF, Sun CC Li M, Liu XY. Clinical study of brain computer interface based electrical stimulation training in improving upper limb dysfunction of patients with stroke. Biomed Eng Clin Med. (2022) 26:163–8. doi: 10.13339/j.cnki.sglc.20220218.014
88. Lee S-H, Kim SS, Lee B-H. Action observation training and brain-computer interface controlled functional electrical stimulation enhance upper extremity performance and cortical activation in patients with stroke: a randomized controlled trial. Physiother Theory Pract. (2022) 38:1126–34. doi: 10.1080/09593985.2020.1831114
89. Ang KK, Guan C, Phua KS, Wang C, Zhou L, Tang KY, et al. Brain-computer interface-based robotic end effector system for wrist and hand rehabilitation: results of a three-armed randomized controlled trial for chronic stroke. Front Neuroeng. (2014) 7:30. doi: 10.3389/fneng.2014.00030
90. Xu Y, Yun J, Jia J, et al. Efficacy of a brain-computer interface combined with functional electrical stimulation training on upper limb function and cognition in elderly stroke patients. Chinese J Elderly Cardiovasc Cerebrovasc Dis. (2018) 20:988–90.
91. Liang SJ, Zhu Y L, Wang W N, Zhuang M, Xu D Y, Liu G P, et al. Application of brain-computer interface technology in the rehabilitation of upper limb dysfunction in stroke patients. Chin J Rehab Med. (2020) 35:185–8. doi: 10.3969/j.issn.1001-1242.2020.02.012
92. Li MF, Jia J, Liu Y. Research on cognitive mechanism of motor lmagerytased brain compvter interface rehabilitation training for stroke patients with severe upper limb paralysis. J Chengdu Med College. (2012) 7:519–23. doi: 10.3969/j.issn.1674-2257.2012.04.004
93. Xiang X, Zhu J, Sun Y, Sun F, Liu X. Clinical study on the treatment of upper extremity dysfunction in the recovery period of ischemic stroke with brain computer interface rehabilitation training system. Med Innov China. (2020) 17:154–8.
94. Ren H, Xie Z. Efficacy of BCI in the rehabilitation of upper limb motor function in patients with stroke. Pract Med China. (2020).
95. Chen S, Cao L, Shu X, Wang H, Ding L, Wang SH, et al. Longitudinal electroencephalography analysis in subacute stroke patients during intervention of Brain-Computer interface with exoskeleton feedback. Front Neurosci. (2020) 14:809. doi: 10.3389/fnins.2020.00809
96. Kim T, Kim S, Lee B. Effects of action observational training plus Brain-Computer Interface-Based functional electrical stimulation on paretic arm motor recovery in patient with stroke: a randomized controlled trial. Occup Ther Int. (2016) 23:39–47. doi: 10.1002/oti.1403
97. Mihara M, Hattori N, Hatakenaka M, Yagura H, Kawano T, Hino T, et al. Near-infrared spectroscopy-mediated neurofeedback enhances efficacy of motor imagery-based training in poststroke victims: a pilot study. Stroke. (2013) 44:1091–8. doi: 10.1161/STROKEAHA.111.674507
98. Wu H, Zhang Y, et al. Effect of task-oriented training based on telerehabilitation platform on motor function and activities of daily living in patients discharged after stroke. Chinese J Phys Med Rehabil. (2022) 44:40–3.
99. Xue J, Zhu Z, et al. Application of stroke remote continuous home upper limb rehabilitation mode based on cloud platform in upper limb function exercise in stroke patients with hemiplegia. Famous Doctors. (2020).
100. Wang R, Zhang Y, et al. Effect of telerehabilitation system on upper limb function in patients discharged with stroke. Chinas Rehabil. (2020) 35:522–5.
101. Wang F. Comparative study on the effect of remote video home rehabilitation guidance and routine home rehabilitation guidance for stroke patients. Shanxi Med Magaz. (2018) 47:1553–4.
102. Gao X, Xia Y, et al. Efficacy of telerehabilitation model based on video interaction in hemiplegia patients with stroke. Continu Med Educ China. (2017) 9:211–3.
103. Chen J, Jin W, Dong WS, Jin Y, Qiao FL, Zhou YF, et al. Effects of home-based telesupervising rehabilitation on physical function for stroke survivors with hemiplegia: a randomized controlled trial. Am J Phys Med Rehabil. (2017) 96:152–60. doi: 10.1097/PHM.0000000000000559
104. Chen J. Effect of remote home rehabilitation on motor and cognitive and balance abilities in stroke hemiplegia. Chinese J Phys Med Rehabil. (2016) 38:909–11.
105. Maeno R, Fujita C, Iwatsuki H. Effect of remote home rehabilitation care on the rehabilitation of hemiplegia patients with stroke. Tianjin Nurs. (2015) 23:393–4.
106. Lin K-H, Chen C-H, Chen Y-Y, Huang W-T, Lai J-S, Yu S-M, et al. Bidirectional and multi-user telerehabilitation system: clinical effect on balance, functional activity, and satisfaction in patients with chronic stroke living in long-term care facilities. Sensors. (2014) 14:12451–66. doi: 10.3390/s140712451
107. Chaiyawat P, Kulkantrakorn K. Randomized controlled trial of home rehabilitation for patients with ischemic stroke: impact upon disability and elderly depression. Psychogeriatrics. (2012) 12:193–9. doi: 10.1111/j.1479-8301.2012.00412.x
108. Redzuan NS, Engkasan JP, Mazlan M, Abdullah SJF. Effectiveness of a video-based therapy program at home after acute stroke: a randomized controlled trial. Arch Phys Med Rehabil. (2012) 93:2177–83. doi: 10.1016/j.apmr.2012.06.025
109. Piron L, Turolla A, Agostini M, Zucconi C, Cortese F, Zampolini M, et al. Exercises for paretic upper limb after stroke: a combined virtual-reality and telemedicine approach. J Rehabil Med. (2009) 41:1016–102. doi: 10.2340/16501977-0459
110. Li J, Wu L, Shang S, et al. Effect of remote home rehabilitation guidance on daily living activities ability and motor function in patients with cerebral infarction. Rehabil Theor Pract China. (2011) 17:887–8.
111. Kwon JS, Park MJ, Yoon IJ, Park SH. Effects of virtual reality on upper extremity function and activities of daily living performance in acute stroke: a double-blind randomized clinical trial. Neuro Rehabilitation. (2012) 31:379–85. doi: 10.3233/NRE-2012-00807
112. Chen J. Effect of VR-Assisted Upper Limb Robot Training on Upper Limb Function and Cognitive Function in Stroke Patients. Wuhan Institute of Sport (2020).
113. Jiang RR. Clinic Research on Robot-Assisted With Virtual Reality Technology for Upper Limb Motor Function and Activity Ability After Stroke. Guangzhou: Guangzhou Medical University (2017). doi: 10.7666/d.D01289658
114. Wei Y. Comparison of the efficacy between traditional rehabilitation and intelligent rehabilitation in upper limb stage Brunnstrom III patients. Disabil. Med China. (2020) 28:21–2.
115. Wang M. Evaluation of the application effect of the upper limb intelligent rehabilitation training system in the rehabilitation treatment of stroke hemiplegia patients. J Shanxi Health Vocat Coll. (2020).
116. Prange GB, Kottink AIR, Buurke JH, Eckhardt MMEM, van Keulen-Rouweler BJ, Ribbers GM, et al. The effect of arm support combined with rehabilitation games on upper-extremity function in subacute stroke: a randomized controlled trial. Neurorehabil Neural Repair. (2015) 29:174–82. doi: 10.1177/1545968314535985
117. Lee KW, Kim SB, Lee JH, Lee SJ, Wan Kim J. Effect of robot-assisted game training on upper extremity function in stroke patients. Ann Rehabil Med. (2017) 41:539–46. doi: 10.5535/arm.2017.41.4.539
118. McNulty PA, Thompson-Butel AG, Faux SG, Lin G, Katrak PH, Harris LR, et al. The efficacy of Wii-based movement therapy for upper limb rehabilitation in the chronic poststroke period: a randomized controlled trial. Int J Stroke. (2015) 10:1253–60. doi: 10.1111/ijs.12594
119. Norouzi-Gheidari N, Hernandez A, Archambault PS, Higgins J, Poissant L, Kairy D. Feasibility, safety and efficacy of a virtual reality exergame system to supplement upper extremity rehabilitation post-stroke: a pilot randomized clinical trial and proof of principle. Int J Environ Res Public Health. (2020) 17:113. doi: 10.3390/ijerph17010113
120. Lin C-H, Chou L-W, Luo H-J, Tsai P-Y, Lieu F-K, Chiang S-L, et al. Effects of computer-aided interlimb force coupling training on paretic hand and arm motor control following chronic stroke: a randomized controlled trial. PLoS ONE. (2015) 10:e0131048. doi: 10.1371/journal.pone.0131048
121. Keskin Y, Atci A, Urkmez B, et al. Efficacy of a video-based physical therapy and rehabilitation system in patients with post-stroke hemiplegia: A randomized, controlled, pilot study. Turk J Geriatr. (2020) 23. doi: 10.31086/tjgeri.2020.145
122. El-Kafy EMA, Alshehri MA, El-Fiky AA-R, Guermazi MA. The effect of virtual reality-based therapy on improving upper limb functions in individuals with stroke: a randomized control trial. Front Aging Neurosci. (2021) 13:731343. doi: 10.3389/fnagi.2021.731343
123. Choi Y-H, Ku J, Lim H, Kim YH, Paik N-J. Mobile game-based virtual reality rehabilitation program for upper limb dysfunction after ischemic stroke. Restor Neurol Neurosci. (2016) 34:455–63. doi: 10.3233/RNN-150626
124. Anwar N, Karimi H, Ahmad A, Mumtaz N, Saqulain G, Gilani SA, et al. novel virtual reality training strategy for poststroke patients: a randomized clinical trial. J Healthc Eng. (2021) 2021:1–6. doi: 10.1155/2021/6598726
125. Kim KH, Kim DH. Effect of virtual reality rehabilitation training on limb function and balance function in elderly patients with hemiplegia after stroke. Chinese J Gerontol. (2019) 39:5191–4.
126. Xiao X, Huang D, et al. Effect of VR training on upper limb motor function in subacute stroke patients. Chinese J Rehabil Med. (2019) 34:1049–53.
127. Bo M, Tian R, et al. Effect of short-term virtual reality rehabilitation training on upper limb function and activities of daily living in stroke hemiplegia patients. Chinese J Rehabil Med. (2017) 32:1288–91.
128. Kim J-H. Effect of virtual reality game training on functional recovery of hemiplegia upper limb in stroke patients. Chinese J Phys Med Rehabil. (2016) 38:401–5.
129. Tian R, Bai M, et al. Effect of virtual reality technology on the upper limb function and the ability of daily living activities in stroke patients. Rehabil Theor Pract China. (2017) 22:1370–1.
130. Lee M-M, Shin D-C, Song C-H. Canoe game-based virtual reality training to improve trunk postural stability, balance, and upper limb motor function in subacute stroke patients: a randomized controlled pilot study. J Phys Ther Sci. (2016) 28:2019–24. doi: 10.1589/jpts.28.2019
131. Kong KH, Loh YJ, Thia E, Chai A, Ng CY, Soh YM, et al. Efficacy of a virtual reality commercial gaming device in upper limb recovery after stroke: a randomized, controlled study. Top Stroke Rehabil. (2016) 23:333–40. doi: 10.1080/10749357.2016.1139796
132. Park M, Ko M-H, Oh S-W, Lee J-Y, Ham Y, Yi H, et al. Effects of virtual reality-based planar motion exercises on upper extremity function, range of motion, and health-related quality of life: a multicenter, single-blinded, randomized, controlled pilot study. J Neuroeng Rehabil. (2019) 16:1–13. doi: 10.1186/s12984-019-0595-8
133. Brunner I, Skouen JS, Hofstad H, Strand LI, Becker F, Sanders AM, et al. Virtual reality training for upper extremity in subacute stroke (VIRTUES): study protocol for a randomized controlled multicenter trial. BMC Neurol. (2014) 14:186. doi: 10.1186/s12883-014-0186-z
134. Choi JH, Han EY, Kim BR, Kim SM, Im SH, Lee SY, et al. Effectiveness of commercial gaming-based virtual reality movement therapy on functional recovery of upper extremity in subacute stroke patients. Ann Rehabil Med. (2014) 38:485–93. doi: 10.5535/arm.2014.38.4.485
135. Thielbar KO, Lord TJ, Fischer HC, Lazzaro EC, Barth KC, Stoykov ME, et al. Training finger individuation with a mechatronic-virtual reality system leads to improved fine motor control post-stroke. J Neuroeng Rehabil. (2014) 11:1–11. doi: 10.1186/1743-0003-11-171
136. Kiper P, Agostini M, Luque-Moreno C, Tonin P, Turolla A. Reinforced feedback in virtual environment for rehabilitation of upper extremity dysfunction after stroke: preliminary data from a randomized controlled trial. BioMed Res Int. (2014) 2014:752128. doi: 10.1155/2014/752128
137. Lee D, Lee M, Lee K, Song C. Asymmetric training using virtual reality reflection equipment and the enhancement of upper limb function in stroke patients: a randomized controlled trial. J Stroke Cerebrovasc Dis. (2014) 23:1319–26. doi: 10.1016/j.jstrokecerebrovasdis.2013.11.006
138. Crosbie JH, Lennon S, McGoldrick MC, McNeill MDJ, McDonough SM. Virtual reality in the rehabilitation of the arm after hemiplegic stroke: a randomized controlled pilot study. Clin Rehabil. (2012) 26:798–806. doi: 10.1177/0269215511434575
139. Yin CW, Sien NY, Ying LA, Chung SF, Tan MLD. Virtual reality for upper extremity rehabilitation in early stroke: a pilot randomized controlled trial. Clin Rehabil. (2014) 28:1107–14. doi: 10.1177/0269215514532851
140. Zhou T, Zhang C, et al. Application of mirror therapy combined with telerehabilitation in upper limb functional exercise in stroke hemiplegia patients. Qilu Nurs J. (2019) 25:129–31.
141. Ögün MN, Kurul R, Yasar MF, Turkoglu SA, Avci S, Yildiz N. Effect of Leap Motion-based 3D immersive virtual reality usage on upper extremity function in ischemic stroke patients. Arq Neuropsiquiatr. (2019) 77:681–8. doi: 10.1590/0004-282X20190129
142. Nijenhuis SM, Prange-Lasonder GB, Stienen AH, Rietman JS, Buurke JH. Effects of training with a passive hand orthosis and games at home in chronic stroke: a pilot randomised controlled trial. Clin Rehabil. (2017) 31:207–16. doi: 10.1177/0269215516629722
143. Wolf SL, Sahu K, Bay RC, Buchanan S, Reiss A, Linder S, et al. The HAAPI (Home Arm Assistance Progression Initiative) trial: a novel robotics delivery approach in stroke rehabilitation. Neurorehabil Neural Repair. (2015) 29:958–68. doi: 10.1177/1545968315575612
144. Chen L, Chen Y, Fu WB, Huang DF, Lo W. The effect of virtual reality on motor anticipation and hand function in patients with subacute stroke: a randomized trial on movement-related potential. Neural Plast. (2022) 2022:7399995. doi: 10.1155/2022/7399995
145. Qian Q, Hu X, Lai Q, Ng SC, Zheng Y, Poon W. Early stroke rehabilitation of the upper limb assisted with an electromyography-driven neuromuscular electrical stimulation-robotic arm. Front Neurol. (2017) 8:447. doi: 10.3389/fneur.2017.00447
146. Huijgen BCH, Vollenbroek-Hutten MMR, Zampolini M, Opisso E, Bernabeu M, Van Nieuwenhoven J, et al. Feasibility of a home-based telerehabilitation system compared to usual care: arm/hand function in patients with stroke, traumatic brain injury and multiple sclerosis. J Telemed Telecare. (2008) 14:249–56. doi: 10.1258/jtt.2008.080104
147. ZJ, He C, Guo F, Xiong CH, Huang XL. Exoskeleton-Assisted anthropomorphic movement training (EAMT) for post stroke upper limb rehabilitation: a pilot randomized controlled trial. Arch Phys Med Rehabil. (2021) 102:2074–82. doi: 10.1016/j.apmr.2021.06.001
148. Li RQ, Wan T, Zi MJ, Duan WH, He LY, Gao RR. Stable angina pectoris of coronary heart disease treated with different acupuncture and moxibustion therapies: a network Meta-analysis. Chin Acupunct Moxib. (2022) 42:1431–8. doi: 10.13703/j.0255-2930.20220513-0002
149. Ögün MN, Kurul R, Yaşar MF, Turkoglu SA, Avci S, Yildiz N. Effect of leap motion-based 3D immersive virtual reality usage on upper extremity function in ischemic stroke patients. Arq Neuropsiquiatr. (2019) 77:681–8. doi: 10.1590/0004-282x20190129
150. Chen WJ. Effect of Upper-Limb Robot Training Assisted by Virtual Reality on Upper Limb Function and Cognitive Function of Stroke Patients. Wuhan: Wuhan Sports Univeristy (2020). doi: 10.27384/d.cnki.gwhtc.2020.000226
151. Kiper P, Szczudlik A, Agostini M, Opara J, Nowobilski R, Ventura L, et al. Virtual reality for upper limb rehabilitation in subacute and chronic stroke: a randomized controlled trial. Arch Phys Med Rehabil. (2018) 99:834–42. doi: 10.1016/j.apmr.2018.01.023
152. Chien WT, Chong YY, Tse MK, Chien CW, Cheng HY. Robot-assisted therapy for upper-limb rehabilitation in subacute stroke patients: a systematic review and meta-analysis. Brain Behav. (2020) 10:e1742. doi: 10.1002/brb3.1742
153. Nomikos PA, Spence N, Alshehri MA. Test-retest reliability of physiotherapists using the action research arm test in chronic stroke. J Phys Ther Sci. (2018) 30:1271–7. doi: 10.1589/jpts.30.1271
154. Spence N, Rodrigues N, Nomikos PA, Yaseen KM, Alshehri MA. Inter-rater reliability of physiotherapists using the action research arm test in chronic stroke. J Musculoskelet Neuronal Interact. (2020) 20:480–7.
155. Mehrholz J, Hädrich A, Platz T, Kugler J, Pohl M. Electromechanical and robot-assisted arm training for improving generic activities of daily living, arm function, and arm muscle strength after stroke. Cochrane Database Syst Rev. (2012) 6: D6876. doi: 10.1002/14651858.CD006876.pub3
156. Rodgers H, Bosomworth H, Krebs HI, van Wijck F, Howel D, Wilson N, et al. Robot assisted training for the upper limb after stroke (RATULS): a multicentre randomised controlled trial. Lancet. (2019) 394:51–62. doi: 10.1016/S0140-6736(19)31055-4
157. Brunner I, Skouen JS, Hofstad H, Aßmus J, Becker F, Sanders AM, et al. Virtual reality training for upper extremity in subacute stroke (VIRTUES): a multicenter RCT. Neurology. (2017) 89:2413–21. doi: 10.1212/WNL.0000000000004744
158. Li X, Wang L, Miao S, Yue Z, Tang Z, Su L, et al. Sensorimotor Rhythm-Brain computer interface with Audio-Cue, motor observation and multisensory feedback for Upper-Limb stroke rehabilitation: a controlled study. Front Neurosci. (2022) 16:808830. doi: 10.3389/fnins.2022.808830
159. Frolov AA, Mokienko O, Lyukmanov R, Biryukova E, Kotov S, Turbina L, et al. Post-stroke rehabilitation training with a Motor-Imagery-Based Brain-Computer interface (BCI)-Controlled hand exoskeleton: a randomized controlled multicenter trial. Front Neurosci. (2017) 11:400. doi: 10.3389/fnins.2017.00400
Keywords: artificial intelligence rehabilitation, stroke, upper limb function, randomized controlled trials, network meta-analysis
Citation: Zhu Y, Wang C, Li J, Zeng L and Zhang P (2023) Effect of different modalities of artificial intelligence rehabilitation techniques on patients with upper limb dysfunction after stroke—A network meta-analysis of randomized controlled trials. Front. Neurol. 14:1125172. doi: 10.3389/fneur.2023.1125172
Received: 16 December 2022; Accepted: 21 March 2023;
Published: 17 April 2023.
Edited by:
Cheong-Meng Chong, University of Macau, ChinaCopyright © 2023 Zhu, Wang, Li, Zeng and Zhang. This is an open-access article distributed under the terms of the Creative Commons Attribution License (CC BY). The use, distribution or reproduction in other forums is permitted, provided the original author(s) and the copyright owner(s) are credited and that the original publication in this journal is cited, in accordance with accepted academic practice. No use, distribution or reproduction is permitted which does not comply with these terms.
*Correspondence: Peizhen Zhang, zhpzh@bsu.edu.cn
†These authors have contributed equally to this work and share first authorship