- Université de Paris, INSERM NeuroDiderot, Paris, France
Rett Syndrome (RTT, OMIM 312750), a unique rare neurodevelopmental disorder, mostly affects females and causes severe multi-disabilities including poor sleep. This meta-analysis systematically reviewed the polysomnographic (PSG) data of individuals with RTT on both sleep macrostructure and sleep respiratory indexes and compared them to literature normative values. Studies were collected from PubMed, Web of Science, PsycINFO, Ebsco, Scopus, and Cochrane Library till 26 April 2022. Across 13 included studies, the 134 selected RTT cases were mostly females being MECP2 (n = 41) and CDKL5 (n = 4) positive. They were further stratified by gene, age, and clinical features. Findings of comparison with literature normative values suggested shorter total sleep time (TST) and sleep onset latency (SOL), twice as long wake after sleep onset (WASO) with lower sleep efficiency (SEI) in RTT, as well as increased non-rapid eye movement stage 3 (stage N3) and decreased rapid eye movement sleep. Based on limited data per stratifications, we found in RTT cases <5 years old lower stage N3, and in RTT cases >5 years old less WASO and more WASO in the epileptic strata. However, meta-results generated from studies designed with comparison groups only showed lower stage N1 in RTT than in healthy comparison, together with similar SEI and stage N3 to primary snoring subjects. For sleep respiratory indexes, severe disordered sleep breathing was confirmed across roughly all RTT strata. We are the first study to meta-analyze PSG data of subjects with RTT, illustrating shorter TST and aberrant sleep staging in RTT that may vary with age or the presence of epilepsy. Severe nocturnal hypoxemia with apneic events was also demonstrated. More studies are needed to explore and elucidate the pathophysiological mechanisms of these sleep findings in the future.
Systematic review registration: https://www.crd.york.ac.uk/prospero/display_record.php?RecordID=198099, identifier: CRD 42020198099.
Introduction
Rett Syndrome (RTT, OMIM 312750) is a rare neurodevelopmental disorder with an approximate incidence of 1/10,000 in women (1). Classic RTT is characterized by stagnation and regression following a 6- to−18 month near-normal developmental period. Prominent features of RTT include loss of acquired hand skills and spoken language, the appearance of stereotypic movements, and gait or motor disabilities (2–4). Atypical RTT is symptomatically varied and named accordingly, such as preserved speech variant (PSV), early seizure onset variant (ESV), congenital variants (CV), and not otherwise specified atypical RTT (NOS-ARTT) (1). The causes of RTT have been strongly linked to the mutations in the gene encoding methyl-CpG-binding protein-2 (MECP2) on chromosome Xq28 (5). Other genetic candidates identified in RTT variants encode forkhead box protein G1 (FOXG1) on chromosome 14q13 (6) and cyclin-dependent kinase-like 5 (CDKL5) on chromosome Xq22.13 (7, 8). Hence, RTT is mainly found in girls. Over the years, diagnosis of RTT is primarily based on the presence or absence of criteria related to their cardinal clinical features (1, 2, 9, 10). Other signs also described in the RTT clinical profile include growth retardation, scoliosis, impaired sleep pattern, epilepsy, breathing disturbance, and autonomic abnormalities.
Previous studies (11–14) reported problematic sleeping in RTT such as frequent night waking [i.e., > 80% (15)] or night laughing [i.e., 77% (16)]. In fact, impaired sleep pattern was added to the supportive criteria since 2002 (9, 17, 18). In recent years, polysomnographic (PSG) studies in RTT have predominantly sought to record and describe sleep (19–22) from a clinical perspective. Regarding the sleep macrostructure, previous studies revealed prominently reduced rapid eye movement (REM) sleep and poor sleep efficiency in RTT (20, 23, 24). Although the breathing disturbances during wake state are well-recognized (25, 26), the findings during the sleep phase were inconsistent (19–21, 27), such that early studies reported normal sleep breathing pattern in RTT.
There is no meta review on PSG of RTT to date. We aimed to summarize the PSG parameters of sleep structure and sleep breathing events in RTT and to assess their differences when compared to normative values from a typically developing (TD) population reported in the literature. We explored additional analyses with respect to RTT features such as genes, age, and the presence of certain clinical features when reported to further adequately document the sleep characteristics in RTT.
Methods
We followed the PRISMA 2009 reporting guideline (28) for this meta-review, which was registered in PROSPERO (CRD 42020198099). The quality of each study was scored via the Study Quality Assessment Tools of the National Institutes of Health (NIH) (29) by both authors. This tool applies to several study designs. We followed the same approach as published (30, 31). Study quality was regrouped on four domains: “study population, definition and selection,” “soundness of information,” “analysis, comparability, and outcomes,” and “interpretation and reporting,” and evaluated as poor, fair, and good. Disagreement in selection, extraction, and quality scoring was resolved by discussion.
Search strategy and selection criteria
A systematic search was performed in PubMed, Web of Science, PsycINFO, Ebsco, Scopus, and Cochrane Library to 26 April 2022 (Figure 1) with the search terms: “Sleep AND Rett Syndrome” (see Supplementary material S1 for more details). Both authors screened and selected PSG (-related) studies on RTT individuals.
Studies were selected when fulfilling the following criteria: (1) original articles published in peer-reviewed journals; (2) RTT clinical or genetic diagnosis reported; (3) PSG data on sleep macrostructure and respiratory parameters (e.g., EEG spectrum analysis on sleep macrostructure was also included) printed numerically or graphically, which could be measured as numerical data. No time limitations or study design restrictions were applied. Studies would be excluded if on animal research (32–46) or if participants were RTT individuals with other central nervous system complications (e.g., neurofibroma) (47) or sleep intervention [e.g., PSG data from RTT cases after adeno(tonsil)ectomy surgery (A&T) history].
Data collection and analysis
Demographic and methodologic information of studies including authors, sample characteristics, study design, PSG application, and conclusion were extracted.
Next, conventional PSG parameters were extracted and categorized into similar scoring methods (18). The definitions are listed in Table 1. For the studies that reported such information graphically, we measured and transferred data into numbers by a WebPlotDigitizer.
All available data were organized as the number of subjects (n), mean, and standard deviation (SD) for meta-analysis. In the publications reporting on cases series, sample mean and SD were calculated to represent the total group, or they were reported in subgroups when stratification was possible. Incompletely reported data and single case studies were excluded from the meta-analysis.
Statistical analysis
Meta-analysis was performed in software Statistica TIBCO Software Inc. (48) version 13 and Meta-analysis with Comprehensive Meta-Analysis version 3.3.070 (Biostat, Englewood, NJ). Our approach consists of two parts.
In the first part, we summarized the extracted data to generate an average reference for RTT, i.e., the effect size (ES) is a pooled mean. These pooled means were subsequently compared to normative values of a TD population from the literature (49, 50) by a standardized mean difference test (SMDTD).
In the second part, in those studies that compared RTT samples to a comparison group, we performed SMDcomparison as ES on the summarized data.
For all the meta-analyses, random effects models were chosen, and the ES was illustrated by forest plots, with the size of the gray square showing the relative weight and the extent representing the 95% confidence intervals (95% CI). Particularly for the forest plots including the SMDTD, the range of normative values will be displayed as reference lines in red. Regarding the inconsistency across studies, Q-test and I2 (i.e., roughly 0 ≤ I2 ≤ 40%: might not be important, 40% < I2 ≤ 75%: may represent moderate heterogeneity, I2 > 75%: considerable heterogeneity) were applied to assess heterogeneity. The variance of the ES across the population of studies was reported by Tau2 (τ2). We performed a sensitivity analysis on RTT characteristics (e.g., gene, age, and the presence of certain clinical features). To test the robustness of our findings, Begg's correlation rank test was used for assessing the risk of publication bias. For all statistical analyses, P < 0.05 was set for statistical significance.
Results
Study selection and characteristics
Thirteen articles reported PSG data of subjects with RTT and fulfilled the criteria for our meta-review (Figure 1). Their summary of study design, participants, sleep assessment, NIH quality score, and the general conclusion is presented in Table 2. These 13 studies were published from 1985 to 2019 from six countries, with the majority being from the United States of America (i.e., six). Regarding the study design, seven case series and six observational studies with a cross-sectional design were included. Only seven studies reported specific diagnostic approaches, clinically and/or genetically. Regarding the sleep assessment, PSG (i.e., in 11 studies), video-polygraph (i.e., in one study), and EEG (i.e., in one study) were found, of which five followed the guidelines of American Academic Sleep Medicine (18) and two followed Rechtschaffen and Kales (58) scoring.
We extracted in total 134 RTT cases aged from one to 33 years old and with sample sizes ranging from 2 to 30 subjects. Nine cases from three studies were excluded because their PSG was recorded after A&T surgery.
For the second part, five studies had a comparison group of a total of 122 subjects (note: one study with a single healthy child as a comparison group was not utilized for this meta calculation). Yet, two of those studies collected comparison subjects from a sample exhibiting primary snoring (i.e., n = 45).
Study quality
Most studies were rated as “fair” quality, with only 2/13 being of poor quality (Table 2). The cutback in quality was chiefly due to the inconsistent reporting of the diagnostic information (Figure 2).
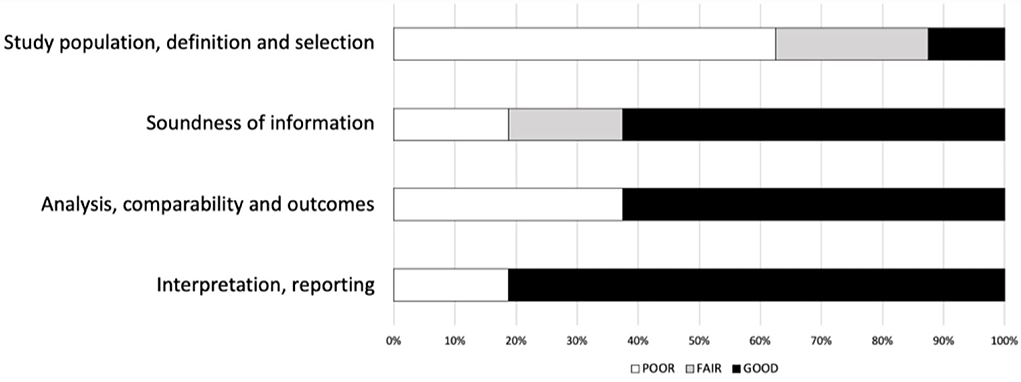
Figure 2. Risk of study quality bias (%). National Institutes of Health (NIH) quality assessment. The NIH quality assessment tool is applicable to different study designs and examines each study's internal validity by a set of items. For each study design, we subsequently regrouped items on four domains: “study population, definition, and selection,” “soundness of information,” “analysis, comparability, and outcomes,” and “interpretation and reporting.” A “poor” rating denotes that none of the items within the domain for the respective study design had an affirmative score; a “fair” rating is if less than half of the items were confirmed, and “good” is when more than half of the items were present. We report them as a proportion of the 13 studies examined to reflect the overall quality. Thus, for the 13 studies, a poor rating of more than 50% on the domain “study population, definition and selection” represents mediocre “reporting subject selection method, inclusion/exclusion criteria, sample characteristics etc”.
Meta-analysis part 1: Pooled mean of psg parameters in subjects with RTT and the comparison to literature normative values
Regarding the PSG data for subjects with RTT, sleep macrostructure parameters were collected from 11/13 studies and sleep-related breathing parameters from 9/13 studies. In addition to total group analysis, we were able to stratify the 134 RTT cases per gene (i.e., MECP2 vs. CDKL5), age, and clinical features (i.e., presence or absence of epilepsy or scoliosis). Of note, the age cut-off being <5 years old vs. >5 years old was copied because several studies sub-grouped RTT cases at the age of 5 years.
Sleep macrostructure
Pooled means of sleep macrostructure parameters and results of SMDTD are tabulated in Table 3. Forest plots are presented in Figures 1–8 in Supplementary material S2. RTT samples slept for about 7 h, resulting in a SEI of about 70%, whilst falling asleep took about 20 min, with wakefulness during the sleep period lasting to an hour or more. The distribution of sleep stages was as follows: stage N1 8%, stage N2 40%, stage N3 35%, and REM 15%.
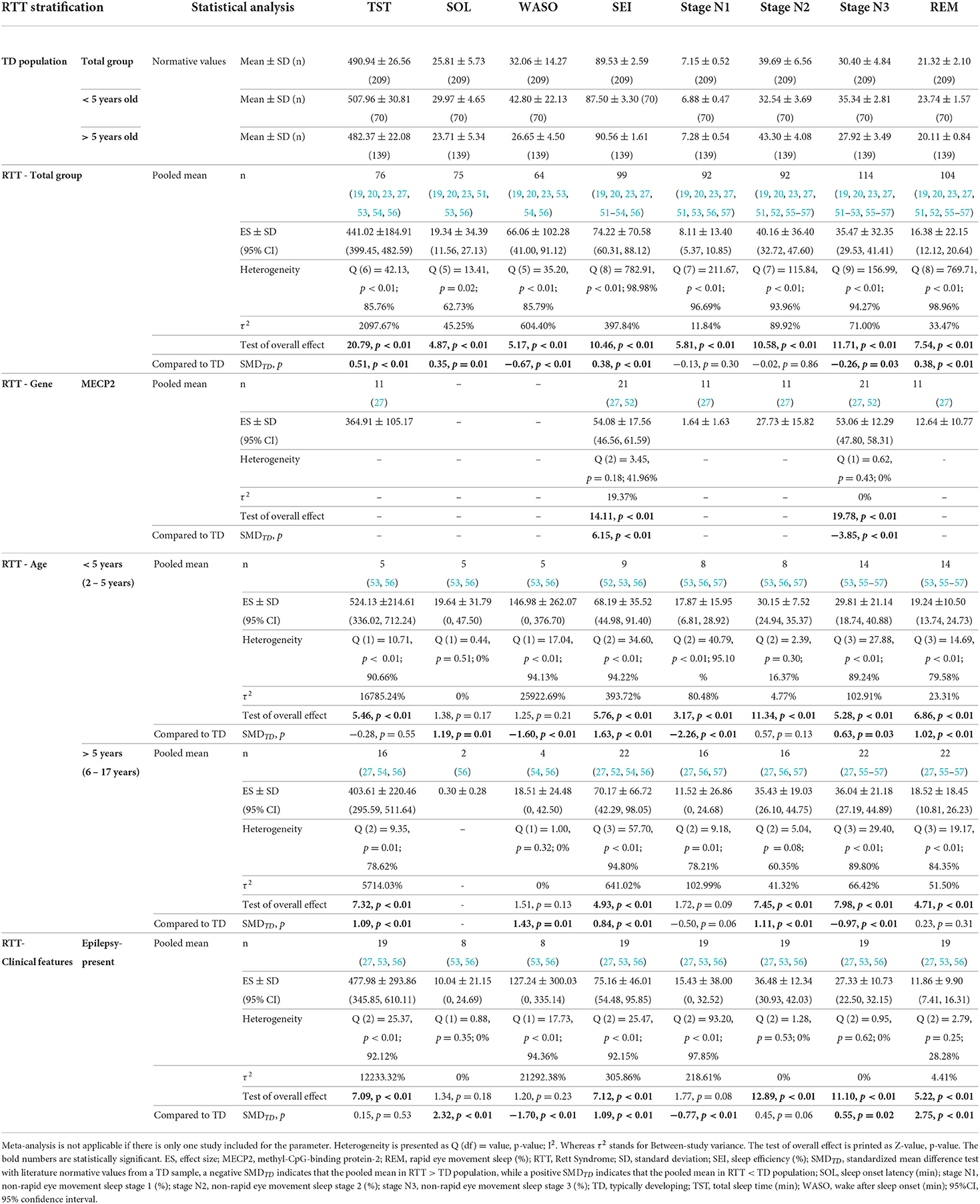
Table 3. Pooled mean of sleep macrostructure in RTT subjects and the comparison with literature normative values for typically developing population (49) (Part 1).
Total sample
In RTT total group SMDTD, we found significantly shorter TST, SOL, and particularly longer WASO. SEI was lower. Regarding the proportion of sleep stages, higher stage N3 and lower REM were seen in RTT.
Per gene strata
Yet based on fewer data, MECP2 mutant cases showed a particularly lower SEI but also higher stage N3. In terms of other sleep macrostructure parameters in the MECP2 stratum, the available data (k = 1, n = 11) was TST (364.91 ± 105.17 min.), stage N1 (1.64 ± 1.63 %), stage N2 (27.73 ± 15.82 %), and REM (12.64 ± 10.77 %). For the RTT cases in the CDKL5 stratum (k = 1, n = 4) a TST (666.20 ± 110.40 min.), SOL (38.80 ± 63.20 min.), WASO (239.00 ± 99.00 min.), SEI (70.60 ± 11.70 %), stage N1 (25.00 ± 4.30 %), stage N2 (43.30 ± 14.90 %), stage N3 (22.80 ± 13.10 %), and REM (8.90 ± 6.60 %) was reported.
Per age strata
The SMDTD results were discrepant between the two age strata except for SEI, i.e., in both age strata, SEI was lower. That is, in <5 years old stratum, longer WASO and shorter SOL were found, presenting further higher stage N1 but lower REM and stage N3, albeit the latter had a small SMDTD. Whilst in older ones, TST and particularly WASO were shorter but no data for SOL was available, and stage N1 and REM were not different from TD peers. However, a decreased stage N2 and an increased stage N3 were identified.
Per clinical features strata
We found the following only for the epilepsy-present stratum: the largest SMDTD for REM but also lower SOL, more WASO and stage N1. Limited data was available to generate a pooled mean analysis for the following strata: i.e., epilepsy-absent RTT case (k = 1, n = 1) being TST (448.00 min), SEI (92.00 %), stage N1 (1.00 %), stage N2 (34.00 %), stage N3 (37.00 %), and REM (27.00 %); scoliosis-present RTT cases (k = 1, n = 12) with TST (349.75 ± 113.20 min), SEI (63.08 ± 22.19 %), stage N1 (2.50 ± 3.37 %), stage N2 (32.17 ± 21.54 %), stage N3 (52.50 ± 25.14 %), and REM (12.17 ± 10.40 %). No sleep macrostructure data was available for scoliosis-absent RTT cases.
Sleep respiratory
The pooled ES and the SMDTD of the sleep respiratory parameters are all presented in Table 4 and Figures 9–13 in Supplementary material S2. AHI in RTT strata were all in the abnormal range.
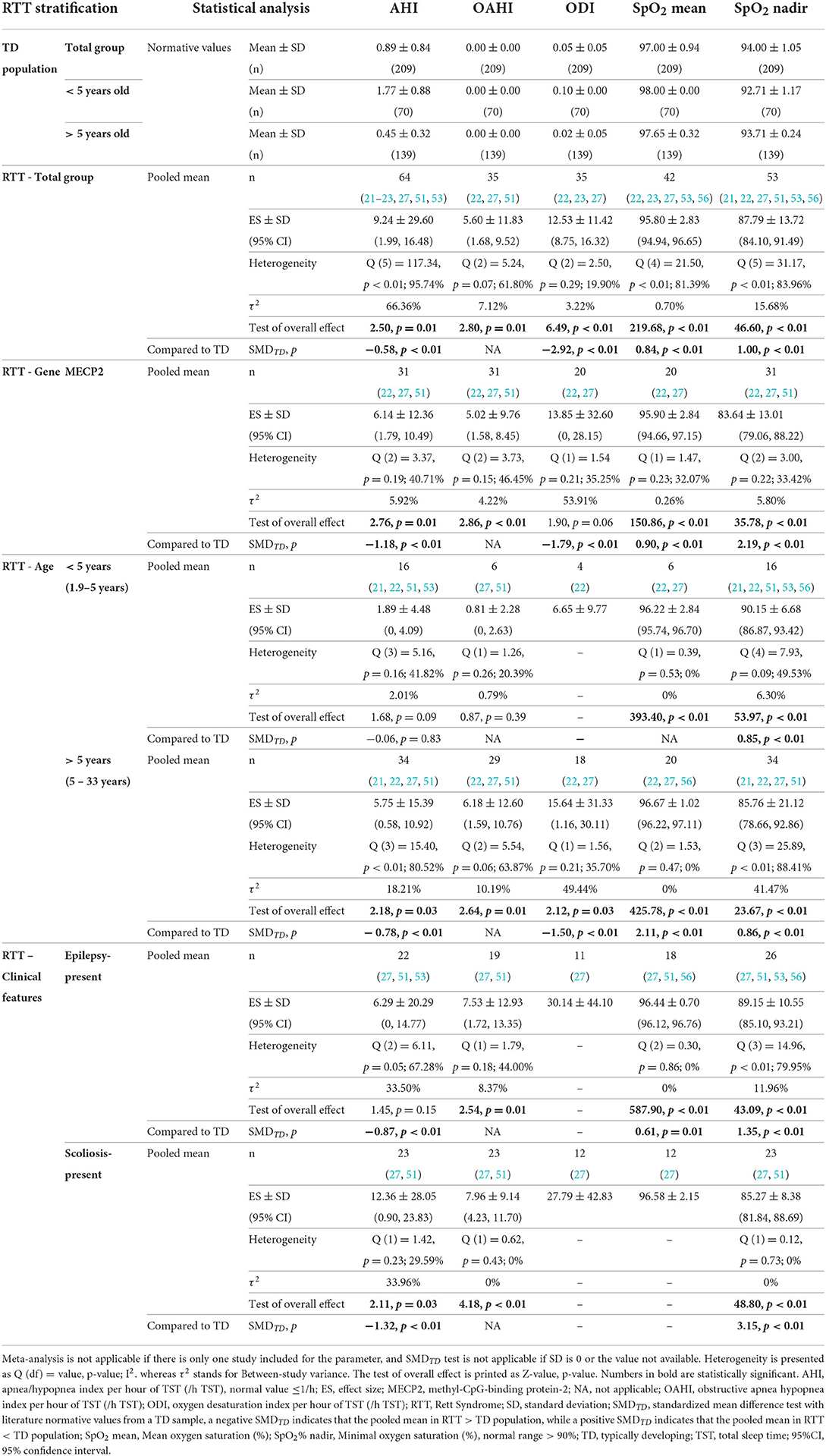
Table 4. Pooled mean of sleep respiratory indexes in RTT subjects and the comparison with literature normative values for typically developing population (50) (Part 1).
Total sample
In RTT total group, SMDTD showed significantly higher AHI, ODI, and lower SpO2%.
Per gene, age, and clinical features strata
Comparable findings were seen in >5 years old, MECP2, epilepsy-present, and scoliosis-present strata. AHI and ODI in these strata were significantly higher than the TD population, whilst SpO2% mean and SpO2% nadir were lower. Particularly, the highest AHI was seen in the scoliosis-present stratum.
We should note the limited data for a pooled mean in epilepsy-present stratum for ODI (k = 1, n = 11, 30.13 ± 44.10 /h TST) and in scoliosis-present stratum for SpO2 mean (%) (k = 1, n = 12, 96.58 ± 2.15 %). Such limitation was more obvious in the other RTT strata, i.e., CDKL5 mutant RTT cases (k = 1, n = 4) being AHI (1.48 ± 2.29 /h TST), SpO2% mean (96.30 ± 0.52 %), and SpO2% nadir (90.67 ± 3.21 %); epilepsy-absent RTT cases (k = 1, n = 5) being AHI (9.70 ± 7.61 /h TST), OAHI (8.46 ± 7.68 /h TST), and SpO2% nadir (87.20 ± 4.86 %) together with (k = 1, n = 1) ODI (2.00 /h TST) and SpO2% mean (98.00 %); and scoliosis-absent RTT cases (k = 1, n = 2) being AHI (4.20 ± 3.96 /h TST), OAHI (3.35 ± 3.46 /h TST), and SpO2% nadir (90.20 ± 1.27 %).
The difference test of OAHI and SpO2% mean in >5 years old stratum was not possible due to the SD of the literature normative value being “0,” but most RTT strata had OAHI >5/h TST.
Meta-analysis part 2: Sleep macrostructure of subjects with RTT when compared to comparison groups as published in the reviewed papers
We collected PSG data from four studies designed with comparison groups. Subjects with RTT recruited in these four studies were primarily classic phenotype under 15 years of age, and comparison groups were age-matched healthy (n = 76) and primary snoring subjects (n = 45) (e.g., k = 2 in each comparison) with limited stratification options. Forest plots are printed in Figure 3. Within the healthy comparison group, parameters of sleep macrostructure were extracted, and only stage N1 in RTT was found to be significantly lower. For the primary snoring group comparison, available data was limited to SEI and stage N3, both being non-significant.
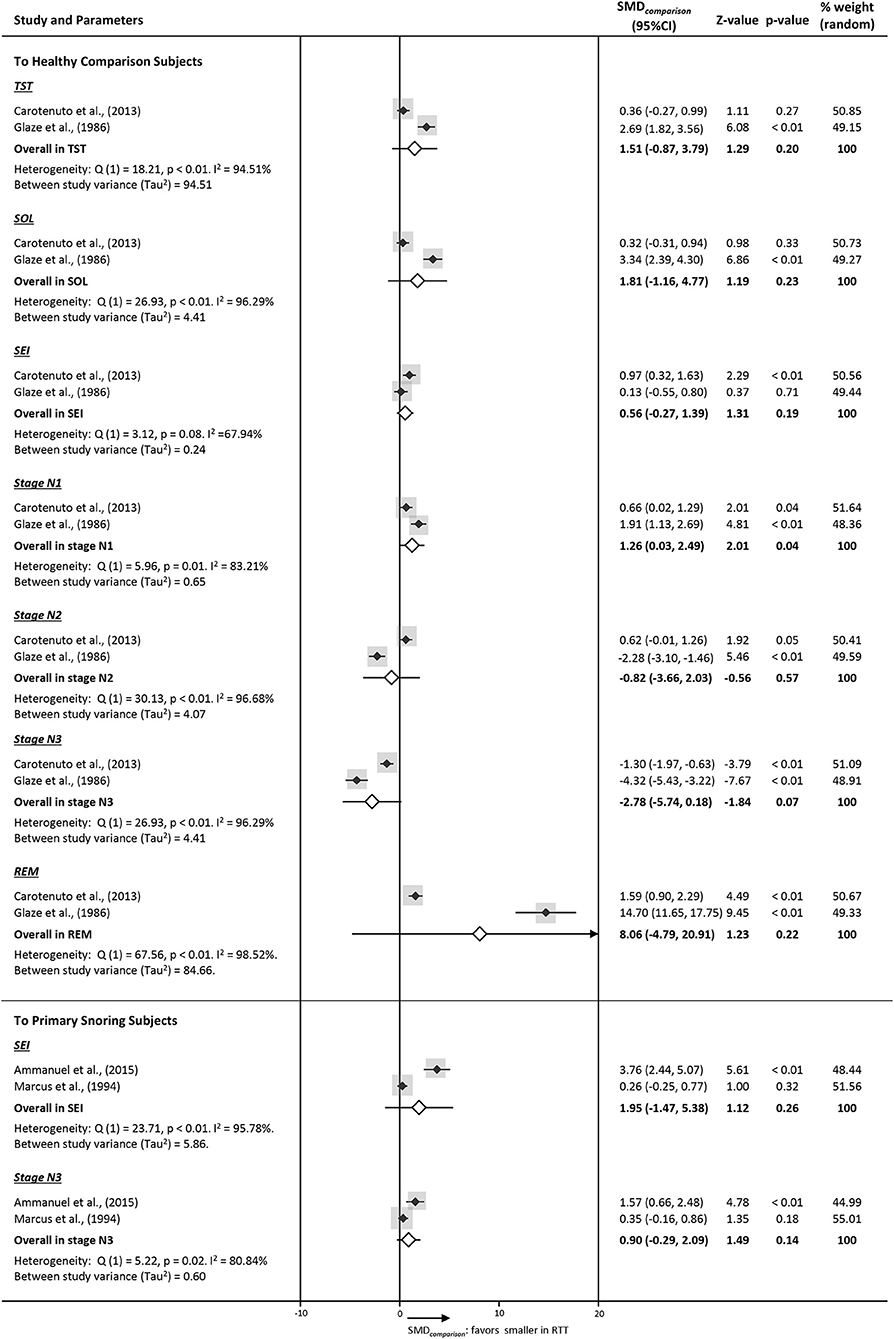
Figure 3. Forest plots of PSG parameters on sleep macrostructure of studies comparing RTT with a comparison group (SMDcomparison). Diamonds indicate standard mean difference (SMDcomparison) with a confidence interval of 95% (error bars 95% CI). The size of the gray square indicates the relative weight of the study on the combined ES.
Publication bias
Publication bias was tested by Begg's correlation rank test and significance was only found for SpO2 nadir (%) (Supplementary material S3). After excluding this study, the findings were unaltered (p = 0.55).
Discussion
We are one of the first systematic reviews providing average pooled sleep data of subjects with RTT, with further stratification per RTT-related genes, age, and the presence of certain clinical features. These data might be used as a reference regarding their sleep macrostructure and sleep respiratory events, with the note that eight studies included epilepsy-sensitive data. Compared to normative sleep study values, disrupted sleep in subjects with RTT can be chiefly characterized by increased WASO, prolonged stage N3 sleep, and attenuated REM sleep. Further, such SMDTD-based findings for RTT were somehow discrepant per stratifications of age and epilepsy. Principally for those younger than 5 years, more stage N1 and decreased stage N3, and for those older than 5 years shorter WASO, whereas for those with epilepsy especially reduced REM sleep was found. Contrariwise, yet given the limited number of studies included in the SMDcomparison analysis, only stage N1 was significantly lower than in healthy peers. Consequently, we may conclude poor sleep suggestive of variations according to certain RTT case features. Meta-findings further demonstrated severe nocturnal hypoxemia with apneic events.
Sleep pattern and sleep stages
The sleep structure is deemed to be impaired in RTT. Firstly, we found consistently reduced SEI in RTT strata, which is further supported by shorter TST and longer WASO in some of our strata results. Such findings suggest poor sleep efficacy and continuity in RTT. Frequent (15, 59, 60) and longlasting (61) night wakenings have been repeatedly reported in previous RTT surveys. As known, when nocturnal sleep is disrupted, the sleep homeostatic system will promote sleep and compensate for the sleep loss (62), resulting in prolonged TST during the sleep “recovery” nights (63, 64). Regarding the TST in our SMDTD results, we found that TST was contrariwise shortened in RTT total group and older stratum (i.e., 6–17 years), whilst not significantly different in the younger and epileptic RTT samples. For the TST reduction, integrating that previously we found that RTT cases with CDKL5 mutation were sleeping significantly longer than those cases with MECP2 mutation but with similar SEI and WASO (24), we may underline the genes-modulated pathological influence on their sleep regulation system. We believe that several other aspects should similarly be taken into consideration. Firstly, the sleep macrostructure is not identical across childhood. That is, the length of sleep time and its distribution within the 24-h period show an inverse relationship with age (65). A decreasing tendency in sleep duration in our reviewed sample, as shown in Table 3, might be assumed. Thus, our findings may suggest that the TST in RTT may decline more with age than TD. Secondly, sleep problems such as wakenings are common in young children (66) as well as in individuals with RTT (14), but in RTT cases they may persist. In fact, a modest peak of disorders of initiating and maintaining sleep in the 8–12 years old RTT age group was reported (14), potentially leading to shorter TST as found in our review. Thirdly, in terms of the presence of epilepsy, the drowsiness caused by epilepsy (67) or severe daytime somnolence due to antiepileptic drugs (AEDs) (68) may modify the sleep–wake cycle. We have to equally note here that the RTT samples in the younger and epilepsy-present strata were of a small sample size and from similar studies (53, 56). Lastly, the PSG recording time, and hence the potentially allowed time in bed and sleep duration, by convention, is being determined by a convenient wake-up timing on the next morning for sleep staff, which is acknowledged and likely discrepant from the usual sleep schedules of subjects with RTT. Alternatively, the “first night effect,” characterized as decreased TST, lower SEI, reduced REM, and longer REM latencies on the first PSG testing night (69, 70) might be considered as well to describe their poor sleep, and only one reviewed study (23) discussed this. Thus, a single night PSG recording during the nocturnal phase might be limitedly representative or may further aggrevate the alterations of sleep in RTT.
Although limited data on SOL was extracted, yet being another aspect of homeostatic recovery regulation, we found a consistent shortened SOL, which may reflect the increased sleep pressure, as reported before (24). Yet, it contradicts with our findings from sleep problem surveys where “difficulty falling asleep” was found in 60.3% of a MECP2 RTT group (14).
Regarding the sleep stage distribution, results for stage N1 and stage N3 sleep were peculiar. For stage N1, the SMDTD showed in the younger and epileptic strata a higher stage N1, but the SMDcomparison showed a decreased proportion (k = 2). Per the forest plot depicting stage N1, in three studies (53, 56, 57) stage N1 was relatively higher (see Figure 5 in Supplementary material S2), yet these studies did not report comparisons to a group and therefore were not included in the part 2 analysis. Further, when we screened the sample characteristics of these three studies, they chiefly represent samples within a 10-year age-range and with severe epilepsy or being treated with AEDs (53, 56) (i.e.: 5/8 of these epileptic RTT cases were samples for <5 years old stratum). Individuals with epilepsy usually have longer stage N1 sleep (71). Although epilepsy is prevalent in RTT, the incidence of epilepsy and the severity of seizure is thought to be milder in classic RTT phenotype than in certain atypical variants (72). In fact, only classic RTT cases were used in the comparison group studies, which could explain our discrepant stage N1 results.
Stage N2 showed a reduction in the older RTT stratum only. In healthy individuals, stage N2 has been reported to be significantly increased with age (65). We could not confirm this typical aging effect of stage N2 in RTT, neither here nor in our previous review (24) on literature case series data.
Though previous studies (23, 27, 51) and our SMDTD illustrated a relative increased stage N3, the stage N3 by SMDcomparison to healthy subjects was not significant. Yet after stratification, we confirmed higher stage N3 in the MECP2 RTT cases (i.e., excluding the CDKL5 from the total sample), as previously illustrated (24). The CDKL5 sample collected in this study was too small for meta-analysis, but we previously reported that they have less stage N3 sleep than MECP2 mutant RTT cases (24). Another confounder in stage N3 sleep findings besides genes might be age-related alterations. That is, here we found that stage N3 was significantly lower in younger RTT cases but higher in the older ones. Such stage N3 alteration is opposite to the age-related proportional decline of the N3 stage in the general population as reported by Ohayon et al. (65). This finding may demonstrate the perturbed slow oscillations occurring in RTT further challenging their sleep stage transition from deeper sleep stages (73, 74).
In terms of REM sleep, reduced REM was reported as a characteristic in several sleep studies of RTT (20, 23, 51) and our case series review (24). We also found decreased REM in subjects with RTT, but could not confirm this in the >5 year-old stratum. Such a dissimilarity, particularly, in earlier studies as increased (75) or decreased (20) REM in older RTT cases have been reported, could be linked to the brainstem dysfunctioning toward sleep cycle generation given a dispersed age at onset. However, based on our previous case series data, REM does not change with chronological age (24), and intergrating with the decline of REM sleep proportion in a healthy population (65), we may assume that the REM in RTT may stagnate already early in the life.
Sleep breathing
Although the breathing pattern in RTT seems more regular during nighttime than daytime, our study provided evidences supporting severe sleep-disordered breathing (SDB). During sleep, significantly more desaturations occur with steady hypoxemia. In fact, a wide spectrum of breathing irregularities during the wake-phase has been vividly described and discussed in RTT (76–78). Some studies (21, 53) likewise illustrated irregular sleep breathing patterns by central apnea, which may be due to immaturity of the respiratory control system (79). Based on plethysmography, dysregulation in the autonomic nervous system especially for younger RTT cases (80) was shown. Meanwhile, such sleep breathing irregularities may cause chronic hypoxemia during sleep as in our results, leading to reduced hypoxic sensitivity for chemoreceptor responding pathways in the brainstem.
In terms of the mechanism, both Mecp2 and Cdkl5 genetic mutations were proven to cause breathing abnormalities in RTT animal models, being more frequent during non-rapid eye movement sleep (NREM) in Cdkl5 mutant mice, which worsens with age in Mecp2 ones (81). The AHI is highly severe in those >5 years old, and with MECP2, epilepsy, and scoliosis in our meta-review. Regarding potentially involved molecules, such as GABA, brain-derived neurotrophic factor (BDNF) and monoaminergic modulators (76) have been suspected; however, the findings remain to be further clarified in human literature.
In view of the possible influence of SDB on the sleep macrostructre, our meta-analysis confirmed that RTT cases had similar SEI and stage N3 to primary snoring subjects (i.e., part 2 meta-analysis). But previous findings on sleep macrostructure differences between subjects with RTT and primary snoring subjects have been scant and inconsistent (19, 52) and consolidated in our meta-review. Thus, the impact of SDB on their sleep macrostructure is still a burning question.
Several limitations of our meta-review should be noted. Variable definitions of PSG parameters and the complexity of symptomatology and pathogenicity in RTT may have largely contributed to the heterogeneity among published studies. Although we did stratification per RTT-related genes, age, and the presence of certain clinical features, PSG data is indeed scant with regard to specific parameters (e.g., SOL), several strata (e.g., CDKL5, absence of epilepsy, and scoliosis), and more powerful study designs (e.g., with the control group). The age cut-off, although to a certain extent arbitrary, was copied from previous studies, allowing the analysis of a maximum amount of data per such stratum. Furthermore, we could not meta-analyze the data per cardinal RTT features due to the fact that studies did not report RTT samples following the guideline designed for cases with MECP2 mutations (4). Cases herein may however be more severe (e.g., breathing, epilepsy) as being referred for a sleep study, and therefore a selection bias may exist. While the TD comparsion sample was all under 18 years of age, only one RTT case in our sample was 33 years old. Although different sleep scoring methods and reporting formats may lead to biases, each sleep stage scoring methodology is standardized (18, 58, 82, 83), and therefore should not lead to too discordant findings. Also, PSG studies of RTT cases with FOXG1 mutation were not found. Thus, our review also underlined the scarcity of PSG investigation in RTT.
Conclusion
The findings are based on a limited number of PSG studies, but in general increased WASO with more stage N3 sleep and less REM sleep was found. Yet, age and epilepsy might be potential moderators of NREM sleep proportional distributions, whereas they might be a mediator for REM sleep generation. During the nighttime, a hypoxic state with apneic events was demonstrated. Our findings may help to elucidate multifactorial pathomechanisms in this complex disease and may stimulate basic research on mechanistic pathways in existing RTT animal models.
Data availability statement
The original contributions presented in the study are included in the article/Supplementary material, further inquiries can be directed to the corresponding author.
Author contributions
KS conceived and planned the presented review. X-YZ executed the review and wrote the first draft. Both authors verified and discussed the results and contributed to the final manuscript.
Acknowledgments
We would like to thank Dr. Daniel C. Tarquinio, Dr. Daniel G. Glaze, and Dr. Marco Carotenuto for providing reprints. The financial support (No. 201806180064) provided by China Scholarship Council (CSC) is acknowledged. KS is a member of the transdiagnostic workgroup of the ERN Ithaca.
Conflict of interest
The authors declare that the research was conducted in the absence of any commercial or financial relationships that could be construed as a potential conflict of interest.
Publisher's note
All claims expressed in this article are solely those of the authors and do not necessarily represent those of their affiliated organizations, or those of the publisher, the editors and the reviewers. Any product that may be evaluated in this article, or claim that may be made by its manufacturer, is not guaranteed or endorsed by the publisher.
Supplementary material
The Supplementary Material for this article can be found online at: https://www.frontiersin.org/articles/10.3389/fneur.2022.963626/full#supplementary-material
Supplementary material S1. Search items applied up to date 26 April 2022.
Supplementary material S2. Figures 1–13: Forest plots of sleep macrostructure and sleep respiratory parameters in RTT (Part 1).
Supplementary material S3. Begg's correlation rank test for publication bias.
References
1. Neul JL, Kaufmann WE, Glaze DG, Christodoulou J, Clarke AJ, Bahi-Buisson N, et al. Rett syndrome: revised diagnostic criteria and nomenclature. Ann Neurol. (2010) 68:944–50. doi: 10.1002/ana.22124
2. Hagberg B, Goutières F, Hanefeld F, Rett A, Wilson J. Rett syndrome: criteria for inclusion and exclusion. Brain Dev. (1985) 7:372–3. doi: 10.1016/S0387-7604(85)80048-6
3. Hagberg B, Witt-Engerström I. Rett syndrome: a suggested staging system for describing impairment profile with increasing age towards adolescence. Am J Med Genet Suppl. (1986) 1:47–59. doi: 10.1002/ajmg.1320250506
4. Kerr AM, Nomura Y, Armstrong D, Anvret M, Belichenko PV, Budden S, et al. Guidelines for reporting clinical features in cases with Mecp2 mutations. Brain Dev. (2001) 23:208–11. doi: 10.1016/S0387-7604(01)00193-0
5. Amir RE, Van den Veyver IB, Wan M, Tran CQ, Francke U, Zoghbi HY. Rett syndrome is caused by mutations in X-linked Mecp2, encoding methyl-Cpg-binding protein 2. Nat Gen. (1999) 23:185–8. doi: 10.1038/13810
6. Ariani F, Hayek G, Rondinella D, Artuso R, Mencarelli MA, Spanhol-Rosseto A, et al. Foxg1 is responsible for the congenital variant of rett syndrome. Am J Hum Genet. (2008) 83:89–93. doi: 10.1016/j.ajhg.2008.05.015
7. Tao J, Van Esch H, Hagedorn-Greiwe M, Hoffmann K, Moser B, Raynaud M, et al. Mutations in the X-Linked Cyclin-Dependent Kinase-Like 5 (Cdkl5/Stk9) Gene Are Associated with Severe Neurodevelopmental Retardation. Am J Hum Genet. (2004) 75:1149–54. doi: 10.1086/426460
8. Weaving LS, Christodoulou J, Williamson SL, Friend KL, McKenzie OL, Archer H, et al. Mutations of Cdkl5 cause a severe neurodevelopmental disorder with infantile spasms and mental retardation. Am J Hum Genet. (2004) 75:1079–93. doi: 10.1086/426462
9. Hagberg B, Hanefeld F, Percy A, Skjeldal O. An update on clinically applicable diagnostic criteria in rett syndrome. Comments to rett syndrome clinical criteria consensus panel satellite to european paediatric neurology society meeting, Baden Baden, Germany, 11 September 2001. Eur J Paediatr Neurol. (2002) 6:293–7. doi: 10.1053/ejpn.2002.0612
10. Group. TRSDCW. Diagnostic criteria for rett syndrome. Ann Neurol. (1988) 23:425–8. doi: 10.1002/ana.410230432
11. Coleman M, Brubaker J, Hunter K, Smith G. Rett syndrome: a survey of North American patients. J Mental Defic Res. (1988) 32 (Pt 2):117–24. doi: 10.1111/j.1365-2788.1988.tb01397.x
12. Robb SA, Harden A, Boyd SG. Rett syndrome: an eeg study in 52 girls. Neuropediatrics. (1989) 20:192–5. doi: 10.1055/s-2008-1071290
14. Spruyt K. Sleep problems in individuals with rett syndrome: a systematic review and meta-analysis. Sleep Epidemiology. (2022) 2:100027. doi: 10.1016/j.sleepe.2022.100027
15. Boban S, Wong K, Epstein A, Anderson B, Murphy N, Downs J, et al. Determinants of sleep disturbances in rett syndrome: novel findings in relation to genotype. Am J Med Genet. A. (2016) 170:2292–300. doi: 10.1002/ajmg.a.37784
16. Wong K, Leonard H, Jacoby P, Ellaway C, Downs J. The trajectories of sleep disturbances in rett syndrome. J Sleep Res. (2015) 24:223–33. doi: 10.1111/jsr.12240
17. Jafari B, Mohsenin V. Polysomnography. Clin Chest Med. (2010) 31:287–97. doi: 10.1016/j.ccm.2010.02.005
18. Iber C, Ancoli-Israel S, Chesson AL, Quan SF. The Aasm Manual for the Scoring of Sleep and Associated Events: Rules, Terminology and Technical Specifications. Darien, IL: American Academy of Sleep Medicine Westchester, IL (2007).
19. Marcus CL, Carroll JL, McColley SA, Loughlin GM, Curtis S, Pyzik P, et al. Polysomnographic characteristics of patients with rett syndrome. J Pediatr. (1994) 125:218–24. doi: 10.1016/S0022-3476(94)70196-2
20. Glaze DG, Frost JD Jr, Zoghbi HY, Percy AK. Rett's syndrome: characterization of respiratory patterns and sleep. Ann Neurol. (1986) 21:377–82. doi: 10.1002/ana.410210410
21. Bassett E, Heinle R, Johnston D. Sleep apnea in patients with rett syndrome: roles for polysomnography and adenotonsillectomy. J Child Neurology. (2016) 31:1633–4. doi: 10.1177/0883073816671439
22. Hagebeuk EE, Bijlmer RP, Koelman JH, Poll-The Poll-The BT. Respiratory disturbances in rett syndrome: don't forget to evaluate upper airway obstruction. J Child Neurol. (2012) 27:888–92. doi: 10.1177/0883073811429859
23. Carotenuto M, Esposito M, D'Aniello A, Rippa CD, Precenzano F, Pascotto A, et al. Polysomnographic findings in Rett syndrome: a case-control study. Sleep Breathing. (2013) 17:93–8. doi: 10.1007/s11325-012-0654-x
24. Zhang X, Spruyt K. Literature cases summarized based on their polysomnographic findings in rett syndrome. Int J Environ Res Public Health. (2022) 19:3422. doi: 10.3390/ijerph19063422
25. Tarquinio DC, Hou W, Neul JL, Berkmen GK, Drummond J, Aronoff E, et al. The course of awake breathing disturbances across the lifespan in rett syndrome. Brain Dev. (2018) 40:515–29. doi: 10.1016/j.braindev.2018.03.010
26. MacKay J, Leonard H, Wong K, Wilson A, Downs J. Respiratory morbidity in rett syndrome: an observational study. Dev Med Child Neurol. (2018) 60:951–7. doi: 10.1111/dmcn.13726
27. Amaddeo A, De Sanctis L, Arroyo JO, Khirani S, Bahi-Buisson N, Fauroux B. Polysomnographic findings in rett syndrome. Eur J Paediatr Neurol. (2019) 23:214–21. doi: 10.1016/j.ejpn.2018.09.003
28. Moher D, Liberati A, Tetzlaff J, Altman DG. Preferred reporting items for systematic reviews and meta-analyses: the prisma statement. PLoS Med. (2009) 6:e1000097. doi: 10.1371/journal.pmed.1000097
29. NHLaBI. Development and Use of Quality Assessment Tools. Available online at: https://www.nhlbi.nih.gov/health-topics/study-quality-assessment-tools (accessed November 04, 2019).
30. Braam W, Spruyt K. Reference intervals for 6-sulfatoxymelatonin in urine: a meta-analysis. Sleep Med Rev. (2022) 63:101614. doi: 10.1016/j.smrv.2022.101614
31. Health NIo. Guidance for Assessing the Quality of Observational Cohort and Cross-Sectional Studies: National Institutes of Health. Available online at: https://www.nhlbi.nih.gov/health-topics/study-quality-assessment-tools (accessed March 06, 2020).
32. Chen Y, Yu J, Niu Y, Qin D, Liu H, Li G, et al. Modeling rett syndrome using talen-edited Mecp2 mutant cynomolgus monkeys. Cell. (2017) 169:945–55.e10. doi: 10.1016/j.cell.2017.04.035
33. Hess-Homeier DL, Fan CY, Gupta T, Chiang AS, Certel SJ. Astrocyte-specific regulation of Hmecp2 expression in Drosophila. Biol Open. (2014) 3:1011–9. doi: 10.1242/bio.20149092
34. Gupta T, Morgan HR, Bailey JA, Certel SJ. Functional conservation of Mbd proteins: Mecp2 and Drosophila Mbd proteins alter sleep. Genes Brain Behav. (2016) 15:757–74. doi: 10.1111/gbb.12314
35. Lo Martire V, Alvente S, Bastianini S, Berteotti C, Silvani A, Valli A, et al. Cdkl5 deficiency entails sleep apneas in mice. J Sleep Res. (2017) 26:495–7. doi: 10.1111/jsr.12512
36. Li Q, Loh DH, Kudo T, Truong D, Derakhshesh M, Kaswan ZM, et al. Circadian rhythm disruption in a mouse model of rett syndrome circadian disruption in rtt. Neurobiol Dis. (2015) 77:155–64. doi: 10.1016/j.nbd.2015.03.009
37. Tsuchiya Y, Minami Y, Umemura Y, Watanabe H, Ono D, Nakamura W, et al. Disruption of Mecp2 attenuates circadian rhythm in Crispr/Cas9-based rett syndrome model mouse. Genes Cells. (2015) 20:992–1005. doi: 10.1111/gtc.12305
38. Moretti P, Bouwknecht JA, Teague R, Paylor R, Zoghbi HY. Abnormalities of Social interactions and home-cage behavior in a mouse model of rett syndrome. Hum Mol Genet. (2005) 14:205–20. doi: 10.1093/hmg/ddi016
39. Ren J, Ding X, Funk GD, Greer JJ. Anxiety-related mechanisms of respiratory dysfunction in a mouse model of rett syndrome. J Neurosci. (2012) 32:17230–40. doi: 10.1523/JNEUROSCI.2951-12.2012
40. D'Cruz JA, Wu C, Zahid T, El-Hayek Y, Zhang L, Eubanks JH. Alterations of cortical and hippocampal Eeg activity in Mecp2-deficient mice. Neurobiol Dis. (2010) 38:8–16. doi: 10.1016/j.nbd.2009.12.018
41. Johnston MV, Ammanuel S, O'Driscoll C, Wozniak A, Naidu S, Kadam SD. Twenty-four hour quantitative-eeg and in-vivo glutamate biosensor detects activity and circadian rhythm dependent biomarkers of pathogenesis in Mecp2 null mice. Front Syst Neurosci. (2014) 8:118. doi: 10.3389/fnsys.2014.00118
42. Wither RG, Colic S, Wu C, Bardakjian BL, Zhang L, Eubanks JH. Daily rhythmic behaviors and thermoregulatory patterns are disrupted in adult female Mecp2-deficient mice. PLoS ONE. (2012) 7:e35396. doi: 10.1371/journal.pone.0035396
43. Fuchs C, Gennaccaro L, Trazzi S, Bastianini S, Bettini S, Lo Martire V, et al. Heterozygous Cdkl5 Knockout female mice are a valuable animal model for Cdkl5 disorder. Neural Plasticity. (2018) 2018:9726950. doi: 10.1155/2018/9726950
44. Robinson L, Plano A, Cobb S, Riedel G. Long-term home cage activity scans reveal lowered exploratory behaviour in symptomatic female rett mice. Behav Brain Res. (2013) 250:148–56. doi: 10.1016/j.bbr.2013.04.041
45. Wang J, Wang Z, Zhang H, Feng S, Lu Y, Wang S, et al. White matter structural and network topological changes underlying the behavioral phenotype of Mecp2 mutant monkeys. Cerebral Cortex. (2021) 31:5396–410. doi: 10.1093/cercor/bhab166
46. Dong HW, Erickson K, Lee JR, Merritt J, Fu C, Neul JL. Detection of neurophysiological features in female R255x Mecp2 mutation mice. Neurobiol Dis. (2020) 145:105083. doi: 10.1016/j.nbd.2020.105083
47. Bhat S, Ming X, Dekermenjian R, Chokroverty S. Continuous spike and wave in slow-wave sleep in a patient with rett syndrome and in a patient with lhermitte-duclos syndrome and neurofibromatosis 1. J Child Neurol. (2014) 29:Np176–80. doi: 10.1177/0883073813509888
48. TIBCO Software Inc,. Statistica (Data Analysis Software System), Version 13. (2018). Available online at: http://tibco.com
49. Scholle S, Beyer U, Bernhard M, Eichholz S, Erler T, Graness P, et al. Normative values of polysomnographic parameters in childhood and adolescence: quantitative sleep parameters. Sleep Med. (2011) 12:542–9. doi: 10.1016/j.sleep.2010.11.011
50. Scholle S, Wiater A, Scholle HC. Normative values of polysomnographic parameters in childhood and adolescence: cardiorespiratory parameters. Sleep Med. (2011) 12:988–96. doi: 10.1016/j.sleep.2011.05.006
51. Sarber KM, Howard JJM, Dye TJ, Pascoe JE, Simakajornboon N. Sleep-disordered breathing in pediatric patients with rett syndrome. J Clin Sleep Med. (2019) 15:1451–7. doi: 10.5664/jcsm.7974
52. Ammanuel S, Chan WC, Adler DA, Lakshamanan BM, Gupta SS, Ewen JB, et al. Heightened delta power during slow-wave-sleep in patients with rett syndrome associated with poor sleep efficiency. PLoS ONE. (2015) 10:e0138113. doi: 10.1371/journal.pone.0138113
53. Hagebeuk EE, van den Bossche RA, de Weerd AW. Respiratory and sleep disorders in female children with atypical rett syndrome caused by mutations in the Cdkl5 gene. Dev Med Child Neurol. (2012) 55:480–4. doi: 10.1111/j.1469-8749.2012.04432.x
54. Schlüter B, Aguigah G, Buschatz D, Trowitzsch E, Aksu F. Polysomnographic recordings of respiratory disturbances in Rett syndrome. J Sleep Res. (1995) 4:203–7. doi: 10.1111/j.1365-2869.1995.tb00216.x
55. Segawa M, Nomura Y. The pathophysiology of the Rett syndrome from the standpoint of polysomnography. Brain Dev. (1990) 12:55–60. doi: 10.1016/S0387-7604(12)80178-1
56. Aldrich MS, Garofalo EA, Drury I. Epileptiform abnormalities during sleep in rett syndrome. Electroencephalogr Clin Neurophysiol. (1990) 75:365–70. doi: 10.1016/0013-4694(90)90081-T
57. Nomura Y, Segawa M, Higurashi M. Rett syndrome–an early catecholamine and indolamine deficient disorder? Brain Dev. (1985) 7:334–41. doi: 10.1016/S0387-7604(85)80040-1
58. Rechtschaffen A, Kales A. A Manual for Standardized Terminology, Techniques and Scoring System for Sleep Stages in Human Subjects. Washington, DC: Public Health Service, US Government Printing Office (1968).
59. Boban S, Leonard H, Wong K, Wilson A, Downs J. Sleep disturbances in rett syndrome: impact and management including use of sleep hygiene practices. Am J Med Genet A. (2018) 176:1569–77. doi: 10.1002/ajmg.a.38829
60. Vignoli A, La Briola F, Peron A, Turner K, Savini M, Cogliati F, et al. Medical care of adolescents and women with rett syndrome: an Italian study. Am J Med Genet Part A. (2012) 158a:13–8. doi: 10.1002/ajmg.a.34367
61. McDougall A, Kerr AM, Espie CA. Sleep disturbance in children with rett syndrome: a qualitative investigation of the parental experience. J Appl Res Intell Disabil. (2005) 18:201–15. doi: 10.1111/j.1468-3148.2005.00220.x
62. Benington JH. Sleep homeostasis and the function of sleep. Sleep. (2000) 23:959–66. doi: 10.1093/sleep/23.7.1j
63. Franken P. Sleep homeostasis. In: Binder MD, Hirokawa N, Windhorst U, editors. Encyclopedia of Neuroscience. Berlin, Heidelberg: Springer Berlin Heidelberg (2009). p. 3721–5. doi: 10.1007/978-3-540-29678-2_5441
64. Institute of Medicine. Sleep Disorders and Sleep Deprivation: An Unmet Public Health Problem. Washington, DC: The National Academies Press (2006). doi: 10.17226/11617
65. Ohayon MM, Carskadon MA, Guilleminault C, Vitiello MV. Meta-analysis of quantitative sleep parameters from childhood to old age in healthy individuals: developing normative sleep values across the human lifespan. Sleep. (2004) 27:1255–73. doi: 10.1093/sleep/27.7.1255
66. Carter KA, Hathaway NE, Lettieri CF. Common sleep disorders in children. Am Fam Physician. (2014) 89:368–77.
67. Manni R, Tartara A. Evaluation of sleepiness in epilepsy. Clin Neurophysiol. (2000) 111(Suppl. 2):S111–4. doi: 10.1016/S1388-2457(00)00410-7
68. Placidi F, Scalise A, Marciani MG, Romigi A, Diomedi M, Gigli GL. Effect of antiepileptic drugs on sleep. Clin Neurophysiol. (2000) 111(Suppl. 2):S115–9. doi: 10.1016/S1388-2457(00)00411-9
69. Agnew HW Jr, Webb WB, Williams RL. The first night effect: an eeg study of sleep. Psychophysiology. (1966) 2:263–6. doi: 10.1111/j.1469-8986.1966.tb02650.x
70. Ding L, Chen B, Dai Y, Li Y. A Meta-analysis of the first-night effect in healthy individuals for the full age spectrum. Sleep Med. (2022) 89:159–65. doi: 10.1016/j.sleep.2021.12.007
72. Tarquinio DC, Hou W, Berg A, Kaufmann WE, Lane JB, Skinner SA, et al. Longitudinal course of epilepsy in Rett syndrome and related disorders. Brain: J. Neurol. (2017) 140:306–18. doi: 10.1093/brain/aww302
73. Ghilardi M, Ghez C, Dhawan V, Moeller J, Mentis M, Nakamura T, et al. Patterns of regional brain activation associated with different forms of motor learning. Brain Res. (2000) 871:127–45. doi: 10.1016/S0006-8993(00)02365-9
74. Banerjee A, Miller MT, Li K, Sur M, Kaufmann WE. Towards a better diagnosis and treatment of Rett syndrome: a model synaptic disorder. Brain. (2019) 142:239–48. doi: 10.1093/brain/awy323
75. Nomura Y, Segawa M, Hasegawa M. Rett syndrome–clinical studies and pathophysiological consideration. Brain Dev. (1984) 6:475–86. doi: 10.1016/S0387-7604(84)80030-3
76. Katz DM, Dutschmann M, Ramirez JM, Hilaire G. Breathing disorders in rett syndrome: progressive neurochemical dysfunction in the respiratory network after birth. Respir Physiol Neurobiol. (2009) 168:101–8. doi: 10.1016/j.resp.2009.04.017
77. Kerr AM. A review of the respiratory disorder in the rett syndrome. Brain Dev. (1992) 14(Suppl.):S43–5.
78. Julu PO, Kerr AM, Apartopoulos F, Al-Rawas S, Engerstrom IW, Engerstrom L, et al. Characterisation of breathing and associated central autonomic dysfunction in the rett disorder. Arch Dis Childh. (2001) 85:29–37. doi: 10.1136/adc.85.1.29
79. Julu PO, Witt Engerström I. Assessment of the maturity-related brainstem functions reveals the heterogeneous phenotypes and facilitates clinical management of rett syndrome. Brain Dev. (2005) 27(Suppl. 1):S43–53. doi: 10.1016/j.braindev.2005.02.012
80. Weese-Mayer DE, Lieske SP, Boothby CM, Kenny AS, Bennett HL, Ramirez JM. Autonomic dysregulation in young girls with rett syndrome during nighttime in-home recordings. Pediatr Pulmonol. (2008) 43:1045–60. doi: 10.1002/ppul.20866
81. Zhang X, Lin JS, Spruyt K. Sleep problems in Rett syndrome animal models: a systematic review. J Neurosci Res. (2020) 99:529–44. doi: 10.1002/jnr.24730
82. Wolpert EA. A manual of standardized terminology, techniques and scoring system for sleep stages of human subjects. Arch Gen Psychiatry. (1969) 20:246–7. doi: 10.1001/archpsyc.1969.01740140118016
Keywords: Rett Syndrome, sleep, polysomnography, electroencephalogram (EEG), sleep disordered breathing (SDB)
Citation: Zhang X-Y and Spruyt K (2022) A meta-review of standard polysomnography parameters in Rett Syndrome. Front. Neurol. 13:963626. doi: 10.3389/fneur.2022.963626
Received: 07 June 2022; Accepted: 01 August 2022;
Published: 20 September 2022.
Edited by:
Aglaia Vignoli, University of Milan, ItalyCopyright © 2022 Zhang and Spruyt. This is an open-access article distributed under the terms of the Creative Commons Attribution License (CC BY). The use, distribution or reproduction in other forums is permitted, provided the original author(s) and the copyright owner(s) are credited and that the original publication in this journal is cited, in accordance with accepted academic practice. No use, distribution or reproduction is permitted which does not comply with these terms.
*Correspondence: Karen Spruyt, a2FyZW4uc3BydXl0QGluc2VybS5mcg==; a2FyZW4uc3BydXl0QHUtcGFyaXMuZnI=