- 1Second Clinical Medical School, Heilongjiang University of Chinese Medicine, Harbin, Heilongjiang, China
- 2Department of Acupuncture, The Second Affiliated Hospital of Heilongjiang University of Chinese Medicine, Harbin, Heilongjiang, China
- 3Department of Acupuncture, The Third Affiliated Hospital of Zhejiang Chinese Medical University, Hangzhou, Zhejiang, China
- 4Department of Neurorehabilitation, Taizhou Enze Medical Center Luqiao Hospital, Taizhou, Zhejiang, China
Background: Recent studies have reported that machine learning (ML), with a relatively strong capacity for processing non-linear data and adaptive ability, could improve the accuracy and efficiency of prediction. The article summarizes the published studies on ML models that predict motor function 3–6 months post-stroke.
Methods: A systematic literature search was conducted in PubMed, Embase, Cochorane and Web of Science as of April 3, 2023 for studies on ML prediction of motor function in stroke patients. The quality of the literature was assessed using the Prediction model Risk Of Bias Assessment Tool (PROBAST). A random-effects model was preferred for meta-analysis using R4.2.0 because of the different variables and parameters.
Results: A total of 44 studies were included in this meta-analysis, involving 72,368 patients and 136 models. Models were categorized into subgroups according to the predicted outcome Modified Rankin Scale cut-off value and whether they were constructed based on radiomics. C-statistics, sensitivity, and specificity were calculated. The random-effects model showed that the C-statistics of all models were 0.81 (95% CI: 0.79; 0.83) in the training set and 0.82 (95% CI: 0.80; 0.85) in the validation set. According to different Modified Rankin Scale cut-off values, C-statistics of ML models predicting Modified Rankin Scale>2(used most widely) in stroke patients were 0.81 (95% CI: 0.78; 0.84) in the training set, and 0.84 (95% CI: 0.81; 0.87) in the validation set. C-statistics of radiomics-based ML models in the training set and validation set were 0.81 (95% CI: 0.78; 0.84) and 0.87 (95% CI: 0.83; 0.90), respectively.
Conclusion: ML can be used as an assessment tool for predicting the motor function in patients with 3–6 months of post-stroke. Additionally, the study found that ML models with radiomics as a predictive variable were also demonstrated to have good predictive capabilities. This systematic review provides valuable guidance for the future optimization of ML prediction systems that predict poor motor outcomes in stroke patients.
Systematic review registration: https://www.crd.york.ac.uk/prospero/display_record.php?ID=CRD42022335260, identifier: CRD42022335260.
1. Introduction
Stroke is an acute cerebrovascular disease caused by sudden rupture of intracranial vessels or vascular obstruction preventing blood from flowing into the brain and thereby leading to brain tissue damage. Based on its pathological pattern, stroke can be classified into ischemic stroke (IS) and hemorrhagic stroke (HS). The Global Burden of Diseases, Injuries, and Risk Factors Study 2017 (GBD 2017) reported that stroke resulted in 6.17 million deaths and is the second leading cause of death and disability worldwide (1). According to the 2021 Guideline for the Prevention of Stroke in Patients With Stroke and Transient Ischemic Attack From the American Stroke Association (ASA), high blood pressure, diet, abdominal obesity, physical inactivity and smoking represent 82% (2) of the population-attributable risk (PAR) in patients with IS and HS. Although most IS patients have received effective treatments, many of them still suffer certain functional impairment after treatment. Motor function outcome in stroke survivors, as a primary determinant of the burden of stroke, directly determines their quality of life. Furthermore, physical disability is a key factor for the occurrence of mental disorders, such as depression, which occurs in 33% of stroke survivors (3). Therefore, the motor function-related outcome is one of the greatest concerns for stroke patients and their families. Clinically, it is extremely significant for clinicians to judge the prognosis of stroke patients and make a long-term treatment plan for those with a poor motor function outcome (4).
With rapid advances in medical and health informatization, medical data in a larger scale can be divided into more types, and the health care field has also entered a new era of big data. Due to the large scale, diversified types and high hidden value of medical data, ML algorithms have been widely used in the medical field (5–8). ML can be defined as a subfield of artificial intelligence (AI) that uses computerized algorithms to automatically improve performance through an iterative learning process or experience (i.e., data collection) (9). Different from traditional prediction models that use selected variables for calculation, ML techniques can easily incorporate a large number of variables to describe the complex and unpredictable nature of human physiology in a clearer way. Therefore, ML may be helpful for clinical prediction and identification of new prognostic markers (10). In recent years, many ML methods have been applied to the diagnosis and assessment of stroke (11, 12), including the evaluation of stroke severity (13), analysis of cerebral edema (14), prediction of hematoma expansion (15), and incidence prediction (16). Therefore, ML model predictions not only aid in disease analysis, prevention, diagnosis, and patient monitoring, but also help clinicians handle massive amounts of data in a more accurate and efficient manner (17).
In the literature, the published systematic reviews lack ML model prediction analysis of motor function 3–6 months post-stroke, especially regarding model predictive capabilities in different outcome cut-off values to accurately determine efficacy of ML predictions. Additionally, individual original studies may not be able to statistically assess the robustness of prediction results. Therefore, this systematic review and meta-analysis was conducted to assess the performance of current ML models as clinical tools for predicting medium- and long-term recovery of motor function in stroke patients.
2. Methods
This systematic review and meta-analysis was performed in accordance with the Preferred Reporting Items for Systematic Reviews and Meta-Analyses 2020 (PRISMA 2020), and prospectively registered in PROSPERO (CRD42022335260).
2.1. Search strategy
A comprehensive and systematic search was conducted in PubMed, Embase, Cochorane, and Web of Science databases. The retrieval was as of April 3, 2023. A researcher (Xiaoning Li) designed the keywords and search strategy of this systematic review, and both subject headings and free words were searched. The complete search strategy can be found in the Supplementary Table S1.
2.2. Inclusion and exclusion criteria
2.2.1. Inclusion criteria
(1) Patients were diagnosed with IS or HS on CT or MRI. IS included large vessel occlusion, anterior circulation infarction and posterior circulation infarction. (2) ML was used to predict motor function in patients 3–6 months post-stroke.
(3) The Modified Rankin Scale was used as the outcome measure. (4) Aged 18 and older. (5) Articles written in English or translated into English. (6) Randomized controlled trials, cohort studies, case-control studies.
2.2.2. Exclusion criteria
(1) Patients were clumsy in physical activities or unable to function independently before stroke (Premorbid Modified Rankin Scale ≥ 2). (2) Cerebral hemorrhage resulted from secondary causes, such as cerebral trauma and subarachnoid hemorrhage. (3) Prediction models applied clinical scoring rather than ML. (4) Case reports, protocols, editorials, and perspectives that have no original data.
2.3. Literature screening and data extraction
All of the retrieved studies were imported into Endnote for management. After automatic and manual removal of duplicates, two researchers (Weiying Zhao and Xue Zheng) independently assessed remaining articles. Titles and abstracts were preliminarily screened before the full texts were downloaded. Then we read the full texts to select eligible studies that meet the inclusion criteria. If there was any dissent on a study, a third researcher (Lei Chi) was consulted to assist in determining whether to include it. Before data extraction, a sheet of standard data extraction was prepared, including data source, Modified Rankin Scale cut-off value that was defined as a poor outcome, outcome prediction time, missing data processing methods, sample sizes of the training and validation sets, validation set confirmation way, internal and external validation information, predictors and their number, as well as ML model types. Data of the accuracy metrics were also collected, including sensitivity, specificity, receiver operator characteristic (ROC), area under the curve (AUC) and other.
2.4. Quality analysis
The ROB of included studies was assessed using PROBAST (18). It involves four major domains: participants, predictors, outcomes and statistic analysis, and reflects the overall ROB and applicability. The four domains include two, three, six and nine signaling questions, respectively. Signaling questions are answered as yes/probable yes (Y/PY), no/probably no (N/PN), or no information (NI). If a domain is answered with at least a N/PN, it is considered at high ROB. When all of the four domains were rated as low ROB, the overall ROB is deemed to be low. Two researchers (CJ and KZ) independently carried out ROB assessment in accordance with PROBAST. Then their assessment results were cross checked. Any dissent was consulted to a third researcher (LW) for final determination.
2.5. Data analysis
We performed a meta-analysis of the metrics (C-statistics and accuracy) for evaluating ML models. If C-statistic lacked 95% confidence interval (CI) and standard error (SE), we referred to the study by Debray TP et al. (19) to estimate its standard error. In case of inaccurate original data, we calculated based on sensitivity and specificity in combination with the sample size of each molecular subtype and model. Given the difference in variables and parameters in ML models, a random-effects model was preferred to perform the meta-analysis. This meta-analysis was conducted using R4.2.0 (R development Core Team, Vienna, http://www.R-project.org). A subgroup analysis by ML models and Modified Rankin Scale threshold was performed in our systematic review and meta-analysis. Heterogeneity was quantified by calculating I2 as a percentage. A low level of heterogeneity was present when I2 was 25%, a moderate level when I2 was 50% and a high level when I2 was 75% (20). Publication bias was examined by creating a funnel plot and Begg's bias test.
3. Results
3.1. Literature search
A total of 23,594 articles were initially searched from PubMed, Embase, Cochorane and Web of Science. In the screening process, not Modified Rankin Scale outcome, Modified Rankin Scale outcome time <90 days, not clearly identify prediction standard all exclusion conditions. After screening, a total of 44 papers were eligible (21–64). The selection process is shown in Figure 1.
3.2. Characteristics of included studies
A total of 44 eligible studies were included in this systematic review, involving 72,368 patients and 136 prediction models. All prediction models were internally validated, and 26 of them were externally validated. Additionally, among the 28 eligible studies, 15 studies were multi-centered (27–29, 39, 45, 47, 52, 55, 58–64); five studies extracted their original data from databases (24, 25, 32, 37, 57); and the remaining 24 studies were single-centered. Most eligible studies focused on IS and 7 studies (23, 32, 39, 40, 48, 60, 63) on HS. A study (32) used the same four models to predict the motor function in patients with IS and HS respectively. The primary outcome was Modified Rankin Scale at 3 to 6 months after the onset of stroke. Due to distinct purposes, these included studies defined poor motor function in a different manner. Specifically, it was defined as Modified Rankin Scale >1 in 4 studies (26, 31, 45, 58), Modified Rankin Scale >3 in 4 (39, 53, 60, 63) studies, Modified Rankin Scale >4 in two (25, 42) studies, and Modified Rankin Scale >2 in other 34 studies. In this study, a total of 13 primary studies using radiomics as predictive factors were identified (27, 30, 36, 37, 49, 51, 52, 54–57, 61, 62), from which 33 prediction models were extracted. Among them, 20 models primarily used MRI images as predictive factors.
In the training set, models of LR (Logistic Regression), RF (Random Forest), SVM (Support Vector Machine), XGB (Extreme Gradient Boosting), ANN (Artificial Neural Networks), DT (Decision Tree), GBM (Gradient Boosting Machine), DNN (Deep Neural Network), ADB (Adaptive Boosting), KNN (K-nearest Neighbors), CNN (Convolutional Neural Network) were applied. C-statistic was used for analysis. Meanwhile, the sensitivity and specificity of LR, RF, SVM, XGB, ANN, DT, ADB, CNN, Naive Bayes were calculated. In contrast, the validation set applied LR, ANN, RF, SVM, XGB, DT, GBM, KNN and GLM and used C-statistics to evaluate these models. The sensitivity and specificity of LR, RF, SVM, XGB, ANN, DT, and KNN were also calculated. Furthermore, these included studies were published from 2017 to 2023, which generally shows an increasing trend year by year. This reflects the rising popularity of ML prediction. Characteristics of included studies are presented in the Supplementary Tables S2–S4.
3.3. Quality assessment
In terms of the evaluation of case source, 99 of 136 models came from retrospective case-control studies, which was rated as high ROB. The high bias of data in retrospective studies caused limited accuracy of models in prediction. Thus these models were at high ROB. The other 37 models extracted clinical data from prospective cohort studies or registration data platforms, thereby rated at low ROB. Regarding the assessment of predictors, 67 models were rated at high ROB. Since researchers knew both predictors and data results in retrospective studies, there was a high ROB in such studies. For the outcome, 109 models were rated at low ROB, and 22 models were at unclear ROB for failing to report whether the predictor information was unclear at the time of outcome determination. Lastly, as for the analysis, 88 models were at high ROB, among them 60 models were rated at high ROB because of sample size <100 or events per variable(EPV) <10, and 43 models at unclear ROB due to a failure to elucidate the processing method of missing data, data complexity and optimal fitting method. The ROB evaluation is presented in Figure 2.
3.4. Variable ordering
The present study summarized and ordered the predictors in included models. All predictors were divided into five categories, which comprehensively covered all-round information of stroke patients. This was helpful for clinicians to provide targeted secondary prevention and health guidance for corresponding patients in the future. Among all predictors, age (patient demographics) was used most frequently in prediction models, followed by initial NIHSS (clinical variables), glucose level (laboratory values), initial Modified Rankin Scale (clinical variables). In terms of the medication history, the frequency of thrombolysis treatment was high (shown in Figure 3).
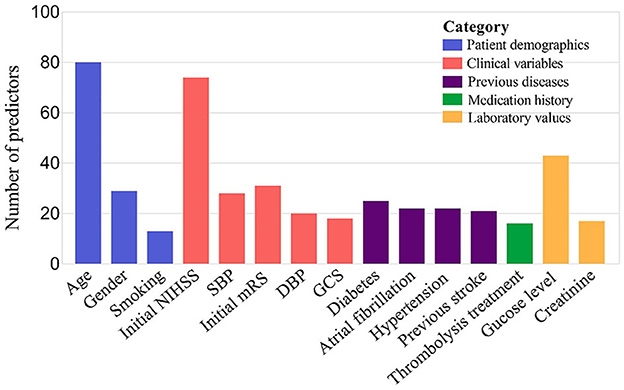
Figure 3. TOP 15 predictors 136 prognostic models for long-term post-stroke ML models. SBP, systolic blood pressure; DBP, diastolic blood pressure; GCS, Glasgow Coma Scale.
3.5. Machine learning outcomes
Firstly, a random-effects model was used to combine the C-statistics in ML models. The overall C-statistic for the 96 models in the training set was 0.81 (95% CI: 0.79; 0.83). The 96 models can be classified into 12 types, which were ordered according to their frequency of application to prediction. Among the 12 model types, the LR model was used 29 times in all eligible studies to predict post-stroke motor function, and the C-statistic was 0.81 (95% CI: 0.78; 0.85). ANN had the best performance in prediction with a C-statistic of 0.91 (95% CI: 0.86; 0.95). The overall C-statistic for 71 models in the validation set was 0.82 (95% CI: 0.80; 0.85). The 71 models were divided into 10 types, which were also ranked according to their application frequency in prediction. Among the nine model types, LR models were used most frequently, namely 20 times, to predict post-stroke motor function, with a C-statistic of 0.82 (95% CI: 0.78; 0.87). The performance of each model can be seen in Table 1 and Figure 4.
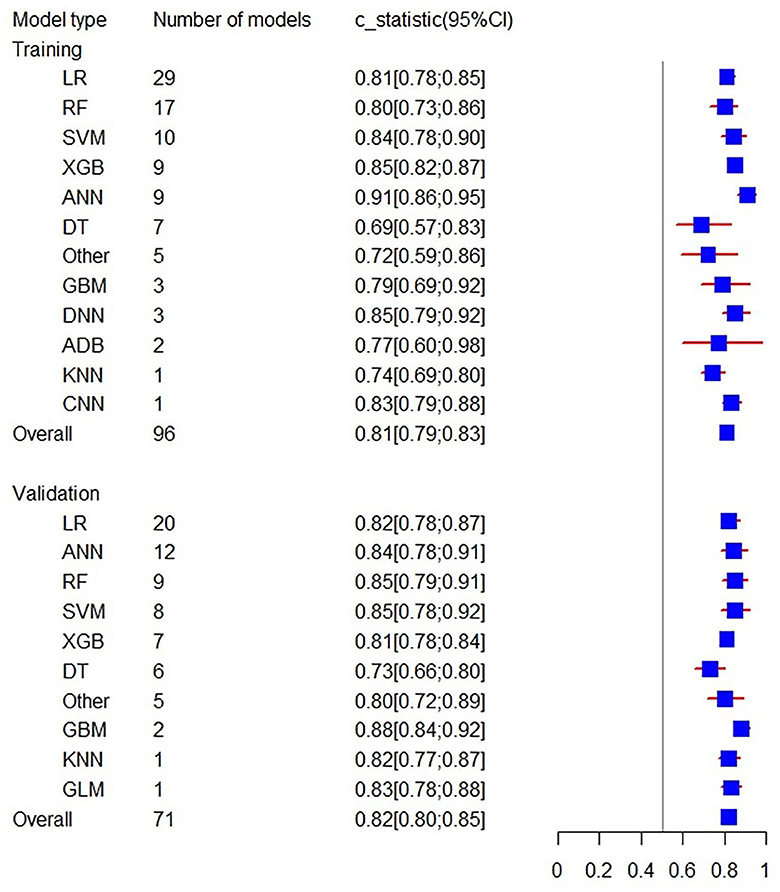
Figure 4. Forest plot of the overall C-statistics predicting 3–6 m Modified Rankin Scale outcomes in stroke patients.
To minimize the heterogeneity of data sources, the present study also performed C-statistic pooling based on different Modified Rankin Scale cutoff thresholds. Among the training set models with Modified Rankin Scale >2, there were 85 in total with a C-statistic of 0.81 (95% CI: 0.78; 0.84), and among the validation set models, there were 48 in total with a C-statistic of 0.84 (95% CI: 0.81; 0.87). In both the prediction model of training and validation sets, the ANN prediction performed the best with C-statistics of 0.91 (95% CI: 0.86; 0.95) and 0.89 (95% CI: 0.83; 0.96), respectively, as shown in Figure 5. For models with Modified Rankin Scale > 1, there were 6 training set models, with an overall C-statistic of 0.78 (95% CI: 0.77; 0.80). There were 14 validation set models, with an overall C-statistic of 0.79 (95% CI: 0.77; 0.82). For models with Modified Rankin Scale >3, there were 5 training set models, with an overall C-statistic of 0.83 (95% CI: 0.78; 0.88). There were 4 validation set models, all of which were LR models, with an overall C-statistic of 0.87 (95% CI: 0.82; 0.92). There were no training set models with Modified Rankin Scale > 4, but there were 5 validation set models, with an overall C-statistic of 0.79 (95% CI: 0.78; 0.81). The performance of each model within the subgroups can be seen in Table 2.
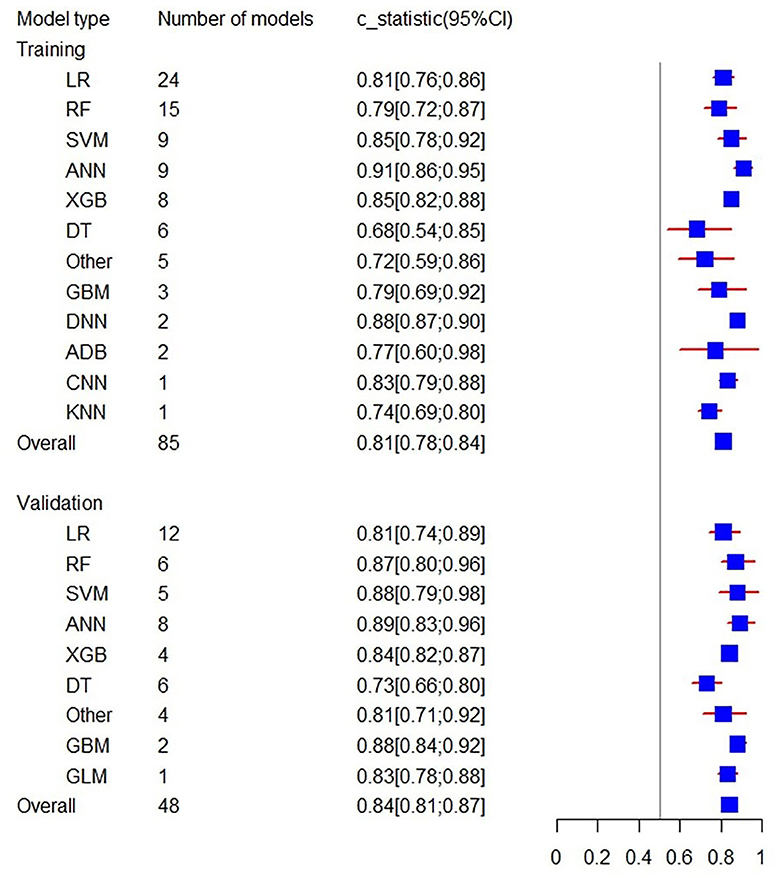
Figure 5. Forest plot of the C-statistics predicting 3–6 m Modified Rankin Scale >2 outcomes in stroke patients.
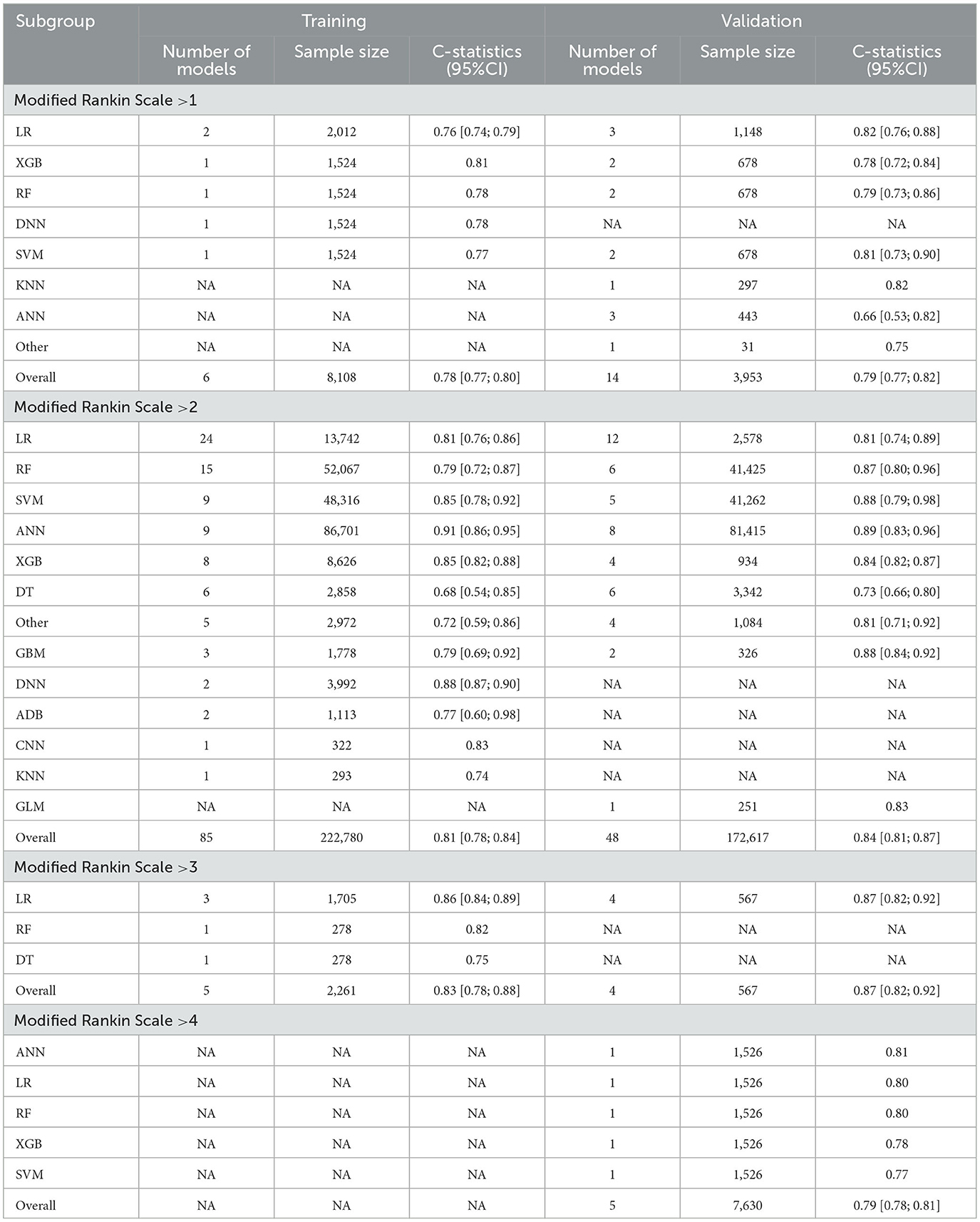
Table 2. C-statistics of subgroups machine learning models (according to threshold of Modified Rankin Scale).
Additionally, the C-statistics were also combined for radiomics-based machine learning prediction models. The training set included a total of 20 prediction models from 8 categories. The overall C-statistic was 0.81 (95% CI: 0.78; 0.84). The most numerous type was the LR model, which also had the best predictive performance with a C-statistic of 0.86 (95% CI: 0.82; 0.91). There were 13 validation set models, with an overall C-statistic of 0.87 (95% CI: 0.83; 0.90). Similarly, the LR model had the best predictive performance with a C-statistic of 0.91 (95% CI: 0.88; 0.95). The performance of the remaining models is provided in Table 3 and Figure 6.
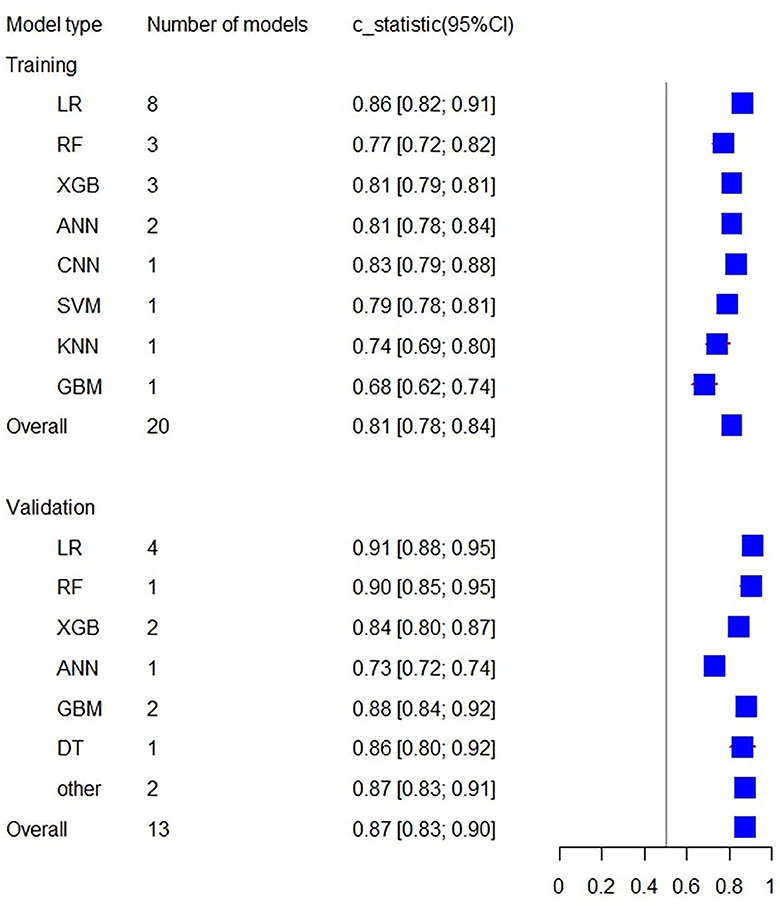
Figure 6. Forest plot of the C-statistics predicting 3–6 m Modified Rankin Scale outcomes in stroke patients (radiomics-based predictors).
3.6. Sensitivity and specificity
In order to avoid data imbalance, the sensitivity and specificity of models in prediction were analyzed. The training set included 39 models in total with the overall sensitivity of 0.72 (95% CI: 0.70; 0.75). LR were used 15 times, with a sensitivity of 0.74 (95% CI: 0.67; 0.79). Additionally, the overall specificity was 0.77 (95% CI: 0.74; 0.80). The validation set included 40 models, and the overall sensitivity and specificity were 0.74 (95%CI: 0.69; 0.79) and 0.72 (95% CI: 0.66; 0.77), respectively. Sensitivity and specificity analyses were also conducted for subgroups with different Modified Rankin Scale thresholds. The sensitivity and specificity of ML models and subgroups were presented in Table 4 and Figure 7.
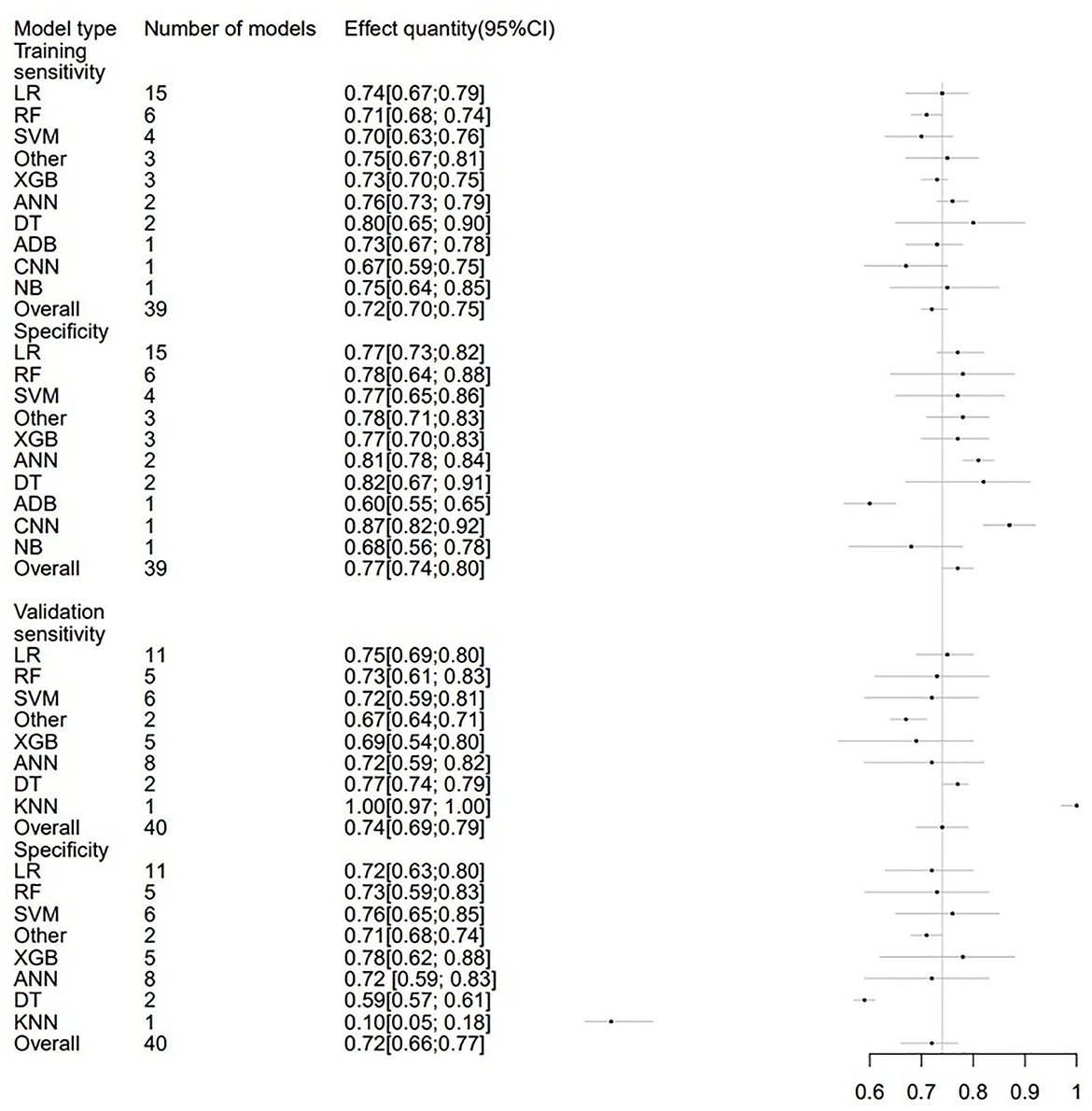
Figure 7. Forest plot of the sensitivity and specificity predicting 3–6 m Modified Rankin Scale outcomes in stroke patients.
3.7. Publication bias
In the meta-analysis of C-statistic, no publication bias was found in the funnel plots for both the training set and validation set of ML models for predicting motor function 3–6 months after stroke. The results of Begg test showed that P = 0.473 in the training set, P = 0.909 in the verification set. The funnel plots are shown in Figures 8, 9. In the meta-analysis of the diagnostic 4-fold table, there was publication bias in the training set of ML models for predicting motor function 3–6 months after stroke, while no publication bias was found in the validation set. The Beg test results showed that P = 0.01 in the training set, P = 0.31 in the validation set. The funnel plots are shown in Figures 10, 11.
4. Discussion
The study reviews the performance of ML models in the prediction of motor function recovery in patients 3–6 months post-stroke. In the case of Modified Rankin Scale >1, >2, >3, >4, the model's predictive performance was favorable. The C-statistics for models with predictive factors based on radiomics were 0.81 (95% CI: 0.78; 0.84) in the training set and 0.87 (95% CI: 0.83; 0.90) in the validation set. The overall sensitivity and specificity of the models were both over 0.70 and relatively balanced. The study makes up the gap and deficiency in current researches on the prediction of motor function recovery in stroke patients, which has significant instruction for clinical practice. In the quality assessment part, the ROB of original studies was analyzed in accordance with the PROBAST standard. This provides detailed suggestions for researchers to design model prediction tests in the future, which is conducive to standardization and unification.
According to this analysis of predictors, age and initial NIHSS, are the most critical predictors for the prognosis of motor function in patients with stroke for 90 to 180 days, followed by glucose level, initial Modified Rankin Scale. This is consistent with the results of previous studies (2, 65, 66) on prediction models and meta-analysis of predictors. A large number of studies show that aged patients with acute IS have higher mortality and poorer quality of life than their young counterparts. For instance, both cerebral infarction complicated with pulmonary infection and hemorrhagic transformation after cerebral infarction are more likely to cause relatively poor outcomes in aged patients (67, 68). NIHSS score has been widely recognized as a key determinant of the prognosis in patients with acute IS in China and abroad (69). Furthermore, a previous study (70) on motor function outcome in stroke patients show that initial measures were found to be the most significant predictors of upper limb recovery; odds ratio 14.84 (95% CI 9.08–24.25) and 38.62 (95% CI 8.40–177.53) respectively.
AI has been widely applied in the diagnosis, classification, and prediction of stroke. One of its biggest advantages is that it can process data endlessly and can perform faster than traditional computer-aided detection and diagnosis (CAD) (71). Although there are more and more studies on post-stroke motor function prognosis, there is still a lack of prediction and guidance for neurological motor function, especially for model evaluation after stratifying prediction outcomes. A previous systematic review (72) collected and summarized clinical prognosis trials for patients with large vessel occlusion undergoing thrombectomy, and predicted 90-day Modified Rankin Scale for 802 patients. The random-effects model showed an AUC of 0.846 (95% CI 0.686–0.902), indicating good predictive performance. However, as most of the limited number of included studies used SVM, a comprehensive comparison of the predictive performance of various models to determine the best model was not possible. Similarly, earlier research (73) in rehabilitation medicine on the prediction of post-stroke function recovery confirmed the application of ML prediction ability in clinical settings but did not provide specific C-statistic values, making it difficult to accurately assess predictive performance. Therefore, conducting a meta-analysis of ML motor function prediction models classified by prognosis outcome and predictive factors is both necessary and valuable, as it deepens the understanding of earlier research and further clarifies the ideal application value of ML in predicting post-stroke outcomes.
For stroke prediction, most existing ML algorithms use binary classification to evaluate the outcome indicator. Conventionally, when Modified Rankin Scale are 0–2, functional outcomes are usually defined as “good”; when Modified Rankin Scale 3–6, functional outcomes are typically defined as “poor.” Studies usually measure Modified Rankin Scale at 90 days post-stroke (38, 74, 75). However, with different patient situations in clinical settings and various research objectives, studies have started using Modified Rankin Scale threshold values such as 0–1 VS 2–6, 0–3 VS 4–6, and conducting later follow-ups. New ML algorithms that incorporate these results will provide greater assistance to clinicians.
The advancement of machine learning has made it possible to transform subjective visual interpretation into objective evaluation driven by image data. Radiomics has emerged in this context. Radiomics is a computer-aided process that can extract a large number of quantitative features from biomedical images in an objective, repeatable, and high-throughput manner (76, 77). These features can be combined with other medical information such as demographics, clinical, histological, or genomic data to improve clinical treatment decision-making and accelerate the progress of precision medicine. A systematic review (78) reveals that the artificial intelligence coupling CNN with image feature has greater sensitivity, up to 83%. ML not only offers promising applications in medical imaging by learning information features and patterns from structured input data, but also promotes the emergence of deep learning (DL) and demonstrates its excellent performance in medical image processing (79, 80). XinruiWang's latest study (81) analyzed ML models to predict the volume of core infarct tissue in AIS patients based on basic CT or MRI imaging at admission. DL models outperformed traditional ML classifiers, with the best performance observed in DL algorithms combined with CT data. Currently, the pooled dice similarity coefficient score of the included ML models for final infarct prediction based on ML was 0.50 (95% CI 0.39–0.61).
In theory, different imaging modalities and parameters provide different diagnostic and prognostic information that can complement each other. Therefore, adjusting and optimizing parameters for multimodal imaging data in radiomics can improve the overall predictive performance. In addition, the study combined clinical data such as clinical symptom assessment, medical history, and laboratory examinations (82, 83). Multidimensional input information consisting of both imaging and clinical data has the potential to establish better prediction models, which is a direction for future research.
4.1. Limitation
However, there are some limitations to the present study that need to be considered. First, due to the different types of algorithms and parameter adjustments, there is inevitably a high degree of heterogeneity between studies. To minimize the heterogeneity, we conducted subgroup analysis according to different Modified Rankin Scale cut-off values and analyzed the performance of predictive factors based on different categories. Moreover, from the summary plot of variables, it can be observed that the predictive factors in each study are similar to some degree and selected from five dimensions. Second, although ML has enormous potential in the computing function of huge data, its “black box” characteristic restricts clinicians from trusting the ML prediction. Meanwhile, due to the instability of association between impact factors, ML model requires plenty of samples to improve its accuracy (84, 85). Third, while using the Modified Rankin Scale as a functional outcome measure can directly elucidate the functional levels, it fails to express the details of various post-stroke neurological symptoms, such as dysarthria and pure sensory stroke. At last, from the literature quality assessment summary table, retrospective case studies are in the majority, leading to a high ROB and poor performance of prediction models. Therefore, in order to avoid high ROB, future clinical studies on ML prediction should collect data from clinical registration platforms or prospective clinical studies. Additionally, the design of clinical protocol should meet the requirement of EPV ≥20 to ensure the reliability of the results of the prediction model.
5. Conclusion
In this study, we conducted a systematic review and meta-analysis of the current research using ML algorithms to predict post-stroke motor function 3–6 months. Due to its good predictive performance, sensitivity, and specificity, ML can be used as an evaluation tool for predicting motor function after stroke. Additionally, the study found that ML models with radiomics as predictive variables also demonstrated good predictive capabilities. The multidimensional input information consisting of both imaging and clinical data has the potential to establish better prediction models that can guide clinical work.
Data availability statement
The original contributions presented in the study are included in the article/Supplementary material, further inquiries can be directed to the corresponding author.
Author contributions
QL wrote the main manuscript and fully participated in all analyses. XL, LC, and LW contributed to the study concept and design. WZ, CJ, XZ, and KZ participated in literature search, data extraction, and quality assessment. All authors have read and approved the final manuscript.
Conflict of interest
The authors declare that the research was conducted in the absence of any commercial or financial relationships that could be construed as a potential conflict of interest.
Publisher's note
All claims expressed in this article are solely those of the authors and do not necessarily represent those of their affiliated organizations, or those of the publisher, the editors and the reviewers. Any product that may be evaluated in this article, or claim that may be made by its manufacturer, is not guaranteed or endorsed by the publisher.
Supplementary material
The Supplementary Material for this article can be found online at: https://www.frontiersin.org/articles/10.3389/fneur.2023.1039794/full#supplementary-material
References
1. Global regional, and national incidence, prevalence, and years lived with disability for 328 diseases and injuries for 195 countries, 1990–2016: 1990–2016: a systematic analysis for the Global Burden of Disease Study 2016. Lancet. (2017) 390:1211–59. doi: 10.1016/S0140-6736(17)32154-2
2. Kleindorfer DO, Towfighi A, Chaturvedi S, Cockroft KM, Gutierrez J, Lombardi-Hill D, et al. Guideline for the prevention of stroke in patients with stroke and transient ischemic attack: a guideline from the American heart association/American stroke association. Stroke. (2021) 52:e364–467. doi: 10.1161/STR.0000000000000375
3. Paolucci S, Iosa M, Coiro P, Venturiero V, Savo A, De Angelis D, et al. Post-stroke depression increases disability more than 15% in ischemic stroke survivors: a case-control study. Front Neurol. (2019) 10:926. doi: 10.3389/fneur.2019.00926
4. Hung MC, Hsieh CL, Hwang JS, Jeng JS, Wang JD. Estimation of the long-term care needs of stroke patients by integrating functional disability and survival. PLoS ONE. (2013) 8:e75605. doi: 10.1371/journal.pone.0075605
5. Shatte ABR, Hutchinson DM, Teague SJ. Machine learning in mental health: a scoping review of methods and applications. Psychol Med. (2019) 49:1426–48. doi: 10.1017/S0033291719000151
6. Li B, Feridooni T, Cuen-Ojeda C, Kishibe T, de Mestral C, Mamdani M, et al. Machine learning in vascular surgery: a systematic review and critical appraisal. NPJ Dig Med. (2022) 5:7. doi: 10.1038/s41746-021-00552-y
7. Windisch P, Koechli C, Rogers S, Schröder C, Förster R, Zwahlen DR, et al. Machine learning for the detection and segmentation of benign tumors of the central nervous system: a systematic review. Cancers. (2022) 14:2676. doi: 10.3390/cancers14112676
8. Jiang F, Jiang Y, Zhi H, Dong Y, Li H, Ma S, et al. Artificial intelligence in healthcare: past, present and future. Stroke Vas Neurol. (2017) 2:230–43. doi: 10.1136/svn-2017-000101
9. Mainali S, Darsie ME, Smetana KS. Machine learning in action: stroke diagnosis and outcome prediction. Front Neurol. (2021) 12:734345. doi: 10.3389/fneur.2021.734345
10. Rubbert C, Patil KR, Beseoglu K, Mathys C, May R, Kaschner MG, et al. Prediction of outcome after aneurysmal subarachnoid haemorrhage using data from patient admission. Eur Radiol. (2018) 28:4949–58. doi: 10.1007/s00330-018-5505-0
11. Ni Y, Alwell K, Moomaw CJ, Woo D, Adeoye O, Flaherty ML, et al. Towards phenotyping stroke: leveraging data from a large-scale epidemiological study to detect stroke diagnosis. PLoS ONE. (2018) 13:e0192586. doi: 10.1371/journal.pone.0192586
12. Arbabshirani MR, Fornwalt BK, Mongelluzzo GJ, Suever JD, Geise BD, Patel AA, et al. Advanced machine learning in action: identification of intracranial hemorrhage on computed tomography scans of the head with clinical workflow integration. NPJ Dig Med. (2018) 1:9. doi: 10.1038/s41746-017-0015-z
13. Park E, Lee K, Han T, Nam HS. Automatic grading of stroke symptoms for rapid assessment using optimized machine learning and 4-limb kinematics: clinical validation study. J Med Internet Res. (2020) 22:e20641. doi: 10.2196/20641
14. Dhar R, Chen Y, An H, Lee JM. Application of machine learning to automated analysis of cerebral edema in large cohorts of ischemic stroke patients. Front Neurol. (2018) 9:687. doi: 10.3389/fneur.2018.00687
15. Liu J, Xu H, Chen Q, Zhang T, Sheng W, Huang Q, et al. Prediction of hematoma expansion in spontaneous intracerebral hemorrhage using support vector machine. EBioMedicine. (2019) 43:454–9. doi: 10.1016/j.ebiom.2019.04.040
16. Arslan AK, Colak C, Sarihan ME. Different medical data mining approaches based prediction of ischemic stroke. Comput Methods Programs Biomed. (2016) 130:87–92. doi: 10.1016/j.cmpb.2016.03.022
17. Patel UK, Anwar A, Saleem S, Malik P, Rasul B, Patel K, et al. Artificial intelligence as an emerging technology in the current care of neurological disorders. J Neurol. (2021) 268:1623–42. doi: 10.1007/s00415-019-09518-3
18. Moons KGM, Wolff RF, Riley RD, Whiting PF, Westwood M, Collins GS, et al. PROBAST: a tool to assess risk of bias and applicability of prediction model studies: explanation and elaboration. Ann Intern Med. (2019) 170:W1–w33. doi: 10.7326/M18-1377
19. Debray TP, Damen JA, Riley RD, Snell K, Reitsma JB, Hooft L, et al. A framework for meta-analysis of prediction model studies with binary and time-to-event outcomes. Stat Methods Med Res. (2019) 28:2768–86. doi: 10.1177/0962280218785504
20. Higgins JP, Thompson SG, Deeks JJ, Altman DG. Measuring inconsistency in meta-analyses. BMJ. (2003) 327:557–60. doi: 10.1136/bmj.327.7414.557
21. Zihni E, Madai VI, Livne M, Galinovic I, Khalil AA, Fiebach JB, et al. Opening the black box of artificial intelligence for clinical decision support: a study predicting stroke outcome. PLoS ONE. (2020) 15:e0231166. doi: 10.1371/journal.pone.0231166
22. Xie Y, Jiang B, Gong E, Li Y, Zhu G, Michel P, et al. JOURNAL CLUB: use of gradient boosting machine learning to predict patient outcome in acute ischemic stroke on the basis of imaging, demographic, and clinical information. AJR Am J Roentgenol. (2019) 212:44–51. doi: 10.2214/AJR.18.20260
23. Wang HL, Hsu WY, Lee MH, Weng HH, Chang SW, Yang JT, et al. Automatic machine-learning-based outcome prediction in patients with primary intracerebral hemorrhage. Front Neurol. (2019) 10:910. doi: 10.3389/fneur.2019.00910
24. van Os HJA, Ramos LA, Hilbert A, van Leeuwen M, van Walderveen MAA, Kruyt ND, et al. Predicting outcome of endovascular treatment for acute ischemic stroke: potential value of machine learning algorithms. Front Neurol. (2018) 9:784. doi: 10.3389/fneur.2018.00784
25. Ramos LA, Kappelhof M, van Os HJA, Chalos V, Van Kranendonk K, Kruyt ND, et al. Predicting poor outcome before endovascular treatment in patients with acute ischemic stroke. Front Neurol. (2020) 11:580957. doi: 10.3389/fneur.2020.580957
26. Park D, Jeong E, Kim H, Pyun HW, Kim H, Choi YJ, et al. Machine learning-based three-month outcome prediction in acute ischemic stroke: a single cerebrovascular-specialty hospital study in South Korea. Diagnostics. (2021) 11:1909. doi: 10.3390/diagnostics11101909
27. Nishi H, Oishi N, Ishii A, Ono I, Ogura T, Sunohara T, et al. Deep learning-derived high-level neuroimaging features predict clinical outcomes for large vessel occlusion. Stroke. (2020) 51:1484–92. doi: 10.1161/STROKEAHA.119.028101
28. Nishi H, Oishi N, Ishii A, Ono I, Ogura T, Sunohara T, et al. predicting clinical outcomes of large vessel occlusion before mechanical thrombectomy using machine learning. Stroke. (2019) 50:2379–88. doi: 10.1161/STROKEAHA.119.025411
29. Nezu T, Hosomi N, Yoshimura K, Kuzume D, Naito H, Aoki S, et al. Predictors of stroke outcome extracted from multivariate linear discriminant analysis or neural network analysis. J Atheroscler Thromb. (2022) 29:99–110. doi: 10.5551/jat.59642
30. Moulton E, Valabregue R, Lehéricy S, Samson Y, Rosso C. Multivariate prediction of functional outcome using lesion topography characterized by acute diffusion tensor imaging. NeuroImage Clin. (2019) 23:101821. doi: 10.1016/j.nicl.2019.101821
31. Lin X, Lin S, Cui X, Zou D, Jiang F, Zhou J, et al. Prediction-driven decision support for patients with mild stroke: a model based on machine learning algorithms. Front Neurol. (2021) 12:761092. doi: 10.3389/fneur.2021.761092
32. Lin CH, Hsu KC, Johnson KR, Fann YC, Tsai CH, Sun Y, et al. Evaluation of machine learning methods to stroke outcome prediction using a nationwide disease registry. Comput Methods Programs Biomed. (2020) 190:105381. doi: 10.1016/j.cmpb.2020.105381
33. Liang Y, Li Q, Chen P, Xu L, Li J. comparative study of back propagation artificial neural networks and logistic regression model in predicting poor prognosis after acute ischemic stroke. Open Med. (2019) 14:324–30. doi: 10.1515/med-2019-0030
34. Li X, Pan X, Jiang C, Wu M, Liu Y, Wang F, et al. Predicting 6-month unfavorable outcome of acute ischemic stroke using machine learning. Front Neurol. (2020) 11:539509. doi: 10.3389/fneur.2020.539509
35. Kim C, Lee SH, Lim JS, Kim Y, Jang MU, Oh MS, et al. Impact of 25-hydroxyvitamin d on the prognosis of acute ischemic stroke: machine learning approach. Front Neurol. (2020) 11:37. doi: 10.3389/fneur.2020.00037
36. Jiang B, Zhu G, Xie Y, Heit JJ, Chen H, Li Y, et al. Prediction of clinical outcome in patients with large-vessel acute ischemic stroke: performance of machine learning vs. SPAN-100. AJNR Am J Neuroradiol. (2021) 42:240–6. doi: 10.3174/ajnr.A6918
37. Hilbert A, Ramos LA, van Os HJA, Olabarriaga SD, Tolhuisen ML, Wermer MJH, et al. Data-efficient deep learning of radiological image data for outcome prediction after endovascular treatment of patients with acute ischemic stroke. Comput Biol Med. (2019) 115:103516. doi: 10.1016/j.compbiomed.2019.103516
38. Heo J, Yoon JG, Park H, Kim YD, Nam HS, Heo JH. Machine learning-based model for prediction of outcomes in acute stroke. Stroke. (2019) 50:1263–5. doi: 10.1161/STROKEAHA.118.024293
39. Hall AN, Weaver B, Liotta E, Maas MB, Faigle R, Mroczek DK, et al. Identifying modifiable predictors of patient outcomes after intracerebral hemorrhage with machine learning. Neurocrit Care. (2021) 34:73–84. doi: 10.1007/s12028-020-00982-8
40. Guo R, Zhang R, Liu R, Liu Y, Li H, Ma L, et al. machine learning-based approaches for prediction of patients' functional outcome and mortality after spontaneous intracerebral hemorrhage. J Personal Med. (2022) 12:112. doi: 10.3390/jpm12010112
41. Feng X, Hua Y, Zou J, Jia S, Ji J, Xing Y, et al. Intelligible models for healthcare: predicting the probability of 6-month unfavorable outcome in patients with ischemic stroke. Neuroinformatics. (2021) 3:6. doi: 10.1007/s12021-021-09535-6
42. Chiu IM, Zeng WH, Cheng CY, Chen SH, Lin CR. Using a multiclass machine learning model to predict the outcome of acute ischemic stroke requiring reperfusion therapy. Diagnostics. (2021) 11:80. doi: 10.3390/diagnostics11010080
43. Chiu HW, Huang YT, Cheng CA, editors. Using artificial neural network to predict functional recovery of patients treated by intravenous thrombolysis in acute ischemic stroke. World Congress on Medical Physics and Biomedical Engineering 2018 Springer. (2019). doi: 10.1007/978-981-10-9035-6_60
44. Chi NF, Chang TH, Lee CY, Wu YW, Shen TA, Chan L, et al. Untargeted metabolomics predicts the functional outcome of ischemic stroke. J Formos Med Assoc. (2021) 120:234–41. doi: 10.1016/j.jfma.2020.04.026
45. Bacchi S, Zerner T, Oakden-Rayner L, Kleinig T, Patel S, Jannes J. Deep Learning in the Prediction of Ischaemic Stroke Thrombolysis Functional Outcomes: A Pilot Study. Acad Radiol. (2020) 27:e19–23. doi: 10.1016/j.acra.2019.03.015
46. Alawieh A, Zaraket F, Alawieh MB, Chatterjee AR, Spiotta A. Using machine learning to optimize selection of elderly patients for endovascular thrombectomy. J Neurointerv Surg. (2019) 11:847–51. doi: 10.1136/neurintsurg-2018-014381
47. Alaka SA, Menon BK, Brobbey A, Williamson T, Goyal M, Demchuk AM, et al. Functional outcome prediction in ischemic stroke: a comparison of machine learning algorithms and regression models. Front Neurol. (2020) 11:889. doi: 10.3389/fneur.2020.00889
48. Phan TG, Chen J, Beare R, Ma H, Clissold B, Van Ly J, et al. Classification of different degrees of disability following intracerebral hemorrhage: a decision tree analysis from VISTA-ICH collaboration. Front Neurol. (2017) 8:64. doi: 10.3389/fneur.2017.00064
49. Zhang XG, Wang JH, Yang WH, Zhu XQ, Xue J, Li ZZ, et al. Nomogram to predict 3-month unfavorable outcome after thrombectomy for stroke. BMC Neurol. (2022) 22:111. doi: 10.1186/s12883-022-02633-1
50. Zhang C, Zhang W, Huang Y, Qiu J, Huang ZX, A. Dynamic nomogram to predict the 3-month unfavorable outcome of patients with acute ischemic stroke. Risk Manag Healthc Policy. (2022) 15:923–34. doi: 10.2147/RMHP.S361073
51. Yao Z, Mao C, Ke Z, Xu Y. An explainable machine learning model for predicting the outcome of ischemic stroke after mechanical thrombectomy. J Neurointervent Surg. (2022) 4:9598. doi: 10.1136/jnis-2022-019598
52. Moulton E, Valabregue R, Piotin M, Marnat G, Saleme S, Lapergue B, et al. Interpretable deep learning for the prognosis of long-term functional outcome post-stroke using acute diffusion weighted imaging. J Cereb Blood Flow Metabol. (2023) 43:198–209. doi: 10.1177/0271678X221129230
53. Ding GY, Xu JH, He JH, Nie ZY. Clinical scoring model based on age, NIHSS, and stroke-history predicts outcome 3 months after acute ischemic stroke. Front Neurol. (2022) 13:935150. doi: 10.3389/fneur.2022.935150
54. Zhou Y, Wu D, Yan S, Xie Y, Zhang S, Lv W, et al. Feasibility of a clinical-radiomics model to predict the outcomes of acute ischemic stroke. Korean J Radiol. (2022) 23:811–20. doi: 10.3348/kjr.2022.0160
55. Xu Q, Zhu Y, Zhang X, Kong D, Duan S, Guo L, et al. Clinical features and FLAIR radiomics nomogram for predicting functional outcomes after thrombolysis in ischaemic stroke. Front Neurosci. (2023) 17:1063391. doi: 10.3389/fnins.2023.1063391
56. Tao Z, Zhou F, Zhang H, Qian M. Value of MRI T2 FLAIR Vascular Hyperintensities combined with DWI ASPECTS in predicting the prognosis of acute cerebral infarction with endovascular treatment. Curr Med Imaging. (2023) 3:3813. doi: 10.2174/1573405619666230201103813
57. Ramos LA, van Os H, Hilbert A, Olabarriaga SD, van der Lugt A, Roos Y, et al. Combination of radiological and clinical baseline data for outcome prediction of patients with an acute ischemic stroke. Front Neurol. (2022) 13:809343. doi: 10.3389/fneur.2022.809343
58. Ping Z, Min L, Qiuyun L, Xu C, Qingke B. Prognostic nomogram for the outcomes in acute stroke patients with intravenous thrombolysis. Front Neurosci. (2022) 16:1017883. doi: 10.3389/fnins.2022.1017883
59. Li J, Zhu W, Zhou J, Yun W, Li X, Guan Q, et al. A Presurgical unfavorable prediction scale of endovascular treatment for acute ischemic stroke. Front Aging Neurosci. (2022) 14:942285. doi: 10.3389/fnagi.2022.942285
60. Li J, Luo D, Peng F, Kong Q, Liu H, Chen M, et al. ANAID-ICH nomogram for predicting unfavorable outcome after intracerebral hemorrhage. CNS Neurosci Ther. (2022) 28:2066–75. doi: 10.1111/cns.13941
61. Kniep HC, Elsayed S, Nawabi J, Broocks G, Meyer L, Bechstein M, et al. Imaging-based outcome prediction in posterior circulation stroke. J Neurol. (2022) 269:3800–9. doi: 10.1007/s00415-022-11010-4
62. Jabal MS, Joly O, Kallmes D, Harston G, Rabinstein A, Huynh T, et al. Interpretable machine learning modeling for ischemic stroke outcome prediction. Front Neurol. (2022) 13:884693. doi: 10.3389/fneur.2022.884693
63. Huang X, Wang D, Zhang Q, Ma Y, Li S, Zhao H, et al. Development and validation of a clinical-based signature to predict the 90-day functional outcome for spontaneous intracerebral hemorrhage. Front Aging Neurosci. (2022) 14:904085. doi: 10.3389/fnagi.2022.904085
64. Hu J, Fang Z, Lu X, Wang F, Zhang N, Pan W, et al. Influence factors and predictive models for the outcome of patients with ischemic stroke after intravenous thrombolysis: a multicenter retrospective cohort study. Oxid Med Cell Longev. (2022) 2022:3363735. doi: 10.1155/2022/3363735
65. Kwon Y, Norby FL, Jensen PN, Agarwal SK, Soliman EZ, Lip GY, et al. Association of smoking, alcohol, and obesity with cardiovascular death and ischemic stroke in atrial fibrillation: the atherosclerosis risk in communities (ARIC) study and cardiovascular health study (CHS). PLoS ONE. (2016) 11:e0147065. doi: 10.1371/journal.pone.0147065
66. Palaiodimou L, Lioutas VA, Lambadiari V, Paraskevas GP, Voumvourakis K, Tsivgoulis G. Glycemia management in acute ischemic stroke: current concepts and novel therapeutic targets. Postgrad Med. (2019) 131:423–37. doi: 10.1080/00325481.2019.1651206
67. Roy-O'Reilly M, McCullough LD. Age and sex are critical factors in ischemic stroke pathology. Endocrinology. (2018) 159:3120–31. doi: 10.1210/en.2018-00465
68. Al Khathaami AM, Al Bdah B, Alnosair A, Alturki A, Alrebdi R, Alwayili S, et al. Predictors of poor outcome in embolic stroke of undetermined source. Neurosciences. (2019) 24:164–7. doi: 10.17712/nsj.2019.3.20190005
69. Ortiz GA, Sacco RLJWEoCT. National institutes of health stroke scale (nihss). J Physiotherapy. (2007) 3:1–9. doi: 10.1002/9780471462422.eoct400
70. Coupar F, Pollock A, Rowe P, Weir C, Langhorne P. Predictors of upper limb recovery after stroke: a systematic review and meta-analysis. Clin Rehabil. (2012) 26:291–313. doi: 10.1177/0269215511420305
71. Chan S, Siegel EL. Will machine learning end the viability of radiology as a thriving medical specialty? Br J Radiol. (2019) 92:20180416. doi: 10.1259/bjr.20180416
72. Teo YH, Lim I, Tseng FS, Teo YN, Kow CS, Ng ZHC, et al. Predicting clinical outcomes in acute ischemic stroke patients undergoing endovascular thrombectomy with machine learning: a systematic review and meta-analysis. Clin Neuroradiol. (2021) 31:1121–30. doi: 10.1007/s00062-020-00990-3
73. Campagnini S, Arienti C, Patrini M, Liuzzi P, Mannini A, Carrozza MC. Machine learning methods for functional recovery prediction and prognosis in post-stroke rehabilitation: a systematic review. J Neuroeng Rehabil. (2022) 19:54. doi: 10.1186/s12984-022-01032-4
74. Brugnara G, Neuberger U, Mahmutoglu MA, Foltyn M, Herweh C, Nagel S, et al. Multimodal predictive modeling of endovascular treatment outcome for acute ischemic stroke using machine-learning. Stroke. (2020) 51:3541–51. doi: 10.1161/STROKEAHA.120.030287
75. Jang SK, Chang JY, Lee JS, Lee EJ, Kim YH, Han JH, et al. Reliability and clinical utility of machine learning to predict stroke prognosis: comparison with logistic regression. J stroke. (2020) 22:403–6. doi: 10.5853/jos.2020.02537
76. Avanzo M, Stancanello J, El Naqa I. Beyond imaging: The promise of radiomics. Physica medica: PM: an international journal devoted to the applications of physics to medicine and biology. J Italian Assoc Biomed Phys (AIFB). (2017) 38:122–39. doi: 10.1016/j.ejmp.2017.05.071
77. Yip SS, Aerts HJ. Applications and limitations of radiomics. Phys Med Biol. (2016) 61:R150–66. doi: 10.1088/0031-9155/61/13/R150
78. Murray NM, Unberath M, Hager GD, Hui FK. Artificial intelligence to diagnose ischemic stroke and identify large vessel occlusions: a systematic review. J Neurointerv Surg. (2020) 12:156–64. doi: 10.1136/neurintsurg-2019-015135
79. Choy G, Khalilzadeh O, Michalski M, Do S, Samir AE, Pianykh OS, et al. Current applications and future impact of machine learning in radiology. Radiology. (2018) 288:318–28. doi: 10.1148/radiol.2018171820
80. Chavva IR, Crawford AL, Mazurek MH, Yuen MM, Prabhat AM, Payabvash S, et al. Deep learning applications for acute stroke management. Ann Neurol. (2022) 92:574–87. doi: 10.1002/ana.26435
81. Wang X, Fan Y, Zhang N, Li J, Duan Y, Yang B. Performance of machine learning for tissue outcome prediction in acute ischemic stroke: a systematic review and meta-analysis. Front Neurol. (2022) 13:910259. doi: 10.3389/fneur.2022.910259
82. Robben D, Boers AMM, Marquering HA, Langezaal L, Roos Y, van Oostenbrugge RJ, et al. Prediction of final infarct volume from native CT perfusion and treatment parameters using deep learning. Med Image Anal. (2020) 59:101589. doi: 10.1016/j.media.2019.101589
83. Winder AJ, Siemonsen S, Flottmann F, Thomalla G, Fiehler J, Forkert ND. Technical considerations of multi-parametric tissue outcome prediction methods in acute ischemic stroke patients. Sci Rep. (2019) 9:13208. doi: 10.1038/s41598-019-49460-y
84. Vayena E, Blasimme A, Cohen IG. Machine learning in medicine: addressing ethical challenges. PLoS Med. (2018) 15:e1002689. doi: 10.1371/journal.pmed.1002689
Keywords: machine learning, model prediction, stroke, motor function, systematic review
Citation: Li Q, Chi L, Zhao W, Wu L, Jiao C, Zheng X, Zhang K and Li X (2023) Machine learning prediction of motor function in chronic stroke patients: a systematic review and meta-analysis. Front. Neurol. 14:1039794. doi: 10.3389/fneur.2023.1039794
Received: 08 September 2022; Accepted: 25 May 2023;
Published: 13 June 2023.
Edited by:
Jean-Claude Baron, University of Cambridge, United KingdomReviewed by:
Lucas Alexandre Ramos, Academic Medical Center, NetherlandsShubham Misra, Yale University, United States
Copyright © 2023 Li, Chi, Zhao, Wu, Jiao, Zheng, Zhang and Li. This is an open-access article distributed under the terms of the Creative Commons Attribution License (CC BY). The use, distribution or reproduction in other forums is permitted, provided the original author(s) and the copyright owner(s) are credited and that the original publication in this journal is cited, in accordance with accepted academic practice. No use, distribution or reproduction is permitted which does not comply with these terms.
*Correspondence: Xiaoning Li, lixiaoning456@163.com