- 1Key Laboratory for the Genetics of Developmental and Neuropsychiatric Disorders, Bio-X Institutes, Shanghai Jiao Tong University, Shanghai, China
- 2Shanghai Key Laboratory of Psychotic Disorders, Brain Science and Technology Research Center, Shanghai Jiao Tong University, Shanghai, China
- 3Shanghai Mental Health Center, Shanghai Jiao Tong University School of Medicine, Shanghai, China
Introduction: Alzheimer's disease (AD) is a type of neurodegenerative disease that has no effective treatment in its late stage, making the early prediction of AD critical. There have been an increase in the number of studies indicating that miRNAs play an important role in neurodegenerative diseases including Alzheimer's disease via epigenetic modifications including DNA methylation. Therefore, miRNAs may serve as excellent biomarkers in early AD prediction.
Methods: Considering that the non-coding RNAs' activity may be linked to their corresponding DNA loci in the 3D genome, we collected the existing AD-related miRNAs combined with 3D genomic data in this study. We investigated three machine learning models in this work under leave-one-out cross-validation (LOOCV): support vector classification (SVC), support vector regression (SVR), and knearest neighbors (KNNs).
Results: The prediction results of different models demonstrated the effectiveness of incorporating 3D genome information into the AD prediction models.
Discussion: With the assistance of the 3D genome, we were able to train more accurate models by selecting fewer but more discriminatory miRNAs, as witnessed by several ML models. These interesting findings indicate that the 3D genome has great potential to play an important role in future AD research.
1. Introduction
1.1. Alzheimer's disease
Alzheimer's disease, one of the most common causes of dementia, is a progressive, persistent, and irreversible neurodegenerative disease affecting the normal functioning of the cerebral cortex and hippocampus (1). The causes of AD may consist of both genetic and epigenetic (environmentally acquired) factors. Risk gene germline mutations can only explain the pathogenesis of a small proportion of patients with AD. There are several hypotheses regarding the pathogenesis of AD, the more recognized ones being the Aβ amyloid (2), the Tau protein (3), the cholinergic depletion (4), the inflammaging (5), the oxidative stress (6), the mitochondrial damage (7), and the disrupted glycolipid metabolism (8). In addition, there are also some causal associations between these hypotheses.
Since patients with early AD (preclinical stage) often do not demonstrate significant disease symptoms, some patients may undergo a preclinical stage of up to 25 years (9). Several studies have analyzed the preventive effects of early screening for AD through meta-analysis and have shown that early intervention in the preclinical stage of AD can effectively slow down cognitive decline in subjects (10–12). Early screening for AD, therefore, is important as it can provide more information on the treatment options for patients with AD and psychological support for both the patients and their family members.
Due to that, the pathogenesis of AD may involve multiple pathogenic pathways acting together. As mentioned in previous studies, traditional pathophysiological biomarkers, in general, are ineffective in terms of specificity and sensitivity when combined, leaving the clinical choice of early AD biomarkers lacking (13). In recent years, several studies have reported that microRNAs (miRNAs) are closely associated with AD, but advanced prediction models and novel biological insights that can benefit the prediction are still insufficient. Therefore, in this study, we focus on miRNA and aim to explore their clinical biomarker potential in early AD detection from the perspective of the 3D genome (14, 15).
1.2. MicroRNA and AD
MicroRNAs are small non-coding RNAs (sncRNAs) consisting of 19–23 nucleotides (nt), and they are essential epigenetic and post-transcriptional regulators that cooperate with messenger RNA (mRNA). miRNAs are highly mobile and permeable, ubiquitous in the human brain and central nervous system, and the smallest eukaryotic nucleic acid (16–18). miRNAs are translocated and released into extracellular fluids, such as plasma/serum, cerebrospinal fluid, saliva, urine, tears, semen, and ovarian follicular fluid, and such secretable hormone-like miRNAs are known as extracellular miRNAs or circulating miRNAs (19). Extracellular miRNAs can be delivered to target cells via extracellular fluid circulation and regulate the corresponding cellular activities (20, 21); moreover, extracellular miRNAs are highly stable and can avoid degradation under stressful conditions such as storage at room temperature for up to 24 h and multiple freeze–thaw cycles (22). These properties indicate the potential and capability of utilizing miRNAs as biomarkers. In fact, some applications of miRNAs have already been explored, such as using them as biomarkers in neurological diseases like Parkinson's disease, Huntington's disease, amyotrophic lateral sclerosis, bipolar disorder, and schizophrenia (23, 24).
Studies have successfully revealed significant correlations between miRNA dysregulation and AD, such as miR-9, miR-34a, miR-125b, miR-146a, and miR-155 (25). Some have analyzed the metabolic pathways of Aβ and tau proteins and identified exosome miRNAs closely related to AD, including miR-193b, miR-342-3p, and miR-451a (26). Moreover, by analyzing the oxidative stress (OS) pathogenesis, researchers have found multiple affected miRNAs, such as miR-200c, miR-26b, miR-107, and miR-210 (27). These findings suggest the key role of miRNAs in AD pathogenesis. How to systematically discover more AD-related miRNAs suitable for AD early screening becomes the next challenge.
1.3. miRNA and 3D genome
As early as the 19th century, several studies observed that chromosomes in the nucleus exhibit a chromatin form and were kept in distinct and relatively fixed regions during interphase, leading to the concept of the “Chromosome Territory (CT)”. In CT, chromosome positions are relatively constant and differentially distributed between cells in which homologous chromosomes tend to separate from each other. In fact, only until the recent development of the chromosome conformation capture (3C) method (28) and its high-throughput method Hi-C (high-throughput chromosome conformation capture) (29), the concept of the 3D genome was systematically introduced.
Our previous studies on disease typing prediction discovered that adding chromatin 3D genome information into deep neural network models could significantly improve prediction accuracy (30, 31). This phenomenon is due to the fact that the 3D genome positions of disease-related DNAs and RNAs in the nucleus play an important role (e.g., the radius distance from chromatin to the center of the nucleus), which also suggests that the broader role of the 3D chromatin conformation in cell function and the mechanistic linkage between them are worth further investigation.
Although extracellular miRNAs are free-floating, the foremost step for their function is the repressive effect in the transcriptional phase. It has been hypothesized that the out-of-nucleus translocation of miRNAs in neurons can occur through the co-delivery of the AGO proteins and target mRNAs containing localization signals (32). In principle, the proximity of miRNAs to target DNA in the chromatin 3D spatial conformation is more efficient in utilizing cellular energy for physiological functions. Therefore, we believe that the 3D genome will play a critical role in boosting such interactions.
To summarize, we analyzed a causal chain of the “chromatin 3D conformation-driven cellular functional block” phenomenon: DNA co-localization → RNA co-expression → protein–protein interaction. These co-expressed RNAs will preferentially aggregate in the nucleus and be transported from specific nuclear pores into the cytoplasm. This allows for the efficient enrichment of small molecules in the cytoplasmic space. Such an approach increases the frequency of miRNA contact with target genes and accomplishes the regulation of genes with lower energy consumption, which is very much in line with the evolutionary rules. We suggest that the topologically associating domain (TAD)-like nuclear regions in the cell influence the cellular state and drive certain cellular behaviors; such blocks, which have a three-dimensional conformation and jointly regulate certain cellular functions, are “functional blocks”. Specifically, we believe that when a “functional block” is abnormally activated/inhibited, it will change the cellular state. When the DNA, the starting “puzzle”, is mutated or transcriptionally repressed, RNA transcription in the same “functional block” will also be abnormal, leading to abnormal protein expression and causing cellular dysfunction. We hypothesize that miRNAs that play essential disease-mediation functions also have certain spatially distributed properties. In this study, therefore, we optimize the miRNA-based AD prediction model by incorporating 3D genome information and further explore and discuss the value of applying 3D genomic information in AD early screening.
2. Methods
2.1. Dataset
The GSE120584 dataset adopted in this study was downloaded from the public database Gene Expression Omnibus (GEO). RNA data were extracted from the serum tissues of 1,309 Japanese individuals, containing 1,021 patients with AD and 288 normal controls (NCs). In this dataset, each miRNA signal value was standardized with the ratio of the average signal of the three internal control miRNA signals. The sample labels are indicated by 0 or 1, with 1 indicating patients with AD and 0 indicating normal control.
2.2. miRNA profiling
To quantify miRNA expression, we downloaded the RAW files of GSE120584 and generated the RNA expression matrix from them. We then converted the miRNA naming format to the latest miRBase V22 version by the miEAA 2.0 platform (33) and subsequently performed manual curation and validation. We mapped all miRNA tags to the human reference genome GRCh 37/hg19 using the R package (bioMart) which belongs to different compartments in the 3D genome. Due to the specificity of the miRNA biogenesis pathway, precursor miRNAs do not show one-to-one correspondence with mature miRNAs; thus, these miRNAs cannot be annotated uniquely and were excluded to ensure the miRNA uniqueness. After the annotation, transcripts with expression scores < 5 in < 1,000 samples were removed. Annotated miRNA quantification data were then adopted for Spearman correlation coefficient calculations and 3D clustering analysis. In the end, we obtained 1,605 valid miRNAs and 214 miRNAs with 3D information.
2.3. Acquisition of 3D coordinates of miRNAs
We constructed 3D genome models using a molecular dynamic approach based on the hESC cell line Hi-C data (34, 35), which generated 300 feasible conformational structures and selected the best-matched model as the 3D genome model for subsequent analyses. Then, by using the transcriptional start site (TSS) position of the miRNAs as an index, we matched miRNAs to the 3D coordinates in the intranuclear space.
2.4. Prediction model and feature selection
Model construction was done by the python library sklearn, and DBSCAN was used for the density-based clustering method (eps = 3). All models were trained by leave-one-out cross-validation. The Spearman correlation coefficient is implemented with scipy in python. While evaluating the models, we set label = 0.5 as the cutoff, 0–0.5 is considered negative results, and 0.5–1 is considered positive results. The whole workflow of miRNA selection and model fitting has been shown in Figure 1.
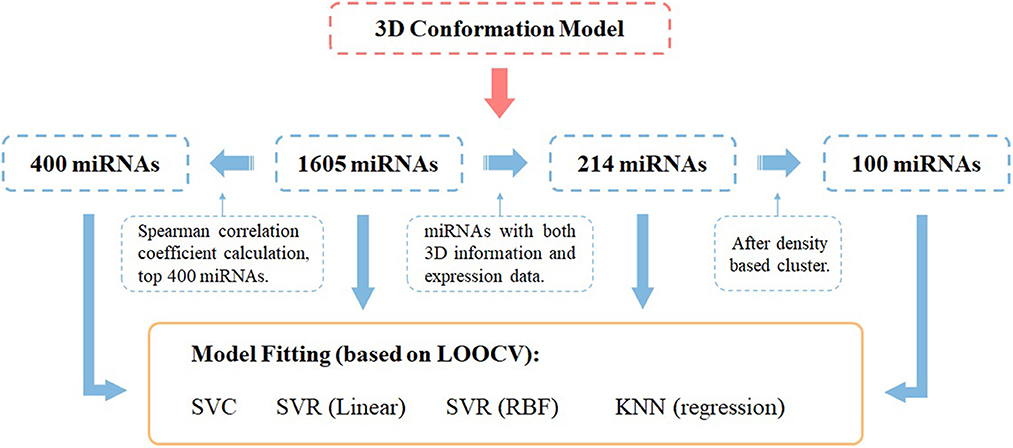
Figure 1. Workflow of miRNA panel selections and their AD score prediction effectiveness evaluated by different ML prediction models under the leave-one-out cross-validation framework.
3. Results
3.1. Clustering analysis of miRNAs on 3D positional
To subsequently combine the 3D distribution of miRNAs for feature selection, we performed density clustering DBSCAN of miRNAs with 3D information encoding, i.e., <x, y, z> coordinates, and obtained nine clusters with multiple miRNAs and 32 standalone clusters with single miRNAs. The clustering result is shown in Figure 2, and as it demonstrates, each miRNA cluster occupies a salient spatial territory.
3.2. Machine learning prediction model optimization
All samples were first divided into two groups according to disease type (0 or 1). We investigated three machine learning models under leave-one-out cross-validation (LOOCV): support vector classification (SVC), support vector regression (SVR), and k-nearest neighbors (KNNs). Both linear and RBF kernels were chosen for SVR.
First, we used all the annotated 1,605 miRNAs as features to perform predictions for AD; Table 1 demonstrates the effectiveness of each model using the all miRNAs feature panel. Subsequently, we performed a Spearman correlation coefficient (SCC)-based selection of the feature miRNAs and selected the top 400 miRNAs with the highest SCC score (p < 0.05). The results showed that prediction accuracies from all models increased to some extent (Table 1).
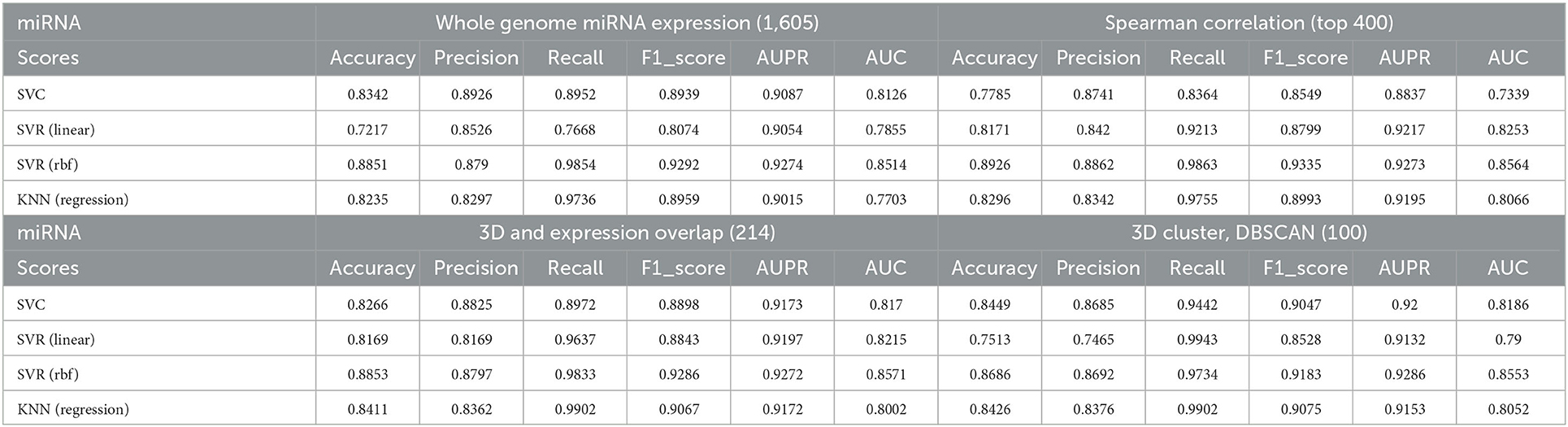
Table 1. Correlations between predicted AD values and true values under different models and different selected miRNA panels.
To better investigate the ability of 3D genome information in contributing to the prediction models, we obtained the spatial coordinates of 214 miRNAs in the hESC nucleus by mapping the gene starting position on chromosomes. We proportionally selected the miRNAs with the highest Spearman correlation within each cluster as representatives of the clusters, yielding 100 miRNAs, and used them to train the model. Note that many informative miRNAs that could not be uniquely mapped to 3D genome space were discarded. The features obtained by such screening could still improve the prediction accuracies of the models, with the SVC model showing the most significant improvement.
In order to better compare the results under different features, we plotted the Precision–Recall curves (Figure 3) and ROC curves (Figure 4) for all models. We also plotted the violin plot (Figure 5) for the prediction results of different models, which demonstrated the effectiveness of incorporating 3D genome information into the AD prediction models.
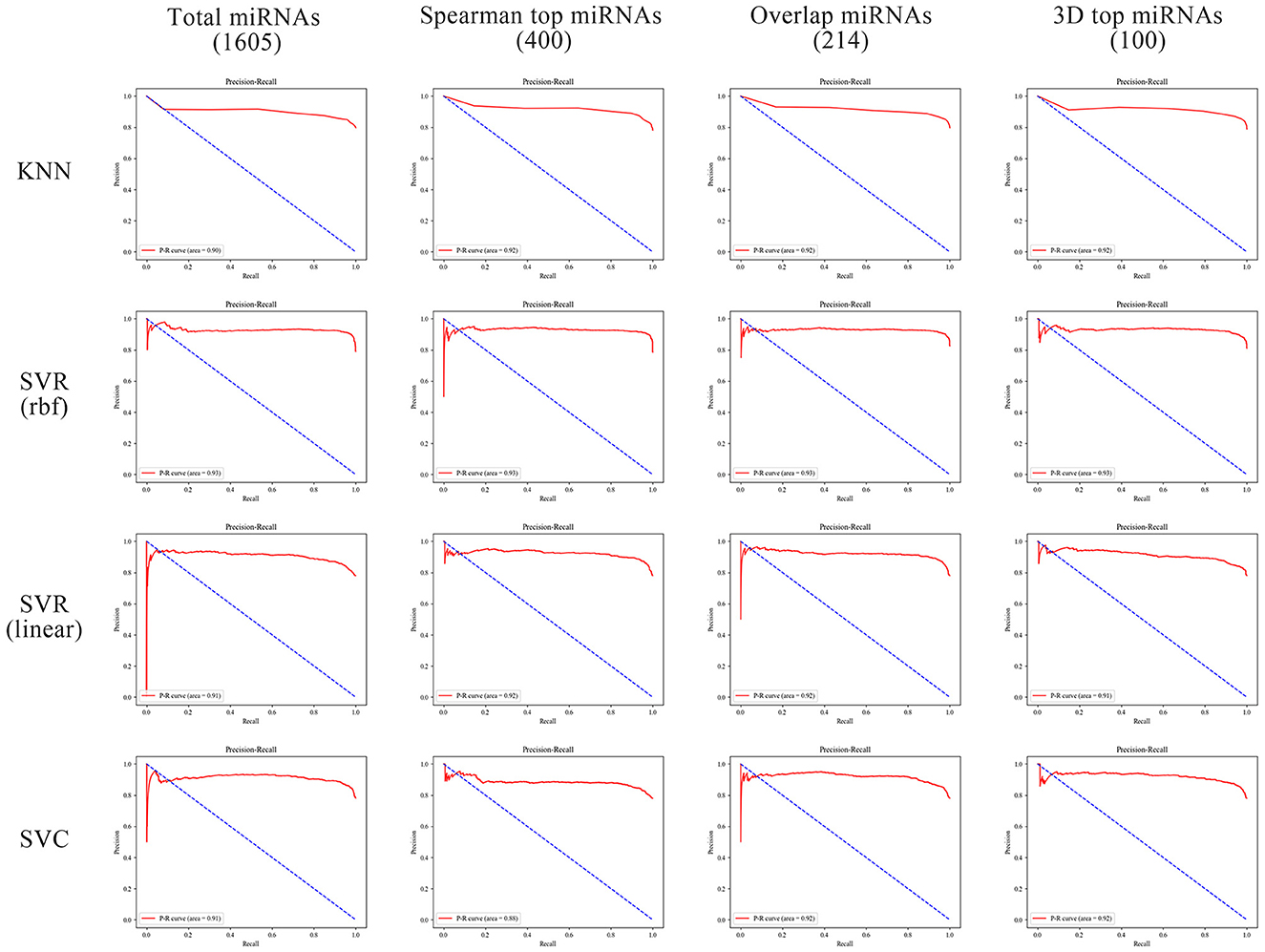
Figure 3. Precision–Recall curves of different ML models built using different miRNAs as prediction feature panels.
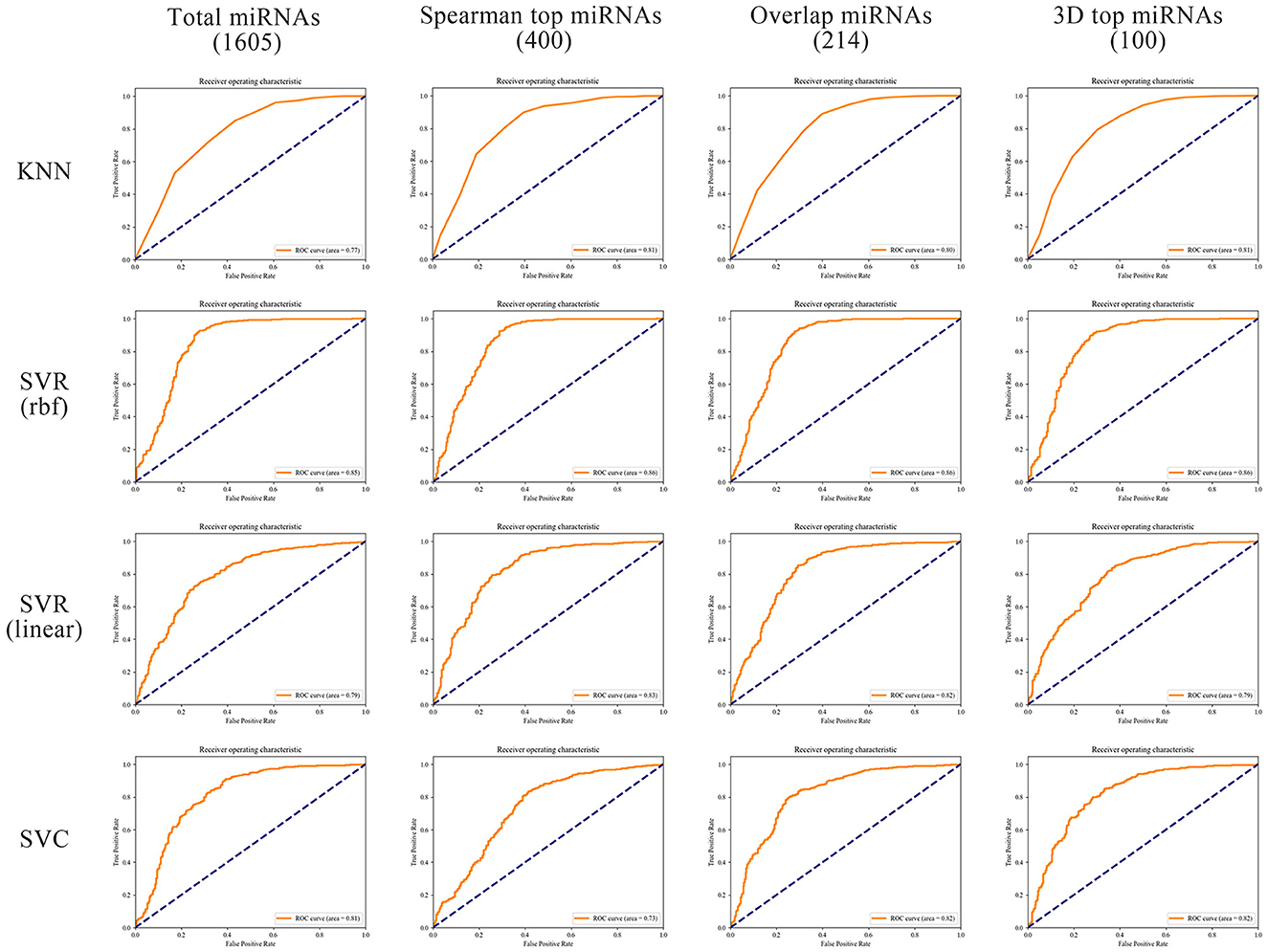
Figure 4. ROC curves of different ML models built using different miRNAs as prediction feature panels.
3.3. Effects of epigenetic functions of miRNAs on AD
Indeed, the pathogenesis of AD is coherently linked to epigenetic phenomena, such as DNA methylation or non-coding RNA interference (36), while miRNAs play a role in the latter (37). During the feature selection, we further analyzed the miRNAs contributing to the prediction models. We found that miR-128 is one of the most contributing miRNAs; miR-128 was reported to downregulate PPAR-γ expression in mouse cortical neurons (MCNs) and Neuro2a (N2a) cells, which affects downstream NF-κB activity and, thus, triggers Aβ-mediated cytotoxicity (38). Similarly, we found another contributing miRNA miR-128, which was also reported to cause dysfunctional synaptic transmission between mossy cells (MCs) and somatostatin (SST) cells by inhibiting the normal function of the STIM2 gene (39). Furthermore, miR-210 in our predictor panel has even been found to play a role in the production of ROS in the brain with altered cholinergic neuronal states (40, 41). These findings provide essential reference information for the study of the pathogenesis of AD, and more relationships between differentially expressed miRNAs and corresponding targets in patients with AD are expected to be discovered in the future.
4. Conclusion and discussion
In this study, we analyzed the feature selection and machine learning prediction model optimization effects of using miRNA expression in AD prediction by incorporating 3D genome information. With the assistance of the 3D genome, we were able to train more accurate models by selecting fewer but more discriminatory miRNAs, as witnessed by all ML models, including SVC, SVR, and KNN. These interesting findings also indicate that the 3D genome has great potential to play an important role in future AD research.
MicroRNA is a typical epigenetic modulator undertaking multiple epigenetic mechanisms (42). Crosstalk between miRNAs and epigenetic regulation is important for neural development (37, 43). The enzymes of epigenetic modification processes can be regulated by miRNAs (44). Abnormal epigenetic regulation leads to abnormal miRNA expression, which further leads to the pathogenic mechanism of several malignancies (45). Specifically, manipulation of levels of mir-137, a miRNA associated with neuropsychiatric disorders in mice induces neurological abnormalities such as synaptic overgrowth, memory deficits, and repetitive behaviors (46).
Recent studies have further demonstrated that miRNAs can influence epigenetic phenomena by regulating the expression of DNA methylesterase. Several studies have found that the downregulation of miR-29 family (including miR-29a, miR-29b, and miR-29c) expression suppresses the expression of retinoblastoma-like protein 2 (Rbl2), which causes DNA methylation deficiency by inhibiting the activity of DNMT3a and DNMT3b (47, 48). It has also been shown that miR-17 and miR-20a in mammalian cells can induce heterochromatin formation in promoter regions with overlapping transcriptional functions and complementary to miRNA seed regions, further revealing a new mechanism of miRNA-regulated chromatin remodeling and gene transcription (49). These results suggest that there is a potentially complicated but strong connection between miRNA and 3D genome and worth in-depth exploration, and the analyses of neurodegenerative diseases such as AD from the novel perspective of 3D genome can be of great interest.
Data availability statement
The original contributions presented in the study are included in the article/supplementary material, further inquiries can be directed to the corresponding authors.
Ethics statement
Ethical review and approval was not required for the study on human participants in accordance with the local legislation and institutional requirements. Written informed consent from the patients/participants or patients/participants' legal guardian/next of kin was not required to participate in this study in accordance with the national legislation and the institutional requirements.
Author contributions
KL, RC, and LL participated in the omics and computational experiments. MF and KS assisted computational experiments. KL provided the figures, tables, and drafted the manuscript. XL provided clinical suggestions about AD and biological insights into miRNA. YS and GH initiated this project, supervised the whole workflow, and edited and finalized the manuscript. All authors reviewed and proofread the manuscript and the experimental results.
Funding
This project was supported by the Key Research and Development Plan of the Ministry of Science and Technology (2022YFE0125300), the National Key Research and Development Program (2016YFC0906400), the Innovation Funding in Shanghai (20JC1418600 and 18JC1413100), the National Natural Science Foundation of China (82071262 and 81671326), the Natural Science Foundation of Shanghai (20ZR1427200 and 20511101900), the Shanghai Municipal Science and Technology Major Project (2017SHZDZX01), the Shanghai Key Laboratory of Psychotic Disorders (13DZ2260500), the Shanghai Leading Academic Discipline Project (B205), and the Shanghai Jiao Tong University STAR Grant (YG2023ZD26, YG2022ZD024, and YG2022QN111).
Acknowledgments
The miRNA expression data were contributed to GEO by Shigemizu et al. using 3D-Gene Human miRNA V21_1.0.0 platform GPL21263 (https://www.ncbi.nlm.nih.gov/geo/query/acc.cgi?acc=GSE120584).
Conflict of interest
The authors declare that the research was conducted in the absence of any commercial or financial relationships that could be construed as a potential conflict of interest.
The reviewer XZ declared a shared affiliation with the authors KL, RC, LL, MF, KS, XL, GH, and YS at the time of review.
Publisher's note
All claims expressed in this article are solely those of the authors and do not necessarily represent those of their affiliated organizations, or those of the publisher, the editors and the reviewers. Any product that may be evaluated in this article, or claim that may be made by its manufacturer, is not guaranteed or endorsed by the publisher.
References
1. Masters CL, Bateman R, Blennow K, Rowe CC, Sperling RA, Cummings JL. Alzheimer's disease. Nat Rev Dis Primers. (2015) 1:15056. doi: 10.1038/nrdp.2015.56
2. Hardy J, Allsop D. Amyloid deposition as the central event in the aetiology of Alzheimer's disease. Trends Pharmacol Sci. (1991) 12:383–8. doi: 10.1016/0165-6147(91)90609-V
3. Strittmatter WJ, Saunders AM, Schmechel D, Pericak-Vance M, Enghild J, Salvesen GS, et al. Apolipoprotein E: high-avidity binding to beta-amyloid and increased frequency of type 4 allele in late-onset familial Alzheimer disease. Proc Natl Acad Sci USA. (1993) 90:1977–81. doi: 10.1073/pnas.90.5.1977
4. Schorderet M. Alzheimer's disease: fundamental and therapeutic aspects. Experientia. (1995) 51:99–105. doi: 10.1007/BF01929348
5. Franceschi C, Capri M, Monti D, Giunta S, Olivieri F, Sevini F, et al. Inflammaging and anti-inflammaging: a systemic perspective on aging and longevity emerged from studies in humans. Mech Ageing Dev. (2007) 128:92–105. doi: 10.1016/j.mad.2006.11.016
6. Cheignon C, Tomas M, Bonnefont-Rousselot D, Faller P, Hureau C, Collin F. Oxidative stress and the amyloid beta peptide in Alzheimer's disease. Redox Biol. (2018) 14:450–64. doi: 10.1016/j.redox.2017.10.014
7. Lin MT, Beal MF. Mitochondrial dysfunction and oxidative stress in neurodegenerative diseases. Nature. (2006) 443:787–95. doi: 10.1038/nature05292
8. Sato N, Morishita R. The roles of lipid and glucose metabolism in modulation of beta-amyloid, tau, and neurodegeneration in the pathogenesis of Alzheimer disease. Front Aging Neurosci. (2015) 7:199. doi: 10.3389/fnagi.2015.00199
9. Bateman RJ, Xiong C, Benzinger TL, Fagan AM, Goate A, Fox NC, et al. Clinical and biomarker changes in dominantly inherited Alzheimer's disease. N Engl J Med. (2012) 367:795–804. doi: 10.1056/NEJMoa1202753
10. Bott NT, Hall A, Madero EN, Glenn JM, Fuseya N, Gills JL, et al. Face-to-face and digital multidomain lifestyle interventions to enhance cognitive reserve and reduce risk of alzheimer's disease and related dementias: a review of completed and prospective studies. Nutrients. (2019) 11:2258. doi: 10.3390/nu11092258
11. Toman J, Klimova B, Valis M. Multidomain lifestyle intervention strategies for the delay of cognitive impairment in healthy aging. Nutrients. (2018) 10:1560. doi: 10.3390/nu10101560
12. Meng X, Fang S, Zhang S, Li H, Ma D, Ye Y, et al. Multidomain lifestyle interventions for cognition and the risk of dementia: a systematic review and meta-analysis. Int J Nurs Stud. (2022) 130:104236. doi: 10.1016/j.ijnurstu.2022.104236
13. Sims R, Hill M, Williams J. The multiplex model of the genetics of Alzheimer's disease. Nat Neurosci. (2020) 23:311–22. doi: 10.1038/s41593-020-0599-5
14. Cheng L, Doecke JD, Sharples RA, Villemagne VL, Fowler CJ, Rembach A, et al. Prognostic serum miRNA biomarkers associated with Alzheimer's disease shows concordance with neuropsychological and neuroimaging assessment. Mol Psychiatr. (2015) 20:1188–96. doi: 10.1038/mp.2014.127
15. Zhao YH, Jaber V, Alexandrov PN, Vergallo A, Lista S, Hampel H, et al. microRNA-based biomarkers in Alzheimer's disease (AD). Front Neurosci. (2020) 14:585432. doi: 10.3389/fnins.2020.585432
16. Pogue AI, Hill JM, Lukiw WJ. MicroRNA (miRNA): sequence and stability, viroid-like properties, and disease association in the CNS. Brain Res. (2014) 1584:73–9. doi: 10.1016/j.brainres.2014.03.042
17. Lukiw WJ. Variability in micro RNA (miRNA) abundance, speciation and complexity amongst different human populations and potential relevance to Alzheimer's disease (AD). Front Cell Neurosci. (2013) 7:133. doi: 10.3389/fncel.2013.00133
18. van den Berg MMJ, Krauskopf J, Ramaekers JG, Kleinjans JCS, Prickaerts J, Briede JJ. Circulating microRNAs as potential biomarkers for psychiatric and neurodegenerative disorders. Prog Neurobiol. (2020) 185:101732. doi: 10.1016/j.pneurobio.2019.101732
19. Backes C, Meese E, Keller A. Specific miRNA disease biomarkers in blood, serum and plasma: challenges and prospects. Mol Diagn Ther. (2016) 20:509–18. doi: 10.1007/s40291-016-0221-4
20. Collino F, Deregibus MC, Bruno S, Sterpone L, Aghemo G, Viltono L, et al. Microvesicles derived from adult human bone marrow and tissue specific mesenchymal stem cells shuttle selected pattern of miRNAs. PLoS ONE. (2010) 5:e11803. doi: 10.1371/journal.pone.0011803
21. Iftikhar H, Carney GE. Evidence and potential in vivo functions for biofluid miRNAs: From expression profiling to functional testing: Potential roles of extracellular miRNAs as indicators of physiological change and as agents of intercellular information exchange. Bioessays. (2016) 38:367–78. doi: 10.1002/bies.201500130
22. Mitchell PS, Parkin RK, Kroh EM, Fritz BR, Wyman SK, Pogosova-Agadjanyan EL, et al. Circulating microRNAs as stable blood-based markers for cancer detection. Proc Natl Acad Sci USA. (2008) 105:10513–8. doi: 10.1073/pnas.0804549105
23. Kamal MA, Mushtaq G, Greig NH. Current update on synopsis of miRNA dysregulation in neurological disorders. CNS Neurol Disord Drug Targets. (2015) 14:492–501. doi: 10.2174/1871527314666150225143637
24. Roy B, Yoshino Y, Allen L, Prall K, Schell G, Dwivedi Y. Exploiting circulating MicroRNAs as biomarkers in psychiatric disorders. Mol Diagn Ther. (2020) 24:279–98. doi: 10.1007/s40291-020-00464-9
25. Alexandrov PN, Dua P, Hill JM, Bhattacharjee S, Zhao Y, Lukiw WJ. microRNA (miRNA) speciation in Alzheimer's disease (AD) cerebrospinal fluid (CSF) and extracellular fluid (ECF). Int J Biochem Mol Biol. (2012) 3:365–73.
26. Soares Martins T, Trindade D, Vaz M, Campelo I, Almeida M, Trigo G, et al. Diagnostic and therapeutic potential of exosomes in Alzheimer's disease. J Neurochem. (2021) 156:162–81. doi: 10.1111/jnc.15112
27. Nunomura A, Perry G. RNA and oxidative stress in Alzheimer's disease: focus on microRNAs. Oxid Med Cell Longev. (2020) 2020:2638130. doi: 10.1155/2020/2638130
28. Dekker J, Rippe K, Dekker M, Kleckner N. Capturing chromosome conformation. Science. (2002) 295:1306–11. doi: 10.1126/science.1067799
29. Lieberman-Aiden E, van Berkum NL, Williams L, Imakaev M, Ragoczy T, Telling A, et al. Comprehensive mapping of long-range interactions reveals folding principles of the human genome. Science. (2009) 326:289–93. doi: 10.1126/science.1181369
30. Yuan YC, Shi Y, Li CY, Kim JM, Cai WD, Han ZG, et al. DeepGene: an advanced cancer type classifier based on deep learning and somatic point mutations. BMC Bioinformat. (2016) 17:476. doi: 10.1186/s12859-016-1334-9
31. Yuan YC, Shi Y, Su XB, Zou X, Luo Q, Feng DD, et al. Cancer type prediction based on copy number aberration and chromatin 3D structure with convolutional neural networks. BMC Genom. (2018) 19:565. doi: 10.1186/s12864-018-4919-z
33. Kern F, Fehlmann T, Solomon J, Schwed L, Grammes N, Backes C, et al. miEAA 20: integrating multi-species microRNA enrichment analysis and workflow management systems. Nucleic Acids Res. (2020) 48:W521–8. doi: 10.1093/nar/gkaa309
34. Shi Y, Guo Z, Su X, Meng L, Zhang M, Sun J, et al. DeepAntigen: a novel method for neoantigen prioritization via 3D genome and deep sparse learning. Bioinformatics. (2020) 36:4894–901. doi: 10.1093/bioinformatics/btaa596
35. Dixon JR, Selvaraj S, Yue F, Kim A, Li Y, Shen Y, et al. Topological domains in mammalian genomes identified by analysis of chromatin interactions. Nature. (2012) 485:376–80. doi: 10.1038/nature11082
36. Perkovic MN, Paska AV, Konjevod M, Kouter K, Strac DS, Erjavec GN, et al. Epigenetics of Alzheimer's disease. Biomolecules. (2021) 11:195. doi: 10.3390/biom11020195
37. Szulwach KE Li XK, Smrt RD Li YJ, Luo YP, Lin L, et al. Cross talk between microRNA and epigenetic regulation in adult neurogenesis. J Cell Biol. (2010) 189:127–41. doi: 10.1083/jcb.200908151
38. Geng L, Zhang T, Liu W, Chen Y. Inhibition of miR-128 abates abeta-mediated cytotoxicity by targeting PPAR-gamma via NF-kappaB inactivation in primary mouse cortical neurons and neuro2a cells. Yonsei Med J. (2018) 59:1096–106. doi: 10.3349/ymj.2018.59.9.1096
39. Deng M, Zhang Q, Wu Z, Ma T, He A, Zhang T, et al. Mossy cell synaptic dysfunction causes memory imprecision via miR-128 inhibition of STIM2 in Alzheimer's disease mouse model. Aging Cell. (2020) 19:e13144. doi: 10.1111/acel.13144
40. Hamada N, Fujita Y, Kojima T, Kitamoto A, Akao Y, Nozawa Y, et al. MicroRNA expression profiling of NGF-treated PC12 cells revealed a critical role for miR-221 in neuronal differentiation. Neurochem Int. (2012) 60:743–50. doi: 10.1016/j.neuint.2012.03.010
41. Li JJ, Dolios G, Wang R, Liao FF. Soluble beta-amyloid peptides, but not insoluble fibrils, have specific effect on neuronal microRNA expression. PLoS ONE. (2014) 9:e90770. doi: 10.1371/journal.pone.0090770
42. Aristizabal MJ, Anreiter I, Halldorsdottir T, Odgers CL, McDade TW, Goldenberg A, et al. Biological embedding of experience: a primer on epigenetics. Proc Natl Acad Sci USA. (2020) 117:23261–9. doi: 10.1073/pnas.1820838116
43. Wakabayashi T, Hidaka R, Fujimaki S, Asashima M, Kuwabara T. MicroRNAs and epigenetics in adult neurogenesis. Adv Genet. (2014) 86:27–44. doi: 10.1016/B978-0-12-800222-3.00002-4
44. Yao Q, Chen Y, Zhou X. The roles of microRNAs in epigenetic regulation. Curr Opin Chem Biol. (2019) 51:11–7. doi: 10.1016/j.cbpa.2019.01.024
45. Fabbri M, Calin GA. Epigenetics and miRNAs in human cancer. Adv Genet. (2010) 70:87–99. doi: 10.1016/B978-0-12-380866-0.60004-6
46. Cheng Y, Wang ZM, Tan W, Wang X, Li Y, Bai B, et al. Partial loss of psychiatric risk gene Mir137 in mice causes repetitive behavior and impairs sociability and learning via increased Pde10a. Nat Neurosci. (2018) 21:1689–703. doi: 10.1038/s41593-018-0261-7
47. Benetti R, Gonzalo S, Jaco I, Munoz P, Gonzalez S, Schoeftner S, et al. A mammalian microRNA cluster controls DNA methylation and telomere recombination via Rbl2-dependent regulation of DNA methyltransferases. Nat Struct Mol Biol. (2008) 15:998. doi: 10.1038/nsmb0908-998b
48. Sinkkonen L, Hugenschmidt T, Berninger P, Gaidatzis D, Mohn F, Artus-Revel CG, et al. MicroRNAs control de novo DNA methylation through regulation of transcriptional repressors in mouse embryonic stem cells. Nat Struct Mol Biol. (2008) 15:259–67. doi: 10.1038/nsmb.1391
Keywords: microRNA, biomarkers, 3D genome, Alzheimer's disease, machine learning
Citation: Li K, Chi R, Liu L, Feng M, Su K, Li X, He G and Shi Y (2023) 3D genome-selected microRNAs to improve Alzheimer's disease prediction. Front. Neurol. 14:1059492. doi: 10.3389/fneur.2023.1059492
Received: 01 October 2022; Accepted: 16 January 2023;
Published: 13 February 2023.
Edited by:
Pedro Gomez-Vilda, Polytechnic University of Madrid, SpainReviewed by:
Weitian Tong, Georgia Southern University, United StatesXiao Zhi, Shanghai Jiao Tong University, China
Cinzia Coppola, Università della Campania Luigi Vanvitelli, Italy
Copyright © 2023 Li, Chi, Liu, Feng, Su, Li, He and Shi. This is an open-access article distributed under the terms of the Creative Commons Attribution License (CC BY). The use, distribution or reproduction in other forums is permitted, provided the original author(s) and the copyright owner(s) are credited and that the original publication in this journal is cited, in accordance with accepted academic practice. No use, distribution or reproduction is permitted which does not comply with these terms.
*Correspondence: Guang He, heguang@sjtu.edu.cn; Yi Shi,
yishi@sjtu.edu.cn
†These authors have contributed equally to this work and share first authorship