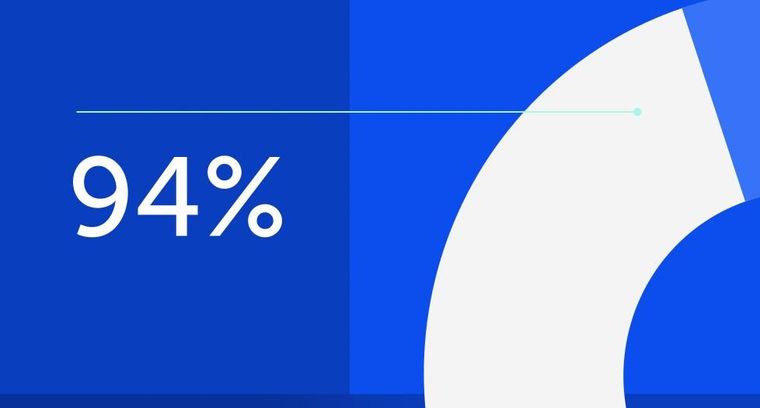
94% of researchers rate our articles as excellent or good
Learn more about the work of our research integrity team to safeguard the quality of each article we publish.
Find out more
SYSTEMATIC REVIEW article
Front. Neurol., 08 September 2022
Sec. Neurorehabilitation
Volume 13 - 2022 | https://doi.org/10.3389/fneur.2022.968818
This article is part of the Research TopicJob Integration/Reintegration of People with Neuromuscular Disorders in the Epoch of “Industry 4.0”View all 5 articles
Individuals of working age affected by neuromuscular disorders frequently experience issues with their capacity to get employment, difficulty at work, and premature work interruption. Anyway, individuals with a disability could be able to return to work, thanks to targeted rehabilitation as well as ergonomic and training interventions. Biomechanical and physiological indexes are important for evaluating motor and muscle performance and determining the success of job integration initiatives. Therefore, it is necessary to determinate which indexes from the literature are the most appropriate to evaluate the effectiveness and efficiency of the return-to-work programs. To identify current and future valuable indexes, this study uses a systematic literature review methodology for selecting articles published from 2011 to March 30, 2021 from Scopus, Web of Science, and PubMed and for checking the eligibility and the potential bias risks. The most used indexes for motor performance assessment were identified, categorized, and analyzed. This review revealed a great potential for kinetic, kinematic, surface electromyography, postural, and other biomechanical and physiological indexes to be used for job integration/reintegration. Indeed, wearable miniaturized sensors, kinematic, kinetic, and sEMG-based indexes can be used to control collaborative robots, classify residual motor functions, and assess pre–post-rehabilitation and ergonomic therapies.
Individuals of working age with neuromuscular illnesses frequently struggle with employability, work challenges, and premature job stoppage (1–3). In any case, employment integration and reintegration have been shown to improve pathological people's overall quality of life (1, 4, 5). Indeed, increasing their working life should be an important element of neuromuscular disorder care in terms of psychological, social, and health wellness (6).
An increase in self-esteem and social wellness, as well as a reduction in workplace prejudice against disabled people, can be achieved by designing an adequate job accommodation (7–9), assistance and improving, among other things, the social environment, support from colleagues and supervisors, job expectations, and ergonomic interventions (10).
Furthermore, understanding of specific work-related difficulties, as well as focused rehabilitative, ergonomic, and training interventions, can enable individuals to return to work. Rehabilitation can play a constructive role by removing barriers to obtaining, retaining, or returning to work (11–18). This concept is supported by these people's contextual ability to maintain an effective motor strategy by adopting different compensatory behaviors during the disease, despite disease progression and motor decline (19–22). Neuropathies, multiple sclerosis, stroke, spastic paraplegia, cerebellar ataxia, dystonia, traumatic spine and brain lesions, and encephalitis are degenerative and acquired neurological diseases that can impact motor function throughout working age and severely limit workers' autonomy and efficiency (6, 23–27). Therefore, workers with neurological illnesses may have motor impairment in numerous motor domains, including hand function, balance, and locomotion, placing a significant burden on society in terms of lower job productivity and expense. Clinicians manage their patients' premature work interruptions (28, 29) by developing appropriate standard and new pharmacological, surgical, and rehabilitation treatments, such as robotic rehabilitation, virtual reality, and neuromodulation (30–34). Indeed, these treatments have the primary goal of restoring patients' motor performance, autonomy, and everyday life, allowing them to return to work and optimize their work capabilities.
Furthermore, novel ergonomic solutions, such as work task rehabilitation and workplace interventions, are being added to job accommodation plans (35–37). Indeed, the fourth industrial revolution has lately opened up new occupational scenarios in which crucial human–robot collaboration (HRC) technologies, such as collaborative robots and exoskeletons, aid workers in their workplaces (3).
When a worker affected by a neurological pathology with motor disorders is reintegrated at work, an exhaustive assessment of his/her residual motor function is of primary importance to design and/or optimally adapt his/her workplace. Therefore, biomechanical and physiological indexes are useful for monitoring motor and muscle performance and verifying the effectiveness of interventions for job integration/reintegration (3).
Furthermore, the efficiency of these ergonomic interventions should be verified and monitored throughout time (3). Kinematic, kinetic, and surface electromyography (sEMG) measurements are now widely used in research laboratories by movement scientists and could be used more and more in clinical practice by health operators, to define quantitatively the form and degree of motor dysfunction, assess the complicated interaction between the fundamental deficit and the adaptive and compensating mechanisms, categorize patients based on their specific neurological condition, and finally monitor pre–post-treatment (3).
As a result, it is important to determine which of the indexes available in the literature are the most suited for assessing the effectiveness and efficiency of return-to-work programs. This research employs a systematic literature review process to suggest present and future important indexes to achieve this purpose.
This study was performed using the systematic review method proposed by the Preferred Reporting Items for Systematic Reviews and Meta-Analysis (PRISMA) (38).
This systematic review considered English articles published from 2011 to March 30, 2021, and the literature search was performed in a systematic manner using the following selected databases: Scopus, Web of Science, and PubMed. According to the database, the annual article production related to this research is starting to grow significantly from 2011, which is the starting year of the analyzed period.
There were four issues of interest in this systematic review (39): job reintegration, indexes, neurological, and quality.
For each issue identified according to the method proposed in the study mentioned in (39), the following keywords were identified as related to that topic and used for online database searching:
• Job Reintegration: “Job Integration,” “Job Reintegration,” “work Integration,” “work Reintegration,” “workplace,” “Return to work Rehabilitation,” “work ability”;
• Indexes: “kinematic index,” “kinetic index,” “force index,” “sEMG index,” “surface electromyography index,” “motor index”;
• Neurological: “Neurological motor disease,” “Neurological motor disorders,” “Neuromuscular motor disease,” “Neuromuscular motor disorders”;
• Quality: “performance,” “monitoring,” “ergonomics,” “quantitative,” “instrumental”.
A total of two, three, and four groups of keywords (one for each issue) were combined in the literature search (1,657 combinations).
We then entered each combination of one, two, three, or four keywords into each of the selected online databases (PubMed, Scopus, and Web of Science) to search for articles.
The literature search was performed by G.C., L.F., A.T., T.V., F.D., and A.R. The articles obtained were imported into Mendeley, and duplicates were removed. Our search was limited to peer-reviewed journal publications, reviews, chapters of books, and conference proceedings. The collected publications were then screened in three steps: (i) the titles were assessed for relevance; (ii) the abstracts were considered; and (iii) the complete text were downloaded when the information was deemed relevant.
Studies were considered eligible if they were written in English, and they investigated subjects using biomechanical and physiological quantitative indexes. The common goal of these eligible studies was to perform a quantitative evaluation of programs/strategies to make patients sufficiently able to return to work. Excluded were narrative and systematic reviews or meta-analyses and purely clinical studies not aimed at evaluating job placement/reintegration. Furthermore, the studies with the following characteristics were also excluded:
• studies that do not consider indexes (biomechanical and physiological indexes) of motor performance for job integration/reintegration;
• studies on simulated data and not on people;
• studies with all or almost all participants of non-working age (>67 years, since the maximum range of retirement age in Italy is 67 years for most professions);
• studies on children/teenagers (<18 years, since in Italy, it is forbidden to work if you are younger than 18 years);
• studies on only work risks assessment.
A total of six reviewers (G.C., L.F., A.T., T.V., F.D., and A.R.) individually evaluated the eligibility for all articles by assessing titles and abstracts. Disagreements among reviewers were resolved by scheduling meetings to discuss and solve them.
In addition to searching databases with the aforementioned keywords, once the authors had identified articles for inclusion in the systematic review, they also examined the bibliography of the selected articles to check whether there were any additional articles that could be included in this systematic review.
From the articles selected as eligible, the authors extracted the data that provided detailed information for each study, using the Population Intervention Comparison Outcome (PICO) framework as a guide when analyzing the eligible articles (40).
More in detail, the authors followed the following steps to extract the data from the selected articles:
• The authors looked for an existing extraction form or tool to help guide them and used existing systematic reviews on our topic to identify what information to collect if they are not sure what to do (41, 42).
• Train the review team on the extraction categories and what type of data would be expected.
• The authors performed a pilot extraction to ensure data extractors were recording similar data and revised the extraction form if needed.
• The authors discussed any discrepancies in extraction throughout the process.
• The review team documented any changes to the process or the form, kept track of the decisions the team made, and the reasoning behind them.
At the end of this procedure, the extracted information included the following:
• characteristics of the participants involved in the study: number of subjects (N), gender (F and M), age (years), height (H) in meters, weight (W) in kg, and/or body mass index (BMI) in kg/m2;
• measurement details: motor task, parameters/indexes names and acronyms if applicable, instrumentation used, and investigated body part;
• aims of the study;
• findings of the study.
A bias represents a characteristic of a study that can introduce a systematic error in the magnitude or direction findings. The potential risk of bias was assessed independently by six authors (G.C., L.F., A.T., T.V., F.D., and A.R.) according to the Cochrane Handbook for Systematic Reviews of Interventions (43) and by using the tool ROBINS-I (44, 45), which was developed for the risk of bias assessment of non-randomized studies of interventions. The authors assessed the following risks of bias (44, 45):
• confounding (D1): occurs when one or more prognostic factors can also predict the baseline intervention;
• selection of participants into study (D2): even though the effects of the interventions are the same, there will be a connection between interventions and outcomes when exclusion of some eligible participants, initial follow-up times for some participants, or certain outcome events are connected to both the intervention and the outcome;
• classification of interventions (D3): by misclassification of intervention status;
• deviation from the intended study (D4): when there are consistent discrepancies between experimental intervention and comparison groups, which represent a deviation from the intended intervention;
• missing data (D5): when later follow-up or information is missing for individuals initially included and followed;
• measurement of outcomes (D6): introduced by errors in measurement of outcome data;
• selection of the reported result (D7): selective reporting of results in a way that depends on the findings.
The study selection process started from the results of the literature database search that yielded 231,793 records, as shown in Figure 1. In particular, 71,317 were found on Scopus, 93,406 on Web of Science, and 67,070 PubMed. After removing the duplicates, the articles were 142,968. These articles were screened by deleting articles on the basis of not connected words (e.g., animal, astronomy, and human resources) and journals (e.g., International Journal of Molecular Science), obtaining 4,119 articles.
Figure 1. PRISMA flowchart related to the steps of a systematic review provided by the journal Frontiers in Neurology.
These articles were screened based on their title, obtaining 1,187 articles. From this group, abstracts were read, and 1,133 were excluded by the screening criteria. Consequently, 54 full-text articles were assessed for eligibility. Finally, after having removed 39 articles by the eligibility criteria, a total of 15 articles were included in this systematic review.
Table 1 shows an overview of the main characteristics of the 15 considered studies (46–60) following the PICO model (40) and highlighting the biomechanical and physiological indexes used. All the articles that met the eligibility criteria are very recent: five were published in 2020, one in 2019, six between 2013 and 2016, and three, the oldest, in 2012.
A total of 1,300 subjects were recruited in the included studies, with 618 males (M) and 612 females (F), and only in one study (60), the gender was not specified. The subjects' mean age varied from <21.36 (57) to 74 (55) years.
A total of eight studies dealt with subjects with low back pain (LBP) (46, 49, 52, 53, 56, 58–60), two studies considered subjects who have survived stroke (SS) (50, 55), one study considered subjects with multiple sclerosis (MS) (48), three subjects with spinal cord injury (SCI) (51, 52, 57), and finally, two addressed healthy subjects (HS) (47, 54).
All studies were carried out in the laboratory (46–60), and three of them also in real-life environments (47, 52, 55).
The following tasks were analyzed (see Table 1):
• lifting task, one study (60);
• rehabilitation exercises, three studies (46, 53, 56);
• daily activities, two studies (47, 55);
• walking task, two studies (47, 48);
• balance, three studies (54, 57, 59);
• reaching and grasping activities, three studies (50, 51, 57);
• typical working activities, one study (52);
• lumbar flexion–extension, one study (49);
• physical performance task, one study (54);
• trunk stability test, one study (58);
• gross arm and fine hand movements, one study (47);
• sitting test, one study (57);
• dexterity task, one study (59).
In total, 41 different kinematic (46–55, 57, 60), 12 kinetic (51, 53, 54, 57), 5 sEMG (46, 56, 59, 60), 3 postural (55, 57), and 4 other indexes (47, 49, 50) were investigated (see Tables 1, 2).
All the outcome parameters of interest are listed and described in Table 2.
In 13 studies, five on LBP (46, 49, 52, 53, 60), two on SS (50, 55), one on MS (48), three on SCI (51, 52, 57), and two on HS (47, 54), 31 kinematic indexes were reported as useful for motor performance assessment (Table 2). More in detail, with regard to the gait: velocity (46, 48, 54), number of steps (55), gait duration (55), cadence (46), step length (46), step time (46), single support time (46), stride length (48), and phase coordination index (48). With regard to other motor tasks, different from gait: limb-use intensity (47), flexion angle (49), bending/flexion speed (49), peak reach velocity (50), reach time (50), contact velocity (50), peak aperture (50), peak grip force (50), fingers range of motion (51), movement duration (52), hand peak velocity (52), torso peak velocity (52), time at torso peak velocity (52), shoulder-to-hand distance at hand peak velocity (52), precedence index (52), lumbar ROM (53), Schober's flexion (53), neck flexion/extension (54), shoulder external rotation (54), hip flexion, internal/external rotation (54), knee flexion/extension (54), virtual time to contact (VTC) (57), and maximum angular displacement (60).
The following kinematic parameters were found to be less significant for characterizing the motor performance of analyzed subjects. Regarding the gait: velocity (55), single support time (48), stance (48), swing (48), double support time (48), and walk distance (54). In other tasks: bending/flexion speed (49), torso travel distance (52), hand travel distance (52), time at hand peak velocity (52), shoulder internal rotation (54), ankle plantar/dorsi flexion (54), and functional boundary (57).
In three studies, one on LBP (53), one on SCI (51), one on HS (54), and five kinetic indexes have been identified as useful for the assessment of motor functions in tasks not including gait: palmar maximum grasp strength (51), pinch maximum grasp strength (51), maximal voluntary isometric torque (53), muscle strength knee flexor/extensor (54), and muscle strength toe flexor (54).
Other kinetic indexes (Table 2) were found to be less significant for characterizing the motor performance of analyzed subjects: muscle strength shoulder internal/external rotators (54), muscle strength elbow flexors/extensor (54), muscle strength hip abductors (54), muscle strength hip internal/external rotators (54), muscle strength ankle plantar flexors/dorsiflexors (54), center of pressure (CoP) velocity (57), and CoP root mean square (RMS) (57).
In three studies (56, 58, 60), four sEMG indexes were identified to be useful results for guiding therapy and determining the level of return to work of subjects with LBP: muscle onset (56), latency time (56), principal component score (58, 60); and EMG ensemble average waveforms (60).
The other sEMG parameter (Table 2), mean muscle activation (59), was found to be less significant for characterizing the motor performance of LBP subjects.
In two studies, three indexes referring to posture were identified, which provide novel information concerning the effectiveness of various rehabilitation approaches for individuals with SS (55) and SCI (57), with the aim of adequate job reintegration: postural transition duration (55), aborted postural transition attempts (55), and instability index (57).
In two studies, one on HS (47) and one on SS (50), three other indexes were identified to be useful for assessing motor performance: mean of magnitude ratio of activity intensity (47), upper-limb performance (47), and reach path ratio (50), while the flexion/extension ratio evaluated in Cimarras-Otal et al. (49) was found to be less significant for characterizing the motor performance of LBP subjects (Table 2).
The results of the risk of bias assessment are reported in the risk of bias summary (Figure 2)—where the authors' judgments are shown for all the seven considered domains and for each study included in this review, according to Higgins and Green (43), McGuinness (44), and Sterne et al. (45)—and in the risk of bias graph (Figure 3), where the authors' judgments are reported for each risk of bias as percentages across the different studies included in this review.
Figure 2. Risk of bias summary: authors' judgments for 15 included studies and for each considered domain.
Figure 3. Risk of bias graph: authors' judgments for each risk of bias reported as percentages of the different studies included in the review.
None of the studies considered was associated with the risk of bias due to confounding (D1), in classifications of interventions (D3), due to deviations from intended interventions (D4), due to missing data (D5), and in selection of the reported result (D7).
Instead, there was a moderate risk of bias due to participant selection (D2) in a single study (47), owing to a poor description of the subjects involved. Furthermore, due to a lack of results for some of the subjects studied, three studies (49, 53, 56) had a moderate risk of bias in outcome measurement (D6).
This systematic review evaluates which biomechanical and physiological indexes present in the literature are the most appropriate to evaluate the effectiveness and efficiency of workplace rehabilitation and work integration interventions for people with neuromuscular disorders that frequently face issues with employment, work difficulties, and premature work interruptions. To reach this aim, the authors found only 15 eligible articles (46–60).
The characteristics of the experimental procedures of these eligible articles suggested some interesting considerations. The samples involved in the studies were constituted by males (618) and females (612) equally, suggesting that findings related to the biomechanical and physiological indexes of motor performance can be generalized for gender. Regarding the age, all the included studies were on people of working age (18 < years <67), and we can affirm that the finding of this study can be generalized for the working age because the age range considered is wide: the subjects' mean age varied from <21.36 (57) up to 74 (55) years.
All the articles resulting from the selection process were published in the last decade, the oldest (three articles) were published in 2012 (50, 53, 56) and the most recent (five articles) in 2020 (48, 49, 51, 54, 59), and this suggests that there is a growing interest in the scientific community in this issue.
Regarding the investigated population, eight studies were on subjects with LBP (46, 49, 52, 53, 56, 58–60), three on spinal cord injury SCI (51, 52, 57), two studies on SS (50, 55), only one study on MS (48), and finally two on HS (47, 54). This result suggests that these biomechanical and physiological indexes of motor performance were used especially for job integration/reintegration of people with back pain disorders (eight studies on 15). This could be explained considering that low-back disorders are the highest incidence that disturbs workers (61–65) with economic cost (in terms of days away from work and work compensation costs) and impact on quality of life that many ergonomic interventions have been proposed during the past 3 decades to mitigate them. Indeed, there were many studies on other pathologies [i.e., Duchenne muscular dystrophy (66), Parkinson (67, 68)], and the indexes not being effectively used for a return to work. This aspect is very important because it suggests the possibility of working on the application of these indexes to facilitate the return to work of people with disabilities.
Furthermore, all the studies were performed in laboratory (46–60), and only three of them also in environments of real life (47, 52, 55). This aspect suggests that in the attempt to investigate the effectiveness of using these indexes in workplaces, measurements should be confirmed/tested in a real work environment.
There are many different work tasks that have been reproduced in the laboratory to evaluate the effectiveness of the different indexes investigated for the purpose of job insertion/re-insertion of individuals with these disorders. In fact, as can be seen in Table 1, 13 different tasks from lifting to walking through reaching and grasping and fine movements were reproduced, but each task was reproduced in one [e.g., lifting (60) and typical working activities (52)], two [e.g., daily activities (47, 55) and walking task (47, 48)], or at most three articles [e.g., balance (54, 57, 59), reaching and grasping activities (50, 51, 57)]. This reflects the wide variety of work tasks and the equal distribution among them.
In these tasks, a total of 41 different kinematic indexes were considered, and 31 of them were found to be useful for motor performance assessment (Table 2).
Furthermore, in addition to the numerous kinematic parameters, these studies investigated other parameters: five kinetic, three postural, and three other (see Tables 1, 2).
This shows the kinematic parameters for quantitative biomechanical indexes are useful and already widely used, while kinetic, postural, and surface electromyography are still underutilized in this area, despite their proven validity and necessity. In fact, when a worker with a neurological pathology and motor disorders returns to work, it is critical to conduct a thorough assessment of his/her residual motor function in order to properly construct and/or modify his/her workplace (3). The wide use of kinematics is likely to be associated with easier use even directly in the work environment, thanks to wearable technologies that are becoming increasingly popular in recent years and that are also easy to use even with user-friendly interfaces. On the other hand, kinetic and postural parameters are still very much constrained to the laboratory environment, even though sensorized shoes are beginning to become more widespread that can replace force platforms in the work environment (69). The critical issues hindering the widespread adoption of sEMG in return-to-work programs are mainly attributable to technical (both monitoring and control functions), methodological, and cultural limitations (3). Regarding monitoring issues, the most critical technical aspects are strongly associated with the sEMG technique such as electrode–skin impedance, noise, and electrode contact stability), while the methodological aspects are associated with
• problems linked to electrode location, size, configuration, and distance;
• presence of crosstalk signals (70);
• placement of sEMG electrodes for long hours;
• selection of the right sensor setup on the base of the neurological pathology and manual handling activity to be investigated;
• management of the sEMG amplitude normalization;
• definition of appropriate sEMG-related outcomes and normative data (3).
Fortunately, with the help of reliable reference materials and tutorials, the impact of these significant difficulties on the sEMG signal quality can be decreased. Particularly, the European Recommendations for Surface Electromyography (71), which needs to be updated, and the Atlas of Muscle Innervation Zones (72) are recommended as guides for the use of sEMG, together with recent tutorials and consensus articles (73–75). Users who are familiar with the contents of these publications and tutorials are aware of the current limits of the sEMG technique, which can only monitor a small number of superficial muscles. Despite this, the technical and methodological shortcomings of the sEMG approach can be addressed, and there is no justification for not using it in return-to-work rehabilitation plans. Furthermore, modern wearable sensors and electronic smart devices such as smartphones and tablets provide convenient workplace monitoring.
The existence of this educational gap is clearly attested to by the global social policies for people with disabilities (76, 77), such as the European Disability Strategy (2010–2020) and the directives 89/654/EEC, 2000/78/EC, and 2000/78/EC. Another example of this education gap is the Job Accommodation Network, which is part of the U.S. Department of Labor Office of Disability Employment Policy and provides a valuable method for including persons with neurological disorders in the workforce (78). Several educational activities should be established to enhance and use the best abilities available in rehabilitation engineering, physiotherapy, occupational therapy, and ergonomics to bridge the gap between the return-to-work idea and other disciplines. Scientific societies in the fields of physiotherapy, ergonomics, occupational medicine, biomechanics, electrophysiology, and kinesiology should promote or, at the very least, supervise and monitor bachelor, master, and PhD programs, and should include continuing education courses on the use of the management of the monitoring and HRC technologies and instrumental recordings (i.e., kinematic, kinetic, and sEMG) specifically geared to teachers in these fields (3). Furthermore, it is absolutely necessary to also include these quantitative instrumental approaches in the international ergonomic standards (79–82).
The bias affecting these results is low overall (Figure 2), so the results can be considered reliable. In fact, only three articles of 15 showed moderate bias (49, 53, 56), and two articles (53, 56) of these three are quite old, both published in 2012.
The examined articles do not refer to the experts who conducted the analyses or who will be required to do so in future, except in four articles where they mentioned physiotherapists, physiatrists, and psychologists (46, 53, 56, 60). We believed that the professionals trained for innovative job accommodation programs, however, should be occupational health and safety technicians and ergonomists who work in a multidisciplinary team also made up of neurologists, occupational physicians, physiatrists, physiotherapists, biomedical engineers, and movement scientists.
In summary, the results of this review show that quantitative biomechanical and physiological indexes are good tools to be used for job integration/reintegration and widely used the kinematic ones, and the electromyographic ones still little used. Indeed, by contributing to prevent the loss of employment and improve overall quality of life, the use of appropriate indexes for motor monitoring in job integration programs of people with neuromuscular diseases will allow to assess the effectiveness of the ergonomic interventions (1, 3). In addition, their utilization will enable more comprehensive job accommodation plans that can incorporate workplace and work task rehabilitation (35–37). The Industry 4.0 era allows for the deployment of human–robot collaboration (HRC) technologies that may support people in real time at their workplaces based on their motor requirements. In this setting, wearable miniaturized sensors, kinematic, kinetic, and sEMG-based indexes can be used to control collaborative robots; classify residual motor functions; and assess pre–post-rehabilitation and ergonomic therapies.
The main limitations of this systematic review are related to the small number of articles that resulted in being eligible, which was mainly due to the narrow eligibility criteria oriented to the occupational field and the quantitative biomechanical indexes. The number of articles grows significantly if we also consider studies based on qualitative (i.e., studies with only questionnaires) and not quantitative indexes and if we do not limit the application to the work environment. Furthermore, another limitation is that the systematic review was not registered in PROSPERO (https://www.crd.york.ac.uk/prospero/).
The original contributions presented in the study are included in the article/supplementary materials, further inquiries can be directed to the corresponding author.
GC, LF, AT, TV, FD, and AR planned the manuscript, performed the literature searches, wrote the text, and revised the manuscript. All authors contributed to the article and approved the submitted version.
The research presented in this article was carried out as part of the SOPHIA Project, which has received funding from the European Union's Horizon 2020 Research and Innovation Program under Grant Agreement No. 871237.
The authors declare that the research was conducted in the absence of any commercial or financial relationships that could be construed as a potential conflict of interest.
All claims expressed in this article are solely those of the authors and do not necessarily represent those of their affiliated organizations, or those of the publisher, the editors and the reviewers. Any product that may be evaluated in this article, or claim that may be made by its manufacturer, is not guaranteed or endorsed by the publisher.
1. Miller A, Dishon S. Health-related quality of life in multiple sclerosis: the impact of disability, gender and employment status. Qual Life Res. (2006) 15:259–71. doi: 10.1007/s11136-005-0891-6
2. Klockgether T. Sporadic ataxia with adult onset: classification and diagnostic criteria. Lancet Neurol. (2010) 9:94–104. doi: 10.1016/S1474-4422(09)70305-9
3. Ranavolo A, Serrao M, Draicchio F. Critical issues and imminent challenges in the use of sEMG in return-to-work rehabilitation of patients affected by neurological disorders in the epoch of Human–Robot collaborative technologies. Front Neurol. (2020) 11:572069. doi: 10.3389/fneur.2020.572069
4. Persechino B, Fontana L, Buresti G, Fortuna G, Valenti A, Iavicoli S. Improving the job-retention strategies in multiple sclerosis workers: the role of occupational physicians. Ind Health. (2019) 57:52–69. doi: 10.2486/indhealth.2017-0214
5. Benedict RH, Wahlig E, Bakshi R, Fishman I, Munschauer F, Zivadinov R, et al. Predicting quality of life in multiple sclerosis: accounting for physical disability, fatigue, cognition, mood disorder, personality, and behavior change. J Neurol Sci. (2005) 231:29–34. doi: 10.1016/j.jns.2004.12.009
6. Ranavolo A, Serrao M, Varrecchia T, Casali C, Filla A, Roca A, et al. The working life of people with degenerative cerebellar ataxia. Cerebellum. (2019) 18:910–21. doi: 10.1007/s12311-019-01065-x
7. Henderson M, Harvey SB, Overland S, Mykletun A, Hotopf M. Work and common psychiatric disorders. J R Soc Med. (2011) 104:198–207. doi: 10.1258/jrsm.2011.100231
8. Matt SB, Butterfield P. Changing the disability climate: promoting tolerance in the workplace. AAOHN J. (2006) 54:129–35. doi: 10.1177/216507990605400306
9. Gates LB. Workplace accommodation as a social process. J Occup Rehabil. (2000) 10:1. doi: 10.1023/A:1009445929841
10. Merz MA, Bricout JC, Koch LC. Disability and job stress: implications for vocational rehabilitation planning. Work. (2001) 17:85–95.
11. Frank AO, Sawney P. Vocational rehabilitation. J R Soc Med. (2003) 96:522–4. doi: 10.1258/jrsm.96.11.522
12. Reneman MF. State of vocational rehabilitation and disability evaluation in chronic musculoskeletal pain conditions. In: Escorpizo R, Brage S, Homa D, Stucki G, editors. Handbook of Vocational Rehabilitation and Disability Evaluation. Handbooks in Health, Work, and Disability. Cham: Springer (2015). p. 187–98.
13. Staubli S, Schwegler U, Schmitt K, Trezzini B. ICF-based process management in vocational rehabilitation for people with spinal cord injury. In: Escorpizo R, Brage S, Homa D, Stucki G, editors. Handbook of Vocational Rehabilitation and Disability Evaluation. Handbooks in Health, Work, and Disability. Cham: Springer (2015). p. 371–96.
14. Bond GR, Campbell K, Becker DR. A test of the occupational matching hypothesis for rehabilitation clients with severe mental illness. J Occup Rehabil. (2013) 23:261–9. doi: 10.1007/s10926-012-9388-7
15. Escorpizo R, Reneman MF, Ekholm J, Fritz J, Krupa T, Marnetoft SU, et al. conceptual definition of vocational rehabilitation based on the ICF: building a shared global model. J Occup Rehabil. (2011) 21:126–33. doi: 10.1007/s10926-011-9292-6
16. Schultz IZ, Stowell AW, Feuerstein M, Gatchel RJ. Models of return to work for musculoskeletal disorders. J Occup Rehabil. (2007) 17:327–52. doi: 10.1007/s10926-007-9071-6
17. Isernhagen SJ. Job matching and return to work: occupational rehabilitation as the link. Work. (2006) 26:237–42.
18. Frank A. Vocational rehabilitation: supporting ill or disabled individuals in (to) work: a UK perspective. Healthcare. (2016) 4:46. doi: 10.3390/healthcare4030046
19. Serrao M, Chini G, Casali C, Conte C, Rinaldi M, Ranavolo A, et al. Progression of gait ataxia in patients with degenerative cerebellar disorders: a 4-year follow-up study. Cerebellum. (2017) 16:629–37. doi: 10.1007/s12311-016-0837-2
20. Jacobi H, du Montcel ST, Bauer P, Giunti P, Cook A, Labrum R, et al. Long-term disease progression in spinocerebellar ataxia types 1, 2, 3, and 6: a longitudinal cohort study. Lancet Neurol. (2015) 14:1101–8. doi: 10.1016/S1474-4422(15)00202-1
21. Dubbioso R, Pellegrino G, Antenora A, De Michele G, Filla A, Santoro L, et al. The effect of cerebellar degeneration on human sensori-motor plasticity. Brain Stimul. (2015) 8:1144–50. doi: 10.1016/j.brs.2015.05.012
22. Serrao M, Chini G, Bergantino M, Sarnari D, Casali C, Conte C, et al. Dataset on gait patterns in degenerative neurological diseases. Data Brief. (2017) 16:806es.c doi: 10.1016/j.dib.2017.12.022
23. Vinci P, Serrao M, Millul A, Deidda A, De Santis F, Capici S, et al. Quality of life in patients with Charcot-Marie-Tooth disease. Neurology. (2005) 65:922–24. doi: 10.1212/01.wnl.0000176062.44360.49
24. Doogan C, Playford ED. Supporting work for people with multiple sclerosis. Mult Scler. (2014) 20:646–50. doi: 10.1177/1352458514523499
25. Raggi A, Covelli V, Schiavolin S, Scaratti C, Leonardi M, Willems M. Work-related problems in multiple sclerosis: a literature review on its associates and determinants. Disabil Rehabil. (2016) 38:936–44. doi: 10.3109/09638288.2015.1070295
26. Hendricks DJ, Sampson E. Accommodating individuals with traumatic brain injury: an analysis of employer-initiated cases handled by the Job Accommodation Network. Work. (2017) 58:29–34. doi: 10.3233/WOR-172594
27. van der Kemp J, Kruithof WJ, Nijboer TCW, van Bennekom CAM, van Heugten C, Visser-Meily JMA. Return to work after mild-to-moderate stroke: work satisfaction and predictive factors. Neuropsychol Rehabil. (2019) 29:638–53. doi: 10.1080/09602011.2017.1313746
28. Toldrá RC, Santos MC. People with disabilities in the labor market: facilitators and barriers. Work. (2013) 45:553–63. doi: 10.3233/WOR-131641
29. Honan CA, Brown RF, Hine DW, Vowels L, Wollin JA, Simmons RD, et al. The multiple sclerosis work difficulties questionnaire. Mult Scler. (2012) 18:871–80. doi: 10.1177/1352458511431724
30. Karamians R, Proffitt R, Kline D, Gauthier LV. Effectiveness of virtual reality- and gaming-based interventions for upper extremity rehabilitation poststroke: a meta-analysis. Arch Phys Med Rehabil. (2020) 101:885–96. doi: 10.1016/j.apmr.2019.10.195
31. Triegaardt J, Han TS, Sada C, Sharma S, Sharma P. The role of virtual reality on outcomes in rehabilitation of Parkinson's disease: meta-analysis and systematic review in 1031 participants. Neurol Sci. (2020) 41:529–36. doi: 10.1007/s10072-019-04144-3
32. Naro A, Leo A, Russo M, Casella C, Buda A, Crespantini A, et al. Breakthroughs in the spasticity management: are non-pharmacological treatments the future? J Clin Neurosci. (2017) 39:16–27. doi: 10.1016/j.jocn.2017.02.044
33. Iosa M, Morone G, Cherubini A, Paolucci S. The three laws of neurorobotics: a review on what neurorehabilitation robots should do for patients and clinicians. J Med Biol Eng. (2016) 36:1–11. doi: 10.1007/s40846-016-0115-2
34. Mangone M, Agostini F, de Sire A, Cacchio A, Chiaramonte A, Butterini G, et al. Effect of virtual reality rehabilitation on functional outcomes for return-to-work patients with Parkinson's disease: an umbrella review of systematic reviews. NeuroRehabilitation. (2022) 1–11. doi: 10.3233/NRE-220029
35. Ashley KD, Lee LT, Heaton K. Return to work among stroke survivors. Workplace Health Saf. (2019) 67:87–94. doi: 10.1177/2165079918812483
36. Skamagki G, King A, Duncan M, Wåhlin C. A systematic review on workplace interventions to manage chronic musculoskeletal conditions. Physiother Res Int. (2018) 23:e1738. doi: 10.1002/pri.1738
37. Hoosain M, de Klerk S, Burger M. Workplace-based rehabilitation of upper limb conditions: a systematic review. J Occup Rehabil. (2019) 29:175–93. doi: 10.1007/s10926-018-9777-7
38. Moher D, Shamseer L, Clarke M, Ghersi D, Liberati A, Petticrew M, et al. Preferred reporting items for systematic review and meta-analysis protocols (PRISMA-P) 2015 statement. Syst Rev. (2015) 4:1. doi: 10.1186/2046-4053-4-1
39. Grant MJ, Booth A. A typology of reviews: an analysis of 14 review types and associated methodologies. Health Info Libr J. (2009) 26:91–108. doi: 10.1111/j.1471-1842.2009.00848.x
40. Leonardo R. PICO model for clinical questions. Evid Based Med Pract. (2018) 3:2. doi: 10.4172/2471-9919.1000115
41. Del Ferraro S, Falcone T, Ranavolo A, Molinaro V. The effects of upper-body exoskeletons on human metabolic cost and thermal response during work tasks-a systematic review. Int J Environ Res Public Health. (2020) 17:7374. doi: 10.3390/ijerph17207374
42. Falcone T, Cordella F, Molinaro V, Zollo L, Del Ferraro S. Real-time human core temperature estimation methods and their application in the occupational field: a systematic review. Measurement. (2021) 183:109776. doi: 10.1016/j.measurement.2021.109776
43. Higgins J, Green S. Cochrane handbook for systematic reviews of interventions. Cochrane Handb Syst Rev Interv. (2019). doi: 10.1002/9781119536604
44. McGuinness LA. Robvis: An R Package and Web Application for Visualising Risk-of-bias Assessments. Available online at: https://github.com/mcguinlu/robvis (accessed March 17, 2020).
45. Sterne JA, Hernán MA, Reeves BC, Savović J, Berkman ND, Viswanathan M, e al. ROBINS-I: a tool for assessing risk of bias in non-randomised studies of interventions. BMJ. (2016) 12:bmj.14919. doi: 10.1136/bmj.i4919
46. Monticone M, Ambrosini E, Rocca B, Magni S, Brivio F, Ferrante S, et al. multidisciplinary rehabilitation programme improves disability, kinesiophobia and walking ability in subjects with chronic low back pain: results of a randomised controlled pilot study. Eur Spine J. (2014) 23:2105–13. doi: 10.1007/s00586-014-3478-5
47. Lee SI, Liu X, Rajan S, Ramasarma N, Choe EK, Bonato P, et al. novel upper-limb function measure derived from finger-worn sensor data collected in a free-living setting. PLoS One. (2019) 14:e0212484. doi: 10.1371/journal.pone.0212484
48. Richmond SB, Swanson CW, Peterson DS, Fling BW. A temporal analysis of bilateral gait coordination in people with multiple sclerosis. Mult Scler Relat Disord. (2020) 45:102445. doi: 10.1016/j.msard.2020.102445
49. Cimarras-Otal C, Marcen-Cinca N, Rabal-Pelay J, Lacrcel-Tejero B, Alczar-Crevilln A, Villalba-Ruete J, et al. Adapted exercises versus general exercise recommendations on chronic low back pain in industrial workers: a randomized control pilot study. Work. (2020) 67:733–40. doi: 10.3233/WOR-203322
50. Schaefer S, DeJong S, Cherry K, Lang C. Grip type and task goal modify reach-to-grasp performance in post-stroke hemiparesis. Motor Control. (2012) 16:2. doi: 10.1123/mcj.16.2.245
51. Correia C, Nuckols K, Wagner D, Zhou YM, Clarke M, Orzel D, et al. Improving grasp function after spinal cord injury with a soft robotic glove. IEEE Trans Neural Syst Rehabil Eng. (2020) 28:1407–15. doi: 10.1109/TNSRE.2020.2988260
52. Kim K, Martin B. Manual movement coordination adapted to spinal cord injury and low back pain. Int J Ind Ergon. (2013) 43:1–8. doi: 10.1016/j.ergon.2012.10.002
53. Bruce-Low S, Smith D, Burnet S, Fisher J, Bissell G, Webster L. One lumbar extension training session per week is sufficient for strength gains and reductions in pain in patients with chronic low back pain ergonomics. Ergonomics. (2012) 55:500–07. doi: 10.1080/00140139.2011.644329
54. Lebde N, Burns J, Mackey M, Baldwin J, McKay M. Normative reference values and physical factors associated with work ability: a cross-sectional observational study. Occup Environ Med. (2020) 77:231–7. doi: 10.1136/oemed-2019-106248
55. Taylor-Piliae RE, Mohler MJ, Najafi B, Coull BM. Objective fall risk detection in stroke survivors using wearable sensor technology: a feasibility study. Top Stroke Rehabil. (2016) 23:393–9. doi: 10.1179/1074935715Z.00000000059
56. Brooks C, Kennedy S, Marshall P. Specific trunk and general exercise elicit similar changes in anticipatory postural adjustments in patients with chronic low back pain: a randomized controlled trial. Spine. (2012) 37:E1543–50. doi: 10.1097/BRS.0b013e31826feac0
57. Shin S, Sosnoff JJ. Spinal cord injury and time to instability in seated posture. Arch Phys Med Rehabil. (2013) 94:1615–20. doi: 10.1016/j.apmr.2013.02.008
58. Moreside JM, Quirk DA, Hubley-Kozey CL. Temporal patterns of the trunk muscles remain altered in a low back-injured population despite subjective reports of recovery. Arch Phys Med Rehabil. (2014) 95:686–98. doi: 10.1016/j.apmr.2013.10.003
59. Rowley KM, Engel T, Kulig K. Trunk and hip muscle activity during the Balance-Dexterity task in persons with and without recurrent low back pain. J Electromyogr Kinesiol. (2020) 50:102378. doi: 10.1016/j.jelekin.2019.102378
60. Hubley-Kozey C, Moreside JM, Quirk DA. Trunk neuromuscular pattern alterations during a controlled functional task in a low back injured group deemed ready to resume regular activities. Work. (2014) 47:87–100. doi: 10.3233/WOR-131689
61. Dick RB, Lowe BD, Lu ML, Krieg EF. Trends in work-related musculoskeletal disorders from the 2002 to 2014 general social survey, quality of work life supplement. J Occup Environ Med. (2020) 62:595–610. doi: 10.1097/JOM.0000000000001895
62. INAIL. Italian Worker's Compensation Authority Annual Report. Part IV. Statistics, Accidents and Occupational Diseases. Italy: INAIL (2011).
63. Kim KH, Kim KS, Kim DS, Jang SJ, Hong KH, Yoo SW. Characteristics of work-related musculoskeletal disorders in Korea and their work-relatedness evaluation. J Kor Med Sci. (2010) 25(Suppl):S77–86. doi: 10.3346/jkms.2010.25.S.S77
64. Okello A, Wafula ST, Sekimpi DK, Mugambe RK. Prevalence and predictors of work-related musculoskeletal disorders among workers of a gold mine in south Kivu, Democratic Republic of Congo. BMC Muscoskel Disord. (2020) 21:797. doi: 10.1186/s12891-020-03828-8
65. Violante FS. Criteria for diagnosis and attribution of an occupational musculoskeletal disease. Med Lavoro. (2020) 111:249–68.
66. Fujii T, Takeshita E, Iwata Y, Yajima H, Nozaki F, Mori M, et al. Cumulative jerk as an outcome measure in nonambulatory Duchenne muscular dystrophy. Brain Dev. (2019) 41:796–802. doi: 10.1016/j.braindev.2019.06.002
67. Pepa L, Ciabattoni L, Verdini F, Capecci M, Ceravolo MG. Smartphone based fuzzy logic freezing of gait detection in parkinson's disease. In: IEEE/ASME 10th International Conference on Mechatronic and Embedded Systems and Applications. Senigallia: IEEE (2014). p. 1–6.
68. Arippa F, Pau M, Cimolin V, Stocchi F, Goffredo M, Franceschini M, et al. novel summary kinematic index for postural characterization in subjects with Parkinson's disease. Eur J Phys Rehabil Med. (2020) 56:142–7. doi: 10.23736/S1973-9087.19.05803-9
69. Ranavolo A, Draicchio F, Varrecchia T, Silvetti A, Iavicoli S, Alberto R, et al. Erratum: wearable monitoring devices for biomechanical risk assessment at work: current status and future challenges-a systematic review. Int J Environ Res Public Health. (2018) 15:2569. doi: 10.3390/ijerph15112569
70. Merletti R, Parker P. Electromyography—Physiology, Engineering and Noninvasive Applications. 1st ed. Hoboken, NJ: John Wiley & Sons, Inc. (2004).
71. Hermens HJ, Freriks B. European Recommendations for Surface Electromyography: Results of the SENIAM Project. Enschede: Roessingh Research and Development (1999).
72. Barbero M, Merletti R, Rainoldi A. Atlas of Muscle Innervation Zones: Understanding Surface Electromyography and its Applications. New York, NY: Springer (2012).
73. Besomi M, Hodges PW, Van Dieën J, Carson RG, Clancy EA, et al. Consensus for experimental design in electromyography (CEDE) project: electrode selection matrix. J Electromyogr Kinesiol. (2019) 48:128–44. doi: 10.1016/j.jelekin.2019.07.008
74. Merletti R, Muceli S. Tutorial Surface EMG detection in space and time: best practices. J Electromyogr Kinesiol. (2019) 49:102363. doi: 10.1016/j.jelekin.2019.102363
75. Merletti R, Cerone GL. Tutorial Surface EMG detection, conditioning and pre-processing: best practices. J Electromyogr Kinesiol. (2020) 54:102440. doi: 10.1016/j.jelekin.2020.102440
76. Krahn GL. WHO World report on disability: a review. Disabil Health J. (2011) 4:141–2. doi: 10.1016/j.dhjo.2011.05.001
77. de Jong PR. Sickness, disability and work: breaking the barriers—a synthesis of findings across OECD countries—OECD. Int Rev für Soz Sicherheit. (2011) 64:117–9. doi: 10.1111/j.1752-1726.2011.01406.x
78. Copaci D, Serrano D, Moreno L, Blanco D. A high-level control algorithm based on sEMG signalling for an elbow joint SMA exoskeleton. Sensors. (2018) 18:2522. doi: 10.3390/s18082522
81. ISO/DIS 11228-3 Ergonomics—Manual Handling—Part 3: Handling of Low Loads at High Frequency. ISO: Geneva (2007).
Keywords: indexes, job reintegration, neurological disorders, monitoring, performance, biomechanics, ergonomics
Citation: Chini G, Fiori L, Tatarelli A, Varrecchia T, Draicchio F and Ranavolo A (2022) Indexes for motor performance assessment in job integration/reintegration of people with neuromuscular disorders: A systematic review. Front. Neurol. 13:968818. doi: 10.3389/fneur.2022.968818
Received: 14 June 2022; Accepted: 08 August 2022;
Published: 08 September 2022.
Edited by:
Andrea Martinuzzi, Eugenio Medea (IRCCS), ItalyReviewed by:
Teresa Paolucci, University of Studies G. d'Annunzio Chieti and Pescara, ItalyCopyright © 2022 Chini, Fiori, Tatarelli, Varrecchia, Draicchio and Ranavolo. This is an open-access article distributed under the terms of the Creative Commons Attribution License (CC BY). The use, distribution or reproduction in other forums is permitted, provided the original author(s) and the copyright owner(s) are credited and that the original publication in this journal is cited, in accordance with accepted academic practice. No use, distribution or reproduction is permitted which does not comply with these terms.
*Correspondence: Giorgia Chini, Zy5jaGluaUBpbmFpbC5pdA==
†These authors have contributed equally to this work and share first authorship
Disclaimer: All claims expressed in this article are solely those of the authors and do not necessarily represent those of their affiliated organizations, or those of the publisher, the editors and the reviewers. Any product that may be evaluated in this article or claim that may be made by its manufacturer is not guaranteed or endorsed by the publisher.
Research integrity at Frontiers
Learn more about the work of our research integrity team to safeguard the quality of each article we publish.