- 1Department of Radiology, The First Affiliated Hospital of Wenzhou Medical University, Wenzhou, China
- 2Department of Neurology, Xiangya Hospital, Central South University, Changsha, China
- 3Department of Neurosurgery, Xiangya Hospital, Central South University, Changsha, China
- 4Department of Radiology, Xiangya Hospital, Central South University, Changsha, China
- 5Clinical Research Center for Epileptic Disease of Hunan Province, Xiangya Hospital, Central South University, Changsha, China
- 6National Clinical Research Center for Geriatric Disorders, Xiangya Hospital, Central South University, Changsha, China
Background: Cerebellar functional alterations are common in patients with mesial temporal lobe epilepsy (MTLE), which contribute to cognitive decline. This study aimed to deepen our knowledge of cerebellar functional alterations in patients with MTLE.
Methods: In this study, participants were recruited from an ongoing prospective cohort of 13 patients with left TLE (LTLE), 17 patients with right TLE (RTLE), and 30 healthy controls (HCs). Functional magnetic resonance imaging data were collected during a Chinese verbal fluency task. Group independent component (IC) analysis (group ICA) was applied to segment the cerebellum into six functionally separated networks. Functional connectivity was compared among cerebellar networks, cerebellar activation maps, and the centrality parameters of cerebellar regions. For cerebellar functional profiles with significant differences, we calculated their correlation with clinical features and neuropsychological scores.
Result: Compared to HCs and patients with LTLE, patients with RTLE had higher cerebellar functional connectivity between the default mode network (DMN) and the oculomotor network and lower cerebellar functional connectivity from the frontoparietal network (FPN) to the dorsal attention network (DAN) (p < 0.05, false discovery rate- (FDR-) corrected). Cerebellar degree centrality (DC) of the right lobule III was significantly higher in patients with LTLE compared to HC and patients with RTLE (p < 0.05, FDR-corrected). Higher cerebellar functional connectivity between the DMN and the oculomotor network, as well as lower cerebellar degree centrality of the right lobule III, was correlated with worse information test performance.
Conclusion: Cerebellar functional profiles were altered in MTLE and correlated with long-term memory in patients.
1. Introduction
Cerebellar alterations are common in mesial temporal lobe epilepsy (MTLE), one of the most prevalent forms of focal epilepsy in adults. The cerebellum is a potential target for seizure control in patients with drug-resistant MTLE because it contributes to cognitive deficiency in MTLE (1–4). Previous studies focused primarily on cerebellar structural abnormalities in MTLE. Cerebellar volume decreased by 4–6.6% in patients with chronic MTLE (5), but not in those with newly diagnosed temporal lobe epilepsy (TLE) (6). Cerebellar volume decreased more with longer disease duration (6–9), higher seizure frequency (6, 10), and a higher total seizure burden (5, 6, 11). Three independent studies reported cerebellar hyperfusion during temporal lobe seizures (12–14). In addition, the cerebellar damage in patients with epilepsy was similar to that in patients with cerebral hypoxia (11). Therefore, cerebellar structural damage was considered to be an acquired abnormality caused by hypoxia, seizure discharges, and hyperfusion during uncontrolled seizures.
The cerebellum is essential for language and long-term memory retrieval, except for motor control (15). Activation in the cerebellum rose with increasing memory load (16, 17). Meanwhile, sequence processing, one of the language functions of the cerebellum, affects the word retrieval strategy during verbal fluency tasks (18–20). Phonemic and semantic verbal fluency tasks are important scales clinically applied for routine and presurgical evaluation of TLE to predict prognosis and postsurgical language outcomes (21–23). According to lesion studies, phonemic verbal fluency was largely attributed to the left frontal cortex and anterior temporal lobe, and semantic verbal fluency was correlated with the left posterior temporal cortex (24). Phonemic verbal fluency requires category switching, causes greater cognitive load, and is, therefore, more dependent on cerebellar function (19, 20). Same as the cerebrum, language function in the cerebellum is lateralized. In right-handed participants, the right posterolateral cerebellum, which is functionally connected to the left prefrontal cortex, supports phonemic processing and linguistic prediction (25, 26). During a phonemic verbal fluency task, the left cerebellum was activated in left-handed participants (25).
Previous studies detected a deviation in cerebellar function in patients with TLE using voxel- and seed-based approaches. During the attentional network test, activation in the cerebellum was reduced in patients with MTLE compared to healthy controls (HC) (27). Impaired functional connectivity between the right dentate nuclei and the left cerebral hemisphere was related to cognitive impairment in MTLE (2, 3). Graph theory analyses provided additional knowledge regarding the role of cerebellar regions in TLE. Centrality statistics represent the importance of a given node in the entire network. In MTLE, cerebellar nodes with higher functional centrality were reported, indicating an attempted compensatory process (28). While the abovementioned approaches unfold cerebellar function at the regional level, the cerebellum is organized as a functional network (29, 30). Group independent component (IC) analysis (group ICA) provides an ideal and robust approach for separating the cerebellum into functionally segregated networks. Phonemic verbal fluency tasks effectively mobilized the cerebellum and provided an ideal tool for studying cerebellar malfunction at the network level. In addition, the clinical application of the Chinese version of the phonemic verbal fluency tasks was delayed due to the linguistic difference between Chinese and Indo-European languages. The involvement of the cerebellum in Chinese verbal fluency remained unclear. Further studies on cerebellar abnormalities of functional networks would deepen our understanding of the pathogenesis of cognitive decline in MTLE.
Based on the Chinese character version of a verbal fluency task, a cross-sectional study aimed to investigate alterations in functional connectivity between cerebellar networks parcellated using group ICA. A comprehensive view of cerebellar functional alterations in MTLE in China was provided using voxel- and seed-based approaches.
2. Methods
2.1. Participants
A total of 30 consecutive participants with temporal lobe epilepsy and hippocampal sclerosis (HS) were selected from an ongoing prospective cohort (31, 32). All participants visited the outpatient department of Xiangya Hospital between 9 November 2018 and 9 January 2021. A total of 30 HC matched for sex, age, and educational level participated in this study. Sex, age, years of education, age of onset, disease duration, number of antiseizure medications (ASMs), and seizure frequency were extracted from the database. 3DT1, T2WI, and T2WI fluid-attenuated inversion recovery sequences were applied to all participants to identify HS and potential lesions. The diagnosis of HS follows a robust protocol (33): (1) Neuroimagers diagnose HS based on visible changes, including decreased hippocampal volume, increased temporal horn volume, gray–white matter boundary blurring, asymmetric hippocampus, loss of internal structure, and increased T2 signals (34); (2) Reduced hippocampal volume calculated with the online automatic segmentation tool Hipposeg (35); and (3) A disagreement between procedures one and two may be reconciled with a blind rater.
Mesial temporal lobe epilepsy was diagnosed and lateralized based on a comprehensive evaluation of semiology, clinical history, electroencephalography, and magnetic resonance imaging (MRI). All participants in our cohort are right-handed (31, 36). Exclusion criteria included: (1) Those who had a psychiatric or neurological disorder other than MTLE, (2) Those who had a cerebral or cerebellar lesion other than HS, (3) Those who were below 16 or over 65 years of age, (4) Those who were unable to endure or comprehend the procedure, (5) Those who had poor imaging quality, including excessive head motion or poor task effect, and (6) Those who received phenytoin treatment (37).
This study was approved by the Ethics Committee of the Xiangya Hospital of Central South University. Written informed consent was obtained from all participants.
2.2. Neuropsychological tests
All participants underwent (1) Montreal Cognitive Assessment (MoCA) (38) to test the overall cognitive function, (2) the Information subtest of the Chinese version of the Wechsler Adult Intelligence Scale (WAIS) as an evaluation for long-term memory (32), (3) Digit Span subsets of WAIS-RC (revised by China) for working memory (39), (4) the Digit Symbol Substitution subset of WAIS-RC to exam the processing speed (32), (5) the Block Design subset of WAIS-RC for perceptual organization, and (6) Verbal Fluency-Chinese Character, Verbal Fluency-Chinese Pinyin (31), and the Boston Naming Test (40) to test the language function.
2.3. MRI data acquisition and preprocessing
Magnetic resonance imaging data were collected at the MRI center of the Xiangya Hospital using a Siemens MAGNETOM Prisma 3.0T MR scanner and standard head coils. Structural images were collected using magnetization-prepared rapid acquisition and a gradient echo sequence (field of view, 233 mm; repetition time, 1 s; echo time, 37 ms; flip angle, 9°; 320 × 320 matrix). When participants performed a Chinese character verbal fluency task (31, 41), functional images were obtained with a gradient echo-planar T2-weighted sequence (field of view, 225 mm; repetition time, 1 s; echo time, 37 ms; flip angle, 52°; 90 × 90 matrix) when conducting the Chinese character version of a phonemic verbal fluency task (41).
The task was divided into five blocks, each containing a 30-s rest module and a 30-s task module. In the rest module, a crosshair fixation would be projected on a white background. Participants were instructed to rest while looking at the screen. In each task module, one Chinese character would be displayed on a white background. Participants were instructed to covertly generate words beginning with the given Chinese character.
Raw images were realigned, co-registered, segmented, normalized, and spatially smoothed (6 mm) with the default preprocessing pipeline (31) of Statistical Parametric Mapping 12 (https://www.fil.ion.ucl.ac.uk/spm/). Next, image data were processed using Toolbox CONN v.20.b (42) (http://www.nitrc.org/projects/conn) for further denoising. Head motion, outlying scan detection using an embedded functional outlier, the effect of modules, and signals in the white matter and cerebrospinal fluid were removed as confounders. A bandpass filter [0.009–0.10 Hz] was applied to remove the noise.
2.4. Group ICA and network connectivity analysis
Toolbox CONN v.20.b was used for group ICA and subsequent network connectivity analysis. According to a previous study, the cerebellum was segmented into six ICs at the group level (43). Then, group cerebellum ICs were reconstructed back to individual ICs. For each participant, the average blood oxygen level-dependent signal time series in each individual IC was extracted for network connectivity analysis. A generalized linear model (GLM) based on semi-partial correlation was applied to calculate individual-level functional connectivity (42). Individual connectivity values were processed using Fisher transformation (inverse hyperbolic tangent functions) and then compared between HC, left TLE (LTLE), and right TLE (RTLE) using analysis of covariance (ANCOVA) and post hoc pairwise comparisons, with sex, age, years of education, and MoCA as covariates of no interest. The significant threshold for network connectivity was p < 0.05, false discovery rate- (FDR-) corrected.
2.5. Voxel-based analysis
SPM 12 was used to perform a two-level voxel-based analysis of cerebellar activation maps. At the individual level, a task-dependent contrast map was calculated for each participant. At the second level, contrast maps were compared between groups via ANCOVA and post- hoc pairwise comparison, with sex, age, years of education, and MoCA as covariates of no interest. Clusters were considered significant at p < 0.05, FDR-corrected with an additional threshold of 10-voxel minimum cluster size.
2.6. Seed-based analysis
The whole brain was parcellated into 210 cortical regions (44), 36 subcortical regions (44), and 26 cerebellar regions (45). For each participant, a GLM based on a bivariate correlation was used to construct a 272 × 272 weighted matrix, which was then transformed into 36 binary matrices with connection density ranging from 5 to 40%, in steps of 1% (46). Under each density, betweenness centrality (BC) and degree centrality (DC) for 26 cerebellar regions were calculated. BC is the frequency that a given node is on the shortest path between all node pairs. DC is the number of suprathreshold connections linked to a particular node. Centralities were compared among the three groups by (1) area under the curve (AUC) across all densities and (2) a subsequent comparison at each density. AUC was calculated using R studio.
The bilateral cerebellar lobule and vermis were defined as seeds in a seed-to-voxel analysis. Functional connectivity between the three regions and whole-brain voxels was computed with CONN v.20.b, based on a GLM and semi-partial correlation. Seed-based functional connectivity was compared among the three groups with ANCOVA and post-hoc pairwise comparison, with sex, age, years of education, and MoCA as covariates. Clusters were considered significant at p < 0.05, FDR-corrected with an additional minimum cluster size threshold of 10 voxels.
2.7. Statistical analysis
IBM SPSS Statistics 23 (https://www.ibm.com/products/spss-statistics) was used for statistical analysis. The distribution of qualitative variables was assessed using the Shapiro–Wilko test. Variables without a normal distribution or homogeneity of variance were compared between the groups with nonparametric approaches and reported as median and interquartile ranges. Sex, age, years of education, and MoCA were compared among the three groups using the chi-square test or the Kruskal–Wallis H-test. ANCOVA or Quade nonparametric ANCOVA and post- hoc pairwise comparisons were used to compare neuropsychological test scores among the three groups, with sex, age, years of education, and MoCA controlled for confounders. Qualitative and categorical variables were compared between the two patient groups using the two-tailed two-sample t-test and Fisher's exact test, respectively.
For functional network connectivity and centrality metrics with a significant group difference, we calculated their partial correlation with age of onset, disease duration, seizure frequency, disease burden = disease duration*seizure frequency, number of ASMs, and neuropsychological scores, adjusting for sex, age, years of education, and MoCA.
A p-value of < 0.05, FDR-corrected, was considered significant.
3. Results
3.1. Demographic and clinical data
The three groups did not differ in age, sex, years of education, and MoCA scores. HC outperformed both patient groups in verbal fluency Pinyin (VFP) scores (p = 0.004 and 0.02, FDR-corrected for HC vs. LTLE and HC vs. RTLE, respectively). Patients with LTLE also had worse Boston Naming (BN) scores than HC and patients with RTLE (p = 0.004 and 0.03, FDR-corrected for LTLE vs. HC and LTLE vs. RTLE, respectively).
Patients with LTLE and RTLE did not differ in their clinical features (see the details in Table 1).
3.2. Group-ICA and network connectivity analysis
Cerebellar ICs are shown in Figure 1. Cerebellar IC 1 contained bilateral crus I and II, the lobules III–VI, and the vermis III–V. IC 2 and IC 6 had relatively symmetric regions because they included the left crus I and II and the right crus I and II, respectively. IC 6 also contained the left crus I, the bilateral lobules VII and VIII. IC 3 comprised the bilateral crus I and II, the lobules IX, and the vermis IX–X. IC 4 encompassed bilateral crus I and II, and the lobules VI–VIII. IC 5 consisted of the vermis IV–IX and the bilateral lobules VIII and IX.
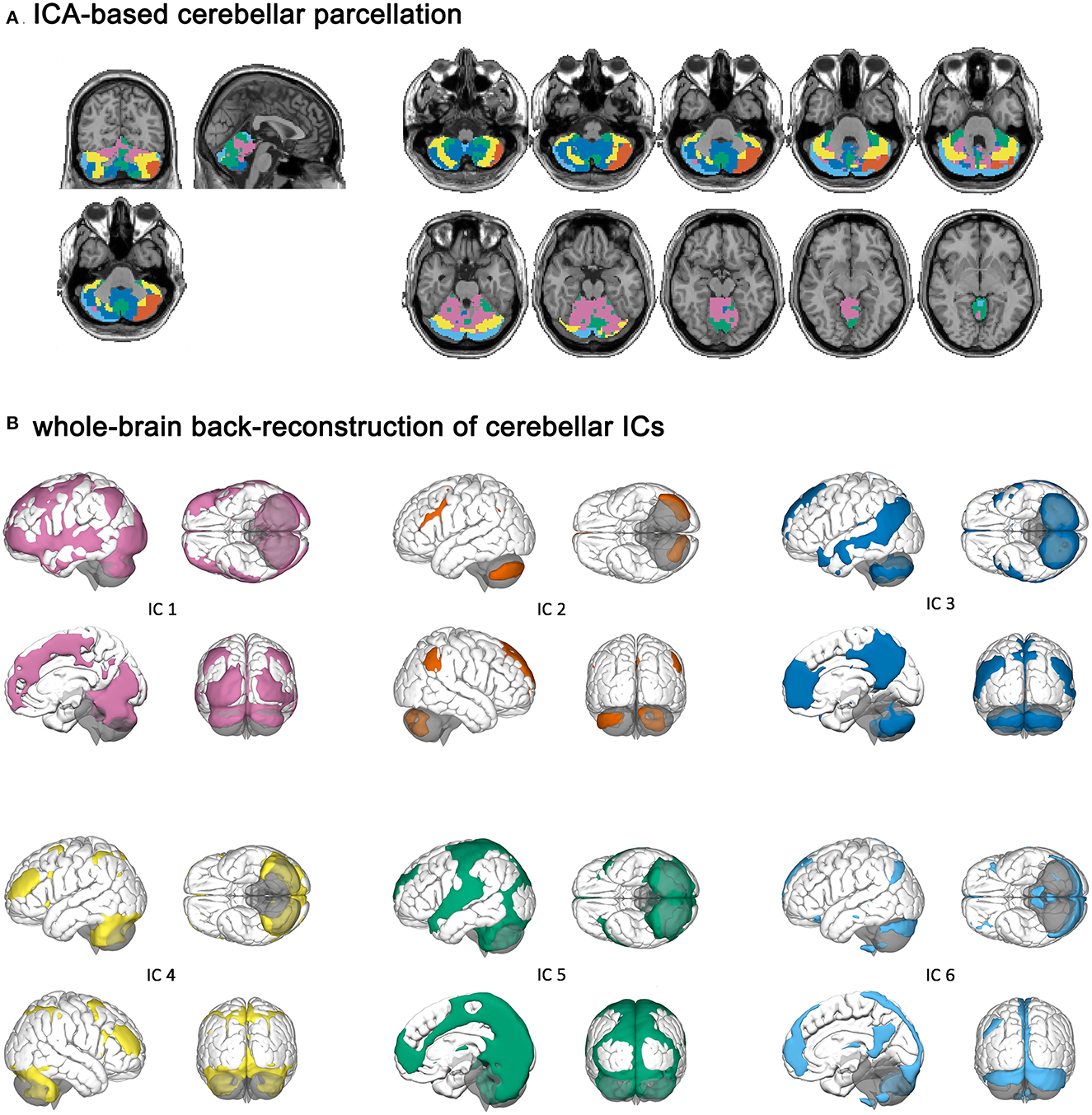
Figure 1. Cerebellar independent components and their cerebral parcellations. (A) ICA-based cerebellar parcellation. (B) Whole-brain back-reconstruction of cerebellar ICs.
Independent component (IC) 1 had a cerebral parcellation, which contained the visual region and frontoparietal network (FPN). IC 2 and IC 6 were connected to the left and right FPN, respectively. IC 6 had a cerebral parcellation, which contained regions similar to the default mode network (DMN). IC 3 had connectivity to the DMN, and IC 4 was connected to the dorsal attention network (DAN). IC 5 had a cerebral parcellation consisting of auditory and sensorimotor regions.
Functional network connectivity from IC 3 to IC 5 (p = 0.04, FDR-corrected), IC 5 to IC 3 (p = 0.04, FDR-corrected), and IC 6 to IC4 (p = 0.04, FDR-corrected) differed significantly among the three groups. Post-hoc comparisons showed that connectivity between IC 3 and IC 5 (Figures 2A, B) was enhanced and connectivity from IC 6 to IC 4 (Figure 2C) was impaired in patients with RTLE compared to HC and patients with LTLE.
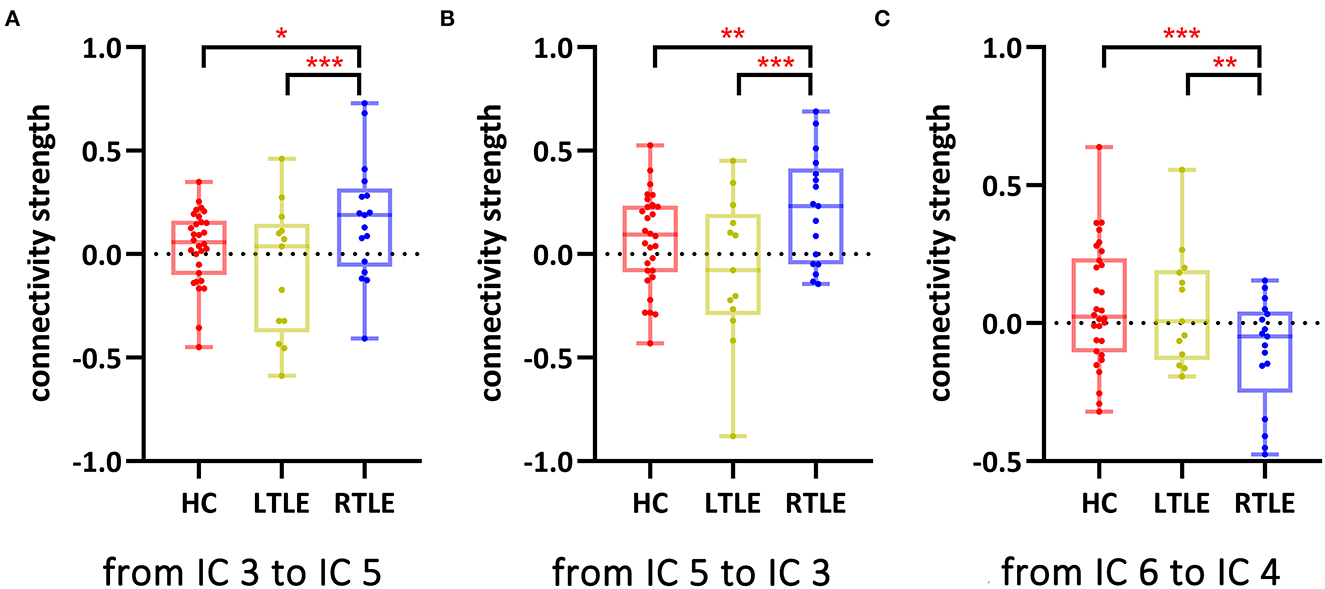
Figure 2. Network functional connectivity with a significant group difference among the three groups. *p < 0.05, FDR-corrected; **p < 0.005, FDR-corrected; ***p < 0.001, FDR-corrected. (A) Network functional connectivity from IC 3 to IC 5; (B) Network functional connectivity from IC 5 to IC 3; and (C) Network functional connectivity from IC 6 to IC 4. HC, healthy controls; IC, independent component; LTLE, left temporal lobe epilepsy; RTLE, right temporal lobe epilepsy.
3.3. Voxel-based analysis
Cerebellar activation maps did not differ among the three groups.
3.4. Seed-based analysis
On the overall scale (Figure 3, boxplot), DC of the right lobule III differed significantly among the three groups (p = 0.05, FDR-corrected), as it was elevated in LTLE compared to HCs (p = 0.002, FDR-corrected) and RLTE (p = 0.03, FDR-corrected). DC of the right lobule III was higher in LTLE than in HC at all densities (p < 0.05, FDR-corrected). When the connectivity density was <32%, LTLE also had a higher DC in the right lobule III than RTLE (p < 0.05, FDR-corrected; Figure 3, line chart). There was a trend (p < 0.05, FDR uncorrected) for the group difference of DC of bilateral lobule X, vermis X, and the BC of the right lobule III, the right lobule X, and vermis X.
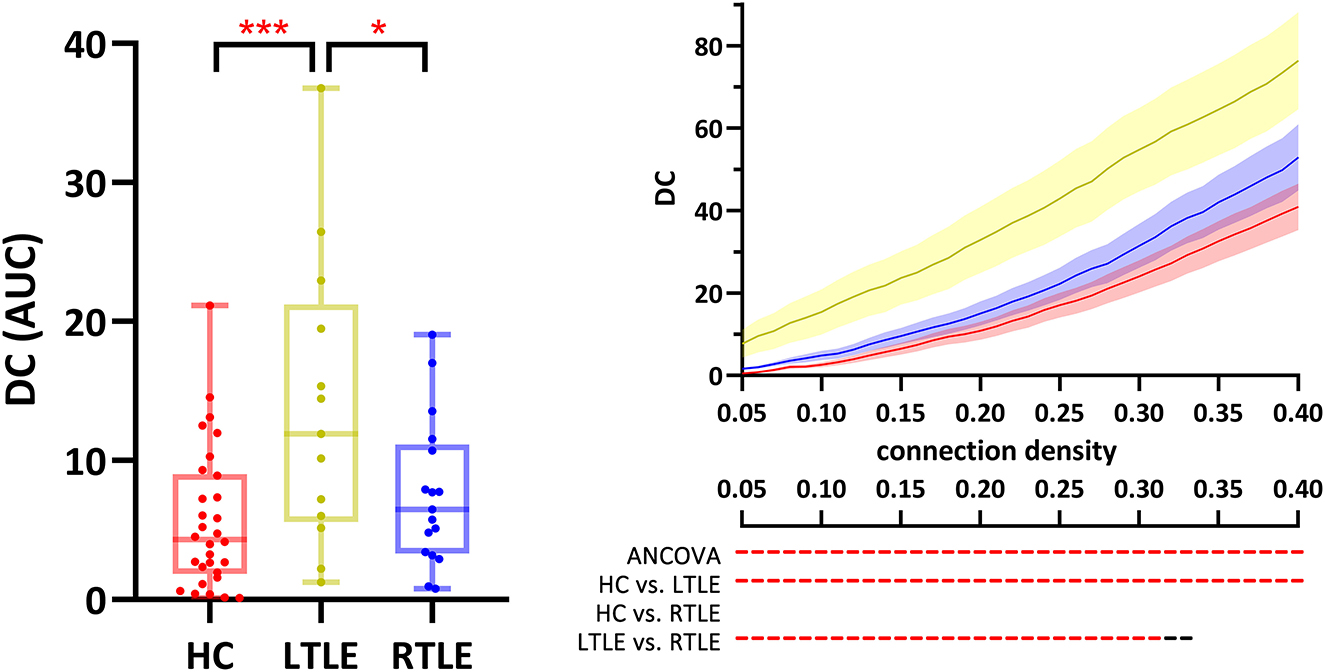
Figure 3. DC of the right cerebellum lobule III was different across the three groups. Red ***, p < 0.001, FDR-corrected; Red - & *, p < 0.05, FDR-corrected; black -, p < 0.05, uncorrected. The boxplot presents the group comparison of AUC, and the line chart shows the group comparison across all connectivity densities. ANCOVA, analysis of covariates; AUC, area under the curve; DC, degree centrality; HC, healthy controls; IC, independent component; LTLE, left temporal lobe epilepsy; RTLE, right temporal lobe epilepsy.
Regarding seed-based functional connectivity, we did not observe any significant differences among the three groups.
3.5. Correlation analysis
In all participants, weaker connectivity from IC 3 to IC 5 (r = −0.33, p = 0.02), from IC 5 to IC 3 (r = −0.29, p = 0.04), and from higher DC of the right lobule III (r = 0.31, p = 0.02) was related to higher scores on Information Tests (Figure 4).
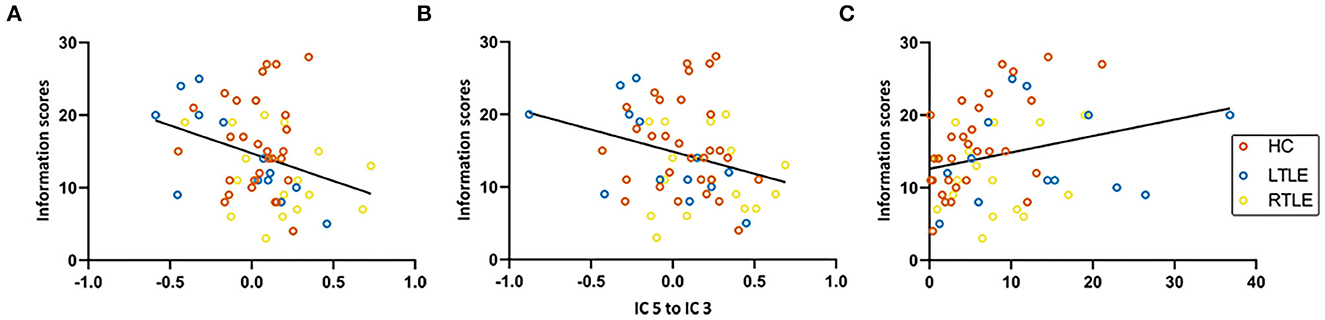
Figure 4. Correlation analysis. HC, healthy controls; IC, independent component; LTLE, left temporal lobe epilepsy; RTLE, right temporal lobe epilepsy. (A) Functional connectivity from IC 3 to IC5. (B) Functional connectivity from IC 5 to IC 3. (C) Degree centrality of right lobule III.
3.6. Sensitivity analysis
One patient with RTLE (female, 29 years old) received topiramate (25 mg, bid). Considering that topiramate was associated with language and cognitive network dysfunction (47, 48), we conducted a sensitivity analysis excluding this patient. The exclusion of this patient did not affect the overall results. In patients with RTLE, functional connectivity between IC 3 and IC 5 and from IC 6 to IC 4 was altered compared to HCs and patients with LTLE. DC of the right lobule III was increased in LTLE compared to HCs (p = 0.002, FDR-corrected) and patients with RTLE (p = 0.03, FDR-corrected).
4. Discussion
Cerebellar abnormality is a common phenotype of MTLE. The cerebellar abnormality contributed to cognitive impairment in MTLE (2, 3, 49). In this study, we applied group ICA and graph theoretical approaches to verbal fluency task-based functional MRI (fMRI) data. We divided the cerebellum into six functionally separated ICs and found disruption in functional connectivity between ICs in patients with RTLE. In LTLE, cerebellar centrality of the right lobule III was significantly increased compared to HC and patients with RTLE. These functional alterations were correlated with a decline in long-term memory in MTLE.
We separated the cerebellum into six functionally discrete components to identify cerebellar networks. Our cerebellar ICs and cerebral parcellations were similar to previously reported atlases. IC 3 and IC 6 contained cerebellar lobule IX, part of the DMN (50, 51), and were connected to cerebral DMN as expected. In our parcellation, IC 1 consists of the primary sensorimotor zone of the cerebellum, and IC 5 is the oculomotor network of the cerebellum (52). Similar to ICs generated with the MELODIC software (29) or the MICA toolbox (43), IC 2 and IC 6 in this study encompassed roughly symmetrical cerebellar regions (crus I and II) and were connected to the right and left FPN, respectively (53). Meanwhile, we noted some differences between our observations and those found in previous studies. In contrast to previous reports in the resting-state data, we noted greater activation in IC 6 than in IC 2 because the Chinese character fluency task was left-hemisphere dominant for right-handed participants. In addition, Alsady et al. observed a cerebellar IC connected to the cerebral DAN and the sensorimotor network. In this study, we separated the cerebellar oculomotor network IC 5, which was connected to the cerebral sensorimotor network, while cerebral DAN was connected to IC 4. Despite the minor discrepancy in group ICA, this study supported other studies regarding cerebellar segregation and cerebro-cerebellar connectivity.
During the task, functional connectivity between IC 3 and IC 5 was enhanced and functional connectivity from IC 6 to IC 4 was impaired in patients with RTLE compared to HCs and patients with LTLE. A mutual connection between IC 3 and IC 5 represented a bidirectional communication between DMN and the sensorimotor system in the cerebellum. Connectivity between the cerebral sensorimotor system and DMN was enhanced in drug-resistant epilepsy (54), frontal lobe epilepsy (55), juvenile myoclonic epilepsy (56), and generalized tonic–clonic seizures (57), and it was thought to lead to epileptic susceptibility (56, 57). Connectivity between the DMN and sensory regions caused lapses in certain ways (58). In addition, hypoconnectivity from IC 6 to IC 4 indicated disconnection between the left FPN, DMN, and DAN. In MTLE, connectivity between DAN and the executive control network was decreased in patients with cognitive impairment (59).
Degree centrality measures the number of suprathreshold connections linked to a certain node. In this study, the right lobule III in LTLE had significantly higher DC than that in RTLE and HCs. The cerebellum became a functional hub for the whole-brain network in MTLE. Garcia-Ramos et al. hypothesized that this is a compensatory reaction as the cerebellum was more integrated into the cerebral network and tried to compensate for the impaired cerebral function (28).
The Chinese version of the Information Test covered basic questions on geography, literature, history, and general knowledge. At the same educational level, the Information Test estimates long-term semantic memory (32). In RTLE, hyperconnectivity between IC 3 and IC 5 contributed to worse performance on the Information Test. In LTLE, higher compensatory DC of the right lobule III was correlated with higher Information Test scores. Though semantic memory was impaired at the same level for LTLE and RTLE in alphabetic languages (60, 61), our previous studies involving more participants reported worse Information Test scores in RTLE compared to HC and LTLE (32, 62), indicating that the Information Test might be a right-hemisphere dominant test in Chinese. Our results indicated that cerebellar disruption and compensation contributed to long-term memory in MTLE and were potential intervention targets for cognitive deficiency in MTLE.
The etiology of cerebellar alterations in TLE remains a controversial topic. Traditionally, cerebellar alterations were considered to be secondary due to seizures or disruption of cerebral networks. Studies focusing on anatomical damage of the cerebellum demonstrated a relationship between more severe cerebellar damage and a higher disease burden. In addition, the volume alteration of the vermis is correlated with the temporal lobe volume (63). However, functional studies of the cerebellum failed to confirm a relationship between disease burden and functional alterations (2, 3). Notably, cerebellar functional abnormalities also did not correlate with the clinical features in this study. Several points might explain the lack of correlation. First, a composite destructive and compensatory process might weaken the correlation between functional abnormalities and clinical features. Second, the cerebellar function was susceptible to ASM. The combinations and types of ASM could have biased the results.
Etiological and functional differences between LTLE and RTLE had been noted in previous studies. The language network (64) and the episodic memory network (65) were contralaterally shifted in LTLE but not in RTLE. Meanwhile, the fiber bundles of the alertness network were impaired, specifically in RTLE (66). The graph theoretical study also revealed different electrophysiological reorganizations in LTLE and RTLE, as functional connectivity was altered in the alpha band in LTLE and in the theta, beta, and gamma bands in RTLE (67). A multivariate pattern classification model constructed with cerebellar and cerebral structural connectivity achieved 93% accuracy in differentiating LTLE from RTLE (68). Consistent with our findings, Zanão et al. observed that the functional connectivity of DMN was enhanced in RTLE, compared to that in LTLE (69). Our result generalized previous findings to the cerebellum and strengthened the idea that LTLE and RTLE might be different epilepsy entities.
This study has limitations. First, ASM affects the results of fMRI (70). Correlation analysis demonstrated that cerebellar function was not biased by the number of ASMs. We also excluded patients who received phenytoin treatment, and we performed a sensitivity analysis excluding a patient who received topiramate. However, different types and combinations of ASM would still influence functional connectivity and correlation analysis. Second, the cerebellar alterations were explored only in fMRI data. The sample sizes of each patient group are also small. Nevertheless, we obtained detailed clinical information and applied robust methods, allowing us to detect cerebellar functional alterations under a stringent threshold. Correlation analysis also excluded potential clinical confounders that might bias the result. Future studies on the combination of structural and functional images in a larger cohort would provide further evidence of cerebellar involvement in MTLE.
5. Conclusion
We noted functional disruption and compensation in the cerebellum of patients with MTLE. Functional connectivity between cerebellar networks was modulated in RTLE, while the centrality of the right lobule III was increased in LTLE. These alterations were correlated with long-term memory in patients. Our results further support the cerebellar involvement in cognitive decline in MTLE and provided potential intervention targets for MTLE.
Data availability statement
The raw data supporting the conclusions of this article will be made available by the authors, without undue reservation.
Ethics statement
The studies involving human participants were reviewed and approved by the Ethics Committee of the Xiangya Hospital of Central South University. Written informed consent to participate in this study was provided by the participants' legal guardian/next of kin.
Author contributions
YP, FX, LL, and BX contributed to the conception and design of the study. YP and KW undertook the analysis and drafted the manuscript. FX, KW, CL, LT, and XL organized the database. FX, KW, CL, LT, MZ, JH, YD, and GW collected the data. All authors contributed to the article and approved the submitted version.
Funding
This study was supported by the National Key Research and Development Program of China (2021YFC1005305), the National Natural Science Foundation of China (82171454), the Key Research and Development Program of Hunan Province (2022SK2042), the Natural Science Foundation of Hunan Province (2020JJ5914), the Innovative Construction Foundation of Hunan Province (2021SK4001), and the National Multidisciplinary Cooperative Diagnosis and Treatment Capacity Project for Major Diseases of Xiangya Hospital, Central South University (z027001).
Conflict of interest
The authors declare that the research was conducted in the absence of any commercial or financial relationships that could be construed as a potential conflict of interest.
Publisher's note
All claims expressed in this article are solely those of the authors and do not necessarily represent those of their affiliated organizations, or those of the publisher, the editors and the reviewers. Any product that may be evaluated in this article, or claim that may be made by its manufacturer, is not guaranteed or endorsed by the publisher.
References
1. Sprengers M, Vonck K, Carrette E, Marson AG, Boon P. Deep brain and cortical stimulation for epilepsy. Cochrane Database Syst Rev. (2017) 7:Cd008497. doi: 10.1002/14651858.CD008497.pub3
2. Nie L, Jiang Y, Lv Z, Pang X, Liang X, Chang W, et al. Deep cerebellar nuclei functional connectivity with cerebral cortex in temporal lobe epilepsy with and without focal to bilateral tonic-clonic seizures: a resting-state fMRI study. Cerebellum. (2021) 21:253–63. doi: 10.1007/s12311-021-01266-3
3. Zhou X, Zhang Z, Liu J, Qin L, Pang X, Zheng J. Disruption and lateralization of cerebellar-cerebral functional networks in right temporal lobe epilepsy: a resting-state fMRI study. Epilepsy Behav. (2019) 96:80–6. doi: 10.1016/j.yebeh.2019.03.020
4. Krook-Magnuson E, Szabo GG, Armstrong C, Oijala M, Soltesz I. Cerebellar directed optogenetic intervention inhibits spontaneous hippocampal seizures in a mouse model of temporal lobe epilepsy. eNeuro. (2014) 1:ENEURO.0005-14.2014. doi: 10.1523/ENEURO.0005-14.2014
5. Hermann BP, Bayless K, Hansen R, Parrish J, Seidenberg M. Cerebellar atrophy in temporal lobe epilepsy. Epilepsy Behav. (2005) 7:279–87. doi: 10.1016/j.yebeh.2005.05.022
6. Hagemann G, Lemieux L, Free SL, Krakow K, Everitt AD, Kendall BE, et al. Cerebellar volumes in newly diagnosed and chronic epilepsy. J Neurol. (2002) 249:1651–8. doi: 10.1007/s00415-002-0843-9
7. Sandok EK, O'Brien TJ, Jack CR, So EL. Significance of cerebellar atrophy in intractable temporal lobe epilepsy: a quantitative MRI study. Epilepsia. (2000) 41:1315–20. doi: 10.1111/j.1528-1157.2000.tb04611.x
8. Oyegbile TO, Bayless K, Dabbs K, Jones J, Rutecki P, Pierson R, et al. The nature and extent of cerebellar atrophy in chronic temporal lobe epilepsy. Epilepsia. (2011) 52:698–706. doi: 10.1111/j.1528-1167.2010.02937.x
9. Guo Q, Wei Z, Fan Z, Hu J, Sun B, Jiang S, et al. Quantitative analysis of cerebellar lobule morphology and clinical cognitive correlates in refractory temporal lobe epilepsy patients. Epilepsy Behav. (2021) 114(Pt A):107553. doi: 10.1016/j.yebeh.2020.107553
10. Kim JS, Koo DL, Joo EY, Kim ST, Seo DW, Hong SB. Asymmetric gray matter volume changes associated with epilepsy duration and seizure frequency in temporal-lobe-epilepsy patients with favorable surgical outcome. J Clin Neurol. (2016) 12:323–31. doi: 10.3988/jcn.2016.12.3.323
11. Salcman M, Defendini R, Correll J, Gilman S. Neuropathological changes in cerebellar biopsies of epileptic patients. Ann Neurol. (1978) 3:10–9. doi: 10.1002/ana.410030104
12. Blumenfeld H, Varghese GI, Purcaro MJ, Motelow JE, Enev M, McNally KA, et al. Cortical and subcortical networks in human secondarily generalized tonic-clonic seizures. Brain. (2009) 132(Pt 4):999–1012. doi: 10.1093/brain/awp028
13. Shin WC, Hong SB, Tae WS, Seo DW, Kim SE. Ictal hyperperfusion of cerebellum and basal ganglia in temporal lobe epilepsy: SPECT subtraction with MRI coregistration. J Nucl Med. (2001) 42:853–8.
14. Won JH, Lee JD, Chung TS, Park CY, Lee BI. Increased contralateral cerebellar uptake of technetium-99m-HMPAO on ictal brain SPECT. J Nucl Med. (1996) 37:426–9.
15. Koziol LF, Budding D, Andreasen N, D'Arrigo S, Bulgheroni S, Imamizu H, et al. Consensus paper: the cerebellum's role in movement and cognition. Cerebellum. (2014) 13:151–77. doi: 10.1007/s12311-013-0511-x
16. Sisakhti M, Sachdev PS, Batouli SAH. The effect of cognitive load on the retrieval of long-term memory: an fMRI study. Front Hum Neurosci. (2021) 15:700146. doi: 10.3389/fnhum.2021.700146
17. O'Hare ED, Lu LH, Houston SM, Bookheimer SY, Sowell ER. Neurodevelopmental changes in verbal working memory load-dependency: an fMRI investigation. Neuroimage. (2008) 42:1678–85. doi: 10.1016/j.neuroimage.2008.05.057
18. Molinari M, Chiricozzi FR, Clausi S, Tedesco AM, De Lisa M, Leggio MG. Cerebellum and detection of sequences, from perception to cognition. Cerebellum. (2008) 7:611–5. doi: 10.1007/s12311-008-0060-x
19. Schweizer TA, Alexander MP, Susan Gillingham BA, Cusimano M, Stuss DT. Lateralized cerebellar contributions to word generation: a phonemic and semantic fluency study. Behav Neurol. (2010) 23:31–7. doi: 10.1155/2010/102421
20. Arasanz CP, Staines WR, Roy EA, Schweizer TA. The cerebellum and its role in word generation: a cTBS study. Cortex. (2012) 48:718–24. doi: 10.1016/j.cortex.2011.02.021
21. Foesleitner O, Sigl B, Schmidbauer V, Nenning KH, Pataraia E, Bartha-Doering L, et al. Language network reorganization before and after temporal lobe epilepsy surgery. J Neurosurg. (2020) 134:1694–702. doi: 10.3171/2020.4.JNS193401
22. Metternich B, Buschmann F, Wagner K, Schulze-Bonhage A, Kriston L. Verbal fluency in focal epilepsy: a systematic review and meta-analysis. Neuropsychol Rev. (2014) 24:200–18. doi: 10.1007/s11065-014-9255-8
23. Bonelli SB, Thompson PJ, Yogarajah M, Vollmar C, Powell RH, Symms MR, et al. Imaging language networks before and after anterior temporal lobe resection: results of a longitudinal fMRI study. Epilepsia. (2012) 53:639–50. doi: 10.1111/j.1528-1167.2012.03433.x
24. Biesbroek JM, Lim JS, Weaver NA, Arikan G, Kang Y, Kim BJ, et al. Anatomy of phonemic and semantic fluency: a lesion and disconnectome study in 1231 stroke patients. Cortex. (2021) 143:148–63. doi: 10.1016/j.cortex.2021.06.019
25. Hubrich-Ungureanu P, Kaemmerer N, Henn FA, Braus DF. Lateralized organization of the cerebellum in a silent verbal fluency task: a functional magnetic resonance imaging study in healthy volunteers. Neurosci Lett. (2002) 319:91–4. doi: 10.1016/S0304-3940(01)02566-6
26. Lesage E, Hansen PC, Miall RC. Right lateral cerebellum represents linguistic predictability. J Neurosci. (2017) 37:6231–41. doi: 10.1523/JNEUROSCI.3203-16.2017
27. Guo L, Bai G, Zhang H, Lu D, Zheng J, Xu G. Cognitive functioning in temporal lobe epilepsy: a BOLD-fMRI study. Mol Neurobiol. (2017) 54:8361–9. doi: 10.1007/s12035-016-0298-0
28. Garcia-Ramos C, Song J, Hermann BP, Prabhakaran V. Low functional robustness in mesial temporal lobe epilepsy. Epilepsy Res. (2016) 123:20–8. doi: 10.1016/j.eplepsyres.2016.04.001
29. Dobromyslin VI, Salat DH, Fortier CB, Leritz EC, Beckmann CF, Milberg WP, et al. Distinct functional networks within the cerebellum and their relation to cortical systems assessed with independent component analysis. Neuroimage. (2012) 60:2073–85. doi: 10.1016/j.neuroimage.2012.01.139
30. Buckner RL, Krienen FM, Castellanos A, Diaz JC, Yeo BT. The organization of the human cerebellum estimated by intrinsic functional connectivity. J Neurophysiol. (2011) 106:2322–45. doi: 10.1152/jn.00339.2011
31. Wang K, Xie F, Liu C, Tan L, He J, Hu P, et al. Abnormal functional connectivity profiles predict drug responsiveness in patients with temporal lobe epilepsy. Epilepsia. (2022) 63:463–73. doi: 10.1111/epi.17142
32. Tan L, Chen Y, Wu W, Liu C, Fu Y. Impaired cognitive abilities in siblings of patients with temporal lobe epilepsy. Neuropsychiatr Dis Treat. (2020) 16:3071–9. doi: 10.2147/NDT.S258074
33. Cook MJ, Fish DR, Shorvon SD, Straughan K, Stevens JM. Hippocampal volumetric and morphometric studies in frontal and temporal lobe epilepsy. Brain. (1992) 115 (Pt 4):1001–15. doi: 10.1093/brain/115.4.1001
34. Mo J, Liu Z. Automated detection of hippocampal sclerosis using clinically empirical and radiomics features. Epilepsia. (2019) 60:2519–29. doi: 10.1111/epi.16392
35. Winston GP, Cardoso MJ, Williams EJ, Burdett JL, Bartlett PA, Espak M, et al. Automated hippocampal segmentation in patients with epilepsy: available free online. Epilepsia. (2013) 54:2166–73. doi: 10.1111/epi.12408
36. Yang N, Waddington G, Adams R, Han J. Translation, cultural adaption, and test-retest reliability of Chinese versions of the Edinburgh Handedness Inventory and Waterloo footedness questionnaire. Laterality. (2018) 23:255–73. doi: 10.1080/1357650X.2017.1357728
37. Ferner R, Day R, Bradberry SM. Phenytoin and damage to the cerebellum - a systematic review of published cases. Expert Opin Drug Saf. (2022) 21:957–77. doi: 10.1080/14740338.2022.2058487
38. Nasreddine ZS, Phillips NA, Bédirian V, Charbonneau S, Whitehead V, Collin I, et al. The montreal cognitive assessment, MoCA: a brief screening tool for mild cognitive impairment. J Am Geriatr Soc. (2005) 53:695–9. doi: 10.1111/j.1532-5415.2005.53221.x
39. Ward LC, Ryan JJ, Axelrod BN. Confirmatory factor analyses of the WAIS-III standardization data. Psychol Assess. (2000) 12:341–5. doi: 10.1037/1040-3590.12.3.341
41. Ci H, van Graan A, Gonzálvez G, Thompson P, Hill A, Duncan JS. Mandarin functional MRI Language paradigms. Brain Behav. (2016) 6:e00525. doi: 10.1002/brb3.525
42. Whitfield-Gabrieli S, Nieto-Castanon A. Conn: a functional connectivity toolbox for correlated and anticorrelated brain networks. Brain Connect. (2012) 2:125–41. doi: 10.1089/brain.2012.0073
43. Moher Alsady T, Blessing EM, Beissner F. MICA-A toolbox for masked independent component analysis of fMRI data. Hum Brain Mapp. (2016) 37:3544–56. doi: 10.1002/hbm.23258
44. Fan L, Li H, Zhuo J, Zhang Y, Wang J, Chen L, et al. The human brainnetome atlas: a new brain atlas based on connectional architecture. Cereb Cortex. (2016) 26:3508–26. doi: 10.1093/cercor/bhw157
45. Tzourio-Mazoyer N, Landeau B, Papathanassiou D, Crivello F, Etard O, Delcroix N, et al. Automated anatomical labeling of activations in SPM using a macroscopic anatomical parcellation of the MNI MRI single-subject brain. Neuroimage. (2002) 15:273–89. doi: 10.1006/nimg.2001.0978
46. Caciagli L, Allen LA, He X. Thalamus and focal to bilateral seizures: a multiscale cognitive imaging study. Neurology. (2020) 95:e2427–e41. doi: 10.1212/WNL.0000000000010645
47. Wandschneider B, Burdett J, Townsend L, Hill A, Thompson PJ, Duncan JS, et al. Effect of topiramate and zonisamide on fMRI cognitive networks. Neurology. (2017) 88:1165–71. doi: 10.1212/WNL.0000000000003736
48. Tang Y, Xia W, Yu X, Zhou B, Wu X, Lui S, et al. Altered cerebral activity associated with topiramate and its withdrawal in patients with epilepsy with language impairment: an fMRI study using the verb generation task. Epilepsy Behav. (2016) 59:98–104. doi: 10.1016/j.yebeh.2016.03.013
49. Pang L, Fan B, Chen Z, Chen Z, Lv C, Zheng J. Disruption of cerebellar-cerebral functional connectivity in temporal lobe epilepsy and the connection to language and cognitive functions. Front Neurosci. (2022) 16:871128. doi: 10.3389/fnins.2022.871128
50. Habas C, Kamdar N, Nguyen D, Prater K, Beckmann CF, Menon V, et al. Distinct cerebellar contributions to intrinsic connectivity networks. J Neurosci. (2009) 29:8586–94. doi: 10.1523/JNEUROSCI.1868-09.2009
51. Damoiseaux JS, Rombouts SA, Barkhof F, Scheltens P, Stam CJ, Smith SM, et al. Consistent resting-state networks across healthy subjects. Proc Natl Acad Sci U S A. (2006) 103:13848–53. doi: 10.1073/pnas.0601417103
52. O'Reilly JX, Beckmann CF, Tomassini V, Ramnani N, Johansen-Berg H. Distinct and overlapping functional zones in the cerebellum defined by resting state functional connectivity. Cereb Cortex. (2010) 20:953–65. doi: 10.1093/cercor/bhp157
53. Krienen FM, Buckner RL. Segregated fronto-cerebellar circuits revealed by intrinsic functional connectivity. Cereb Cortex. (2009) 19:2485–97. doi: 10.1093/cercor/bhp135
54. Fu C, Aisikaer A, Chen Z, Yu Q, Yin J, Yang W. Antiepileptic efficacy and network connectivity modulation of repetitive transcranial magnetic stimulation by vertex suppression. Front Hum Neurosci. (2021) 15:667619. doi: 10.3389/fnhum.2021.667619
55. Widjaja E, Zamyadi M, Raybaud C, Snead OC, Smith ML. Abnormal functional network connectivity among resting-state networks in children with frontal lobe epilepsy. AJNR Am J Neuroradiol. (2013) 34:2386–92. doi: 10.3174/ajnr.A3608
56. Vollmar C, O'Muircheartaigh J, Barker GJ, Symms MR, Thompson P, Kumari V, et al. Motor system hyperconnectivity in juvenile myoclonic epilepsy: a cognitive functional magnetic resonance imaging study. Brain. (2011) 134(Pt 6):1710–9. doi: 10.1093/brain/awr098
57. Zhang Y, Huang G, Liu M, Li M, Wang Z, Wang R, et al. Functional and structural connective disturbance of the primary and default network in patients with generalized tonic-clonic seizures. Epilepsy Res. (2021) 174:106595. doi: 10.1016/j.eplepsyres.2021.106595
58. Huang S, Li Y, Zhang W, Zhang B, Liu X, Mo L, et al. Multisensory competition is modulated by sensory pathway interactions with fronto-sensorimotor and default-mode network regions. J Neurosci. (2015) 35:9064–77. doi: 10.1523/JNEUROSCI.3760-14.2015
59. Zhang C, Yang H, Qin W, Liu C, Qi Z, Chen N, et al. Characteristics of resting-state functional connectivity in intractable unilateral temporal lobe epilepsy patients with impaired executive control function. Front Hum Neurosci. (2017) 11:609. doi: 10.3389/fnhum.2017.00609
60. Tramoni-Negre E, Lambert I, Bartolomei F, Felician O. Long-term memory deficits in temporal lobe epilepsy. Rev Neurol. (2017) 173:490–7. doi: 10.1016/j.neurol.2017.06.011
61. Leritz EC, Grande LJ, Bauer RM. Temporal lobe epilepsy as a model to understand human memory: the distinction between explicit and implicit memory. Epilepsy Behav. (2006) 9:1–13. doi: 10.1016/j.yebeh.2006.04.012
62. He J, Xiao B, Liu C, Wang K, Tan L, Long L. Feature of cognitive dysfunction in patients with temporal lobe epilepsy and its clinical influencing factors. J Cent South Univ Med Sci. (2021) 46:240–8. doi: 10.11817/j.issn.1672-7347.2021.200770
63. Marcián V, Mareček R, Pail M, Brázdil M. Cerebrocerebellar structural covariance in temporal lobe epilepsy with hippocampal sclerosis. Epilepsy Behav. (2020) 111:107180. doi: 10.1016/j.yebeh.2020.107180
64. Nenning KH, Fösleitner O, Schwartz E, Schwarz M, Schmidbauer V, Geisl G, et al. The impact of hippocampal impairment on task-positive and task-negative language networks in temporal lobe epilepsy. Clin Neurophysiol. (2021) 132:404–11. doi: 10.1016/j.clinph.2020.10.031
65. Sideman N, Chaitanya G, He X, Doucet G, Kim NY, Sperling MR, et al. Task activation and functional connectivity show concordant memory laterality in temporal lobe epilepsy. Epilepsy Behav. (2018) 81:70–8. doi: 10.1016/j.yebeh.2018.01.027
66. Liu HH, Wang J, Chen XM, Li JP, Ye W, Zheng J. Interhemispheric functional and structural alterations and their relationships with alertness in unilateral temporal lobe epilepsy. Eur Rev Med Pharmacol Sci. (2016) 20:1526–36.
67. Jin SH, Jeong W, Chung CK. Mesial temporal lobe epilepsy with hippocampal sclerosis is a network disorder with altered cortical hubs. Epilepsia. (2015) 56:772–9. doi: 10.1111/epi.12966
68. Fang P, An J, Zeng LL, Shen H, Chen F, Wang W, et al. Multivariate pattern analysis reveals anatomical connectivity differences between the left and right mesial temporal lobe epilepsy. NeuroImage Clin. (2015) 7:555–61. doi: 10.1016/j.nicl.2014.12.018
69. Zanão TA, Lopes TM, de Campos BM, Yasuda CL, Cendes F. Patterns of default mode network in temporal lobe epilepsy with and without hippocampal sclerosis. Epilepsy Behav. (2021) 121(Pt B):106523. doi: 10.1016/j.yebeh.2019.106523
Keywords: temporal lobe epilepsy, cerebellum, fMRI, verbal fluency task, independent component analysis (ICA), graph theory
Citation: Peng Y, Wang K, Liu C, Tan L, Zhang M, He J, Dai Y, Wang G, Liu X, Xiao B, Xie F and Long L (2023) Cerebellar functional disruption and compensation in mesial temporal lobe epilepsy. Front. Neurol. 14:1062149. doi: 10.3389/fneur.2023.1062149
Received: 05 October 2022; Accepted: 06 January 2023;
Published: 02 February 2023.
Edited by:
Clarissa Lin Yasuda, State University of Campinas, BrazilReviewed by:
Panpan Hu, Anhui Medical University, ChinaRui Feng, Fudan University, China
Rebecca Kerestes, Monash University, Australia
Copyright © 2023 Peng, Wang, Liu, Tan, Zhang, He, Dai, Wang, Liu, Xiao, Xie and Long. This is an open-access article distributed under the terms of the Creative Commons Attribution License (CC BY). The use, distribution or reproduction in other forums is permitted, provided the original author(s) and the copyright owner(s) are credited and that the original publication in this journal is cited, in accordance with accepted academic practice. No use, distribution or reproduction is permitted which does not comply with these terms.
*Correspondence: Lili Long, longlili1982@126.com; Fangfang Xie,
76335922@qq.com
†These authors have contributed equally to this work and share first authorship