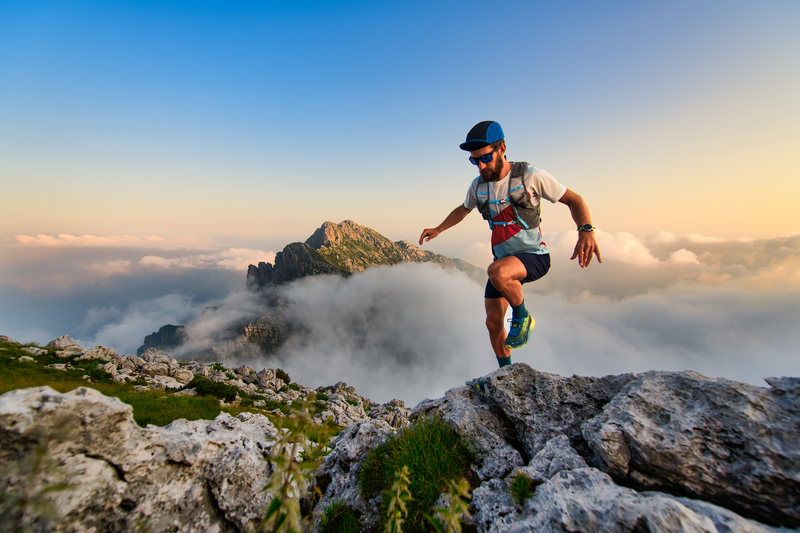
95% of researchers rate our articles as excellent or good
Learn more about the work of our research integrity team to safeguard the quality of each article we publish.
Find out more
ORIGINAL RESEARCH article
Front. Neurol. , 03 August 2022
Sec. Neurotrauma
Volume 13 - 2022 | https://doi.org/10.3389/fneur.2022.944608
Objective: This study was designed to develop and validate a risk-prediction nomogram to predict a 6-month unfavorable prognosis in patients with traumatic brain-injured (TBI) undergoing primary decompressive craniectomy (DC).
Methods: The clinical data of 391 TBI patients with primary DC who were admitted from 2012 to 2020 were reviewed, from which 274 patients were enrolled in the training group, while 117 were enrolled in the internal validation group, randomly. The external data sets containing 80 patients were obtained from another hospital. Independent predictors of the 6-month unfavorable prognosis were analyzed using multivariate logistic regression. Furthermore, a nomogram prediction model was constructed using R software. After evaluation of the model, internal and external validations were performed to verify the efficiency of the model using the area under the receiver operating characteristic curves and the calibration plots.
Results: In multivariate analysis, age(p = 0.001), Glasgow Score Scale (GCS) (p < 0.001), operative blood loss of >750 ml (p = 0.045), completely effaced basal cisterns (p < 0.001), intraoperative hypotension(p = 0.001), and activated partial thromboplastin time (APTT) of >36 (p = 0.012) were the early independent predictors for 6-month unfavorable prognosis in patients with TBI after primary DC. The AUC for the training, internal, and external validation cohorts was 0.93 (95%CI, 0.89–0.96, p < 0.0001), 0.89 (95%CI, 0.82–0.94, p < 0.0001), and 0.90 (95%CI, 0.84–0.97, p < 0.0001), respectively, which indicated that the prediction model had an excellent capability of discrimination. Calibration of the model was exhibited by the calibration plots, which showed an optimal concordance between the predicted 6-month unfavorable prognosis probability and actual probability in both training and validation cohorts.
Conclusion: This prediction model for a 6-month unfavorable prognosis in patients with TBI undergoing primary DC can evaluate the prognosis accurately and enhance the early identification of high-risk patients.
The significant role of decompressive craniectomy (DC) in the treatment of severe traumatic brain injury (TBI) has been verified by a large number of clinical trials and experiences (1). To date, two famous multicenter randomized controlled trials (RCTs) have been conducted on the indication and effectiveness of DC in the treatment of TBI, both of which focus on patients undergoing secondary DC (2, 3). However, few studies have investigated primary DC as a point of interest. In emergency situations, such as a massive intracranial lesion, an observably progressive decline in the patient's consciousness, and even hernia, primary DC may be the only way to relieve high intracranial pressure (ICP) refractory to medical treatment and save the patient's life (4–6). However, a series of factors, such as critical condition at presentation, prompt change in status after admission, and insufficient preoperative preparation, have resulted in high postoperative mortality and unreasonable prognosis for some patients after primary DC; furthermore, most of the deaths occur in the early stage of the disease (7–11).
In our previous study on early predictors of 30-day mortality after primary DC in patients with TBI, we observed an early death rate of 30.8% (12). However, not all patients who survived the decompressive procedure showed a promising prognosis. Some patients fell into a vegetative state or even died of organ failure a few months later, which resulted in the placement of not only a heavy economic burden but also an extremely large psychological burden on the relatives of the patient (7, 8, 13). However, doctors can find it difficult to give a clear prognosis to patients after DC based only on their experience. Therefore, it is necessary to establish a model to predict patient prognosis early that can not only enable us to understand disease progression but also take reasonable interventional measures in the early stages after trauma to improve prognosis. In addition, the early prediction model should not only provide a reference for the decision-making of families with different cultures and beliefs but also encourage the rational allocation of medical resources.
As a continuation of our previous study, in the present study, we built an early (soon after DC) prediction model for the 6-month unfavorable clinical outcomes of patients with TBI after primary DC and then further validated the constructed model internally and externally so that it can be used conveniently in the clinic. The outcome variable we used was the Glasgow Outcome Scale (GOS) score (14). Notably, we only regarded death and vegetative state (GOS scores of 1 and 2, respectively) as unfavorable outcomes in this study. The reason for this classification is that severe disability seems to be more acceptable to the patient's family than a vegetative state (15).
Clinical data were obtained from the database of Ganzhou People's Hospital, Jiangxi Province, China. We retrospectively reviewed the records of 422 patients with primary DC from January 2012 to January 2020. For these patients, primary DC was performed as the first therapeutic procedure after the initial phase of resuscitation (usually <24 h). Our exclusion criteria were as follows: (1) since the object of the study is primary DC, secondary DC should not be considered; (2) loss to follow-up or incomplete data within 6 months; (3) abandonment by the guardians or accidental death; (4) posterior fossa decompression or craniocerebral penetrating injury; (5) severe underlying pathologies or brain stem injury; and (6) bilateral massive cerebral infarction and circulatory failure after admission. After applying these criteria, 391 patients were finally enrolled in this study.
The 391 selected patients were additionally divided into a training cohort (n = 274) and an internal validation cohort (n = 117) randomly at a ratio of 7:3. The training data were used to construct the nomogram, whereas the internal validation data were used to verify the repeatability of the prediction model.
External validation was also conducted in this study. The external data sets were obtained from the Department of Neurosurgery of Huichang County People's Hospital, a single comprehensive medical center that serves more than 700,000 people in China. From April 2016 to March 2019, 80 patients underwent primary DC due to craniocerebral injury. By querying the follow-up records of these patients and applying the current study protocols, 74 patients were finally included in the external validation cohort.
The GOS was used to obtain an assessment of the quality of life. According to the follow-up results, the status of each patient at the end of the observation period was recorded and evaluated according to the GOS(15), of which a score of 1 was death and a score from 2 to 5 represented different survival states from an unfavorable to a favorable outcome. Accordingly, the patients were divided into two groups: the patients with a score of 1–2 were placed in the 6-month unfavorable prognosis group, whereas patients with scores of 3, 4, and 5 were included in the 6-month favorable prognosis group.
(1) The demographic baseline data that characterized the study population included age and sex.
(2) The clinical features included the Glasgow Coma Scale (GCS) score and pupil status at the time of DC, abrupt changes soon after admission, hypoxia before the operation, and injury severity score (ISS) (16) of each patient.
(3) Imaging features obtained from the computerized tomography (CT) scans of each patient included subdural hematoma, epidural hematoma, cerebral contusion, subarachnoid hemorrhage, and the status of the cistern. The status of the cistern was defined as either partially or completely effaced.
(4) Surgical data included operative blood loss, intraoperative hypotension, and postoperative hematoma.
(5) We classified the laboratory auxiliary examination results according to the most common clinical classification and relevant literature, including hemoglobin (HB) (g/L), fibrin (FIB) (g/L), activated partial thromboplastin time (APTT) (s), blood glucose (mmol/L), and blood calcium (mmol/L) (16–19).
The data from the training cohort (n = 274) were used to perform univariate and multivariate analyses to identify the independent predictive factors for a poor outcome and formulate a nomogram. Then, the internal (n = 117) and external (n = 74) validation cohorts were used to validate the nomogram in terms of discrimination and calibration. The discriminative power of the nomogram was evaluated by the area under the receiver operating characteristic (ROC) curve (AUC). The nomogram was calibrated by assessing the concordance between the predicted and observed probabilities. In the present study, calibration plots were constructed to evaluate the goodness-of-fit of the nomogram.
All continuous variables in the present study had non-normal distributions and thus are expressed as medians (with 25th and 75th quartiles), while categorical variables are presented as frequencies (percentages). For non-normally distributed variables, the Mann–Whitney U-test was used, and for categorical variables, the chi-square test was used when appropriate.
A univariate analysis was conducted for the training cohort to test potential predictive factors, and odds ratios (ORs) and their 95% confidence intervals (CIs) were calculated. Any variables found to be significant (p < 0.05) or nearly significant (p <0.2) were qualified for the multivariate analysis.
To facilitate the interpretation of the results, we transformed the continuous variables into categorical variables. According to our clinical experience and values in the literature, some variables, such as Hb, FIB, APTT, serum glucose, and serum calcium, were dichotomized using cutoff points of 10 g/dL, <2 g/L, >36 s, >200 mg/dL, and <2.1 mmol/L, respectively, while the GCS score ( ≤ 5, 5–8, >8) was considered a tricategorical variable (18–24). The best cutoff values for ISS and blood loss were 25 and 750 ml, respectively, according to the maximum Youden's index obtained from ROC curve analysis. Due to the inconsistent classification of age in each study, after analyzing the segmented effect of the relationship between age and prognosis and referencing the CRASH model (25), we finally classified age into eight levels: <20, 20–24, 25–29, 30–34, 35–44, 45–54, 55–64, and ≥65. To assess the independent predictors for an unfavorable 6-month neurological outcome, a multivariate logistic regression model (Forward: LR) was further built.
An individualized nomogram model for predicting the 6-month outcome was then constructed based on the results of multivariate logistic regression in the training cohort. To evaluate the discriminability and calibration of the model, data from the internal and external cohorts were applied to the nomogram. The discriminability of the nomogram was assessed in terms of the AUC with a 95% CI (26). The value of the AUC should fall between 0.5 and 1, in which an AUC>0.75 is considered favorable discrimination. To evaluate the calibration of the nomogram, bootstrap-corrected calibration plots were generated. In view of some limitations of the Hosmer–Lemeshow test, in the present study, we directly observed the concordance between the predicted and observed probabilities (27).
The univariate and multivariate analyses were performed using SPSS (version 20.0, IBM SPSS Statistics). ROC curves were generated using MedCalc software (version 15.8, Belgium). The model was constructed and validated using R for Windows (version 4.0.3, USA).
After applying the inclusion and exclusion criteria, a total of 391 patients with primary DC were enrolled, of whom 274 and 117 were randomly included in the training and internal validation cohorts, respectively. There were 182 (46.5%) 6-month poor prognosis events during the follow-up period for the entire cohort. The median age at presentation was 47 years (range 35–59.5), and the number of male patients in our study was 316 (80.8%), indicating a sex preference. The median GCS score at the time of DC was 6 (range 4–8). Due to having undergone severe trauma, the patients' ISSs were universally high, with a median score of 26 (range 17–33). Table 1 summarizes patient demographics, clinical characteristics, and surgical and laboratory data for the total, training, and validation cohorts. Additionally, all the indicators were comparable between the training and internal validation groups, which indicated that the randomization protocol was reasonable.
The results of the univariate analysis in the training cohort are summarized in Table 2, which demonstrate that the majority of the variables, except sex, cerebral contusion, and postoperative hematoma were considered potential predictors for an unfavorable 6-month prognosis (p <0.2). Before conducting the multivariate analysis, continuous variables were transformed into categorical variables according to our statistical protocols, as described in detail in the “Methods-Data statistical analysis” section.
Table 3 summarizes the results of the multivariate logistic regression analysis, which identified age (p = 0.001), GCS score (p < 0.001), operative blood loss of >750 ml (p = 0.045), completely effaced basal cisterns (p < 0.001), intraoperative hypotension (p = 0.001), and APTT>36 (p = 0.012) as significant predictors of an unfavorable 6-month prognosis. These independent risk factors were then incorporated to construct a prognostic nomogram for the early assessment of the 6-month prognosis (Figure 1). As shown in the nomogram, a score was obtained for each predictor, which was then summarized as the total score. The predicted risk corresponding to the total score is the probability of an overall 6-month unfavorable prognosis after primary DC.
Figure 1. Nomogram to predict the probability of 6-month prognosis in patients with TBI undergoing primary DC (when using this nomogram, the value of each status of the patient will be located on each variable axis, and a vertical line will be drawn to determine the score of each variable value. The total score of the sum of all variable values is on the total score axis. The total score axis corresponds to the probability of a poor prognosis).
The nomogram was internally and externally validated based on the discriminability and calibration ability of the model. The discriminability of the nomogram was estimated in terms of the AUC with 95% CI. ROC curves were drawn for the training, internal, and external validation cohorts (Figure 2). The AUCs for the training, internal, and external validation cohorts were 0.93 (95% CI, 0.89–0.96, p < 0.0001), 0.89 (95% CI, 0.82–0.94, p < 0.0001), and 0.90 (95% CI, 0.84–0.97, p < 0.0001), respectively, which indicated that our prediction model had excellent discriminability for the 6-month prognosis after primary DC and an ideal concordance in discrimination for both the training and validation cohorts. The calibration ability of the model is exhibited with bootstrap-corrected calibration plots (Figure 3), which show an optimal concordance between the predicted and actual probabilities of a 6-month unfavorable prognosis in both the training and validation cohorts.
Figure 2. ROC curves for the training, internal, and external validation cohorts, respectively. (A) Training cohorts (B) internal validation cohorts (C) external validation cohorts (AUC = 0.93 vs. 0.89 vs. 0.90).
Figure 3. Calibration plots of the nomogram for the training, internal, and external validation cohorts, respectively. (A) Training cohorts (B) internal validation cohorts (C) external validation cohorts.
Despite a lack of nationwide epidemiological statistics, a large number of deaths and disabilities caused by TBI have become a serious public health threat in China (28). However, the high mortality of primary DC and the economic burden of the long-term nursing of patients with severe disability or an irreversible vegetative status have led to endless problems for the patient's family and society (8, 29). Due to the lack of well-matched prediction models, currently, we must evaluate the prognostic risk of patients with primary DC and consider how to implement effective regimens to substantially ameliorate the prognosis beyond an initial phase, generally relying on personal experience. At present, only two mainstream prediction models have been published for the prognosis of patients with TBI: the IMPACT model and the CRASH model (25, 30). However, these two models do not specifically focus on TBI patients with DC. Consequently, we aimed to establish an early, accurate, objective, and convenient prediction model for severe TBI patients with primary DC based on clinical indicators after admission.
This study constructed a risk prediction nomogram, a clear tool representing the prediction model, which could be directly applied in the clinic. Before establishing the prediction model, we determined age, GCS score, operative blood loss, completely effaced basal cisterns, intraoperative hypotension, and APTT as significant predictors of an unfavorable 6-month prognosis in patients with TBI after primary DC.
In terms of the multivariate analysis of our study, the correlation between age ≥65 years old and poor prognosis was extremely strong (OR = 24.114), which indicated that elderly patients are more prone to an unfavorable prognosis than young patients. This conclusion is similar to that published in the literature. Paldor et al. (31) performed a retrospective chart review of patients with TBI older than 65 years old submitted to DC and found that <2% had a good outcome 6 months after discharge, while three-fourths of the elderly patients who died were in a vegetative state or were severely disabled. Barthélemy et al. (32) conducted a systematic review of the TBI-related literature between 2011 and 2015 and concluded that younger patients had significantly lower mortality and higher GOS scores than older patients after evaluating the 6-month and 1-year mortality and the GOS score at discharge, taking into account the risk of bias in the RCT. We believe that a substantial portion of elderly patients experience underlying diseases, which tend to deteriorate after DC and evolve into serious postoperative complications, ultimately affecting the prognosis (33). Additionally, neuroplasticity in aged individuals is inferior to that of younger individuals, as is the tolerability of some post-traumatic complications, such as ischemia, hypoxia, and shock. These factors may significantly reduce the probability of recovery from severe trauma in elderly patients even well-after the trauma.
In our study, the GCS score was one of the predictors of an unfavorable 6-month prognosis, especially when it was <5, and had a strong correlation (OR = 19.391) with the catastrophic outcome. A very low GCS score (3 or 4) often indicates that brain stem function is life-threateningly compromised and is generally accompanied by unfavorable imaging features, such as an effaced basal cistern, which was another predictor for an unfavorable 6-month prognosis in our study. Once the signs of constriction or occlusion of the cisterna pontis and/or cisterna ambiens appear on CT, the patient's intracranial pressure will eventually increase. An extremely high ICP will lead to a decrease in intracranial vascular compliance and a sharp reduction in cerebral blood flow. When the cerebral perfusion pressure is lower than 50 mmHg, regardless of the duration, critical consequences could develop (34). Even if these patients survive, it can be difficult for them to recover to a favorable condition. However, some studies have found that a lower GCS score does not necessarily lead to adverse outcomes, especially in pediatric patients. The unimaginable rehabilitation effect may be achieved by prompt decompression and the doctors' confidence (35).
The influence of blood loss on the prognosis of patients undergoing DC has been mentioned occasionally in the literature, indicating that it may primarily affect mortality in pediatric patients. In a study based on a series of children (<15 years old) undergoing DC, the authors found that blood loss was one of the paramount elements in determining prognosis; when the blood loss was >300 ml, the prognosis was significantly worse (36). Desgranges also observed similar results, where among pediatric patients, when the intraoperative blood loss was over 50% of the blood volume, coagulation indexes, such as the international normalized ratio (INR), were significantly higher than with less blood loss (37). In the present study, we observed that intraoperative blood loss was an independent predictor of prognosis in both adults and children. Since DC carries a high risk of bleeding, a large amount of intraoperative blood loss will worsen the hemodynamics during the perioperative period, resulting in a significant increase in the probability of a poor prognosis. Thus, we suggest that the control of intraoperative blood loss is of great importance in the prognosis of patients and thus is deserving of attention from every surgeon.
Some studies have attempted to describe the mechanism by which hypotension after TBI increases in-hospital mortality (38, 39). We observed that intraoperative hypotension was strongly correlated with an unfavorable 6-month prognosis in multivariate analysis (OR: 9.562, p = 0.001). We believe that this may be the result of the following: (1) some patients with severe craniocerebral injury were hypotensive before surgery due to blood loss, traumatic shock, and other reasons. If there is too much blood loss during DC, the patient's hypotension will gradually progress to intractable intraoperative hypotension; (2) the rapid release of intracranial pressure when the dura mater is opened, causing a sudden change in sympathetic tension and a sharp decrease in vascular compliance; (3) obstruction of vascular access, which may hinder the resuscitation of blood volume (40); and (4) hemodynamic instability, especially hypotension during the operation, leading to serious insufficient cerebral perfusion. Even if the patient survives, a series of secondary brain injuries can occur (37).
Some previous studies have reported that abnormal coagulation function is highly correlated with an unfavorable prognosis after TBI (23, 41). In the multivariate analysis of our study, among the various coagulation-related factors, only APTT was an independent predictor of an unfavorable 6-month prognosis (OR = 5.488, p = 0.012). APTT reflects the comprehensive activity of the endogenous coagulation pathway, which is related to the main coagulation factors, such as VII, XI, VIII, and IX. A prolonged APTT after trauma is caused by activation of the coagulation pathway and the consumption or dysfunction of plasma coagulation factors, which often indicates that the patient is in a hypercoagulable state after the initial period of injury (41). In view of the urgency requiring primary DC, it is necessary to rectify abnormal coagulation function as soon as possible with procedures such as fresh plasma infusion and administration of coagulation factors. This requires not only the attention of doctors but also the cooperation of all departments of the trauma center.
Based on the above factors, we constructed a risk prediction nomogram and validated it with internal and external datasets, demonstrating the practical applicability of the model. In this study, the internal and external validation datasets were used to assess the discriminability and calibration ability, respectively, of the model. Models are often internally validated with homologous data to demonstrate their repeatability, while external validation is used to examine the portability and generalization of the model (27). After importing the internal and external datasets, the AUC of the model remained above 0.85, while the nomogram-predicted probabilities fairly well-matched the observed probabilities, which indicated that the model for predicting the overall 6-month prognosis of patients with TBI after primary DC had a favorable goodness-of-fit, robustness, and predictive power. It is noteworthy that from the calibration plot for internal validation, the calibration curve deviates slightly downward from the reference line when the prediction probability is over 60%, which showed a slight risk overestimation. From the calibration plot of external validation, the performance of external validation is similar to that of internal validation, especially when the prediction probability is higher than 30% and lower than 80%, that is, also a minor overestimation of risk, and this bias should be taken into account when using the model.
However, in clinical practice, the model should be used as an “early warning signal” to remind doctors to pay attention to risk factors, especially controllable factors such as hypotension and abnormal coagulation function, to minimize the probability of an adverse prognosis. We should also note that the prediction model cannot replace the doctor's clinical judgment. For instance, controversy remains regarding whether certain high-risk patients (low GCS score with bilateral dilated pupils, for example) should be actively undergoing DC. We need to use the prediction model to assist the patient's family members in making decisions without violating the principles of treatment and medical ethics.
Several limitations of this investigation must be addressed. This is a single-center retrospective study, thus, there will be unavoidable selection bias. However, we strictly formulated the inclusion and exclusion criteria, which made the data we collected more homogeneous and truly reflective of the actual situation. Additionally, we will conduct a multicenter study in the future, which has been supported by another fund. Another limitation is that we did not include ICP data in the study. Given the large number of patients with TBI and the relative shortage of ICP equipment, our ICP monitor gives priority to patients undergoing secondary DC. ICP monitors were only used for a few patients with primary DC, and thus the associated data may have been significantly biased.
We constructed a prediction model for the 6-month unfavorable prognosis for patients with TBI undergoing primary DC based on early clinical indicators. The internal and external validation results of the prediction model were encouraging. The prediction model was displayed in the form of a nomogram, which was used to evaluate the prognosis of such patients early, accurately, objectively, and conveniently, and can help to enhance the early identification of high-risk patients.
The raw data supporting the conclusions of this article will be made available by the authors, without undue reservation.
The studies involving human participants were reviewed and approved by Clinical Research Ethics Committee of Ganzhou People's Hospital. Written informed consent to participate in this study was provided by the participants' legal guardian/next of kin.
RY designed this study. ZT, KH, MZo, MZh, and QH performed data collection. WW conducted the statistical analysis. ZT and QJ drafted the manuscript. All authors contributed to the article and approved the submitted version.
This study was funded by the Science and Technology Department of Jiangxi Province (No. 20203BBGL73174) and the Ganzhou Municipal Health Committee (No. 2022-2-039).
I really appreciate my teacher RY who in the past 12 years has always encouraged and helped me when I treat my patients so that I can overcome any difficulties over and over again.
The authors declare that the research was conducted in the absence of any commercial or financial relationships that could be construed as a potential conflict of interest.
All claims expressed in this article are solely those of the authors and do not necessarily represent those of their affiliated organizations, or those of the publisher, the editors and the reviewers. Any product that may be evaluated in this article, or claim that may be made by its manufacturer, is not guaranteed or endorsed by the publisher.
1. Carney N, Totten A, O'Reilly C, Ullman J, Hawryluk G, Bell M, et al. Guidelines for the management of severe traumatic brain injury, 4th edition. Neurosurgery. (2017) 80:6–15. doi: 10.1227/NEU.0000000000001432
2. Cooper D, Rosenfeld J, Murray L, Arabi Y, Davies A, D'Urso P, et al. Decompressive craniectomy in diffuse traumatic brain injury. N Engl J Med. (2011) 364:1493–502. doi: 10.1056/NEJMoa1102077
3. Hutchinson P, Kolias A, Timofeev I, Corteen E, Czosnyka M, Timothy J, et al. Trial of Decompressive Craniectomy for Traumatic Intracranial Hypertension. N Engl J Med. (2016) 375:1119–30. doi: 10.1056/NEJMoa1605215
4. Wettervik T, Lenell S, Nyholm L, Howells T, Lewén A, Enblad P. Decompressive craniectomy in traumatic brain injury: usage and clinical outcome in a single centre. Acta Neurochir. (2018) 160:229–37. doi: 10.1007/s00701-017-3418-3
5. Yang C, Zhang J, Zhu G, Guo H, Gao F, Wang B, et al. Effects of primary decompressive craniectomy on the outcomes of serious traumatic brain injury with mass lesions, and independent predictors of operation decision. World Neurosurg. (2021) 148:E396–405. doi: 10.1016/j.wneu.2020.12.158
6. Benveniste R, Cajigas I, Jagid J, Wu E. Intracranial hypertension after primary decompressive craniectomy for head trauma. World Neurosurg. (2022) 157:e351–6. doi: 10.1016/j.wneu.2021.10.088
7. Kramer A, Deis N, Ruddell S, Couillard P, Zygun D, Doig C, et al. Decompressive craniectomy in patients with traumatic brain injury: are the usual indications congruent with those evaluated in clinical trials? Neurocrit Care. (2016) 25:10–9. doi: 10.1007/s12028-015-0232-8
8. Tapper J, Skrifvars MB, Kivisaari R, Siironen J, Raj R. Primary decompressive craniectomy is associated with worse neurological outcome in patients with traumatic brain injury requiring acute surgery. Surg Neurol Int. (2017) 8:141. doi: 10.4103/sni.sni_453_16
9. Ahmadi S, Meier U, Lemcke J. Detailed long-term outcome analysis after decompressive craniectomy for severe traumatic brain injury. Brain Inj. (2010) 24:1539–49. doi: 10.3109/02699052.2010.523049
10. Park J, Park J, Kim S, Lim Y, You N, Ahn Y, et al. Outcomes of ultra-early decompressive craniectomy after severe traumatic brain injury-treatment outcomes after severe TBI. Korean J Neurotrauma. (2014) 10:112. doi: 10.13004/kjnt.2014.10.2.112
11. Abouhashem S, Albakry A, El-Atawy S, Mohamed F, Elgammal S, Khattab O. Prediction of early mortality after primary decompressive craniectomy in patients with severe traumatic brain injury. Egy J Neurosurg. (2021) 36:96. doi: 10.1186/s41984-020-00096-5
12. Tang Z, Yang K, Zhong M, Yang R, Zhang J, Jiang Q, et al. Predictors of 30-Day Mortality in Traumatic Brain-Injured Patients after Primary Decompressive Craniectomy. World Neurosurg. (2019). doi: 10.1016/j.wneu.2019.10.053
13. Manskow U, Sigurdardottir S, Røe C, Andelic N, Skandsen T, Damsgård E, et al. Factors affecting caregiver burden 1 year after severe traumatic brain injury: a prospective nationwide multicenter study. J Head Trauma Rehabil. (2015) 30:411–23. doi: 10.1097/HTR.0000000000000085
14. Yamal J, Hannay HJ, Gopinath S, Aisiku IP, Benoit JS, Robertson CS. Glasgow outcome scale measures and impact on analysis and results of a randomized clinical trial of severe traumatic brain injury. J Neurotraum. (2019) 36:2484–92. doi: 10.1089/neu.2018.5939
15. Amirjamshidi A, Eftekhar B, Abouzari M, Rashidi A. The relationship between Glasgow coma/outcome scores and abnormal CT scan findings in chronic subdural hematoma. Clin Neurol Neurosur. (2007) 109:152–7. doi: 10.1016/j.clineuro.2006.07.007
16. Baker S, O'Neill B, Haddon W, Long W. The injury severity score: a method for describing patients with multiple injuries and evaluating emergency care. J Trauma. (1974) 14:187–96. doi: 10.1097/00005373-197403000-00001
17. Zygun D, Steiner L, Johnston A, Hutchinson P, Al-Rawi P, Chatfield D, et al. Hyperglycemia and brain tissue pH after traumatic brain injury. Neurosurgery. 2004) 55:877–81. doi: 10.1227/01.NEU.0000137658.14906.E4
18. Juratli T, Zang B, Litz R, Sitoci K, Aschenbrenner U, Gottschlich B, et al. Early hemorrhagic progression of traumatic brain contusions: frequency, correlation with coagulation disorders, and patient outcome: a prospective study. J Neurotrauma. (2014) 31:1521–7. doi: 10.1089/neu.2013.3241
19. Manuel V, Martin S, Juan S, Fernando M, Frerk M, Thomas K, et al. Hypocalcemia as a prognostic factor in mortality and morbidity in moderate and severe traumatic brain injury. Asian J Neurosurg. (2015) 10:190–4. doi: 10.4103/1793-5482.161171
20. Solla D, Amorim R, Kolias AG, Hutchinson PJ, Paiva WS. Incremental prognostic value of coagulopathy in addition to the crash score in traumatic brain injury patients. Neurocrit Care. 2020) 5. doi: 10.1007/s12028-020-00991-7
21. White C, Griffith S, Caron J. Early progression of traumatic cerebral contusions: characterization and risk factors. J Trauma. (2009) 67:508–14. doi: 10.1097/TA.0b013e3181b2519f
22. Yuan F, Ding J, Chen H, Guo Y, Wang G, Gao W, et al. Predicting progressive hemorrhagic injury after traumatic brain injury: derivation and validation of a risk score based on admission characteristics. J Neurotrauma. (2012) 29:2137–42. doi: 10.1089/neu.2011.2233
23. Yuan Q, Sun Y, Wu X, Yu J, Li Z, Du Z, et al. Coagulopathy in traumatic brain injury and its correlation with progressive hemorrhagic injury: a systematic review and meta-analysis. J Neurotrauma. (2016) 33:1279–91. doi: 10.1089/neu.2015.4205
24. Tsai Y, Wu S, Hsieh T, Liu H, Huang C, Chou S, et al. Association of stress-induced hyperglycemia and diabetic hyperglycemia with mortality in patients with traumatic brain injury: analysis of a propensity score-matched population. Int J Env Res Pub He. (2020) 17:4266. doi: 10.3390/ijerph17124266
25. Steyerberg E, Mushkudiani N, Perel P, Butcher I, Lu J, McHugh G, et al. Predicting outcome after traumatic brain injury: development and international validation of prognostic scores based on admission characteristics. Plos Med. (2008) 5:e165. doi: 10.1371/journal.pmed.0050165
26. Harrell F. Evaluating the yield of medical tests. JAMA: J Am Med Assoc. (1982) 247:2543. doi: 10.1001/jama.1982.03320430047030
27. Alba A, Agoritsas T, Walsh M, Hanna S, Iorio A, Devereaux P, et al. Discrimination and calibration of clinical prediction models: users' guides to the medical literature. JAMA. (2017) 318:1377–84. doi: 10.1001/jama.2017.12126
28. Cheng P, Yin P, Ning P, Wang L, Cheng X, Liu Y, et al. Trends in traumatic brain injury mortality in China, 2006-2013: a population-based longitudinal study. Plos Med. (2017) 14:e1002332. doi: 10.1371/journal.pmed.1002332
29. GBD TBIA. Global, regional, and national burden of traumatic brain injury and spinal cord injury, 1990–2016: a systematic analysis for the global burden of disease study 2016. Lancet Neurol. (2019) 18:56–87. doi: 10.1016/S1474-4422(18)30415-0
30. Perel P, Arango M, Clayton T, Edwards P, Komolafe E, Poccock S, et al. Predicting outcome after traumatic brain injury: practical prognostic models based on large cohort of international patients. BMJ. (2008) 336:425–9. doi: 10.1136/bmj.39461.643438.25
31. Paldor I, Peso D, Sviri G. Decompressive craniectomy in elderly patients with traumatic brain injury. J Clin Neurosci. (2020) 78:30. doi: 10.1016/j.jocn.2020.03.030
32. Barthélemy EJ, Melis M, Gordon E, Ullman JS, Germano IM. Decompressive craniectomy for severe traumatic brain injury: a systematic review. World Neurosurg. (2016) 88:411–20. doi: 10.1016/j.wneu.2015.12.044
33. Ostermann R, Joestl J, Tiefenboeck T, Lang N, Platzer P, Hofbauer M. Risk factors predicting prognosis and outcome of elderly patients with isolated traumatic brain injury. J Orthop Surg Res. (2018) 13:277. doi: 10.1186/s13018-018-0975-y
34. Güiza F, Depreitere B, Piper I, Citerio G, Chambers I, Jones P, et al. Visualizing the pressure and time burden of intracranial hypertension in adult and pediatric traumatic brain injury. Intensive Care Med. (2015) 41:1067–76. doi: 10.1007/s00134-015-3806-1
35. Thomale U, Graetz D, Vajkoczy P, Sarrafzadeh A. Severe traumatic brain injury in children–a single center experience regarding therapy and long-term outcome. Childs Nerv Syst. (2010) 26:1563–73. doi: 10.1007/s00381-010-1103-4
36. Khan S, Shallwani H, Shamim M, Murtaza G, Enam S, Qureshi R, et al. Predictors of poor outcome of decompressive craniectomy in pediatric patients with severe traumatic brain injury: a retrospective single center study from Pakistan. Childs Nerv Syst. (2014) 30:277–81. doi: 10.1007/s00381-013-2225-2
37. Desgranges F, Javouhey E, Mottolese C, Migeon A, Szathmari A, Baudin F, et al. Intraoperative blood loss during a decompressive craniectomy for refractory intracranial hypertension after traumatic brain injury in children. Child's nervous system. ChNS: Off J Int Soc Ped Neurosurg. (2014) 33:1393–8. doi: 10.1007/s00381-014-2417-4
38. Zebrack M, Dandoy C, Hansen K, Scaife E, Mann N, Bratton S. Early resuscitation of children with moderate-to-severe traumatic brain injury. Pediatrics. (2009) 124:56–64. doi: 10.1542/peds.2008-1006
39. Spaite D, Hu C, Bobrow B, Chikani V, Barnhart B, Gaither J, et al. Association of out-of-hospital hypotension depth and duration with traumatic brain injury mortality. Ann Emerg Med. (2017) 70:522–30. doi: 10.1016/j.annemergmed.2017.03.027
40. Miller P, Mack C, Sammer M, Rozet I, Lee L, Muangman S, et al. The incidence and risk factors for hypotension during emergent decompressive craniotomy in children with traumatic brain injury. Anesth Analg. (2006) 103:869–75. doi: 10.1213/01.ane.0000237327.12205.dc
Keywords: traumatic brain injury, decompressive craniectomy, nomogram, validation, prognosis
Citation: Tang Z, Hu K, Yang R, Zou M, Zhong M, Huang Q, Wei W and Jiang Q (2022) Development and validation of a prediction nomogram for a 6-month unfavorable prognosis in traumatic brain-injured patients undergoing primary decompressive craniectomy: An observational study. Front. Neurol. 13:944608. doi: 10.3389/fneur.2022.944608
Received: 15 May 2022; Accepted: 12 July 2022;
Published: 03 August 2022.
Edited by:
Renato Anghinah, University of São Paulo, BrazilReviewed by:
Ana Cristina Veiga Silva, Hospital da Restauração, BrazilCopyright © 2022 Tang, Hu, Yang, Zou, Zhong, Huang, Wei and Jiang. This is an open-access article distributed under the terms of the Creative Commons Attribution License (CC BY). The use, distribution or reproduction in other forums is permitted, provided the original author(s) and the copyright owner(s) are credited and that the original publication in this journal is cited, in accordance with accepted academic practice. No use, distribution or reproduction is permitted which does not comply with these terms.
*Correspondence: Qiuhua Jiang, amlhbmdxaDE5NjhAMTI2LmNvbQ==
†These authors have contributed equally to this work
Disclaimer: All claims expressed in this article are solely those of the authors and do not necessarily represent those of their affiliated organizations, or those of the publisher, the editors and the reviewers. Any product that may be evaluated in this article or claim that may be made by its manufacturer is not guaranteed or endorsed by the publisher.
Research integrity at Frontiers
Learn more about the work of our research integrity team to safeguard the quality of each article we publish.