- 1Department of Geriatrics, The First People's Hospital of Chenzhou, Chenzhou, China
- 2Department of Endocrinology, The First People's Hospital of Chenzhou, Chenzhou, China
- 3Shanghai Jiao Tong University College of Basic Sciences, Shanghai, China
Objective: This study aims to analyze the efficacy and mechanism of action of the Shunaoxin pill in preventing cognitive impairment in diabetic patients using network pharmacology.
Methods: The main active compounds of the Shunaoxin pills and their action targets were identified via the TCMSP and Batman-TCM databases. The GEO database was used to identify the genes in type 2 diabetic individuals associated with cognitive impairment. Subsequently, a common target protein-protein interaction (PPI) network was constructed using the STRING database, and targets associated with diabetes and cognitive impairment were screened by performing a topological analysis of the PPI network. The AutoDock Vina software was used for molecular docking to evaluate the reliability of the bioinformatic analysis predictions and validate the interactions between the active ingredients of the Shunaoxin pill and proteins associated with diabetes and cognitive impairment.
Results: Based on the TCMSP and Batman-Tcm platform, 48 active ingredients of the Shunaoxin pill were identified, corresponding to 222 potential action targets. Further analysis revealed that 18 active components of the Shunaoxin pill might contribute to cognitive impairment in type 2 diabetic patients. Molecular docking simulations demonstrated that the active ingredients of the Shunaoxin pill (hexadecanoic acid, stigmasterol, beta-sitosterol, and angelicin) targeted four core proteins: OPRK1, GABRA5, GABRP, and SCN3B.
Conclusion: Active ingredients of the Shunaoxin pill may alleviate cognitive impairment in diabetic patients by targeting the proteins OPRK1, GABRA5, GABRP, and SCN3B.
Introduction
Diabetes mellitus (DM) is a metabolic disorder characterized by hyperglycemia caused by genetic and environmental factors and is the third most common non-communicable disease (1–4). DM has become a major health problem worldwide owing to its increasing prevalence and associated disability and mortality (5–7). The global prevalence of diabetes is expected to increase to 7.7% by 2030 (8, 9), and ~592 million people are expected to suffer from diabetes by 2035 (10). Furthermore, diabetic complications also increase dramatically as the incidence of diabetes increases (11). Due to inadequate insulin secretion or insulin resistance, T2DM patients experience hyperglycemia, leading to chronic damage to blood vessels, neurons, brains, and other organs (12, 13). Moreover, diabetes may also cause nervous system complications, such as cognitive impairment (14, 15). Mild cognitive impairment is estimated to affect 45% of type 2 diabetes patients (16). Learning and memory impairment are the most distinctive features of cognitive impairment in DM (17, 18). Diabetic patients may suffer from cognitive impairment, leading to abnormalities in brain neuroplasticity and energy metabolism (19). DM is associated with cognitive impairment in the elderly, a risk factor for dementia, including Alzheimer's disease (AD) (20, 21). Cognitive impairment may also result in brain deterioration or neurodegenerative diseases (16, 22). In addition, it is estimated that diabetes causes ~10–15% of dementia cases, with patients demonstrating poorer self-management skills and glycemic control (16, 23). The healthcare costs for dementia patients are 1.5 times higher than control subjects of the same age group without dementia (24), and the financial figures indicate a significant burden for patients with cognitive impairment and society. However, the pathophysiology of diabetes-induced cognitive impairment remains poorly understood, and timely and adequate diagnostic and therapeutic tools are still lacking.
Chinese medicines have multi-faceted and multi-channel effects (25–28). Network pharmacology enables the exploration of the active ingredients and potential targets of Chinese medicine by providing a holistic view of complex systems interacting with multiple disease targets (29, 30). As a result, network pharmacology has gained popularity for studying key disease-related targets and biological functions and predicting potential synergistic mechanisms against complex diseases (31, 32). Shunaoxin pills, a drug with significant therapeutic potential, have been shown to be able to dilate the thoracic aorta of isolated rats (33). A clinical study investigating the effects of the Shunaoxin pill has reported an alleviation in diabetes-induced cognitive decline (34). The Shunaoxin pill consists of two herbs, Chuanxiong and Angelica, both of which can improve chronic cerebral ischemia (35). Researchers have found that the chemical components of the Shunaoxin pill, including ferulic acid and ligustilide, possess hypoglycemic, antioxidant, and anti-inflammatory properties (36–38). Furthermore, the Shunaoxin pill can be used to treat a variety of cardiovascular and cerebrovascular diseases, such as cerebral ischemia and inadequate blood supply to the vertebral basilar artery (39). The mechanisms of cognitive impairment vary across different types of diabetes. Cognitive impairment in type 1 diabetes is associated with persistent hyperglycemic states, diabetic ketoacidosis, and hypoglycemic episodes (40, 41). On imaging, type 2 diabetes mellitus (T2DM) patients with cognitive impairment often exhibit cerebral vasculopathy, cortical atrophy, and hippocampal volume reduction (18, 42). In addition, neuroinflammation and oxidative stress are often observed in diabetes-related cognitive impairment (43–47). Therefore, the Shunaoxin pill can somewhat alleviate diabetes-related cognitive impairment. However, it is still unclear how the Shunaoxin pill prevents cognitive impairment in diabetics.
This study applied network pharmacology and molecular docking techniques to determine Shunaoxin's mechanism of action in improving cognitive impairment in diabetic patients. The study aimed to provide scientific support for traditional Chinese medicine as a treatment for cognitive impairment in diabetics from a modern medical perspective.
Materials and methods
Screening of active ingredients and drugs' targets
The active ingredients of Chuanxiong Rhizoma, Angelicae Sinensis Radix, and the drug composition of the Shunaoxin pill were searched on the TCMSP with the following screening conditions: oral bioavailability (OB) ≥30%, drug-likeness (DL) ≥0.18, and half-life (HL) ≥4. The active ingredients of Chuanxiong Rhizoma and Angelicae Sinensis Radix were searched in the Batman-Tcm database (http://bionet.ncpsb.org.cn/batman-tcm/) to obtain potential targets. The score cutoff was set to 100, and the adjusted P_value cutoff was set to 0.05. Subsequently, the Uniprot database (https://www.uniprot.org/) was used to identify the gene names of the matching targets (48, 49).
Disease target acquisition
The gene microarray data related to “cognitive impairment in type 2 diabetes” was downloaded from the GEO (gene expression omnibus) database (https://www.ncbi.nlm.nih.gov/geo/). The study data were obtained from 19 healthy adults and 17 patients with type 2 diabetes from the microarray dataset GSE138260, 15 patients with Alzheimer's disease, and 18 patients with Alzheimer's disease combined with type 2 diabetes from the microarray dataset GSE161355. Microarray data background correction, normalization, and expression value calculation were performed using the Bioconductor R package in R software. The limma package was used to calculate the differentially expressed mRNAs between the two groups. The screening criteria for differential genes were set as P < 0.05 and expression change ≥1.5-fold (|log2 FC|≥0.58). Upregulated mRNA expression was defined as log2FC ≥0.58, and down-regulated mRNA expression was defined as log2FC ≤−0.58. Finally, the differentially expressed genes (DEGs) for cognitive impairment in type 2 diabetic patients were finally derived. The heatmap package was used for cluster analysis of the screened DEGs, and the P of the differentially expressed data were transformed to –log10, which was grouped according to log2 FC.
Common target screening of active ingredients and diseases and PPI network construction
The R software (https://www.r-project.org/) and Perl programs were used to identify the intersection between disease targets and drug targets, and the Venn diagram was generated by the Venny 2.1 software (http://bioinfogp.cnb.csic.es/tools/venny/index.html). The protein–protein interaction (PPI) was constructed using the STRING database (https://string-db.org/). The protein species was set to “Homo sapiens,” medium confidence was set to 0.4, and other parameters were kept to the default settings. The potential protein-protein interaction network (PPI network) was obtained by Cytoscape 3.7.2 software (https://cytoscape.org/), and topological analysis of the PPI network was performed to screen the key targets.
Gene ontology biofunctional analysis and Kyoto encyclopedia of genes and genomes pathway enrichment
The clusterProfilerGO R package was used to analyze the common targets of the Shunaoxin pill active ingredients (50). Gene ontology (GO) analysis is mainly used to characterize the functions of gene products, including cellular components (CC), molecular functions (MF), and biological processes (BP). The Kyoto encyclopedia of genes and genomes (KEGG) pathway enrichment analysis was also performed by applying the clusterProfilerKEGG package, while the corresponding signaling pathways were mapped using the path view package. Moreover, the degree of core pathway enrichment was analyzed to investigate the possible biological functions and signaling pathway mechanisms of the active ingredients of the Shunaoxin pill in the treatment of cognitive impairment in type 2 diabetic patients based on the enrichment factor values.
Molecular docking verification
The interactions between the top four core active ingredients and the core proteins obtained from the preliminary network pharmacology screening were validated by molecular screening. The structural formula of the active ingredient was downloaded from the PubChem database (https://pubchem.ncbi.nlm.nih.gov/), and the corresponding 3D structure was produced with Chem3D software (51). The PDB format of the core protein structural domain was then downloaded from the PDB database (http://www.rcsb.org/), and protein dehydration and dephosphorylation were performed by PyMOL software. The active drug ingredient and core protein gene file were converted from PDB format to PDBQT format by the AutoDockTools 1.5.6 software, and the active pockets were identified. Finally, the Vina script was run to calculate the molecular binding energy and molecular docking results, while docking sites were identified with Discovery Studio 2019, and the LibDockScore was calculated for flexible binding. The molecular docking results were imported into PyMOL software for molecular docking conformation display. The binding energy of <0 indicates spontaneous binding of the ligand and the receptor. For the results showing Vina binding energy ≤−5.0 kcal/mol and LibDockScore >100, the ligand-receptor complexes were examined in 3D and 2D to evaluate the reliability of the bioinformatics predictions as per previous research (52–55).
Results
Active drug ingredients and corresponding targets
The relevant action targets of the Shunaoxin pill active ingredients were obtained from the TCMSP database, and the Uniprot database (https://www.uniprot.org/) was used to correct the matching target gene names. Finally, 48 active ingredients were obtained, corresponding to 222 potential targets. Cytoscape 3.7.2 software was used to construct the topology of the Shunaoxin pill active ingredient target network (Figure 1). Eight active ingredients were identified, namely hexadecanoic acid, ethanol, 13-methyl pentadecanoic acid, methyl pentadecanoate, pentadecanoic acid, azelaic acid, decanoic acid, angelicin. These may be the main active ingredients in Shunaoxin pills.
Screening for disease targets
To screen for protein targets associated with diabetes and cognitive impairment, 993 differentially expressed mRNAs were screened in the GSE138260 dataset, including 652 upregulated and 341 downregulated mRNAs. The GSE161355 dataset screened 196 differentially expressed mRNAs, including 145 upregulated and 51 downregulated mRNAs. Figures 2A,B display the heat maps based on the p-value screening of the top 100 most significant DEGs. Those processed data were imported into R to generate volcano plots, as shown in Figures 2C,D. Diabetes and cognitive impairment may be associated with these differential mRNAs.
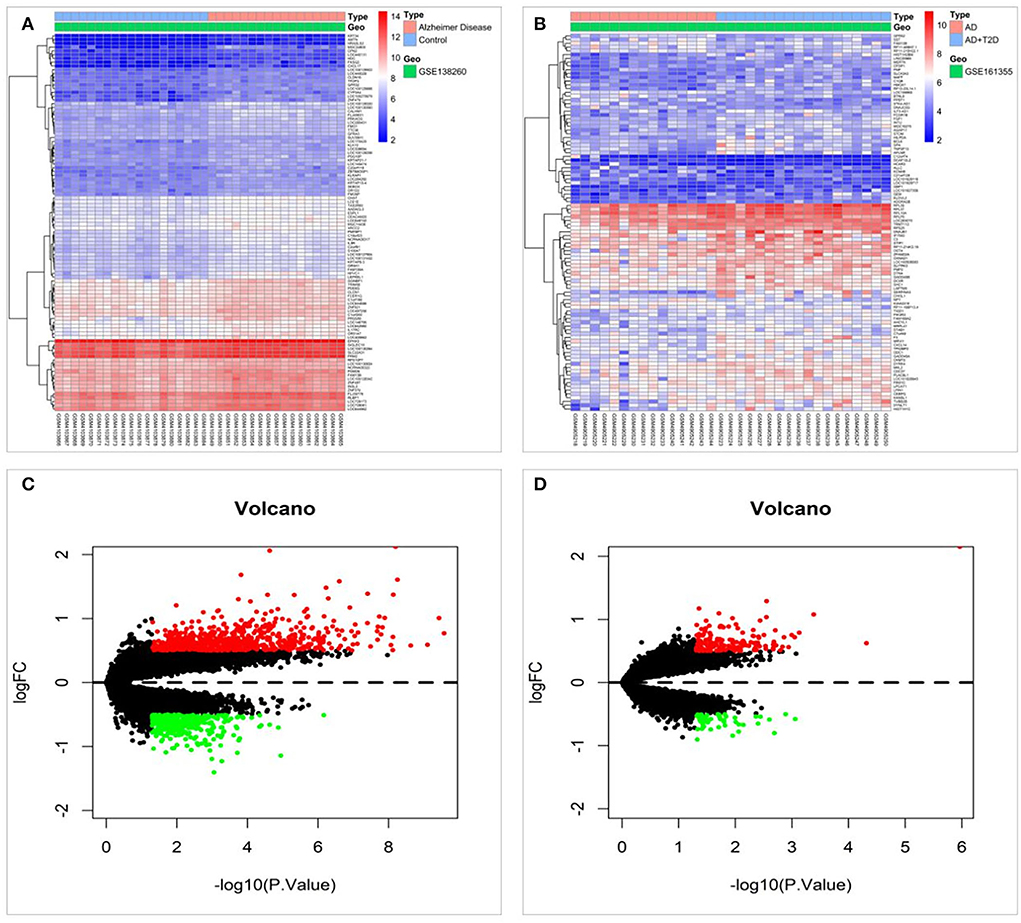
Figure 2. Differentially expressed mRNAs were screened in the GSE138260 and GSE161355 datasets. (A) Heat map of differentially expressed genes in the GSE138260 dataset. (B) Heat map of differentially expressed genes in the GSE161355 dataset. (C) Volcano plot of differentially expressed genes in the GSE138260 dataset. (D) Volcano plot of differentially expressed genes in the GSE161355 dataset.
Common target screening and interaction network construction
All active ingredient targets of the Shunaoxin pill, the GSE138260 dataset, and the GSE161355 dataset were imported into the online Venn diagram production site jvenn, and 18 intersecting potential targets of action were obtained (Figure 3). Cytoscape 3.7.2 was used to construct the target network for cognitive impairment in type 2 diabetics (Figures 4A,B). The intersecting target genes were imported into the STRING online analysis website (https://string-db.org/), and the protein-protein interaction results were exported (Figure 4C). After that, the CytoHubba plugin was used to obtain the core 16 potential target genes based on the degree algorithm as in previous research (Figure 4D) (56). Therefore, the Shunaoxin pill may target proteins that have been identified as targets as a result of these screenings.
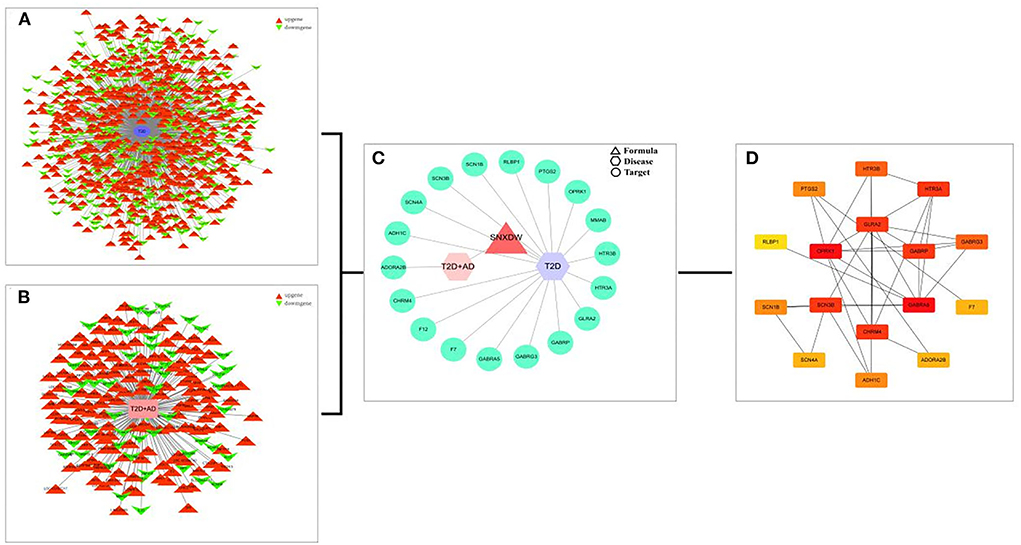
Figure 4. Interaction networks. (A) GSE138260 differentially expressed genes interaction network. (B) GSE161355 differentially expressed gene interaction network. (C) PPI network was constructed by Shunaoxin pill-disease-target genes. (D) Core 16 potential target genes were calculated based on degree values.
GO and KEGG enrichment analysis results
The Bioconductor package and cluster profile package in R were used to analyze GO and KEGG pathway enrichment analysis of Shunaoxin pill target proteins. GO analysis revealed that the 18 potential targets were mainly enriched in the biological processes of membrane potential regulation, chloride transmembrane transport, chloride transport, etc (Figure 5A). In addition, cell composition (CC) was mainly enriched in ion channel complexes, transmembrane transporter complexes, transporter complexes, etc (Figure 5B); molecular function (MF) was enriched primarily in neurotransmitter receptor activity, gated channel activity, extracellular ligand-gated ion channel activity, etc (Figure 5C). These GO enrichment pathways are shown in Figure 5D.
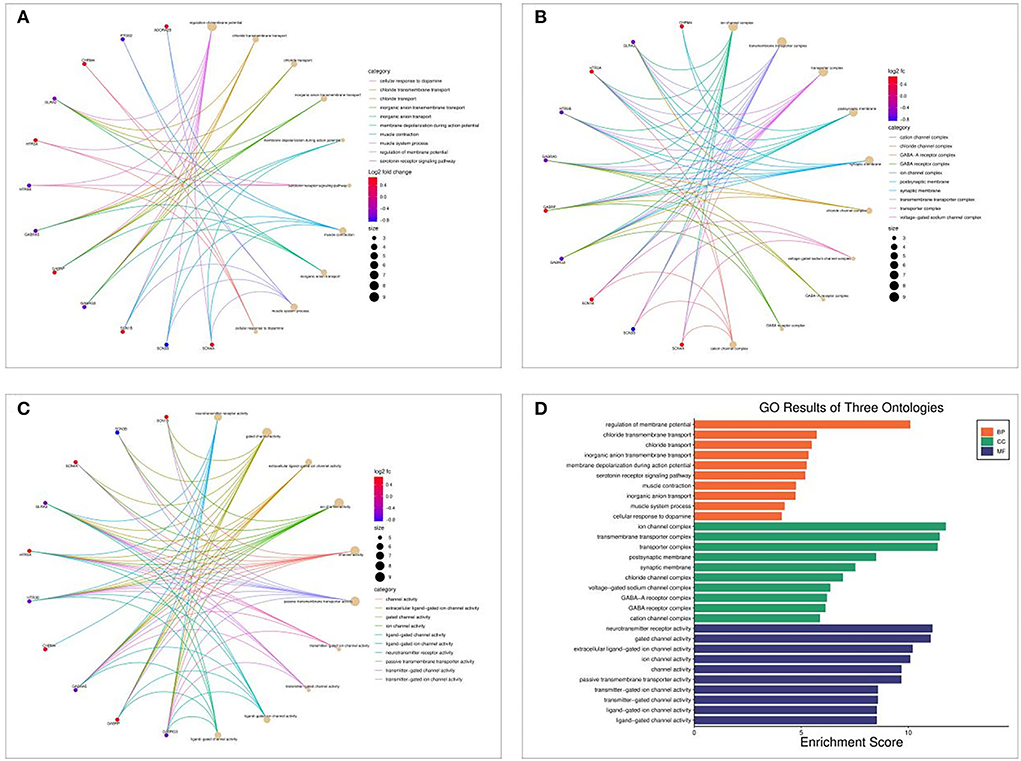
Figure 5. GO enrichment analysis of 18 potential targets of Shunaoxin pill for the treatment of cognitive impairment in patients with type 2 diabetes. (A) BP functional analysis chord diagram. (B) CC functional analysis chord diagram. (C) MF functional analysis chord diagram. (D) GO enrichment analysis histogram.
KEGG pathway enrichment analysis revealed that it was mainly concentrated in inflammatory signaling pathways such as neuroactive ligand-receptor interaction, nicotine addiction, retrograde endocannabinoid signaling, taste transduction, and GABAergic synapse (Figure 6A). The path view package in R software was used to display the signaling pathway map associated with cognitive impairment in Shunaoxin pill-treated patients with type 2 diabetes (Figure 6B). The neuroactive ligand-receptor interaction signaling pathway was found to be enriched in the potential target proteins of the Shunaoxin pill.
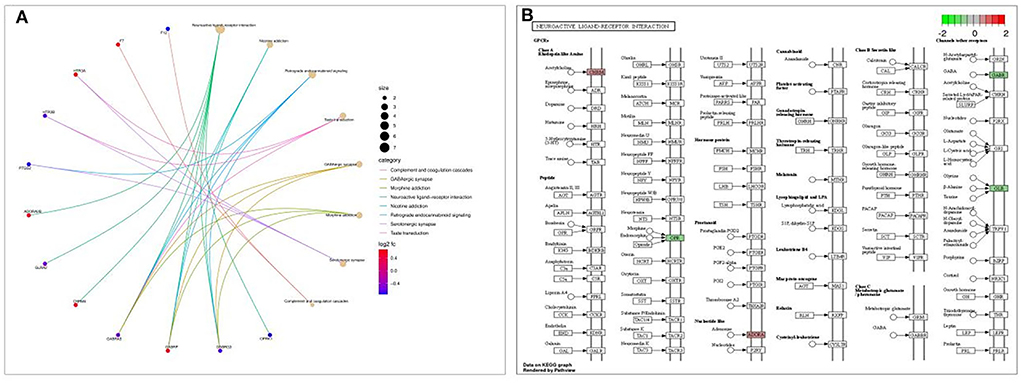
Figure 6. Enrichment analysis. (A) KEGG enrichment analysis bubble diagram. (B) Neuroactive ligand-receptor interaction signaling pathway.
Results of molecular docking
The 3D structures of hexadecanoic acid, stigmasterol, beta-sitosterol, and angelicin were drawn based on their structural formulae using Chem3D software. The 3D structures of the core proteins OPRK1, GABRA5, GABRP, and SCN3B were downloaded from the PDB database and exported to PDB format. The Vina script was used to calculate the binding energy of ligands and receptors, as shown in Table 1. GABRA5, GABRP, and SCN3B were all unable to form a stable docking model with hexadecanoic acid due to binding energies >−5.0 kcal/mol. However, the binding energies of the remaining dockers were all lower than −5.0 kcal/mol, indicating stable docking. In addition, the active molecules and corresponding target proteins were docked using the Discovery Studio 2019 software, and the LibDockScore was calculated, as shown in Table 1. The results suggested that the active ingredients hexadecanoic acid, stigmasterol, beta-sitosterol, and angelicin were able to dock semi-flexibly with the core proteins OPRK1, GABRA5, GABRP, and SCN3B, revealing the docking sites. The docking model LibDockScore of core protein OPRK1 with active ingredients hexadecanoic acid, stigmasterol, and beta-sitosterol, core protein GABRA5 with active ingredient beta-sitosterol, and core protein SCN3B with active ingredients stigmasterol and beta-sitosterol were all >100 (Figures 7, 8). The dimer formed by active ingredient stigmasterol and core protein OPRK1 was the most stable in terms of Root Mean Square Deviation (RMSD), chemical energy, and docking fraction, while the dimer formed by active ingredient beta-sitosterol and core protein SCN3B was the second most stable. Finally, the results of the compounds exported by Vina and the three-dimensional and 2-dimensional molecular docking presentations with protein ligands were imported into Pymol using Discovery Studio 2019 software (Figures 7, 8). In this study, it was demonstrated that the beneficial effects of Shunaoxin pill on diabetic cognitive impairment are mediated by the active ingredients hexadecanoic acid, stigmasterol, beta-sitosterol, and angelicin on the diabetic cognitive impairment-related proteins OPRK1, GABRA5, GABRP, and SCN3B.
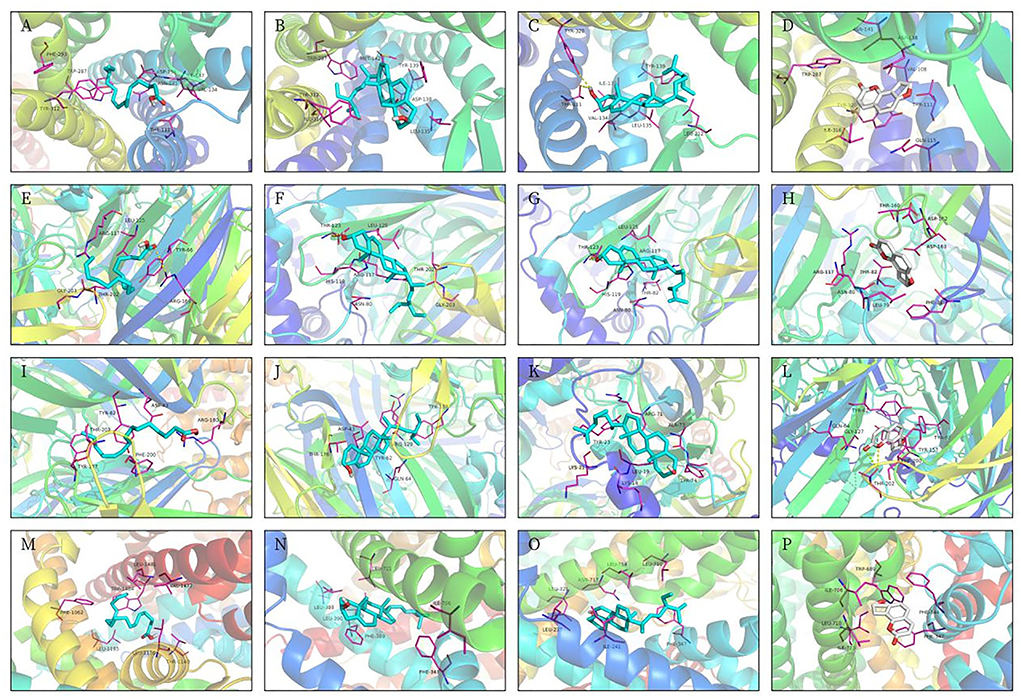
Figure 7. Molecular docking 3D models. (A) OPRK1-hexadecanoic acid complex. (B) OPRK1-stigmasterol complex. (C) OPRK1-beta-sitosterol complex. (D) OPRK1-angelicin complex. (E) GABRA5-Hexadecanoic acid complex. (F) GABRA5-stigmasterol complex. (G) GABRA5-beta-sitosterol complex. (H) GABRA5-angelicin complex. (I) GABRP-hexadecanoic acid complex. (J) GABRP-stigmasterol complex. (K) GABRP-beta-sitosterol complex. (L) GABRP-angelicin complex. (M) SCN3B-Hexadecanoic acid complex. (N) SCN3B-stigmasterol complex. (O) SCN3B-beta-sitosterol complex. (P) SCN3B-angelicin complex.
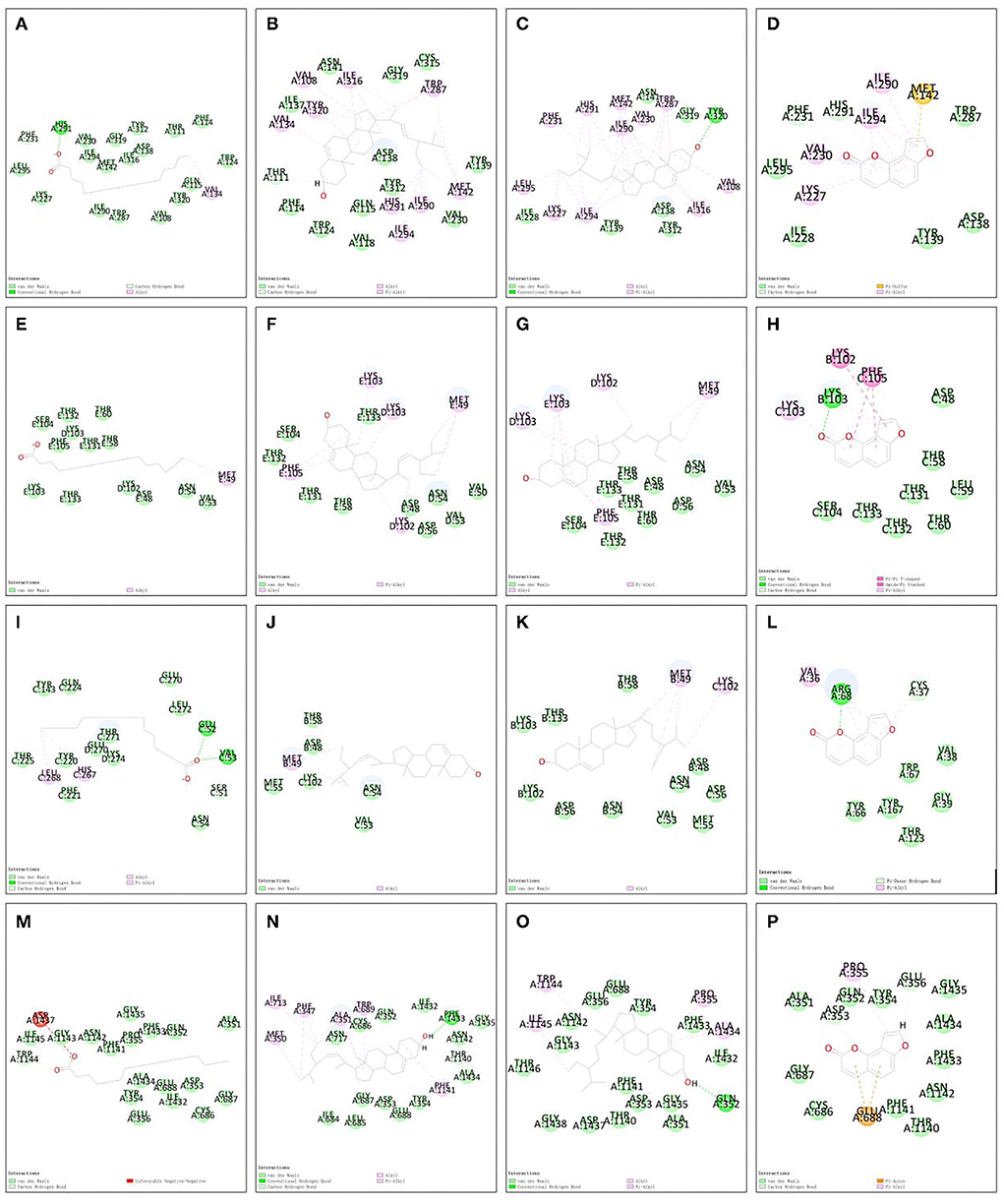
Figure 8. Molecular docking 2D models. (A) OPRK1-hexadecanoic acid complex. (B) OPRK1-stigmasterol complex. (C) OPRK1-beta-sitosterol complex. (D) OPRK1-angelicin complex. (E) GABRA5-hexadecanoic acid complex. (F) GABRA5-stigmasterol complex. (G) GABRA5-beta-sitosterol complex. (H) GABRA5-angelicin complex. (I) GABRP-hexadecanoic acid complex. (J) GABRP-stigmasterol complex. (K) GABRP-beta-sitosterol complex. (L) GABRP-angelicin complex. (M) SCN3B-hexadecanoic acid complex. (N) SCN3B-stigmasterol complex. (O) SCN3B-beta-sitosterol complex. (P) SCN3B-angelicin complex.
Discussion
In this study, 18 potential targets of the Shunaoxin pill were obtained, and 16 potential core target genes were identified. Subsequently, a Shunaoxin pill-disease-target gene PPI network was constructed. The core proteins identified in PPI were OPRK1, GABRA5, GABRP, and SCN3B.
The OPRK1 gene encodes an opioid receptor, and methylation of the gene has been linked to the development of Alzheimer's disease. It is considered a drug target for the treatment of neurological diseases, playing an essential role in the development of cognitive impairment (57). The opioid receptors are mainly located in the hippocampal region, where neuroprotective effects reduce beta-amyloid production (58–60). Hiramatsu et al. reported that in a mouse model, OPRK1 agonists slowed the progression of cognitive dysfunction due to Aβ deposition (61). Diabetes can lead to massive production of islet amyloid polypeptide (IAPP), which crosses the blood-brain barrier and is deposited in the brain (hippocampus), further causing misfolding and aggregation of β-amyloid, resulting in cognitive impairment (62, 63). Hippocampal volume atrophy is observed in patients with type 2 diabetes who develop cognitive impairment (18, 42). Thus, the OPRK1 gene may be involved in the development of cognitive impairment in diabetes by affecting β-amyloid. In the present study, the Shunaoxin pill active ingredient stigmasterol was found to bind the most stably with the core protein OPRK1 in T2DM-induced cognitive impairment. This further confirms that OPRK1 plays a vital role in diabetic cognitive impairment.
GABRA5 encodes a receptor for the α5 subunit of GABA and is highly expressed in pyramidal neurons of the hippocampus (64). Furthermore, GABRA5 gene expression levels were correlated with memory function and learning index (65). In aged rats, GABRA5-encoded receptor protein expression was also associated with cognitive performance, spatial working memory, and neuronal apoptosis in aged rats (66). Moreover, it is involved in the neurophysiological features of cognitive decline in rats and humans (65). Although the exact mechanism is unknown, cognitive deficits are mainly associated with hippocampal damage in T2DM (67). In this study, GABRA5 was found to be involved in diabetes-related cognitive impairment as one of the core target proteins of the Shunaoxin pill, which may be related to its high expression in the hippocampus.
GABRP is the π-subunit of the amino acid-like inhibitory neurotransmitter gamma-aminobutyric acid (GABA) A receptor (68). GABRP was found to improve glucose tolerance and increase insulin sensitivity in the peripheral tissues of diabetic mice, interacting with GABA to maintain normal metabolism and blood glucose stability (69, 70). This study identified GABRP as one of the core target proteins of the Shunaoxin pill for preventing diabetes-related cognitive impairment. Brain insulin resistance and disorders of intracellular glucose metabolism are associated with abnormal glucose transport in diabetes (71). Brain insulin resistance in the brain may fail to stimulate the clearance of Aβ. Accumulating Aβ in neurons leads to neurodegeneration or neuronal loss, which causes cognitive impairment (72). According to previous studies, GABRP may protect against diabetes-related cognitive impairment by maintaining normal metabolism and glucose stability.
The sodium channel β3 subunit (SCN3B) is an ion channel gene that is upregulated in the dorsal root ganglion during nerve injury, suggesting neuropathic injury (73). Stimulation of peripheral nerves in diabetic peripheral neuropathy (DPN) patients was demonstrated to activate cognitive-related areas of the brain, such as the temporal lobe and hippocampus (74). Furthermore, the reduced sensory conduction velocity of the peroneal nerve is often seen in DPN. In the present study, beta-sitosterol, an active ingredient in the Shunaoxin pill, was shown to bind stably to SCN3B, thus possibly playing a role in the development of diabetes-induced cognitive impairment. Therefore, we hypothesize that its mechanism of action on cognition may involve neurotransmission sodium channels.
The findings of this study provide a foundation for further exploration of TCM therapeutic targets for diabetic cognitive impairment. Network pharmacology revealed that the active ingredients in the Shunaoxin pill—hexadecanoic acid, stigmasterol, beta-sitosterol, and angelicin—could be semi-flexibly docked to the receptor-ligands of their respective core proteins, OPRK1, GABRA5, GABRP, and SCN3B. The active ingredient stigmasterol formed the most stable dimer with the core protein OPRK1, and the active ingredient beta-sitosterol formed the second most stable dimer with the core protein SCN3B. The present study illustrates the action of the active ingredients—hexadecanoic acid, stigmasterol, beta-sitosterol, and angelicin—on the diabetic cognitive impairment-related proteins OPRK1, GABRA5, GABRP, and SCN3B that are responsible for the beneficial properties of the Shunaoxin pill on cognitive impairment in diabetic patients.
However, this study also has some limitations. This study is based on network pharmacology and lacks experiments exploring the specific mechanism of the Shunaoxin pill's active ingredients targeting core proteins in diabetic cognitive impairment. Further validation of core proteins in clinical samples of the disease should be performed. Since cognitive impairment includes multiple disorders, this study focused only on AD-related datasets; future studies should explore more cognitive impairment-related datasets.
Conclusion
This study demonstrated that the Shunaoxin pill is pharmacologically effective for cognitive impairment in diabetic patients. Its active ingredients, hexadecanoic acid, stigmasterol, beta-sitosterol, and angelicin, target the proteins OPRK1, GABRA5, GABRP, and SCN3B associated with diabetic cognitive impairment. This research provides a foundation for further exploration of TCM therapeutic targets for cognitive impairment in diabetic patients.
Data availability statement
The original contributions presented in the study are included in the article/supplementary materials, further inquiries can be directed to the corresponding author.
Author contributions
YG and NL proposed and designed the study and collected and analyzed the data. YG provided the analysis tools and performed quality control. YG, NL, and XK wrote the manuscript. All authors contributed to the article and approved the submitted version.
Conflict of interest
The authors declare that the research was conducted in the absence of any commercial or financial relationships that could be construed as a potential conflict of interest.
Publisher's note
All claims expressed in this article are solely those of the authors and do not necessarily represent those of their affiliated organizations, or those of the publisher, the editors and the reviewers. Any product that may be evaluated in this article, or claim that may be made by its manufacturer, is not guaranteed or endorsed by the publisher.
Abbreviations
DM, diabetes mellitus; T2DM, type 2 diabetes mellitus; SNX, Shunaoxin dropping pills; OPRK1, opioid receptor kappa 1; GABA, gamma-aminobutyric acid; GABRA5, gamma-aminobutyric acid type A receptor subunit alpha5; GABRP, gamma-aminobutyric acid type A receptor subunit pi; SCN3B, sodium voltage-gated channel beta subunit 3.
References
1. Makar AB, McMartin KE, Palese M, Tephly TR. Formate assay in body fluids: application in methanol poisoning. Biochem Med. (1975) 13:117–26. doi: 10.1016/0006-2944(75)90147-7
2. Wang J, Xiao M, Wang J, Wang S, Zhang J, Guo Y, et al. NRF2-related epigenetic modifications in cardiac and vascular complications of diabetes mellitus. Front Endocrinol. (2021) 12:598005. doi: 10.3389/fendo.2021.598005
3. Evans JL, Goldfine ID. A new Road for treating the vascular complications of diabetes: so let's step on the gas. Diabetes. (2016) 65:346–8. doi: 10.2337/dbi15-0029
4. Zhou Q, Ge Q, Ding Y, Qu H, Wei H, Wu R, et al. Relationship between serum adipsin and the first phase of glucose-stimulated insulin secretion in individuals with different glucose tolerance. J Diabetes Investig. (2018) 9:1128–34. doi: 10.1111/jdi.12819
5. Li Y, Teng D, Shi X, Qin G, Qin Y, Quan H, et al. Prevalence of diabetes recorded in mainland China using 2018 diagnostic criteria from the American Diabetes Association: national cross sectional study. BMJ. (2020) 2020:m997. doi: 10.1136/bmj.m997
6. Vos T, Abajobir AA, Abate KH, Abbafati C, Abbas KM, Abd-Allah F, et al. Global, regional, and national incidence, prevalence, and years lived with disability for 328 diseases and injuries for 195 countries, 1990–2016: a systematic analysis for the Global Burden of Disease Study 2016. Lancet. (2017) 390:1211–59. doi: 10.1016/S0140-6736(17)32154-2
7. Suviranta T, Timonen J, Martikainen J, Aarnio E. The effects of reimbursement reform of antidiabetic medicines from the patients' perspective—a survey among patients with type 2 diabetes in Finland. BMC Health Serv Res. (2019) 19:769. doi: 10.1186/s12913-019-4633-9
8. Shaw JE, Sicree RA, Zimmet PZ. Global estimates of the prevalence of diabetes for 2010 and 2030. Diabetes Res Clin Pract. (2010) 87:4–14. doi: 10.1016/j.diabres.2009.10.007
9. Xu X, Pan X, Li S. Prospective analysis of the efficacy of beraprost sodium combined with alprostadil on diabetic nephropathy and influence on renninangiotensin system and TNF-α. Exp Ther Med. (2020) 19:639–45. doi: 10.3892/etm.2019.8265
10. Guariguata L, Whiting DR, Hambleton I, Beagley J, Linnenkamp U, Shaw JE. Global estimates of diabetes prevalence for 2013 and projections for 2035. Diabetes Res Clin Pract. (2014) 103:137–49. doi: 10.1016/j.diabres.2013.11.002
11. Mayer-Davis EJ, Lawrence JM, Dabelea D, Divers J, Isom S, Dolan L, et al. Incidence trends of type 1 and type 2 diabetes among youths, 2002–2012. N Engl J Med. (2017) 376:1419–29. doi: 10.1056/NEJMoa1610187
12. Geijselaers SLC, Sep SJS, Stehouwer CDA, Biessels GJ. Glucose regulation, cognition, and brain MRI in type 2 diabetes: a systematic review. Lancet Diabetes Endocrinol. (2015) 3:75–89. doi: 10.1016/S2213-8587(14)70148-2
13. Koekkoek PS, Kappelle LJ, van den Berg E, Rutten GEHM, Biessels GJ. Cognitive function in patients with diabetes mellitus: guidance for daily care. Lancet Neurol. (2015) 14:329–40. doi: 10.1016/S1474-4422(14)70249-2
14. Ahtiluoto S, Polvikoski T, Peltonen M, Solomon A, Tuomilehto J, Winblad B, et al. Diabetes, Alzheimer disease, and vascular dementia: a population-based neuropathologic study. Neurology. (2010) 75:1195–202. doi: 10.1212/WNL.0b013e3181f4d7f8
15. Zhu L, Li C, Du G, Pan M, Liu G, Pan W, Li X. High glucose upregulates myosin light chain kinase to induce microfilament cytoskeleton rearrangement in hippocampal neurons. Mol Med Rep. (2018) 18:216–22. doi: 10.3892/mmr.2018.8960
16. You Y, Liu Z, Chen Y, Xu Y, Qin J, Guo S, et al. The prevalence of mild cognitive impairment in type 2 diabetes mellitus patients: a systematic review and meta-analysis. Acta Diabetol. (2021) 58:671–85. doi: 10.1007/s00592-020-01648-9
17. Kessels RPC, Kappelle LJ, Biessels GJ. A 4-year follow-up study of cognitive functioning in patients with type 2 diabetes mellitus. Diabetologia. (2010) 53:58–65. doi: 10.1007/s00125-009-1571-9
18. McCrimmon RJ, Ryan CM, Frier BM. Diabetes and cognitive dysfunction. Lancet. (2012) 379:2291–9. doi: 10.1016/S0140-6736(12)60360-2
19. Lei H, Hu R, Luo G, Yang T, Shen H, Deng H, et al. Altered structural and functional MRI connectivity in type 2 diabetes mellitus related cognitive impairment: a review. Front Hum Neurosci. (2022) 15:755017. doi: 10.3389/fnhum.2021.755017
20. Strachan MWJ, Reynolds RM, Frier BM, Mitchell RJ, Price JF. The relationship between type 2 diabetes and dementia. Br Med Bull. (2008) 88:131–46. doi: 10.1093/bmb/ldn042
21. Bohnen NI, Kotagal V, Müller MLTM, Koeppe RA, Scott PJH, Albin RL, et al. Diabetes mellitus is independently associated with more severe cognitive impairment in Parkinson's disease. Parkinsonism Relat Disord. (2014) 20:1394–8. doi: 10.1016/j.parkreldis.2014.10.008
22. Cheong JLY, de Pablo-Fernandez E, Foltynie T, Noyce AJ. The association between type 2 diabetes mellitus and Parkinson's disease. JPD. (2020) 10:775–89. doi: 10.3233/JPD-191900
23. Biessels GJ, Reagan LP. Hippocampal insulin resistance and cognitive dysfunction. Nat Rev Neurosci. (2015) 16:660–71. doi: 10.1038/nrn4019
24. Vagelatos NT, Eslick GD. Type 2 diabetes as a risk factor for Alzheimer's disease: the confounders, interactions, and neuropathology associated with this relationship. Epidemiol Rev. (2013) 35:152–60. doi: 10.1093/epirev/mxs012
25. Song Y, Yang J, Jing W, Wang Q, Liu Y, Cheng X, et al. Systemic elucidation on the potential bioactive compounds and hypoglycemic mechanism of Polygonum multiflorum based on network pharmacology. Chin Med. (2020) 15:121. doi: 10.1186/s13020-020-00401-2
26. Tao P, Ji J, Gu S, Wang Q, Xu Y. Progress in the mechanism of autophagy and traditional Chinese medicine herb involved in dementia. Front Pharmacol. (2022) 12:825330. doi: 10.3389/fphar.2021.825330
27. Chen Y, Luo Z, Lin J, Qi B, Sun Y, Li F, et al. Exploring the potential mechanisms of Melilotus officinalis (L) Pall in chronic muscle repair patterns using single cell receptor-ligand marker analysis and molecular dynamics simulations. Disease Markers. (2022) 2022:1–11. doi: 10.1155/2022/9082576
28. Chen Y, Luo Z, Sun Y, Li F, Han Z, Qi B, et al. Exercise improves choroid plexus epithelial cells metabolism to prevent glial cell-associated neurodegeneration. Front Pharmacol. (2022) 13:1010785. doi: 10.3389/fphar.2022.1010785
29. Poornima P, Kumar JD, Zhao Q, Blunder M, Efferth T. Network pharmacology of cancer: understanding complex interactomes to designing multi-target specific therapeutics from nature. Pharmacol Res. (2016) 111:290–302. doi: 10.1016/j.phrs.2016.06.018
30. Lee H-S, Lee I-H, Park S-I, Lee D-Y. Network pharmacology-based investigation of the system-level molecular mechanisms of the hematopoietic activity of Samul-Tang, a traditional Korean herbal formula. Evid Based Complement Altern Med. (2020) 2020:1–17. doi: 10.1155/2020/9048089
31. Zhang R, Zhu X, Bai H, Ning K. Network pharmacology databases for traditional chinese medicine: review and assessment. Front Pharmacol. (2019) 10:123. doi: 10.3389/fphar.2019.00123
32. Li S, Zhang B. Traditional Chinese medicine network pharmacology: theory, methodology, and application: traditional Chinese medicine network pharmacology: theory, methodology, and application. Chin J Nat Med. (2014) 11:110–20. doi: 10.3724/SP.J.1009.2013.00110
33. Huo L, Zhang J, Qu Z, Chen H, Li Y, Gao W. Vasorelaxant effects of Shunaoxin pill are mediated by NO/cGMP pathway, HO/CO pathway, and calcium channel blockade in isolated rat thoracic aorta. J Ethnopharmacol. (2015) 173:352–60. doi: 10.1016/j.jep.2015.07.048
34. Zhou H, Qu Z, Zhang J, Liu Y, Yang H, Chen H, et al. Antidiabetic effect of ligustilide-rich total lactones derived from Shunaoxin dropping pills on mice with type 2 diabetes induced by a high-fat diet and streptozotocin. RSC Adv. (2016) 6:109132–42. doi: 10.1039/C6RA24274J
35. Shi J, Li R, Yang S, Phang Y, Zheng C, Zhang H. The protective effects and potential mechanisms of Ligusticum chuanxiong: focus on anti-inflammatory, antioxidant, and antiapoptotic activities. Evid Based Complement Altern Med. (2020) 2020:1–9. doi: 10.1155/2020/8205983
36. Choi HG, Tran PT, Lee J-H, Min BS, Kim JA. Anti-inflammatory activity of caffeic acid derivatives isolated from the roots of Salvia miltiorrhiza Bunge. Arch Pharm Res. (2018) 41:64–70. doi: 10.1007/s12272-017-0983-1
37. Schwager J, Gagno L, Richard N, Simon W, Weber P, Bendik I. Z-ligustilide and anti-inflammatory prostaglandins have common biological properties in macrophages and leukocytes. Nutr Metab (Lond). (2018) 15:4. doi: 10.1186/s12986-018-0239-1
38. Mitra S, Tareq AM, Das R, Emran TB, Nainu F, Chakraborty AJ, et al. Polyphenols: a first evidence in the synergism and bioactivities. Food Rev Int. (2022) 2022:1–23. doi: 10.1080/87559129.2022.2026376
39. Wu K, Wang Z-Z, Liu D, Qi X-R. Pharmacokinetics, brain distribution, release, and blood-brain barrier transport of Shunaoxin pills. J Ethnopharmacol. (2014) 151:1133–40. doi: 10.1016/j.jep.2013.12.027
40. Semenkovich K, Bischoff A, Doty T, Nelson S, Siller AF, Hershey T, et al. Clinical presentation and memory function in youth with type 1 diabetes: Memory Effects of DKA and Hyperglycemia. Pediatr Diabetes. (2016) 17:492–9. doi: 10.1111/pedi.12314
41. Li W, Huang E, Gao S. Type 1 diabetes mellitus and cognitive impairments: a systematic review. JAD. (2017) 57:29–36. doi: 10.3233/JAD-161250
42. Brundel M, Kappelle LJ, Biessels GJ. Brain imaging in type 2 diabetes. Eur Neuropsychopharmacol. (2014) 24:1967–81. doi: 10.1016/j.euroneuro.2014.01.023
43. Hsieh C-F, Liu C-K, Lee C-T, Yu L-E, Wang J-Y. Acute glucose fluctuation impacts microglial activity, leading to inflammatory activation or self-degradation. Sci Rep. (2019) 9:840. doi: 10.1038/s41598-018-37215-0
44. Cervellati C, Trentini A, Pecorelli A, Valacchi G. Inflammation in neurological disorders: the thin boundary between brain and periphery. Antioxid Redox Signal. (2020) 33:191–210. doi: 10.1089/ars.2020.8076
45. Mao X-Y, Cao D-F, Li X, Yin J-Y, Wang Z-B, Zhang Y, et al. Huperzine A ameliorates cognitive deficits in streptozotocin-induced diabetic rats. IJMS. (2014) 15:7667–83. doi: 10.3390/ijms15057667
46. Farbood Y, Ghaderi S, Rashno M, Khoshnam SE, Khorsandi L, Sarkaki A, et al. Sesamin: a promising protective agent against diabetes-associated cognitive decline in rats. Life Sci. (2019) 230:169–77. doi: 10.1016/j.lfs.2019.05.071
47. Liu C, Cui G, Zhu M, Kang X, Guo H. Neuroinflammation in Alzheimer's disease: chemokines produced by astrocytes and chemokine receptors. Int J Clin Exp Pathol. (2014) 7:8342–55.
48. Xu X, Zhang W, Huang C, Li Y, Yu H, Wang Y, et al. Novel chemometric method for the prediction of human oral bioavailability. IJMS. (2012) 13:6964–82. doi: 10.3390/ijms13066964
49. Wan Y, Xu L, Liu Z, Yang M, Jiang X, Zhang Q, et al. Utilising network pharmacology to explore the underlying mechanism of Wumei Pill in treating pancreatic neoplasms. BMC Complement Altern Med. (2019) 19:158. doi: 10.1186/s12906-019-2580-y
50. Luo W, Brouwer C. Pathview: a R/Bioconductor package for pathway-based data integration and visualization. Bioinformatics. (2013) 29:1830–1. doi: 10.1093/bioinformatics/btt285
51. Kim S, Chen J, Cheng T, Gindulyte A, He J, He S, et al. PubChem in 2021: new data content and improved web interfaces. Nucleic Acids Res. (2021) 49:D1388–95. doi: 10.1093/nar/gkaa971
52. Veber DF, Johnson SR, Cheng H-Y, Smith BR, Ward KW, Kopple KD. Molecular properties that influence the oral bioavailability of drug candidates. J Med Chem. (2002) 45:2615–23. doi: 10.1021/jm020017n
53. Zhang Q, Yang J, Yang C, Yang X, Chen Y. Eucommia ulmoides oliver-tribulus terrestris l. drug pair regulates ferroptosis by mediating the neurovascular-related ligand-receptor interaction pathway- a potential drug pair for treatment hypertension and prevention ischemic stroke. Front Neurol. (2022) 13:833922. doi: 10.3389/fneur.2022.833922
54. Kang X, Sun Y, Yi B, Jiang C, Yan X, Chen B, et al. Based on network pharmacology and molecular dynamics simulations, baicalein, an active ingredient of Yiqi Qingre Ziyin method, potentially protects patients with atrophic rhinitis from cognitive impairment. Front Aging Neurosci. (2022) 14:880794. doi: 10.3389/fnagi.2022.880794
55. Lu L, Kang X, Yi B, Jiang C, Yan X, Chen B, et al. Exploring the Mechanism of Yiqi Qingre Ziyin method in regulating neuropeptide expression for the treatment of atrophic rhinitis. Dis Markers. (2022) 2022:1–12. doi: 10.1155/2022/4416637
56. Chin C-H, Chen S-H, Wu H-H, Ho C-W, Ko M-T, Lin C-Y. cytoHubba: identifying hub objects and sub-networks from complex interactome. BMC Syst Biol. (2014) 8:S11. doi: 10.1186/1752-0509-8-S4-S11
57. Liu G, Ji H, Liu J, Xu C, Chang L, Cui W, et al. Association of OPRK1 and OPRM1 methylation with mild cognitive impairment in Xinjiang Han and Uygur populations. Neuroscience Letters. (2017) 636:170–6. doi: 10.1016/j.neulet.2016.11.018
58. Daumas S, Betourne A, Halley H, Wolfer DP, Lipp H-P, Lassalle J-M, et al. Transient activation of the CA3 Kappa opioid system in the dorsal hippocampus modulates complex memory processing in mice. Neurobiol Learn Mem. (2007) 88:94–103. doi: 10.1016/j.nlm.2007.02.001
59. Cai Z, Ratka A. Opioid system, and Alzheimer's disease. Neuromol Med. (2012) 14:91–111. doi: 10.1007/s12017-012-8180-3
60. Wang Y, Wang Y-X, Liu T, Law P-Y, Loh HH, Qiu Y, et al. μ-Opioid receptor attenuates a β oligomers-induced neurotoxicity through mTOR signaling. CNS Neurosci Ther. (2015) 21:8–14. doi: 10.1111/cns.12316
61. Hiramatsu M, Inoue K, Kameyama T. Dynorphin A-(1-13) and (2-13) improve β-amyloid peptide-induced amnesia in mice. NeuroReport. (2000) 11:431–5. doi: 10.1097/00001756-200002280-00001
62. Ferreira S, Raimundo A, Menezes R, Martins I. Islet amyloid polypeptide and amyloid beta peptide roles in Alzheimer's disease: two triggers, one disease. Neural Regen Res. (2021) 16:1127. doi: 10.4103/1673-5374.300323
63. Wang Y, Westermark GT. The amyloid forming peptides islet amyloid polypeptide and amyloid β interact at the molecular level. IJMS. (2021) 22:11153. doi: 10.3390/ijms222011153
64. Jacob TC. Neurobiology and therapeutic potential of α5-GABA type A receptors. Front Mol Neurosci. (2019) 12:179. doi: 10.3389/fnmol.2019.00179
65. Haberman R, Quigley C, Gallagher M. Characterization of CpG island DNA methylation of impairment-related genes in a rat model of cognitive aging. Epigenetics. (2012) 7:1008–19. doi: 10.4161/epi.21291
66. Shan L, Ma D, Zhang C, Xiong W, Zhang Y. miRNAs may regulate GABAergic transmission associated genes in aged rats with anesthetics-induced recognition and working memory dysfunction. Brain Res. (2017) 1670:191–200. doi: 10.1016/j.brainres.2017.06.027
67. Bonds JA, Shetti A, Stephen TKL, Bonini MG, Minshall RD, Lazarov O. Deficits in hippocampal neurogenesis in obesity-dependent and -independent type-2 diabetes mellitus mouse models. Sci Rep. (2020) 10:16368. doi: 10.1038/s41598-020-73401-9
68. Söderhielm PC, Klein AB, Bomholtz SH, Jensen AA. Profiling of GABAA and GABAB receptor expression in the myometrium of the human uterus. Life Sci. (2018) 214:145–52. doi: 10.1016/j.lfs.2018.10.033
69. Purwana I, Zheng J, Li X, Deurloo M, Son DO, Zhang Z, et al. GABA promotes human β-cell proliferation and modulates glucose homeostasis. Diabetes. (2014) 63:4197–205. doi: 10.2337/db14-0153
70. Reetz A, Solimena M, Matteoli M, Folli F, Takei K, De Camilli P, et al. and pancreatic beta-cells: colocalization of glutamic acid decarboxylase (GAD) and GABA with synaptic-like microvesicles suggests their role in GABA storage and secretion. EMBO J. (1991) 10:1275–84. doi: 10.1002/j.1460-2075.1991.tb08069.x
71. Nguyen TT, Ta QTH, Nguyen TKO, Nguyen TTD, Van Giau V. Type 3 diabetes and its role implications in Alzheimer's disease. IJMS. (2020) 21:3165. doi: 10.3390/ijms21093165
72. Nguyen TT, Ta QTH, Nguyen TTD, Le TT, Vo VG. Role of insulin resistance in the Alzheimer's disease progression. Neurochem Res. (2020) 45:1481–91. doi: 10.1007/s11064-020-03031-0
73. Dong Y, Chen Y, Yao B, Song P, Xu R, Li R, et al. Neuropathologic damage induced by radiofrequency ablation at different temperatures. Clinics. (2022) 77:100033. doi: 10.1016/j.clinsp.2022.100033
Keywords: Shunaoxin pill, diabetes, cognitive impairment, network pharmacology, molecular docking
Citation: Guo Y, Luo N and Kang X (2022) Potential mechanism of the Shunaoxin pill for preventing cognitive impairment in type 2 diabetes mellitus. Front. Neurol. 13:977953. doi: 10.3389/fneur.2022.977953
Received: 25 June 2022; Accepted: 26 September 2022;
Published: 21 October 2022.
Edited by:
Jun Xu, Capital Medical University, ChinaReviewed by:
Jiang-Hua Le, Affiliated Hospital of Guilin Medical University, ChinaXiaoyu Zhao, Shandong University of Traditional Chinese Medicine, China
Copyright © 2022 Guo, Luo and Kang. This is an open-access article distributed under the terms of the Creative Commons Attribution License (CC BY). The use, distribution or reproduction in other forums is permitted, provided the original author(s) and the copyright owner(s) are credited and that the original publication in this journal is cited, in accordance with accepted academic practice. No use, distribution or reproduction is permitted which does not comply with these terms.
*Correspondence: Yuejie Guo, eXVnb3U3NDUxQDE2My5jb20=
†These authors have contributed equally to this work