- 1Department of Neurology, Nanjing Brain Hospital, Nanjing Medical University, Nanjing, China
- 2MEG Center, Nanjing Brain Hospital, Nanjing, China
Objective: This study aimed to investigate the relationship between cognitive function sleep spiking activation and brain activity in self-limited epilepsy with centrotemporal spikes (SeLECTS).
Methods: We used spike-wave index (SWI), which means the percentage of the spike and slow wave duration to the total non-REM (NREM) sleep time, as the grouping standard. A total of 14 children with SeLECTS (SWI ≥ 50%), 21 children with SeLECTS (SWI < 50%), and 20 healthy control children were recruited for this study. Cognitive function was evaluated using the Wechsler Intelligence Scale for Children, Fourth Edition (Chinese version) (WISC-IV). Magnetic source activity was assessed using magnetoencephalography calculated for each frequency band using the accumulated source imaging (ASI) technique.
Results: Children with SeLECTS (SWI ≥ 50%) had the lowest cognitive function scores, followed by those with SeLECTS (SWI < 50%) and then healthy controls. There were significant differences in the localization of magnetic source activity between the three groups: in the alpha (8–12 Hz) frequency band, children with SeLECTS (SWI ≥ 50%) showed deactivation of the medial frontal cortex (MFC) region; in the beta (12–30 Hz) frequency band, children with SeLECTS (SWI ≥ 50%) showed deactivation of the posterior cingulate cortex (PCC) segment; and in the gamma (30–80 Hz) frequency band, children in the healthy group showed activation of the PCC region.
Conclusion: This study revealed significant decreases in cognitive function in children with SeLECTS (SWI ≥ 50%) compared to children with SeLECTS (SWI < 50%) and healthy children, as well as significant differences in magnetic source activity between the three groups. The findings suggest that deactivation of magnetic source activity in the PCC and MFC regions is the main cause of cognitive function decline in SeLECTS patients with some frequency dependence.
Introduction
Self-limited epilepsy with centrotemporal spikes (SeLECTS), which is also known as Rolandic epilepsy (RE) and Benign childhood epilepsy with centrotemporal spikes (BECTS), is the most common type of childhood idiopathic epilepsy (1). The International League Against Epilepsy (ILAE) has changed the term “benign” to “self-limited” in the latest version of the definition in 2022, taking into account that the typical evolution of this type of epilepsy is age-related onset and remission (2, 3). In China, the mean age of onset of SeLECTS is 6.85 years and the male to female ratio is 6:4 (4). Typical clinical presentation is limited motor-sensory seizures on one side of the mouth and face, with extension to generalized tonic-clonic seizures (5). In these patients, electroencephalogram (EEG) commonly shows normal background activity and spike discharges in the centrotemporal region with high amplitude that are usually more pronounced during sleep (6). While SeLECTS was previously thought to have a favorable prognosis, a growing number of studies suggest that, even in adulthood when patients are seizure-free, SeLECTS is associated with greater neuropsychiatric dysfunction (7–9).
Children with SeLECTS show significantly more discharges during sleep, some of which reach electrical status epilepticus (ESES) (10). This group of patients is characterized by a poor prognosis and a negative impact on the development of cognitive function (11). It is widely believed that frequent epileptiform discharges affect the formation and establishment of synapses, damage neural circuits, and produce changes in the structure and function of brain tissue that affect the entire functional network of the brain and lead to cognitive decline (12–14). In a clinical study, Overvliet et al. (15) found an association between cognitive impairment and nocturnal epileptiform discharges. This association was interpreted as a disruption of the functional connections responsible for the corresponding cognitive ability. In a functional magnetic resonance imaging (fMRI) study of nine children with SeLECTS with ESES, we observed alterations in the salience network (SE) and central executive network (CEN) (10).
ESES is an interictal EEG pattern that occurs during slow wave sleep (16). ESES patterns usually consist of continuous, symmetrical spike patterns with variable discharge frequencies, usually in the range of 1.5–3 HZ. The spike-wave index (SWI), which is the percentage of the spike and slow wave duration to the total non-REM (NREM) sleep time, is commonly used to measure the severity of ESES (17). In general, the higher the SWI, the more epileptiform discharges and the more severe the cognitive impairment (18). It is now generally accepted that a SWI of ≥85% can be considered ESES, but some studies have also shown that cognitive and behavioral impairment can occur even with a low percentage of sleep. So some authors also use lower SWI values as a cut-off (19, 20), or consider that a SWI of at least ≥50 should trigger the possibility of an ESES related syndrome (21). In the current study, we decided to divide patients in two groups according to a SWI threshold at 50%, and all EEG interpretations were performed by two experienced EEG physicians.
Magnetoencephalography (MEG) is a non-invasive neuroimaging method with ultra-high spatial and temporal resolution (22). Compared to EEG, the magnetic field is not attenuated as it passes through the skull and scalp, allowing for clearer detection of brain magnetic activity (23). MEG can capture higher frequency information compared to fMRI, which can only retain low frequency information (24, 25). Given the frequency-dependent nature of brain activity (26), MEG can be used to more accurately localize neuromagnetic brain activity in each frequency band.
Previous studies have investigated differences in cognitive function in children with SeLECTS in longitudinal comparisons, as well as differences before and after medication administration (27–29). However, few studies have compared the cognitive function of children with SeLECTS without antiepileptic drugs (AEDs) in a cross-sectional manner with different evolutionary patterns, especially using the MEG technique (30, 31). We use EEG as a basis for grouping because children with developmental epileptic encephalopathy with spike-and-wave activation in sleep (DEE-SWAS) and epileptic encephalopathy with spike-and-wave activation in sleep (EE-SWAS), as defined by the latest ILAE definitions, whose diagnostic criteria include significant behavioral regression and negative myoclonus in addition to EEG manifestations, are difficult to diagnose at first presentation. Most of the patients diagnosed were already taking AEDs, which interfered with the results of this experiment. Therefore, in this trial we used only the EEG, an objective indicator, as a basis for grouping. In the present study, we compared the intensity and localization of magnetic sources in different frequency bands in children with SeLECTS (SWI ≥ 50%), children with SeLECTS (SWI < 50%), and healthy control (HC) children. Cognitive function in the three groups was assessed using the Wechsler Intelligence Scale for Children, Fourth Edition (Chinese version) (WISC-IV). We tentatively hypothesized that SeLECTS children (SWI ≥ 50%) would have the worst cognitive function and that there would be differences in magnetic source activity intensity and magnetic source localization between the three groups.
Experimental procedures
Participants
Between October 2020 and October 2021, 21 children were diagnosed with SeLECTS (SWI ≥ 50%) and 24 children were diagnosed with SeLECTS (SWI < 50%) only at the Neurology Department of the Nanjing Children's Hospital and Nanjing Brain Hospital. Of the SeLECTS (SWI < 50%) patients, two were taking AEDs and one had a history of encephalitis; thus, 21 children were included in this study. Of the SeLECTS patients (SWI ≥ 50%), six had a history of AEDs and one had an autism spectrum disorder; thus, only 14 patients were recruited for this study. We also recruited 20 HC children matched with the patients in terms of age, gender, parents educational background, and family socioeconomic status. The inclusion criteria for patients were as follows: (1) a diagnosis of SeLECTS according to the ILAE 2022 classification of epilepsy syndrome (32); (2) no use of AEDs at the time of enrollment; (3) normal brain MRI image; and (4) no other types of epilepsy or other major neuropsychiatric disorders. The exclusion criteria were: (1) did not cooperate when MEG and MRI data were collected which interfered with data collection; (2) brain MRI showed abnormalities; (3) a diagnosis of other types of epilepsy or major neuropsychiatric disorders; and (4) the patients parents did not cooperate with the examination or provide informed consent. All patients were seizure-free for at least 72 h before and 24 h after the MEG and MRI scans were performed.
Based on previous studies (19–21), we hypothesize that there is a significant difference between the cognitive function, brain activity of children with SeLECTS using SWI = 50% as the cut-off. In the SeLECTS (SWI ≥ 50%) group, two EEG results for patients within 1 month showing SWI ≥ 50%; the SWI (%) was obtained as the total number of minutes of all spike and slow-wave abnormalities divided by the total number of minutes of NREM and multiplied by 100 (33). All patients were subjected to sleep deprivation to better record the EEG during sleep. The recording time was 2 h and the SWI was calculated on 45 min of NREM sleep. EEG reports for all patients issued by two experienced EEG physicians.
This study was approved by the Medical Ethics Committee of Nanjing Medical University, Nanjing Brain Hospital and Nanjing Children's Hospital. In addition, the parents of all participants in the study provided informed consent.
Neuropsychological assessment
For all participants, cognitive function was assessed using the Wechsler Children Intelligence Scale, Fourth Edition (Chinese version) (WISC-IV). The test comprises 14 sub-tests that provide a full-scale intelligence quotient (FSIQ) to account for overall cognitive abilities as well as four additional composite scores that account for cognitive abilities in different areas (34). The four composite scores are the Verbal Comprehension Index (VCI), Perceptual Reasoning Index (PRI), Working Memory Index (WMI), and Processing Speed Index (PSI). The VCI, which includes tests of analogy, vocabulary, comprehension, and general knowledge, measures language learning ability, concept formation, abstract thinking, and analytical generalization. The PRI includes block design, picture concepts, matrix reasoning, and fill-in-the-blank tests, which measure reasoning ability, spatial perception, and visual organization, respectively. The WMI includes a recited number test, alphabetic-numeric alignment, and arithmetic test to measure memory ability, comprehension, and application of external information. The PSI includes decoding tests, symbol retrieval, and scratch tests to measure the speed of understanding simple information and the speed and accuracy of recording (35, 36).
MEG recordings
MEG data were collected in the magnetic shielding room of the MEG Center of Nanjing Brain Hospital using a full-head CTF-275 channel MEG system (VSM Medical Technology Company, Canada). In preparation for data collection, subjects were asked to make sure they were free of any metal objects and to fix three coils at the base of their nose and in front of each ear to determine the position of their head in the MEG system. During data collection, subjects were asked to remain relaxed, stay still, keep their eyes closed, stay awake, and keep their mouth slightly open. If a subject's head moved more than 5 mm during the data collection process, the data were discarded and re-collected. The sampling frequency of MEG was 6,000 Hz and at least six 120 s sessions of MEG data were collected per subject. Noise was eliminated using third-order gradients before data acquisition.
MRI scan
All participants underwent a 3.0 T MRI (Siemens, Germany) scan after MEG data collection. Before MRI scanning, coils similar to those used in MEG data collection were placed at the nose root and in front of both ears to locate each subjects head position.
Data preprocessing
MEG data were processed through the following steps: First, we excluded MEG waveforms with significant noise and artifacts (amplitude > 6 pT), based on previous studies (37). Next, we filtered the MEG data in the frequency range 1–70 Hz and used it to identify high amplitude peaks in SeLECTS. To reduce the impact of spikes, we selected MEG data without spikes for a continuous period of 60 s for processing. Finally, we analyzed the six frequency bands of the selected waveform, namely delta (1–4 Hz), theta (4–8 Hz), alpha (8–12 Hz), beta (12–30 Hz), gamma (30–80 Hz), and ripple (80–250 Hz). The data were filtered before analysis to avoid environmental alternating current (AC) power interference around the 50 Hz band. MEG data from all subjects were analyzed using the MEG processor (https://sites.google.com/site/braincloudx/).
Source localization
Based on previous studies (38–40), we used accumulated source imaging (ASI) to analyze neuromagnetic source activity in multiple frequency bands in the selected MEG data. The sum of the volumes of source activity over a period of time is defined as ASI, which can locate the neuromagnetic sources. ASI can be expressed by the following equation:
Where, ASI represents the accumulated source strength at position r, s indicates the time slice, n represents the time point of the MEG data, and Q represents the source activity at source r and at time point t. We defined s ≥ 1 and s ≤ n/2.
We used two-step beamforming to calculate the source activity and locate the source (37). First, we calculated the lead domain for each source (or voxel), which is called a voxel-based partial sensor, and generated the MEG data matrix. Next, to minimize the influence of coherent sources in the localization of the source, we performed a partial sensor overlay for each voxel (37). We then calculated the covariance of all sensors. We calculated two sets of source images using the vector beamformer and estimated the coherent source and source direction using the variance matrix vector beamformer (41). After these steps, we used the scalar beamformer to generate the source activity (or virtual sensor waveform). The algorithm has been described in detail and validated in prior studies (41).
For each subject, the entire brain was scanned at a resolution of 6 mm. In cases where the distance between two voxels was < 10 mm, they were considered as one source. The individual MRI data were merged with the MEG data by fixing the nasal root and three points in front of the ear at the time of data acquisition. This approach allows us to segment and visualize brain regions.
Statistical analysis
Fisher's exact probability method was used to examine the localization of the main neuromagnetic sources in the three groups. One-way analysis of variance (ANOVA) was performed on the source strength, Wechsler scale scores, and subject age. We also conducted a homogeneity test of variance. We compared the age at onset and duration of disease between the SeLECTS (SWI ≥ 50%) group and SeLECTS (SWI < 50%) group using the two-sample t-test. Pearson or Spearman correlation analysis was used to analyze the relationship between the intensity of the magnetic source and the clinical characteristics of each group of frequency bands. The level of statistical significance was set at p < 0.05 with Bonferroni multiple comparison correction (e.g., for six bands in three groups, p < 0.002). All statistical analyses were performed using SPSS version 24.0 for Windows (SPSS Inc., Chicago, IL, USA).
Results
Participants
The final sample included 14 children with SeLECTS (SWI ≥ 50%) including eight females, with a mean age of 96.94 ± 13.68 months, a mean age of onset of 90.89 ± 15.08 months, a mean disease duration of 6.05 ± 6.06 months, and an average of 2.64 ± 1.45 seizures. The 21 children with SeLECTS (SWI < 50%), which included 10 males, had a mean age of 102.59 ± 19.20 months, a mean age of onset of 97.84 ± 16.25 months, a mean disease duration of 5.22 ± 6.68 months, and an average of 2.00 ± 1.18 seizures. The statistical analysis showed no significant differences between the two groups in terms of gender, age, age of onset, disease duration, or number of seizures. The detailed data of the participants are shown in Table 1.
WISC-IV scores
As shown in Table 2, there were statistically significant differences between the three groups in terms of total score and PRI, with the SeLECTS (SWI ≥ 50%) group having the lowest score followed by the SeLECTS (SWI < 50%) group; the HC group had the highest score. In terms of VCI and WMI, both the SeLECTS (SWI ≥ 50%) group and SeLECTS (SWI < 50%) group had significantly lower scores than the HC group, but there was no statistically significant difference between the scores of the SeLECTS (SWI ≥ 50%) group and SeLECTS (SWI < 50%) group. There were no significant group differences between the PSI scores. Additional details of the performance for each group are provided in Figure 1.
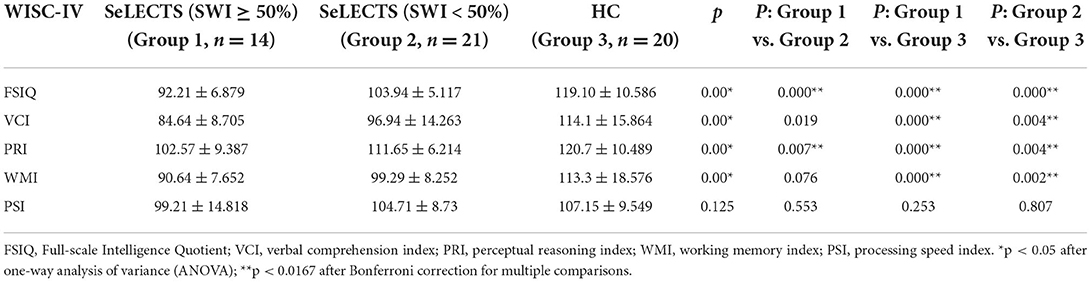
Table 2. Comparison of WISC-IV scores between the SeLECTS (SWI ≥ 50%), SeLECTS (SWI < 50%), and HC groups.
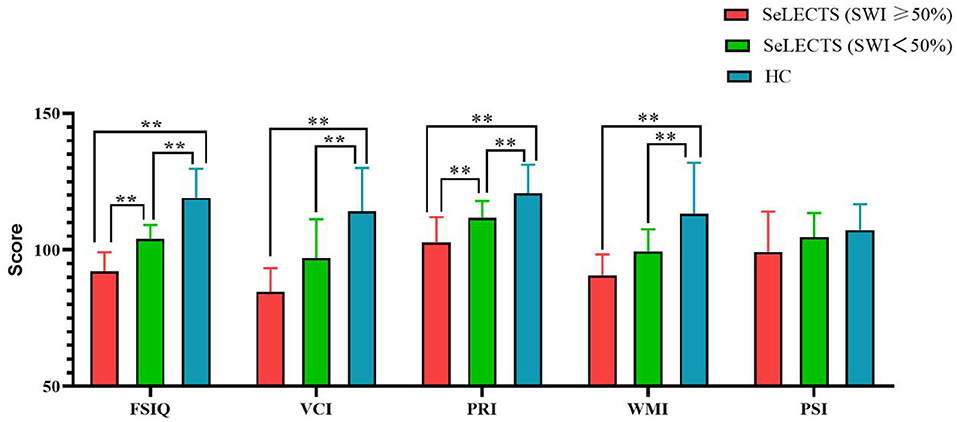
Figure 1. Comparison of WISC-IV scores between the SeLECTS (SWI ≥ 50%), SeLECTS (SWI < 50%), and HC groups. FSIQ, Full-scale Intelligence Quotient; VCI, verbal comprehension index; PRI, perceptual reasoning index; WMI, working memory index; PSI, processing speed index. **, The p-value was statistically significant.
Source location
According to the whole brain accumulative magnetic source imaging, there were 1–3 primary magnetic source locations per subject in the resting state (Figure 2), including the peri-Rolandic area (PR), posterior cingulate cortex (PCC), medial frontal cortex (MFC), medial temporal lobe (MTL), and deep brain area (DBA). In the low frequency band (<80 Hz), there was a significant difference in source localization between the three groups; however, in the high frequency band (>80 Hz), there was not. Specifically, in the alpha (8–12 Hz) band, the SeLECTS (SWI < 50%) group and HC group were mainly localized in the MFC and PCC regions, while the magnetic source in the SeLECTS (SWI ≥ 50%) group was mainly localized in the PCC region. In the gamma band (30–80 Hz), the SeLECTS (SWI ≥ 50%) group and SeLECTS (SWI < 50%) group were mainly localized in the MFC region, while the HC group was mainly localized in the MFC and PCC regions. In the beta (12–30 Hz) frequency band, we found group differences in localization in the PCC region. Post-hoc analysis showed a statistically significant difference in localization in the SeLECTS (SWI ≥ 50%) group compared to the SeLECTS (SWI < 50%) group and HC group, but no statistically significant difference between the SeLECTS group and healthy controls.
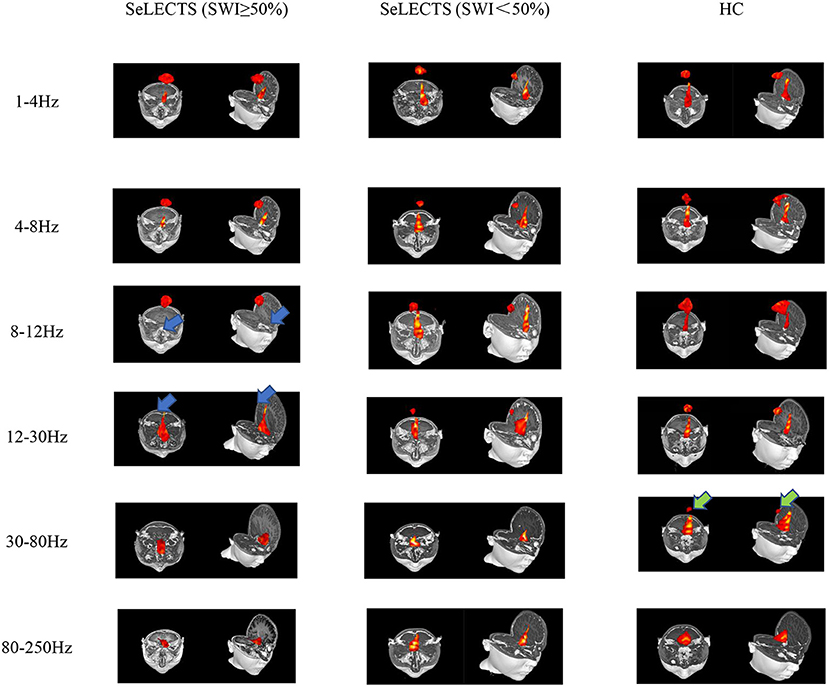
Figure 2. Typical magnetic source localization in the frequency band of 1–250 Hz for three groups of subjects. In the 8–12 and 12–30 Hz frequency bands, the SeLECTS (SWI ≥ 50%) group was inactivated in the PCC region, as indicated by the blue arrows. In the 30–80 Hz band, the HC group is activated in the PCC region as shown by the green arrow.
We did not find significant differences in the other three frequency bands. However, as shown in Figure 2, in the higher frequency bands the magnetic source activity is more frequent in the DBA region than in the lower frequency bands, while in the lower frequency bands the magnetic source activity in the PR region is more frequent than in the higher frequency bands. The localization and statistical comparison of the main magnetic sources in each frequency band for the three groups are detailed in Table 3.
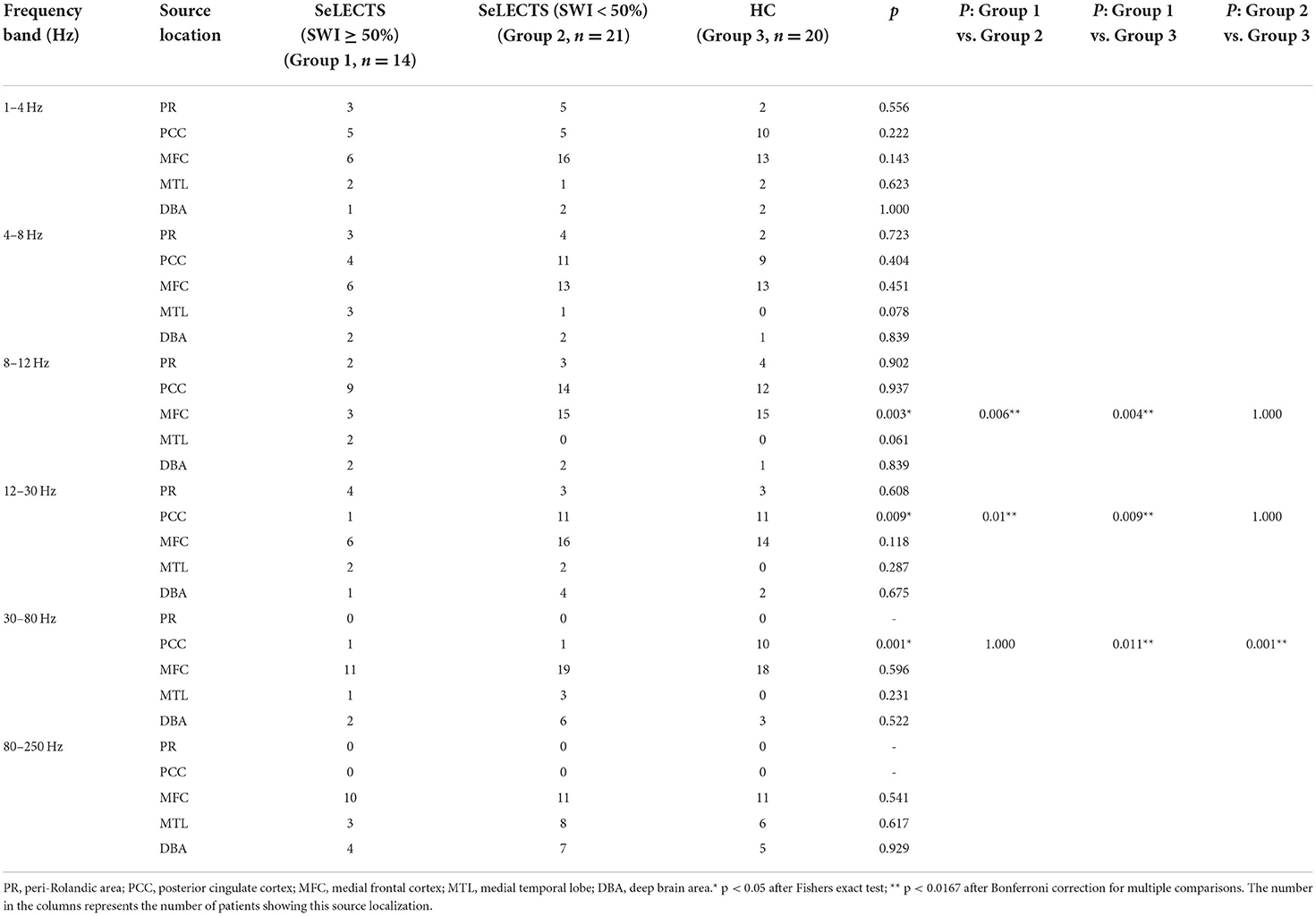
Table 3. Comparison of predominant neuromagnetic activity in the SeLECTS (SWI ≥ 50%), SeLECTS (SWI < 50%), and HC groups.
Source strength
In the delta (1–4 Hz) frequency band, the magnetic source intensity of the SeLECTS (SWI ≥ 50%) group was significantly higher than that of the SeLECTS (SWI < 50%) group and HC group. However, there was no significant difference between the magnetic source intensity of the SeLECTS (SWI < 50%) group and HC group. In the alpha (8–12 Hz) and gamma (30–80 Hz) frequency bands, we found a statistically significant difference in the magnetic source intensity between the three groups; however, the pairwise comparison showed no statistically significant difference between the two groups. In the other three frequency bands, there were no statistically significant group differences. Detailed statistical analysis of the neuromagnetic sources is shown in Table 4.
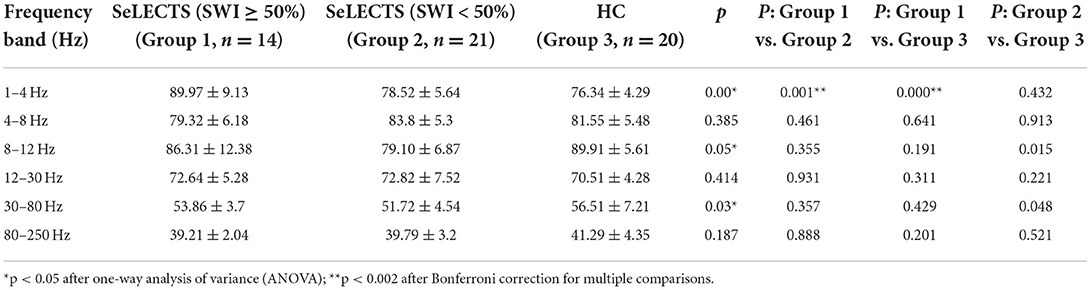
Table 4. Comparison of neuromagnetic source strength between the SeLECTS (SWI ≥ 50%), SeLECTS (SWI < 50%), and HC groups.
Clinical correlation
Our analysis revealed no significant correlation between the magnetic source intensity and clinical data in the SeLECTS (SWI ≥ 50%) group and SeLECTS (SWI < 50%) group. Similarly, there was no significant correlation between the age of onset and disease duration in the two groups.
Discussion
This study has revealed significant differences in neuropsychological evaluation results between unmedicated children with SeLECTS (SWI < 50%) and unmedicated children with SeLECTS (SWI ≥ 50%). Similarly, there were significant differences in magnetic source localization and magnetic source intensity between these two groups compared to the HC group, and they showed some frequency dependence. This is tentatively consistent with our hypothesis that frequent discharges during sleep would alter normal brain magnetic source activity and thus affect cognitive function. To the best of our knowledge, this is the first MEG study of unmedicated SeLECTS (SWI < 50%), unmedicated SeLECTS (SWI ≥ 50%), and HC subjects, as previous studies have mainly used fMRI and focused on relevant functional networks of the brain (42–44).
Clinical data and characteristics
Previous studies (10, 44) suggest that children with SeLECTS (SWI ≥ 50%) have an earlier age of onset, longer disease duration, and more seizures than children with SeLECTS (SWI < 50%). However, we found no such patterns in our sample. During patient recruitment, we found that parents who become more aware of epilepsy disorders are inclined to go to the hospital immediately for even minor petit mal seizures or nocturnal episodes. This means that, regardless of the outcome of SeLECTS, in most cases patients were recruited at the time of the first one or two seizures, resulting in no statistically significant difference between the two groups in terms of duration of illness and number of seizures. However, at follow-up, we found that children with SeLECTS (SWI ≥ 50%) were not as well controlled as children with SeLECTS (SWI < 50%), even with use of AEDs, and continued to have seizures. This further confirms that children with SeLECTS (SWI ≥ 50%) have poorer outcomes, more difficult seizure control, and lower neuropsychological scores (42).
We found no significant difference in the age of onset of SeLECTS (SWI ≥ 50%) compared to SeLECTS (SWI < 50%) children, which is likely because of the sample size. In addition, we did not find a significant correlation between the age at onset and duration of illness. We do not believe that there is a simple linear relationship between magnetic source intensity, age at onset, number of episodes, and duration of illness, which we plan to reconfirm in a larger sample (45).
Neuropsychological test results
We found that SeLECTS (SWI < 50%) patients and SeLECTS (SWI ≥ 50%) scored significantly lower than healthy controls on both the VCI and WMI, although there was no significant difference between the two patient groups. This finding is similar to the results of previous scales assessing SeLECTS patients with unilateral and bilateral discharges (46). In the SeLECTS (SWI ≥ 50%) group, four patients had bilateral discharges; in the SeLECTS (SWI < 50%) group, all 21 had unilateral discharges. However, we did not find a correlation between SeLECTS (SWI ≥ 50%) and bilateral discharges. We hope to expand the sample size in a future study. In terms of total score and PRI, not only did the SeLECTS (SWI ≥ 50%) and SeLECTS (SWI < 50%) group score significantly lower than the HC group, the SeLECTS (SWI ≥ 50%) group also scored significantly lower than the SeLECTS (SWI < 50%) group. There is growing evidence (44, 47, 48) that, because the brain is at a critical stage of development during childhood, frequent epileptiform discharges during this period can affect the formation and development of neural protrusions, leading to widespread cognitive impairment in children with SeLECTS. The neural network theory of epilepsy suggests that abnormal discharges during the interictal period may affect the neuropsychological development of children more than the clinical seizures themselves (9, 49, 50). This could explain why children with SeLECTS (SWI ≥ 50%) have worse cognitive function than SeLECTS (SWI < 50%) patients and healthy controls (42, 44); the higher the number of discharges during the interictal period, the greater the impact on cognitive function.
Our findings showed no significant difference in PSI between the SeLECTS (SWI ≥ 50%) group, SeLECTS (SWI < 50%) group, and healthy controls, which is inconsistent with previous studies of cognitive function in patients with initially diagnosed SeLECTS (35). However, some studies have reported non-significant differences between SeLECTS (SWI < 50%) patients and healthy controls in terms of PSI (46). A follow-up study of SeLECTS patients taking medication for 1 year found no significant increase in PSI over the1 year period (51). In terms of overall trends, we found that the PSI scores and mean scores of children with SeLECTS (SWI ≥ 50%) and SeLECTS (SWI < 50%) were lower than those of healthy controls, but it did not reach the level of statistical significance. Thus, we plan to expand the sample size for further research.
Source localization
The magnetic source analysis revealed that the SeLECTS (SWI ≥ 50%) group showed extensive deactivation of the PCC and MFC regions, specifically in the MFC region in the alpha (8–12 Hz) frequency band and in the PCC region in the beta (12–30 Hz) frequency band. In addition, in the gamma (30–80 Hz) frequency band, the SeLECTS (SWI ≥ 50%) group and SeLECTS (SWI < 50%) group showed inactivation in the PCC region. Although a clear mechanism of cognitive decline in children with SeLECTS has not been identified, some studies suggest that frequent interictal discharges can lead to altered magnetic sources in important brain regions, thus affecting the corresponding functions (40, 52, 53).
The brain functions with network properties that dynamically regulate information interactions between various systems. The default mode network (DMN) is an important network that maintains the basic state of the nervous system, remaining active during resting states and being inhibited during working states or in the presence of external stimuli. The DMN consists mainly of discrete, bilateral, and symmetrical cortical areas located in the medial and lateral parietal, medial prefrontal, and medial and lateral temporal cortices of the brain (54, 55). The MFC and PCC are well-known as core regions of the DMN, and the SeLECTS (SWI ≥ 50%) and SeLECTS (SWI < 50%) groups showed deactivation of MFC and PCC regions in the corresponding frequency bands, suggesting that DMN impairment is an important cause of cognitive decline in children with SeLECTS (56, 57).
Previous studies have found that prefrontal regions in patients with SeLECTS are associated with neuropsychological deficits (10). A study of brain white matter in patients with SeLECTS similarly found that reduced function of frontal regions may be responsible for cognitive impairment (58). In the present study, the SeLECTS (SWI ≥ 50%) group under the alpha frequency band showed deactivation in the MFC region compared to the SeLECTS (SWI < 50%) and HC groups, which explains why the SeLECTS (SWI ≥ 50%) group had poorer cognitive function. Similarly, a study by Li et al. (45) found that magnetic source activity in children with SeLECTS with poorer cognitive function was deactivated in the MFC region.
In a study of changes in brain magnetic activity and cognitive function in SeLECTS patients before and after AEDs, it was found that children with SeLECTS showed activation of the PCC region in several frequency bands after treatment, especially the gamma frequency band. Treated SeLECTS patients also showed significant improvement in VCI and PRI scores, suggesting that PCC regions are closely related to verbal comprehension and perceptual reasoning functions (51). Similarly, our study found that both the SeLECTS (SWI ≥ 50%) and SeLECTS (SWI < 50%) groups were inactivated in the PCC region in the gamma frequency band compared to the HC group. In the beta frequency band, only the SeLECTS (SWI ≥ 50%) group showed deactivation in the PCC region, while the SeLECTS (SWI < 50%) and HC groups showed activation, suggesting that PCC deactivation in multiple frequency bands affects, not only a specific band, affects patients cognitive function.
Previous studies (55, 56) suggest that network reorganization of the DMN leads to altered cognitive function in SeLECTS patients. Thus, in the present study, we hypothesized a causal relationship between magnet source inactivation and network reorganization, and that magnet source inactivation would lead to impaired corresponding functional connectivity of the DMN (45). In addition, we found no significant difference in the number of seizures between the SeLECTS (SWI ≥ 50%) and SeLECTS (SWI < 50%) groups. However, there was a significant difference in cognitive function between the two groups, which suggests that frequent nighttime sleep discharges are the main cause of cognitive decline in SeLECTS patients, rather than seizures.
Notably, not only in the SeLECTS group, but also in a MEG study of Childhood absence epilepsy (CAE), we found that the higher the frequency band, the more the magnetic source activity in epileptic patients tended to be distributed in the deep brain; the exact mechanism of this has not yet been clarified (40). In the future, we plan to analyze magnetic source activity in higher frequency bands by expanding the sample size and using more sophisticated MEG processing software.
Source strength
In the delta (1–4 Hz) frequency band, the magnetic source intensity in the SeLECTS (SWI ≥ 50%) group was significantly higher than that in the SeLECTS (SWI < 50%) group and HC group. In a prior MEG study (35) examining the cognitive function of children with SeLECTS not taking AEDs, we found that, in the gamma (30–80 Hz) frequency band, the magnetic source activity intensity was highest in SeLECTS children in the group with poorer cognitive function. These results are consistent with a previous MEG study (41) showing that increased magnetic source intensity in children with epilepsy during the interictal period was attributed to frequent discharges. Another MEG study (53) of interictal discharges in SeLECTS confirmed our finding that patients with epilepsy who had interictal discharges had higher magnetic source intensity in the delta (1–4 Hz) frequency band.
Limitations
This study is subject to several limitations. Firstly, the sample size for each group was relatively small. We plan to expand the sample size to validate the experimental results in future studies. Secondly, because unable to assess the patient's eventual cognitive decline, we were making impossible to make a diagnosis of DEE-SWAS and EE-SWAS, and we will more fully document the patient's seizures and subsequent cognitive decline in a follow-up study. As a third point, we artifacts in EMG, ECG, and other signals may interfere with the acquisition of MEG information, although we utilized various methods to eliminate these effects. Finally, the technology of the magnetic source imaging software used in this study is limited; therefore, we need to use other magnetic source imaging software in follow-up research to validate these results.
Conclusion
This study investigated the relationship between magnetic brain activity and cognitive function in children with SeLECTS (SWI ≥ 50%) compared to SeLECTS (SWI < 50%) patients and healthy controls. We found a significant decrease in cognitive function in the SeLECTS (SWI ≥ 50%) group compared to the SeLECTS (SWI < 50%) and HC groups, and a significant difference in magnetic source activity between the three groups. Deactivation of magnetic source activity in the PCC and MFC regions was the main cause of cognitive function decline in SeLECTS patients and showed some frequency dependence. We intend to follow up with these patients and to clarify the prognosis of SeLECTS (SWI ≥ 50%) vs. SeLECTS (SWI < 50%) and the corresponding changes in magnetic brain activity.
Data availability statement
The raw data supporting the conclusions of this article will be made available by the authors, without undue reservation.
Ethics statement
The studies involving human participants were reviewed and approved by the Medical Ethics Committee of Nanjing Medical University, Nanjing Brain Hospital and Nanjing Children's Hospital. Written informed consent to participate in this study was provided by the patients/participants' legal guardian/next of kin. Written informed consent was obtained from the minor(s)' legal guardian/next of kin for the publication of any potentially identifiable images or data included in this article.
Author contributions
YaL, YiL, JS, and XW designed the research. YaL, YX, KN, and PW recruited the participants and analyzed the data. YaL, YW, KZ, and QC acquired the images. XW revised the manuscript. All authors approved the final submitted version and agreed to be accountable for its content.
Funding
This study was supported by the General Program of Natural Science Foundation of Jiangsu Province (Grant No. BK20191127), the Health Department of Jiangsu Province (Grant No. H2018062), the Medical and Health International Cooperation Project of Nanjing Municipal Science and Technology Bureau (Grant No. 201911044), and the National Natural Science Foundation of China (Grant No. 82071455).
Conflict of interest
The authors declare that the research was conducted in the absence of any commercial or financial relationships that could be construed as a potential conflict of interest.
Publisher's note
All claims expressed in this article are solely those of the authors and do not necessarily represent those of their affiliated organizations, or those of the publisher, the editors and the reviewers. Any product that may be evaluated in this article, or claim that may be made by its manufacturer, is not guaranteed or endorsed by the publisher.
References
1. Dryzałowski P, Józwiak S, Franckiewicz M, Strzelecka J. Benign epilepsy with centrotemporal spikes - current concepts of diagnosis and treatment. Neurol Neurochir Pol. (2018) 52:677–89. doi: 10.1016/j.pjnns.2018.08.010
2. Specchio N, Wirrell EC, Scheffer IE, Nabbout R, Riney K, Samia P, et al. International league against epilepsy classification and definition of epilepsy syndromes with onset in childhood: position paper by the ILAE task force on nosology and definitions. Epilepsia. (2022) 63:1398–442. doi: 10.1111/epi.17241
3. Berg AT, Berkovic SF, Brodie MJ, Buchhalter J, Cross JH, van Emde Boas W, et al. Revised terminology and concepts for organization of seizures and epilepsies: report of the ILAE commission on classification and terminology, 2005-2009. Epilepsia. (2010) 51:676–85. doi: 10.1111/j.1528-1167.2010.02522.x
4. Liu MJ, Su XJ, Md XS, Wu GF, Zhang YQ, Gao L, et al. Clinical features of benign epilepsy of childhood with centrotemporal spikes in chinese children. Medicine. (2017) 96:e5623. doi: 10.1097/MD.0000000000005623
5. Piccinelli P, Borgatti R, Aldini A, Bindelli D, Ferri M, Perna S, et al. Academic performance in children with rolandic epilepsy. Dev Med Child Neurol. (2008) 50:353–6. doi: 10.1111/j.1469-8749.2008.02040.x
6. Parisi P, Paolino MC, Raucci U, Ferretti A, Villa MP, Trenite DK. “Atypical forms” of benign epilepsy with centrotemporal spikes (BECTS): how to diagnose and guide these children. a practical/scientific approach. Epilepsy Behav. (2017) 75:165–9. doi: 10.1016/j.yebeh.2017.08.001
7. Genizi J, Shamay-Tsoory SG, Shahar E, Yaniv S, Aharon-Perez J. Impaired social behavior in children with benign childhood epilepsy with centrotemporal spikes. J Child Neurol. (2012) 27:156–61. doi: 10.1177/0883073811414420
8. Ciumas C, Laurent A, Saignavongs M, Ilski F, de Bellescize J, Panagiotakaki E, et al. Behavioral and fMRI responses to fearful faces are altered in benign childhood epilepsy with centrotemporal spikes (BCECTS). Epilepsia. (2017) 58:1716–27. doi: 10.1111/epi.13858
9. Vannest J, Tenney JR, Gelineau-Morel R, Maloney T, Glauser TA. Cognitive and behavioral outcomes in benign childhood epilepsy with centrotemporal spikes. Epilepsy Behav. (2015) 45:85–91. doi: 10.1016/j.yebeh.2015.01.041
10. He W, Liu H, Liu Z, Wu Q. Electrical status epilepticus in sleep affects intrinsically connected networks in patients with benign childhood epilepsy with centrotemporal spikes. Epilepsy Behav. (2020) 106:107032. doi: 10.1016/j.yebeh.2020.107032
11. Galanopoulou AS, Bojko A, Lado F, Moshé SL. The spectrum of neuropsychiatric abnormalities associated with electrical status epilepticus in sleep. Brain Dev. (2000) 22:279–95. doi: 10.1016/S0387-7604(00)00127-3
12. Li R, Liao W, Yu Y, Chen H, Guo X, Tang YL, et al. Differential patterns of dynamic functional connectivity variability of striato-cortical circuitry in children with benign epilepsy with centrotemporal spikes. Hum Brain Mapp. (2018) 39:1207–17. doi: 10.1002/hbm.23910
13. Magara S, Komatsubara T, Hojo M, Kobayashi Y, Yoshino M, Saitoh A, et al. The association of epileptic focus estimated by magnetoencephalography with cognitive function in non-lesional epilepsy with continuous spikes and waves during slow wave sleep (ECSWS) children. Brain Dev. (2019) 41:163–72. doi: 10.1016/j.braindev.2018.09.005
14. Wickens S, Bowden SC, D'Souza W. Cognitive functioning in children with self-limited epilepsy with centrotemporal spikes: a systematic review and meta-analysis. Epilepsia. (2017) 58:1673–85. doi: 10.1111/epi.13865
15. Overvliet GM, Besseling RM, Jansen JF, van der Kruijs SJ, Vles JS, Hofman PA, et al. Early onset of cortical thinning in children with rolandic epilepsy. Neuroimage Clin. (2013) 2:434–9. doi: 10.1016/j.nicl.2013.03.008
16. Patry G, Lyagoubi S, Tassinari CA. Subclinical “electrical status epilepticus” induced by sleep in children. a clinical and electroencephalographic study of six cases. Arch Neurol. (1971) 24:242–52. doi: 10.1001/archneur.1971.00480330070006
17. Weber AB, Albert DV, Yin H, Held TP, Patel AD. Diagnosis of electrical status epilepticus during slow-wave sleep with 100 seconds of sleep. J Clin Neurophysiol. (2017) 34:65–8. doi: 10.1097/WNP.0000000000000307
18. Wiwattanadittakul N, Depositario-Cabacar D, Zelleke TG. Electrical status epilepticus in sleep (ESES) - treatment pattern and EEG outcome in children with very high spike-wave index. Epilepsy Behav. (2020) 105:106965. doi: 10.1016/j.yebeh.2020.106965
19. Kevelam SH, Jansen FE, Binsbergen E, Braun KP, Verbeek NE, Lindhout D, et al. Copy number variations in patients with electrical status epilepticus in sleep. J Child Neurol. (2012) 27:178–82. doi: 10.1177/0883073811416006
20. Inutsuka M, Kobayashi K, Oka M, Hattori J, Ohtsuka Y. Treatment of epilepsy with electrical status epilepticus during slow sleep and its related disorders. Brain Dev. (2006) 28:281–6. doi: 10.1016/j.braindev.2005.09.004
21. Scheltens-de Boer M. Guidelines for EEG in encephalopathy related to ESES/CSWS in children. Epilepsia. (2009) 50:13–7. doi: 10.1111/j.1528-1167.2009.02211.x
22. Wilson TW, Heinrichs-Graham E, Proskovec AL, McDermott TJ. Neuroimaging with magnetoencephalography: a dynamic view of brain pathophysiology. Transl Res. (2016) 175:17–36. doi: 10.1016/j.trsl.2016.01.007
23. Guggisberg AG, Honma SM, Findlay AM, Dalal SS, Kirsch HE, Berger MS, et al. Mapping functional connectivity in patients with brain lesions. Ann Neurol. (2008) 63:193–203. doi: 10.1002/ana.21224
24. Burgess RC. Evaluation of brain connectivity: the role of magnetoencephalography. Epilepsia. (2011) 52:28–31. doi: 10.1111/j.1528-1167.2011.03148.x
25. Harmsen IE, Rowland NC, Wennberg RA, Lozano AM. Characterizing the effects of deep brain stimulation with magnetoencephalography: a review. Brain Stimul. (2018) 11:481–91. doi: 10.1016/j.brs.2017.12.016
26. Wang Z, Zhang Z, Liao W, Xu Q, Zhang J, Lu W, et al. Frequency-dependent amplitude alterations of resting-state spontaneous fluctuations in idiopathic generalized epilepsy. Epilepsy Res. (2014) 108:853–60. doi: 10.1016/j.eplepsyres.2014.03.003
27. Kudo T, Nishida T, Yagi K. Discontinuation and duration of antiepileptic drug therapy: a retrospective study of factors for specific epileptic syndromes. Epilepsia. (2004) 45:26–32. doi: 10.1111/j.0013-9580.2004.458006.x
28. Stephen J, Weir CJ, Chin RF. Temporal trends in incidence of Rolandic epilepsy, prevalence of comorbidities and prescribing trends: birth cohort study. Arch Dis Child. (2020) 105:569–74. doi: 10.1136/archdischild-2019-318212
29. Xu Q, Hu Z, Yang F, Bernhardt BC, Zhang Q, Stufflebeaskm SM, et al. Resting state signal latency assesses the propagation of intrinsic activations and estimates anti-epileptic effect of levetiracetam in Rolandic epilepsy. Brain Res Bull. (2020) 162:125–31. doi: 10.1016/j.brainresbull.2020.05.016
30. Wu L, Yang X, Wang X, Yu S, Yang B. The attention networks in benign epilepsy with centrotemporal spikes: a long-term follow-up study. J Clin Neurosci. (2021) 88:22–7. doi: 10.1016/j.jocn.2021.03.022
31. Tekgul H, Kanmaz S, Serin HM, Yilmaz S. Spike wave characteristics and temporal spike evolution on serial EEG in childhood epilepsy with centrotemporal spikes. Seizure. (2021) 87:75–80. doi: 10.1016/j.seizure.2021.02.032
32. Fisher RS, Cross JH, French JA, Higurashi N, Hirsch E, Jansen FE, et al. Operational classification of seizure types by the international league against epilepsy: position paper of the ILAE commission for classification and terminology. Epilepsia. (2017) 58:522–30. doi: 10.1111/epi.13670
33. Gencpinar P, Dundar NO, Tekgul H. Electrical status epilepticus in sleep (ESES)/continuous spikes and waves during slow sleep (CSWS) syndrome in children: an electroclinical evaluation according to the EEG patterns. Epilepsy Behav. (2016) 61:107–11. doi: 10.1016/j.yebeh.2016.05.014
34. Baron IS. Test review: wechsler intelligence scale for children-fourth edition (WISC-IV). Child Neuropsychol. (2005) 11:471–5. doi: 10.1080/09297040590951587
35. Li Y, Sun Y, Zhang T, Shi Q, Sun J, Xiang J, et al. The relationship between epilepsy and cognitive function in benign childhood epilepsy with centrotemporal spikes. Brain Behav. (2020) 10:e01854. doi: 10.1002/brb3.1854
36. Yang P, Cheng CP, Chang CL, Liu TL, Hsu HY, Yen CF. Wechsler Intelligence Scale for Children 4th edition-Chinese version index scores in Taiwanese children with attention-deficit/hyperactivity disorder. Psychiatry Clin Neurosci. (2013) 67:83–91. doi: 10.1111/pcn.12014
37. Xiang J, Tenney JR, Korman AM, Leiken K, Rose DF, Harris E, et al. Quantification of interictal neuromagnetic activity in absence epilepsy with accumulated source imaging. Brain Topogr. (2015) 28:904–14. doi: 10.1007/s10548-014-0411-5
38. Jiang W, Sun J, Xiang J, Sun Y, Tang L, Zhang K, et al. Altered neuromagnetic activity in persistent postural-perceptual dizziness: a multifrequency magnetoencephalography study. Front Hum Neurosci. (2022) 16:759103. doi: 10.3389/fnhum.2022.759103
39. Miao A, Wang Y, Xiang J, Liu Q, Chen Q, Qiu W, et al. Ictal source locations and cortico-thalamic connectivity in childhood absence epilepsy: associations with treatment response. Brain Topogr. (2019) 32:178–91. doi: 10.1007/s10548-018-0680-5
40. Sun J, Gao Y, Miao A, Yu C, Tang L, Huang S, et al. Multifrequency dynamics of cortical neuromagnetic activity underlying seizure termination in absence epilepsy. Front Hum Neurosci. (2020) 14:221. doi: 10.3389/fnhum.2020.00221
41. Xiang J, Korman A, Samarasinghe KM, Wang X, Zhang F, Qiao H, et al. Volumetric imaging of brain activity with spatial-frequency decoding of neuromagnetic signals. J Neurosci Methods. (2015) 239:114–28. doi: 10.1016/j.jneumeth.2014.10.007
42. Ucar HK, Arhan E, Aydin K, Hirfanoglu T, Serdaroglu A. Electrical status epilepticus during sleep (ESES) in benign childhood epilepsy with centrotemporal spikes (BCECTS): insights into predictive factors, and clinical and EEG outcomes. Eur Rev Med Pharmacol Sci. (2022) 26:1885–96. doi: 10.26355/eurrev_202203_28334
43. Uliel-Sibony S, Kramer U. Benign childhood epilepsy with centro-temporal spikes (BCECTSs), electrical status epilepticus in sleep (ESES), and academic decline–how aggressive should we be? Epilepsy Behav. (2015) 44:117–20. doi: 10.1016/j.yebeh.2015.01.004
44. Nissenkorn A, Pappo A, Feldmann Y, Heimer G, Bar-Yosef O, Tzadok M, et al. Influence of epileptic activity during sleep on cognitive performance in benign childhood epilepsy with centrotemporal spikes. Eur J Paediatr Neurol. (2017) 21:858–63. doi: 10.1016/j.ejpn.2017.07.001
45. Li Y, Sun Y, Niu K, Wang P, Xiang J, Chen Q, et al. The relationship between neuromagnetic activity and cognitive function in benign childhood epilepsy with centrotemporal spikes. Epilepsy Behav. (2020) 112:107363. doi: 10.1016/j.yebeh.2020.107363
46. Wang P, Li Y, Sun Y, Sun J, Niu K, Zhang K, et al. Altered functional connectivity in newly diagnosed benign epilepsy with unilateral or bilateral centrotemporal spikes: a multi-frequency MEG study. Epilepsy Behav. (2021) 124:108276. doi: 10.1016/j.yebeh.2021.108276
47. De Negri M, Baglietto MG, Battaglia FM, Gaggero R, Pessagno A, Recanati L. Treatment of electrical status epilepticus by short diazepam (DZP) cycles after DZP rectal bolus test. Brain Dev. (1995) 17:330–3. doi: 10.1016/0387-7604(95)00076-N
48. Luo C, Zhang Y, Cao W, Huang Y, Yang F, Wang J, et al. Altered structural and functional feature of striato-cortical circuit in benign epilepsy with centrotemporal spikes. Int J Neural Syst. (2015) 25:1550027. doi: 10.1142/S0129065715500276
49. Kobayashi K, Nishibayashi N, Ohtsuka Y, Oka E, Ohtahara S. Epilepsy with electrical status epilepticus during slow sleep and secondary bilateral synchrony. Epilepsia. (1994) 35:1097–103. doi: 10.1111/j.1528-1157.1994.tb02561.x
50. Samaitiene R, Norkuniene J, Tumiene B, Grikiniene J. Sleep and behavioral problems in rolandic epilepsy. Pediatr Neurol. (2013) 48:115–22. doi: 10.1016/j.pediatrneurol.2012.10.012
51. Niu K, Li Y, Zhang T, Sun J, Sun Y, Shu M, et al. Impact of antiepileptic drugs on cognition and neuromagnetic activity in childhood epilepsy with centrotemporal spikes: a magnetoencephalography study. Front Hum Neurosci. (2021) 15:720596. doi: 10.3389/fnhum.2021.720596
52. Zhang K, Sun J, Sun Y, Niu K, Wang P, Wu C, et al. Pretreatment source location and functional connectivity network correlated with therapy response in childhood absence epilepsy: a magnetoencephalography study. Front Neurol. (2021) 12:692126. doi: 10.3389/fneur.2021.692126
53. Zhang T, Shi Q, Li Y, Gao Y, Sun J, Miao A, et al. Frequency-dependent interictal neuromagnetic activities in children with benign epilepsy with centrotemporal spikes: a magnetoencephalography (MEG) study. Front Hum Neurosci. (2020) 14:264. doi: 10.3389/fnhum.2020.00264
54. Raichle ME. The brain's default mode network. Annu Rev Neurosci. (2015) 38:433–47. doi: 10.1146/annurev-neuro-071013-014030
55. Parsons N, Bowden SC, Vogrin S, D'Souza WJ. Default mode network dysfunction in idiopathic generalised epilepsy. Epilepsy Res. (2020) 159:106254. doi: 10.1016/j.eplepsyres.2019.106254
56. Fahoum F, Zelmann R, Tyvaert L, Dubeau F, Gotman J. Epileptic discharges affect the default mode network–FMRI and intracerebral EEG evidence. PLoS ONE. (2013) 8:e68038. doi: 10.1371/journal.pone.0068038
57. Tenney JR, Fujiwara H, Horn PS, Vannest J, Xiang J, Glauser TA, et al. Low- and high-frequency oscillations reveal distinct absence seizure networks. Ann Neurol. (2014) 76:558–67. doi: 10.1002/ana.24231
Keywords: electrical status epilepticus of sleep (ESES), magnetoencephalography (MEG), cognitive function, brain activity, self-limited epilepsy with centrotemporal spikes, sleep spiking activation
Citation: Li Y, Li Y, Sun J, Niu K, Wang P, Xu Y, Wang Y, Chen Q, Zhang K and Wang X (2022) Relationship between brain activity, cognitive function, and sleep spiking activation in new-onset self-limited epilepsy with centrotemporal spikes. Front. Neurol. 13:956838. doi: 10.3389/fneur.2022.956838
Received: 30 May 2022; Accepted: 07 October 2022;
Published: 09 November 2022.
Edited by:
Julia Jacobs, University of Freiburg Medical Center, GermanyReviewed by:
Maria Paola Canevini, Dipartimento di Scienze della Salute Università degli Studi, Milano, ItalyPatrick Van Bogaert, Université d'Angers, France
Copyright © 2022 Li, Li, Sun, Niu, Wang, Xu, Wang, Chen, Zhang and Wang. This is an open-access article distributed under the terms of the Creative Commons Attribution License (CC BY). The use, distribution or reproduction in other forums is permitted, provided the original author(s) and the copyright owner(s) are credited and that the original publication in this journal is cited, in accordance with accepted academic practice. No use, distribution or reproduction is permitted which does not comply with these terms.
*Correspondence: Xiaoshan Wang, d2FuZ3hpYW9zaGFuMTk3MUAxNjMuY29t