- 1Department of Cardiology, Smidt Heart Institute, Cedars-Sinai Medical Center, Los Angeles, CA, United States
- 2Department of Neurology and Medicine, Cedars-Sinai Medical Center, Los Angeles, CA, United States
- 3David Geffen School of Medicine at UCLA, Los Angeles, CA, United States
Background and objectives: Recognized as a potential risk factor for Alzheimer's disease and related dementias (ADRD), blood pressure variability (BPV) could be leveraged to facilitate identification of at-risk individuals at a population level. Granular BPV data are available during acute care hospitalization periods for potentially high-risk patients, but the incident ADRD risk association with BPV measured in this setting is unknown. Our objective was to evaluate the relation of BPV, measured during acute care hospitalization, and incidence of ADRD.
Methods: We retrospectively studied adults, without a prior ADRD diagnosis, who were admitted to a large quaternary care medical center in Southern California between January 1, 2013 and December 31, 2019. For all patients, determined BPV, calculated as variability independent of the mean (VIM), using blood pressure readings obtained as part of routine clinical care. We used multivariable Cox proportional hazards regression to examine the association between BP VIM during hospitalization and the development of incident dementia, determined by new ICD-9/10 coding or the new prescription of dementia medication, occurring at least 2 years after the index hospitalization.
Results: Of 81,892 adults hospitalized without a prior ADRD diagnosis, 2,442 (2.98%) went on to develop ADRD (2.6 to 5.2 years after hospitalization). In multivariable-adjusted Cox models, both systolic (HR 1.05, 95% CI 1.00–1.09) and diastolic (1.06, 1.02–1.10) VIM were associated with incident ADRD. In pre-specified stratified analyses, the VIM associations with incident ADRD were most pronounced in individuals over age 60 years and among those with renal disease or hypertension. Results were similar when repeated to include incident ADRD diagnoses made at least 1 or 3 years after index hospitalization.
Discussion: We found that measurements of BPV from acute care hospitalizations can be used to identify individuals at risk for developing a diagnosis of ADRD within approximately 5 years. Use of the readily accessible BPV measure may allow healthcare systems to risk stratify patients during periods of intense patient-provider interaction and, in turn, facilitate engagement in ADRD screening programs.
Introduction
The growing burden of Alzheimer's disease and Related Dementias (ADRD) has prompted renewed calls for improved prognostic tools to help identify at risk patients prior to the development of clinical disease, when interventions to mitigate or slow cognitive decline may be more efficacious (1). The risk of ADRD increases following acute care hospitalization, indicating that focusing efforts on risk stratification of hospitalized patients may identify individuals who are more likely to go on to develop cognitive impairment (2, 3). While multiple screening modalities including cognitive testing, neurologic imaging, and even novel biomarkers have been developed, the feasibility of deploying these at scale among all patients is limited (4–10).
Conversely, hypertension represents a well-recognized and easily accessible ADRD risk factor readily assessed at a population level (11). Unfortunately, due to acute illness, pain, and medications, mean blood pressure assessment during acute hospitalizations does not necessarily reflect either hypertensive status or the severity of hypertension in the outpatient setting (12). Beyond the degree of blood pressure elevation, however, blood pressure variability (BPV) has also been linked to ADRD risk, with a recent meta-analysis indicating that the relative association between BPV and ADRD may be stronger than that of mean blood pressure alone (13, 14). While numerous cohort studies have evaluated the association of intermediate and long-term BPV with ADRD, little is known regarding potential association with short-term, acute BPV during hospital admission and subsequent ADRD diagnoses (15–20). Given that readily available and granular BPV data can be collected and analyzed from a hospitalization, often involving patients with at least age-based risk for ADRD, we sought to determine whether BPV measurements generated in this particular clinical setting could be useful for estimating risk for subsequent incidence of ADRD.
Methods
Study design and data extraction
We identified all patients admitted to Cedars-Sinai Medical Center (CSMC) between 2013 and 2019; Cedars-Sinai is a large, quaternary care facility located in Southern California, with a catchment area that includes a diverse population of 1.8 million individuals. We extracted demographics (age, sex, race/ethnicity), smoking status, and clinical comorbidities (diabetes, coronary artery disease, transient ischemic attack, stroke, hypertension, depression, atrial fibrillation or flutter, and concussion) using International Classification of Diseases (ICD)-9 and ICD-10 codes (Supplementary Table 1) at the time of hospitalization during the study period. We then extracted all inpatient systolic and diastolic blood pressure measurements from the hospitalization and calculated variability independent of the mean (VIM), as described previously (21).
Variability independent of the mean calculation
Briefly, VIM is calculated first as the standard deviation of BP readings divided by the mean BP raised to the power of x, where x is obtained from fitting a non-linear regression model among the entire sample where standard deviation = a*meanx. This quantity is then multiplied by the sample mean BP raised to the power of x. Other methods of quantifying BPV such as standard deviation, coefficient of variation, and mean real variability are highly correlated with mean blood pressuring, limiting their utility in differentiating the effect of BPV from mean blood pressure. VIM was chosen for this study given its independence from mean blood pressure, allowing for decoupling of effects of mean blood pressure on BPV (22, 23).
Statistical analysis
Our primary outcome was new dementia diagnosis, which we defined as the presence of ICD-9 or ICD-10 codes for dementia or the initiation of dementia medication (Supplementary Table 1). Analytical models were constructed to account for key clinical variables prioritized within a conceptual framework linking BPV with ADRD risk (Supplementary Figure 1) that is based on previously published evidence (14, 16, 24). We used multivariable Cox proportional hazards regression to examine the association between BP VIM during hospitalization and the development of incident dementia during follow-up. Cox models were adjusted for age, sex, race/ethnicity, smoking status, hospital length of stay, ICU status during hospitalization, number of BP readings, mean systolic and diastolic BP, and all extracted clinical comorbidities.
Given that dementia diagnoses tend to occur late in the onset of the disease, we assumed that a patient's BP VIM during hospitalization could only be associated with a captured diagnosis of dementia that occurred at least 2 years after the end of the hospitalization (25, 26). Therefore, only hospitalizations with at least 2 years of event-free follow-up (blanking period) were included in the analysis. In sensitivity analyses, we altered the follow-up periods to either 1 year or 3 years after hospital discharge. Follow-up time was defined as time from discharge until dementia diagnosis, death, or latest recorded outpatient visit up to December 31, 2019. For patients with multiple qualifying hospitalizations, BP VIM and follow-up time were calculated using the most recent hospitalization. We excluded patients with no qualifying hospitalizations, no recorded outpatient visits, or who were aged <18 years at the time of index hospitalization. We conducted all statistical analyses using R (v4.1.1) and considered statistical significance as a two-tailed P-value < 0.05.
Standard protocol approvals, registrations, and patient consents
Study procedures were reviewed and approved by the Cedars-Sinai institutional review board (Study 00000603), with a waiver for informed consent.
Results
We identified a total of n = 81,892 hospitalized patients without prior ADRD meeting follow up criteria, with an average age of 55.9 ± 18.8 years at the time of admission, of whom 50,808 (62.0%) were female. The median hospital length of stay was 65.7 (43.3, 103.6) hours, with 7,372 (9.0%) of patients admitted to the intensive care unit during their hospitalization. The most common clinical comorbidity was hypertension (29.0%), followed by depression (12.1%), and coronary artery disease (11.5%). The mean systolic and diastolic blood pressures during hospital stays were 122.4 ± 14.3 mmHg and 68.4 ± 9.2 mmHg, respectively, with a median of 40 (16, 43) BP measurement per patient. A total of 2,442 (3.0%) individuals developed incident ADRD at least 2 years following hospital discharge (Table 1); follow up of the total cohort ranged from 2.6 to 5.2 years, with a mean follow up of 3.6 years.
In multivariable analysis, greater inpatient systolic (Hazards Ratio 1.05, 95% CI 1.00–1.09) and diastolic (1.06, 1.02–1.10) VIM were each associated with increased risk of incident dementia at least 2 years following hospitalization (Table 2). As expected, greater mean systolic BP was also associated with increased ADRD risk (1.01 per 1 mmHg increase in systolic BP, 1.01–1.01). In sex stratified analyses, only diastolic VIM among females remained significantly associated with incident ADRD (1.07, 1.02–1.13), though no differences in risk by sex was appreciated.
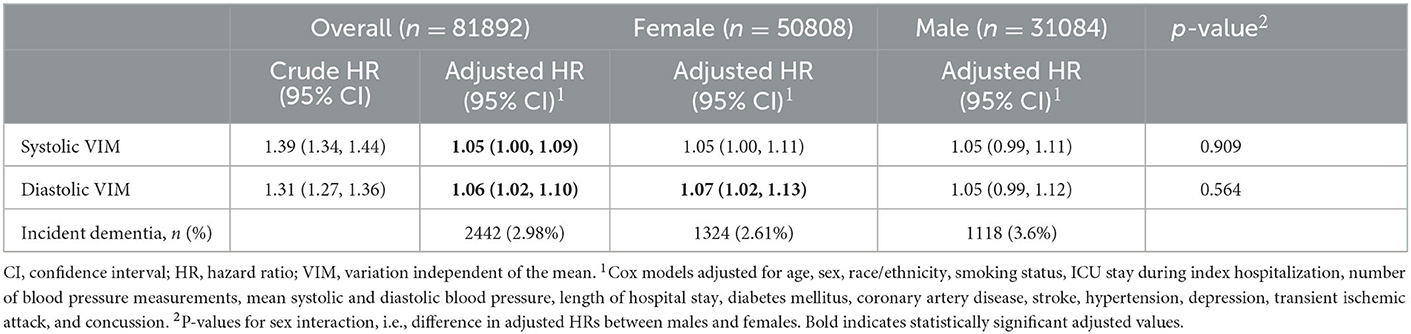
Table 2. Risk of incident ADRD diagnosis in the 2 years following an acute care hospitalization, overall and stratified by sex.
Stratification by clinical characteristics demonstrated significant associations between higher systolic and diastolic VIM among individuals over the age of 60 at the time of hospitalization, but not those ≤60 years of age. Greater systolic and diastolic VIM were also associated with ADRD among those with renal disease and those with hypertension, but not those with stroke or diabetes (Figure 1; Supplementary Table 2).
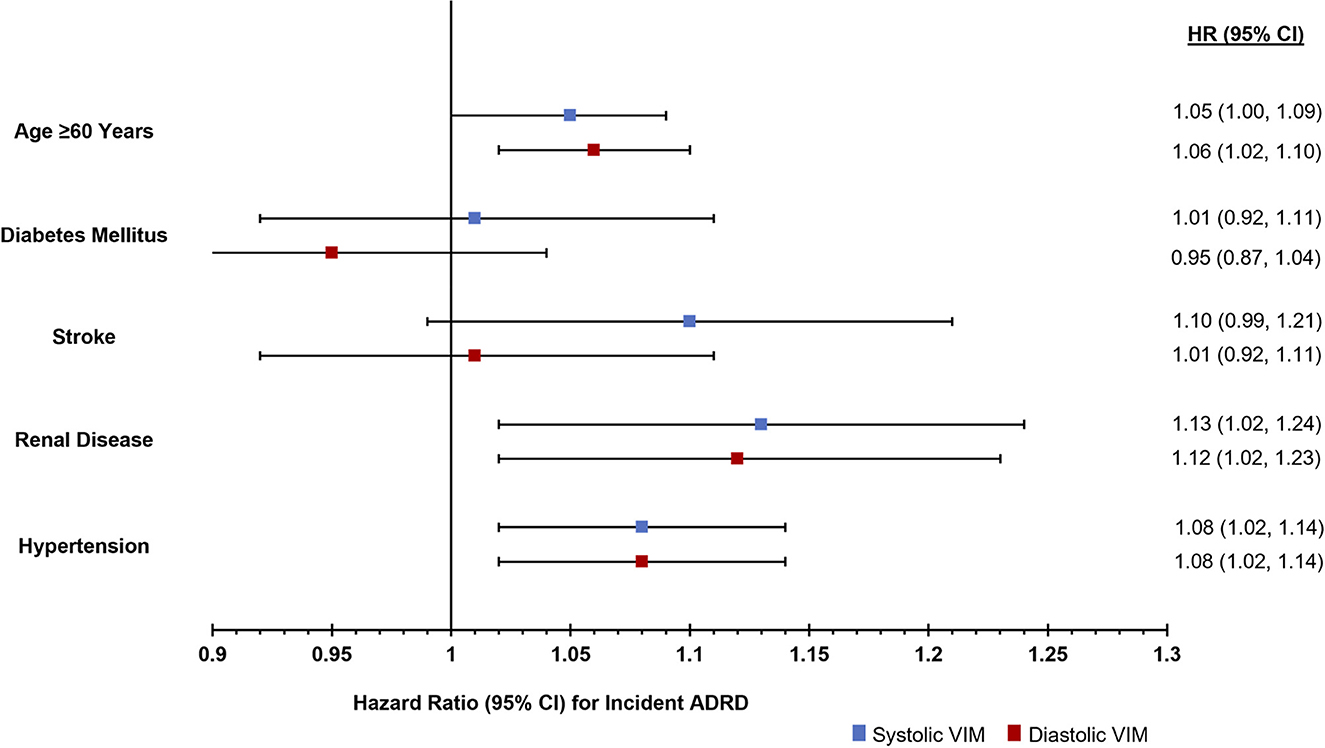
Figure 1. Risk of incident ADRD diagnosis in the 2 years following an acute care hospitalization stratified by comorbidities.
In sensitivity analyses, a total of 106,000 individuals had qualifying hospitalizations with at least 1 year of follow up data, of whom 3,437 (3.2%) developed incident dementia at least 1 year after hospitalization. Greater systolic (1.08, 1.04–1.11) and diastolic (1.06, 1.03–1.09) VIM were each associated with increased ADRD during the follow up period, a finding that was robust to stratification by sex, without differences appreciated between women and men. Conversely, a total of 62,615 individuals had qualifying hospitalizations with at least 3 years of follow up data, of whom 1,683 (2.7%) developed incident dementia at least 3 years after hospitalization. Greater diastolic (1.06, 1.03–1.09), but not systolic (1.03, 0.98–1.08) VIM was associated with increased ADRD during the follow up period. In sex stratified analyses, diastolic VIM remained significant only for females, but without a detectable difference between sexes (Supplementary Table 3).
Discussion
In a large cohort study of over 80,000 hospitalized individuals, we found that greater BPV, derived from clinically generated data during hospital admission, is associated with incident ADRD diagnosis at least 2 years after acute care hospitalization. This association held for both systolic and diastolic VIM, with robust diastolic VIM findings to variation in the duration of follow up. The utilization of routinely captured, readily accessible, and quantifiable data to assist in patient and population level ADRD risk assessment, represents a novel and effective approach to appropriately target further testing and treatment to high-risk individuals. In the future, we anticipate inpatient BPV serving as a risk marker that may prompt clinicians to pursue diagnostic ADRD testing.
Worldwide an estimated 46.8 million people are afflicted with dementia, with rates expected to triple by 2050 due to increasing life expectancy (27). Due in part to high failure rates of clinical trials aimed at Alzheimer's disease modification, in recent years there has been increased emphasis on risk stratification and risk reduction (28). Midlife vascular risk factors, including hypertension, have been associated with elevated brain amyloid deposition and an increased risk of developing dementia (29). While antihypertensive therapy especially in midlife represents a potentially viable intervention to reduce risk for dementia, there are inconsistencies on optimal blood pressure targets and uncertainties on the choice of antihypertensive agents (30). Visit-to-visit intra individual BPV in the outpatient setting has been associated with higher risk of dementia and cognitive impairment and may provide an alternate link to blood pressure and dementia risk (14). To our knowledge, there has not been a study that examined BPV in the acute hospital setting and the risk for dementia.
Our findings address two important gaps in current risk prediction methodologies. First, the use of routinely generated clinical data, easily accessible from the electronic health record (EHR), assists in reducing the burden of large scale ADRD screening volumes. By identifying patients at high-risk for incident or undiagnosed ADRD, screening can be targeted to an enriched population most likely to benefit. Other well recognized, easily accessible clinical variables indicative of increased ADRD risk such as advanced age and high blood pressure are exceedingly common, providing poor discrimination for truly high-risk individuals. Importantly, BP data obtained in cohort studies are typically captured using high-fidelity protocols that are not often used in clinical practice (31–34). Our results demonstrate a preserved signal of BPV associated ADRD risk in clinically generated data, including BP measures obtained using different techniques and devices as is the norm in practice, opening the possibility of using this metric in real-world patient care settings. Second, the use of data solely from an acute hospitalization creates an opportunity to provide risk assessment for individuals without care continuity in a single healthcare system. The disjointed nature of US healthcare systems may preclude any single organization's ability to use outpatient VIM to predict ADRD risk. Further, some patients are unable or choose not to obtain consistent care from a single system, hindering clinicians' ability to effectively track, identify and manage early signs of ADRD. Inpatient hospital stays offer a unique opportunity of high healthcare contact for patients during which otherwise unrecognized or untreated conditions can be addressed. High blood pressure variability may now help clinicians identify and screen patients for ADRD, either during hospitalization or through coordination of outpatient care. Sharing of positive screening results with patients may also spur greater engagement with the medical system, overcoming inertia that could otherwise delay diagnosis until later stages of disease (35).
In stratified analyses, we found that BPV's association with ADRD was particularly noted among those over the age of 60, as well as those with renal disease and a history of hypertension. These findings are not surprising given both the increased incidence of ADRD with advancing age, as well as the known associations between hypertension and both kidney disease and ADRD. Further linking these factors, the association between hypertension and ADRD is strongest for individuals in mid- to late-life (11). Screening efforts focused on patients with high BPV in this age range, particularly those with a history of hypertension or kidney disease may offer the most efficacious use of limited screening capacity. In the absence of any sex differences for systolic VIM, sex-stratified analyses suggested that diastolic VIM may be associated with ADRD in women and not in men. This finding could be related to females having smaller caliber arteries than males, even after adjusting for body size, (36) and thus a greater end-organ sensitivity to fluctuations in basal hemodynamic pressure; (37) additional studies are needed to further investigate and validate these findings.
The biological underpinnings of the association of BPV and ADRD remains unclear. Vascular dysfunction and damage represent pathophysiologic mechanisms of cognitive impairment which can occur acutely, as with stroke, or chronically, as with ADRD (38–40). Greater BPV has been linked to increased vascular dysfunction, providing a potential mechanism of BPV leading to future ADRD. Such a relationship would indicate that reducing BPV, through either pharmacologic or non-pharmacologic mechanisms, may result in reductions in vascular disease and subsequent ADRD diagnosis or disease progression. Conversely, autonomic dysfunction has long been a recognized sequela of ADRD, with patients suffering from orthostatic hypotension, heart rate variability, and constipation (41, 42). In this setting, BPV may represent a ‘canary in the coalmine' of early ADRD, signaling future cognitive dysfunction before neurologic effects become clinically relevant (43–45). In this situation, BPV may represent both an early warning sign for future ADRD, as well as a potential surrogate marker for efficacy of disease management.
Limitations
Several limitations of this study merit consideration. First, the retrospective nature of the analysis clearly prohibits causal conclusions. As detailed above, however, the identification of BPV and ADRD using real-world data, may still prove clinically useful, as the purpose if one of risk identification, rather than demonstrating causality. Further, the analysis relies on administrative coding for identification of the outcome and patient level comorbidities. Fortunately, these codes have been well studied in other analysis and demonstrated the ability to appropriately identify patients with the specified conditions (46–48). Along these lines, other recognized ADRD risk factors including family history of dementia, physical activity, and educational attainment could not be accurately extracted from the EHR, and as such, were not included in the model, potentially confounding our results (49, 50). Prescription of antihypertensive medications was also not included in our models as these medications are typically discontinued or otherwise adjusted during hospitalization. Again, given the goal of identifying a marker of ADRD risk using data that is readily available at a population level in real work settings, the results still point to high inpatient VIM as a potential mechanism for identifying high-risk patients at the point of care.
Conclusion
The derivation of BPV using clinically generated blood pressure measurements during acute care hospitalizations can be used to identify individuals at high-risk for the development of ADRD in the short to intermediate time horizon. The use of this marker may allow healthcare systems to risk stratify patients at a population level during a time of high patient-provider interaction to engage patients in ADRD screening programs.
Data availability statement
The datasets presented in this article are not readily available due to the sensitive nature of the data collected for this study. Requests to access the dataset from qualified researchers trained in protocols on the protection of human subjects may be sent to Cedars-Sinai Medical Center at YmlvZGF0YWNvcmVAY3Nocy5vcmc=.
Ethics statement
The studies involving human participants were reviewed and approved by the Cedars-Sinai Institutional Review Board. Written informed consent for participation was not required for this study in accordance with the national legislation and the institutional requirements.
Author contributions
Co-authors JE, PB, SC, and ZT were responsible for the acquisition of data. JE, SC, and ZT were responsible for the study concept/design. JE, MD, MW, SC, and ZT were responsible for the analysis or interpretation of data. All authors contributed to the article and approved the submitted version.
Funding
This work was supported in part by Cedars-Sinai Medical Center, the Erika J Glazer Family Foundation, and NIH grants R01-HL131532 and K23-HL153888. No funders had a role in the design/conduct of the study; collection, management, analysis, and interpretation of the data; preparation, review, or approval of the manuscript; and decision to submit the manuscript for publication.
Conflict of interest
The authors declare that the research was conducted in the absence of any commercial or financial relationships that could be construed as a potential conflict of interest.
Publisher's note
All claims expressed in this article are solely those of the authors and do not necessarily represent those of their affiliated organizations, or those of the publisher, the editors and the reviewers. Any product that may be evaluated in this article, or claim that may be made by its manufacturer, is not guaranteed or endorsed by the publisher.
Supplementary material
The Supplementary Material for this article can be found online at: https://www.frontiersin.org/articles/10.3389/fneur.2023.1085885/full#supplementary-material
References
1. Martin S, Kelly S, Khan A, Cullum S, Dening T, Rait G, et al. Attitudes and preferences towards screening for dementia: a systematic review of the literature. BMC Geriatr. (2015) 15:66. doi: 10.1186/s12877-015-0064-6
2. Sipilä PN, Lindbohm JV, Singh-Manoux A, Shipley MJ, Kiiskinen T, Havulinna AS, et al. Long-term risk of dementia following hospitalization due to physical diseases: a multicohort study. Alzheimer's Dementia. (2020) 16:1686–95. doi: 10.1002/alz.12167
3. Guerra C, Linde-Zwirble WT, Wunsch H. Risk factors for dementia after critical illness in elderly medicare beneficiaries. Critic Care. (2012) 16:R233. doi: 10.1186/cc11901
4. Tsoi KKF, Chan JYC, Hirai HW, Wong SYS, Kwok TCY. Cognitive tests to detect dementia: a systematic review and meta-analysis. JAMA Intern Med. (2015) 175:1450–8. doi: 10.1001/jamainternmed.2015.2152
5. Burhan AM, Bartha R, Bocti C, Borrie M, Laforce R, Rosa-Neto P, et al. Role of emerging neuroimaging modalities in patients with cognitive impairment: a review from the canadian consensus conference on the diagnosis and treatment of dementia 2012. Alzheimer's Res Ther. (2013) 5:S4. doi: 10.1186/alzrt200
6. McMillan CT, Avants BB, Cook P, Ungar L, Trojanowski JQ, Grossman M. The power of neuroimaging biomarkers for screening frontotemporal dementia. Hum Brain Mapp. (2014) 35:4827–40. doi: 10.1002/hbm.22515
7. Kim Y, Kim J, Son M, Lee J, Yeo I, Choi KY, et al. Plasma protein biomarker model for screening Alzheimer disease using multiple reaction monitoring-mass spectrometry. Sci Rep. (2022) 12:1282. doi: 10.1038/s41598-022-05384-8
8. Gunes S, Aizawa Y, Sugashi T, Sugimoto M, Rodrigues PP. Biomarkers for Alzheimer's disease in the current state: a narrative review. Int J Mol Sci. (2022) 23:4962. doi: 10.3390/ijms23094962
9. Chambers LW, Sivananthan S, Brayne C. Is dementia screening of apparently healthy individuals justified? Adv Prev Med. (2017) 2017:9708413–9708413. doi: 10.1155/2017/9708413
10. Boustani M, Callahan CM, Unverzagt FW, Austrom MG, Perkins AJ, Fultz BA, et al. Implementing a screening and diagnosis program for dementia in primary care. J Gen Intern Med. (2005) 20:572–7. doi: 10.1007/s11606-005-0103-7
11. Walker KA, Sharrett AR, Wu A, Schneider AL, Albert M, Lutsey PL, et al. Association of midlife to late-life blood pressure patterns with incident dementia. JAMA. (2019) 322:535–45. doi: 10.1001/jama.2019.10575
12. Brook RD, Weder AB, Rajagopalan S. Environmental hypertensionology the effects of environmental factors on blood pressure in clinical practice and research. J Clin Hypertens. (2011) 13:836–42. doi: 10.1111/j.1751-7176.2011.00543.x
13. Kim Y, Lim JS, Oh MS Yu KH, Lee JS, Park JH, et al. Blood pressure variability is related to faster cognitive decline in ischemic stroke patients: PICASSO subanalysis. Sci Rep. (2021) 11:5049. doi: 10.1038/s41598-021-83945-z
14. De Heus RA, Tzourio C, Lee EJ, Opozda M, Vincent AD, Anstey KJ, et al. Association between blood pressure variability with dementia and cognitive impairment: a systematic review and meta-analysis. Hypertension. (2021) 78:1478–89. doi: 10.1161/HYPERTENSIONAHA.121.17797
15. Yoo JE, Shin DW, Han K, Kim D, Lee SP, Jeong SM, et al. Blood pressure variability and the risk of dementia. Hypertension. (2020) 75:982–90. doi: 10.1161/HYPERTENSIONAHA.119.14033
16. Ma Y, Tully PJ, Hofman A, Tzourio C. Blood pressure variability and dementia: a state-of-the-art review. Am J Hypertens. (2020) 33:1059–66. doi: 10.1093/ajh/hpaa119
17. Alpérovitch A, Blachier M, Soumaré A, Ritchie K, Dartigues JF, Richard-Harston S, et al. Blood pressure variability and risk of dementia in an elderly cohort, the Three-City Study. Alzheimer's Dementia. (2014) 10:S330–7. doi: 10.1016/j.jalz.2013.05.1777
18. Ma Y, Wolters FJ, Chibnik LB, Licher S, Ikram MA, Hofman A, et al. Variation in blood pressure and long-term risk of dementia: a population-based cohort study. PLoS Med. (2019) 16:e1002933. doi: 10.1371/journal.pmed.1002933
19. Oishi E, Ohara T, Sakata S, Fukuhara M, Hata J, Yoshida D, et al. Day-to-day blood pressure variability and risk of dementia in a general japanese elderly population. Circulation. (2017) 136:516–25. doi: 10.1161/CIRCULATIONAHA.116.025667
20. van Middelaar T, van Dalen JW, van Gool WA, van den Born BJ, van Vught LA., Moll van Charante EP, et al. Visit-to-visit blood pressure variability and the risk of dementia in older people. J Alzheimer's Dis. (2018) 62:727–35. doi: 10.3233/JAD-170757
21. Ebinger JE, Driver M, Ouyang D, Botting P, Ji H, Rashid MA, et al. Variability independent of mean blood pressure as a real-world measure of cardiovascular risk. EClinicalMed. (2022) 48:101442. doi: 10.1016/j.eclinm.2022.101442
22. Nwabuo CC, Yano Y, Moreira HT, Appiah D, Vasconcellos HD, Aghaji QN, et al. Association between visit-to-visit blood pressure variability in early adulthood and myocardial structure and function in later life. JAMA Cardiol. (2020) 5:795–801. doi: 10.1001/jamacardio.2020.0799
23. Yano Y. Visit-to-visit blood pressure variability—what is the current challenge? Am J Hypertens. (2016) 30:112–4. doi: 10.1093/ajh/hpw124
24. Jia P, Lee HW, Chan JY, Tsoi KK, Yiu KK. The association between long term blood pressure variability with incident dementia and cognitive impairment: a systematic review and meta-analysis. Alzheimer's Dementia. (2021) 17:e053738. doi: 10.1002/alz.053738
25. Speechly CM, Bridges-Webb C, Passmore E. The pathway to dementia diagnosis. Med J Aust. (2008) 189:487–9. doi: 10.5694/j.1326-5377.2008.tb02140.x
26. Knopman D, Donohue JA, Gutterman EM. Patterns of care in the early stages of Alzheimer's disease: impediments to timely diagnosis. J Am Geriatr Soc. (2000) 48:300–4. doi: 10.1111/j.1532-5415.2000.tb02650.x
27. Beard JR, Officer A, De Carvalho IA, Sadana R, Pot AM, Michel JP, et al. The World report on ageing and health: a policy framework for healthy ageing. Lancet. (2016) 387:2145–54. doi: 10.1016/S0140-6736(15)00516-4
28. Crous-Bou M, Minguillón C, Gramunt N, Molinuevo JL. Alzheimer's disease prevention: from risk factors to early intervention. Alzheimer's Research & Therapy. (2017) 9:71. doi: 10.1186/s13195-017-0297-z
29. Gottesman RF, Schneider AL, Zhou Y, Coresh J, Green E, Gupta N, et al. Association between midlife vascular risk factors and estimated brain amyloid deposition. JAMA. (2017) 317:1443–50. doi: 10.1001/jama.2017.3090
30. Iadecola C, Gottesman RF. Neurovascular and cognitive dysfunction in hypertension. Circ Res. (2019) 124:1025–44. doi: 10.1161/CIRCRESAHA.118.313260
31. Drawz PE, Agarwal A, Dwyer JP, Horwitz E, Lash J, Lenoir K, et al. Concordance between blood pressure in the systolic blood pressure intervention trial and in routine clinical practice. JAMA Intern Med. (2020) 180:1655–63. doi: 10.1001/jamainternmed.2020.5028
32. Tang O, Juraschek SP, Appel LJ, Cooper LA, Charleston J, Boonyasai RT, et al. Comparison of automated clinical and research blood pressure measurements: Implications for clinical practice and trial design. J Clin Hypertens. (2018) 20:1676–82. doi: 10.1111/jch.13412
33. Kallioinen N, Hill A, Horswill MS, Ward HE, Watson MO. Sources of inaccuracy in the measurement of adult patients' resting blood pressure in clinical settings: a systematic review. J Hypertens. (2017) 35:421–41. doi: 10.1097/HJH.0000000000001197
34. Powers BJ, Olsen MK, Smith VA, Woolson RF, Bosworth HB, Oddone EZ. Measuring blood pressure for decision making and quality reporting: where and how many measures? Ann Intern Med. (2011) 154:781–8. doi: 10.7326/0003-4819-154-12-201106210-00005
35. Galvin JE, Tolea MI, Chrisphonte S. What older adults do with the results of dementia screening programs. PLoS ONE. (2020) 15:e0235534. doi: 10.1371/journal.pone.0235534
36. Hiteshi AK Li D, Gao Y, Chen A, Flores F, Mao SS, et al. Gender differences in coronary artery diameter are not related to body habitus or left ventricular mass. Clin Cardiol. (2014) 37:605–9. doi: 10.1002/clc.22310
37. Haidar MA, van Buchem MA, Sigurdsson S, Gotal JD, Gudnason V, Launer LJ, et al. Wave reflection at the origin of a first-generation branch artery and target organ protection. Hypertension. (2021) 77:1169–77. doi: 10.1161/HYPERTENSIONAHA.120.16696
38. Shabir O, Berwick J, Francis SE. Neurovascular dysfunction in vascular dementia, Alzheimer's and atherosclerosis. BMC Neurosci. (2018) 19:62. doi: 10.1186/s12868-018-0465-5
39. Kisler K, Nelson AR, Montagne A, Zlokovic BV. Cerebral blood flow regulation and neurovascular dysfunction in Alzheimer disease. Nat Rev Neurosci. (2017) 18:419–34. doi: 10.1038/nrn.2017.48
40. Faraco G, Iadecola C. Hypertension. Hypertension. (2013) 62:810–7. doi: 10.1161/HYPERTENSIONAHA.113.01063
41. Allan LM. Diagnosis and management of autonomic dysfunction in dementia syndromes. Curr Treat Options Neurol. (2019) 21:38–38. doi: 10.1007/s11940-019-0581-2
42. Weinstein G, Davis-Plourde K, Beiser AS, Seshadri S. Autonomic imbalance and risk of dementia and stroke: the framingham study. Stroke. (2021) 52:2068–76. doi: 10.1161/STROKEAHA.120.030601
43. De Pablo-Fernandez E, Tur C, Revesz T, Lees AJ, Holton JL, Warner TT. Association of autonomic dysfunction with disease progression and survival in parkinson disease. JAMA Neurol. (2017) 74:970–6. doi: 10.1001/jamaneurol.2017.1125
44. Goldstein DS, Sewell L, Sharabi Y. Autonomic dysfunction in PD: a window to early detection? J Neurol Sci. (2011) 310:118–22. doi: 10.1016/j.jns.2011.04.011
45. Tada M, Onodera O, Tada M, Ozawa T, Piao YS, Kakita A, et al. Early development of autonomic dysfunction may predict poor prognosis in patients with multiple system atrophy. Arch Neurol. (2007) 64:256–60. doi: 10.1001/archneur.64.2.256
46. McGuinness LA, Warren-Gash C, Moorhouse LR, Thomas SL. The validity of dementia diagnoses in routinely collected electronic health records in the United Kingdom: a systematic review. Pharmacoepidemiol Drug Saf. (2019) 28:244–55. doi: 10.1002/pds.4669
47. Wilkinson T, Schnier C, Ly A, Sudlow C. Validation of dementia coding in hospital admissions, death and primary care data. Int J Popul Data Sci. (2017) 1:100. doi: 10.23889/ijpds.v1i1.100
48. Wei MY, Luster JE, Chan C-L, Min L. Comprehensive review of ICD-9 code accuracies to measure multimorbidity in administrative data. BMC Health Serv Res. (2020) 20:489. doi: 10.1186/s12913-020-05207-4
49. Fayosse A, Nguyen DP, Dugravot A, Dumurgier J, Tabak AG, Kivimäki M, et al. Risk prediction models for dementia: role of age and cardiometabolic risk factors. BMC Med. (2020) 18:107. doi: 10.1186/s12916-020-01578-x
Keywords: dementia, blood pressure variability, hypertension, hospitalization, risk prediction
Citation: Ebinger JE, Driver MP, Botting P, Wang M, Cheng S and Tan ZS (2023) Association of blood pressure variability during acute care hospitalization and incident dementia. Front. Neurol. 14:1085885. doi: 10.3389/fneur.2023.1085885
Received: 31 October 2022; Accepted: 17 January 2023;
Published: 07 February 2023.
Edited by:
Peng Li, Harvard Medical School, United StatesReviewed by:
Junhong Zhou, Harvard Medical School, United StatesCraig S. Anderson, University of New South Wales, Australia
Copyright © 2023 Ebinger, Driver, Botting, Wang, Cheng and Tan. This is an open-access article distributed under the terms of the Creative Commons Attribution License (CC BY). The use, distribution or reproduction in other forums is permitted, provided the original author(s) and the copyright owner(s) are credited and that the original publication in this journal is cited, in accordance with accepted academic practice. No use, distribution or reproduction is permitted which does not comply with these terms.
*Correspondence: Joseph E. Ebinger, Y2RhLXJlc2VhcmNoQGNzaHMub3Jn