- 1The First Clinical Medical College of Gansu University of Chinese Medicine (Gansu Provincial Hospital), Lanzhou, China
- 2The Department of Neurology, Gansu Provincial Hospital, Lanzhou, China
Background: Cognitive dysfunction in cerebral small vessel disease (CSVD) is a common cause of vascular dementia. The purpose of this study was to find independent risk factors for the development of cognitive dysfunction in patients with CSVD and establish a risk prediction model, in order to provide a reference for clinical diagnosis and treatment of such patients.
Methods: In this study, clinical data of patients with CSVD admitted to the Department of Neurology in Gansu Provincial Hospital from December 2019 to December 2021 were collected, and 159 patients were finally included after strict screening according to the inclusion and exclusion criteria. There were 43 patients with normal function and 116 patients with cerebral small vessel disease cognitive impairment (CSVDCI). The logistic multivariable regression model was used to screen out the independent risk factors of cognitive dysfunction in patients with CSVD, and the nomogram of cognitive dysfunction in patients with CSVD was constructed based on the results of the logistic multivariable regression analysis. Finally, the accuracy of the prediction model was evaluated by C-index, calibration curve, receiver operating characteristic (ROC) curve, and decision curve analysis (DCA).
Results: The results of multivariable logistic regression analysis showed that hypertension (OR = 2.683, 95% CI 1.119–6.432, P = 0.027), homocysteine (Hcy) (OR = 1.083, 95% CI 1.026–1.143, P = 0.004), total CSVD MRI Score (OR = 1.593, 95% CI 1.025–2.475, P = 0.039) and years of schooling (OR = 0.883, 95% CI 0.798–0.978, P = 0.017) were independent risk factors for the development of cognitive dysfunction in patients with CSVD. The C-index of this prediction model was 0.806 (95% CI 0.735–0.877), and the calibration curve, ROC curve, and DCA curve all showed good predictive power in the nomogram.
Conclusions: The nomogram constructed in this study has high accuracy and clinical utility in predicting the occurrence of cognitive dysfunction in patients with CSVD. For patients with CSVD with the above risk factors, active clinical intervention and prevention are required during clinical consultation and disease management to avoid cognitive impairment as much as possible.
Introduction
Vascular cognitive impairment is one of the common causes of dementia in patients, of which cognitive dysfunction in CSVD accounts for a large proportion (1, 2). CSVD is a group of pathological processes affecting the penetrating arteries, microarteries, capillaries, small veins, and microvenules of the brain due to one or more etiologies, which in turn leads to brain parenchymal damage, and approximately 45% of cognitive decline and overall life loss (3–7). It has a great impact on the quality of life of patients. According to the etiology, CSVD includes six major categories, (5) including type 1:arteriolosclerosis (or age-related and vascular risk-factor-related small vessel diseases), type 2: sporadic and hereditary cerebral amyloid angiopathy, type 3: inherited or genetic small vessel diseases distinct from cerebral amyloid angiopathy, type 4: inflammatory and immunologically mediated small vessel diseases, type 5: venous collagenosis, and type 6: other small vessel diseases. Among them, type 1 and type 2 (6) are the most common types, and this study focuses on type 1 (3).
At present, the pathogenesis of the disease is still unclear, and some studies have found that age, living habits, and risk factors related to cerebrovascular disease may affect the cognitive dysfunction of patients with CSVD (3, 8–10). However, the results of risk factors were not consistent, and there was no nomogram for establishing predictive models based on relevant risk factors. This study expects to enrich the necessary findings. As a new type of statistical prediction model, nomogram can convert risk factors into a graph of a continuous scoring system. Compared with traditional predictive analysis models, nomogram has many advantages such as high accuracy, flexibility in use, and ease of generalization (11–13). Nomograms can be used clinically to predict risk, to help physicians make personalized treatment decisions and to be more easily understood and cooperated with by patients in doctor-patient communication, and are now used in a variety of clinical studies. This study aimed to find possible risk factors for the development of cognitive dysfunction in CSVD patients and to construct a predictive model for the visual presentation of the nomogram, in order to provide a reference for clinicians in the assessment, disease management, and clinical interventions for patients with CSVD.
Methods
Patients and selection criteria
A total of 159 patients with CSVD admitted to the Department of Neurology in Gansu Provincial Hospital from December 2019 to December 2021 were selected, and the clinical data of these patients were statistically analyzed. Among them, 43 patients with normal cognitive function in CSVD and 116 patients with CSVDCI. The inclusion criteria were as follows: (1) the diagnoses of the patients were all in accordance with the Chinese Consensus on the Diagnosis and Management of Cerebral Small Vessel Disease (14); (2) cranial magnetic resonance imaging (MRI) examination showed one or more of the following imaging features: lacunar infarction (LI), white matter hyperintensity (WMH), enlarged perivascular space (ePVS), and cerebral microbleeds (CMBs); (3) age ≥ 50 years; (4) only patients with small atherosclerotic CSVD were included (15); (5) no history of stroke or dementia; (6) not taking any medications that affect cognitive function, such as anticholinergics, sedative-hypnotics, antihistamines, etc.; (7) patients and their families agreed and voluntarily joined this study. The exclusion criteria were as follows: (1) patients with contraindications to MRI examination; (2) Imaging findings of intracranial occupying lesions such as brain tumors, hydrocephalus, or non-cerebral small vessel white matter lesions (e.g., multiple sclerosis, tuberous disease, brain radiation therapy); (3) patients with other central nervous system lesions or severe medical diseases; (4) patients with a history of depression and bipolar disorder; (5) patients with a history of COVID-19; (6) patients with congenital mental retardation and dementia caused by other diseases (such as severe mental illness, epilepsy, alcohol and drug abuse, poisoning, etc.).
Study variables
Patients' age, sex, ethnicity, BMI, years of schooling, smoking (defined as smoking ≥1 cigarette per day on average and smoking history ≥1 year), drinking (defined as drinking ≥50 ml of liquor on average per day, ≥1 time per week on average and drinking history ≥1year), hypertension, coronary heart disease, diabetes, systolic blood pressure (in the early morning of the second day of admission to the hospital), triglycerides (TG), total cholesterol (TC), low-density lipoprotein (LDL), homocysteine (Hcy), C-reactive protein (CRP), blood urea nitrogen (BUN), creatinine (Cr), and carotid atheromatous plaque were collected as clinical factors.
Cognitive function assessment
Cognitive assessment was based on the MOCA scale (16, 17), which includes eight cognitive domains including abstract thinking, attention and concentration, executive function, language, memory, visual structure skills, computation, and orientation. A MOCA total score of 26–30 indicates normal cognitive function, and a total score of <26 indicates cognitive dysfunction. If the education years ≤ 12, 1 point will be added.
The total CSVD MRI score
MRI images were blindly reviewed by two experienced neurologists with reference to the STRIVE criteria (18), and a total CSVD MRI score (8) was used to reflect CSVD severity: 1 point for LI ≥1; 1 point for CMBs ≥1; 1 point for moderate to severe ePVS (grade 2–4); Fazekas graded 3 points for periventricular white matter hyperintensity or ≥2 points for deep brain white matter hyperintensity, 1 point. The total score is 0–4 points, and the degree is graded: mild (0–1 points), moderate to severe (2–4 points). The scoring criteria for WMH, LI, CMBs, and ePVS are shown in Table 1.
Statistical analysis
Data from this study were statistically analyzed using SPSS 26.0 and R software (version 4.1.2, https://www.r-project.org/). Numeric variables are expressed as χ ± s, and independent samples t-test was used when both samples were from normal overall and the overall variance was equal; count data are expressed as absolute values or rates. Categorical variables were analyzed using the χ2 test (Pearson chi-square, Continuous correction chi-squared test, Fisher's exact test), possible risk factors were analyzed using logistic univariate regression analysis, and clinical factors with differential results (P < 0.05) from logistic univariate regression analysis were further included in the logistic multivariable regression analysis. Logistic multivariable regression models were analyzed using stepwise regression (Forward: LR) to screen for independent risk factors and establish regression equations. Based on the screened independent risk factors, a nomogram of the prediction model was constructed using the R software package “rms.” The receiver operating characteristic (ROC) curve of the prediction model was plotted, and the discriminatory ability of the model was evaluated by the area under the curve (AUC). The accuracy of the model was evaluated by calculating the consistency index (C-index) and plotting the calibration curve. The AUC values and C-index are between 0.50–0.70 for low accuracy, between 0.71–0.90 for moderate accuracy, and above 0.90 for high accuracy. In addition, a decision curve analysis (DCA) of the model was developed using the “ggDCA” package to quantify the net benefit rate at different threshold probabilities to assess the clinical validity of the model. P < 0.05 was considered statistically different, and confidence intervals for the parameters were estimated at 95% confidence intervals.
Results
Comparison of baseline information between the two groups of patients
A total of 159 CSVD patients were included after strict screening according to the inclusion and exclusion criteria. Among them, 43 patients with normal cerebral small vessel disease cognitive function (CSVD) and 116 patients with cerebral small vessel disease cognitive function impairment (CSVDCI). There are 77 males and 82 females; 152 cases were Han Chinese and 7 cases were Hui or Dongxiang, and the patients' ages ranged from 50 to 88 years. The baseline information is shown in Table 2.
Logistic univariate regression analysis results
The univariate logistic analysis of all clinical factors showed that age, systolic blood pressure, years of schooling, hypertension, TG, BUN, Hcy, CRP, total CSVD MRI Score, and carotid atheromatous plaque were factors associated with the development of cognitive dysfunction in patients with CSVD (P < 0.05). In contrast, sex, ethnicity, smoking, drinking, coronary heart disease, diabetes, BMI, LDL, Cr, and TC were not factors associated with the development of cognitive dysfunction in patients with CSVD (P ≥ 0.05). The results are shown in Table 3.
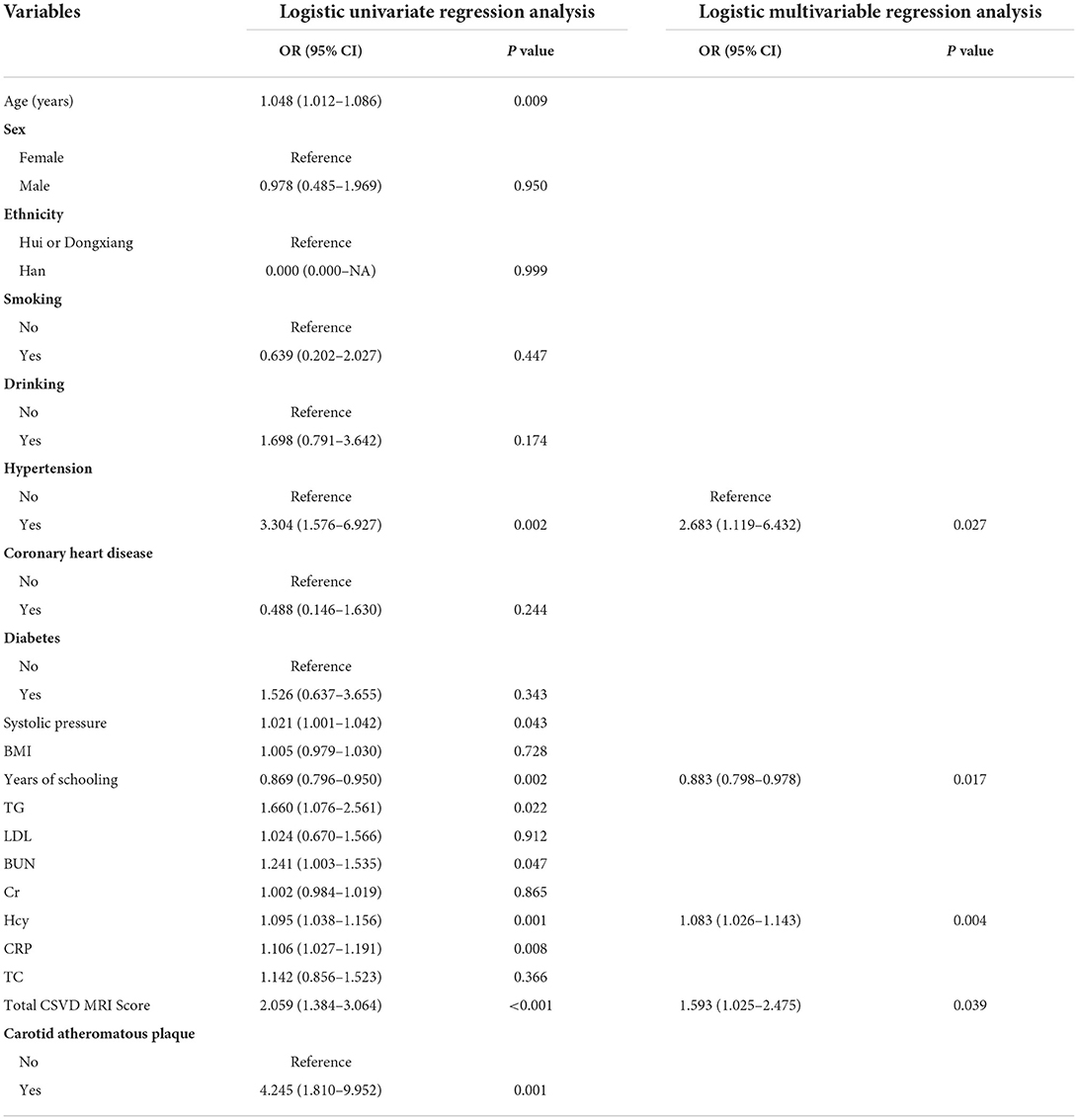
Table 3. Logistic univariate and multivariable regression analysis of cognitive impairment in patients with CSVD.
Logistic multivariable regression analysis results
The clinical factors with significant (P < 0.05) univariate analysis results were further included in the logistic multivariable regression analysis, and the results showed that hypertension, years of schooling, Hcy, and total CSVD MRI Score were independent risk factors for the development of cognitive dysfunction in patients with CSVD (P < 0.05). Among them, high years of schooling was a protective factor, and hypertension, high Hcy, and high total CSVD MRI Sorce were risk factors. The results are shown in Table 3.
Construction of the nomogram
In this study, based on the results of logistic multivariable regression analysis, four independent risk factors of cognitive impairment in CSVD patients were incorporated into the nomogram, and a nomogram was established to predict the risk of cognitive impairment in CSVD patients. For each patient, the total score was obtained by summing the scores of the corresponding variables, and the predicted probability of cognitive dysfunction for that patient was finally calculated by converting the relationship between the total score and the probability of occurrence of the outcome event as a function of the total score (Figure 1).
Internal validation of nomogram and model performance
In this study, the Bootstrap validation method was used to plot the calibration curve of the prediction model, the 45° line was used as the optimal model, and the results showed that the prediction results of the nomogram were in good agreement with the actual results, as shown in Figure 2. The C-index is 0.806 (95% CI 0.735–0.877), indicating that the model has good discriminatory ability and accuracy.
In this study, four independent factors and the nomogram were used as measures in the ROC curve analysis (Figure 3). The results showed that the nomogram AUC value was 0.806, which was the largest, and the AUCs for hypertension, Hcy, total CSVD MRI Sorce, and years of schooling were 0.632, 0.712, 0.697, and 0.659, respectively, indicating that the predictive power of the nomogram is better than that of individual factors.
In the DCA curve of the nomogram, the blue dotted line indicates a benefit rate of 0 assuming that all CSVD patients do not develop cognitive impairment and are left untreated; The green dotted line indicates that all CSVD patients developed cognitive impairment and all received treatment, with the net benefit being a backslash line with a negative slope. Within the threshold probability range of the DCA curves, patients had a higher net benefit than the other two extreme curves. The cut-off value obtained by ROC curve analysis of the nomogram (76.60%) was within the threshold probability range of the DCA curve, indicating the clinical validity of the model. Therefore, setting 76.60% as the threshold probability value for diagnosing cognitive dysfunction in patients with CSVD and treating patients with clinical interventions showed a good net benefit (Figure 4).
Discussion
Cerebral small vessel disease (CSVD) is a common age-related cerebrovascular disease, and its pathological changes may be related to slowly accumulating tissue damage, which is one of the main causes of cognitive decline in the elderly (19). At present, the pathogenesis of cognitive dysfunction in CSVD remains unclear. It has been suggested that cognitive dysfunction in CSVD patients may be related to atherosclerosis and microvascular dysfunction (20, 21), which may affect cerebral blood perfusion, neurogenesis, and brain self-regulation, resulting in neurological dysfunction and cognitive dysfunction in CSVD patients (20–22). The purpose of this study was to investigate the independent risk factors for the development of cognitive dysfunction in patients with CSVD, with the aim of providing a reference for clinical diagnosis and treatment.
Hypertension is one of the independent risk factors for the development of cognitive dysfunction in patients with CSVD (23, 24), and the results of our study found that hypertension is an independent risk factor for the development of cognitive dysfunction in patients with CSVD. A prospective study showed that patients with CSVD combined with hypertension had a 1.5-fold increased risk of cognitive dysfunction compared with patients with normal blood pressure (25), and the result is the same as the results of most studies. Patients with CSVD combined with hypertension are prone to cognitive impairment probably because hypertension causes a series of oxidative stress and inflammatory processes, resulting in changes in neural, vascular and endothelial functions, and accelerates the process of pathological changes in CSVD, such as white matter hyper-signal, lacunar cerebral infarction, peripheral vascular gap enlargement, and cerebral microhemorrhage (23, 24, 26).
The total CSVD MRI score is a combination of four different MRI markers to indicate the degree of brain damage in CSVD patients (27). The results of our study found that the total CSVD MRI score was an independent risk factor for the development of cognitive dysfunction in patients with CSVD, and the total CSVD MRI score was positively associated with the development of cognitive dysfunction in patients with CSVD. When performing cognitive function tests, the heavier the total CSVD MRI load, the lower the score obtained by the patient. A prospective study by Jiang Y et al. (28) also showed that the total CSVD MRI load at the beginning of the study for participants included in the study was strongly associated with a decrease in MMSE scores at the end of follow-up, and that patients were at higher risk of developing dementia when more than three CSVD imaging markers were present at the beginning of the study. In addition, Li X et al. (29) showed that the total CSVD MRI score was an independent risk factor for cognitive dysfunction in the overall cognitive domain and more than three cognitive domains, and the results of our study are consistent with the findings of all these studies. The effect of The total CSVD MRI score on cognitive function may be due to its corresponding pathological changes that damage the structural and functional brain networks of patients (30), which in turn affects the functional integration capacity of structural brain networks and leads to cognitive dysfunction in CSVD patients (31).
Hcy is a sulfur-containing amino acid produced during methionine metabolism, which generates oxygen radicals with strong oxidative effects on vascular endothelial cells and is defined as a reactive vascular damage amino acid (32). High Hcy can cause endothelial dysfunction in the body and plays an important role in the pathogenesis of neurological disorders such as stroke and cognitive impairment. The results of our study found that high Hcy was an independent risk factor for the development of cognitive dysfunction in patients with CSVD, and previous studies have also shown that elevated Hcy is significantly associated with the development of cognitive dysfunction in patients (33). When the organism's Hcy is elevated, its induced oxidative stress, endothelial dysfunction, inflammation, and endoplasmic reticulum (ER) stress cause neurovascular dysfunction (34), which in turn puts CSVD patients at increased risk of cognitive dysfunction.
Years of schooling were considered to be significantly associated with the occurrence of cognitive dysfunction in patients with CSVD, and the results of our study showed that years of schooling were a protective factor against the occurrence of cognitive dysfunction in patients with CSVD, and the higher the level of schooling in patients with CSVD, the slower the decline in cognitive function. Most studies have shown that the higher the level of schooling of CSVD patients, the lower the odds of cognitive dysfunction (35, 36). Thus, low years of education are a risk factor for the development of cognitive dysfunction in patients with CSVD, and changes in cognitive function over time in patients are influenced by years of schooling (37, 38). In addition, higher levels of schooling are associated with greater neural reserve capacity formed in brain networks, which in turn promotes the development of compensatory neural circuits, ultimately improving resistance to inflammatory brain damage and delaying the onset of cognitive dysfunction (39, 40).
This study constructed a nomogram of predictive models based on four independent risk factors in patients with CSVD. Previous studies have performed nomogram construction based on CSVD patients, but no study has done a nomogram on the occurrence of cognitive dysfunction in patients with CSVD. In addition, the accuracy of the model was evaluated using calibration curves, ROC curves, and DCA curves in this study, and the results showed that the model has good accuracy and discriminatory ability, and has clinical validity, which can provide some reference for disease management of CSVD patients. However, our study also has some limitations. First, this study is a single-center retrospective study with limited sample size, and the results may have some inevitable biases. Second, this study did not conduct a stratified study on patients with different cognitive domains, and further research is needed to further explore. Third, the patients included in this study were those admitted to the hospital for treatment, and it is possible that the results of the study are not applicable to outpatients. Finally, the results of this study need to be further analyzed and validated by multicenter studies with larger sample sizes and prospective studies, to find more clinical predictors of cognitive dysfunction in CSVD patients and to provide greater clinical reference value for clinical intervention and delay the process of cognitive dysfunction in CSVD patients.
Conclusion
In conclusion, hypertension, Hcy, total CSVD MRI Score, and years of schooling are independent risk factors affecting the development of cognitive dysfunction in patients with CSVD. Early clinical interventions targeting relevant independent risk factors are particularly important in the assessment and management of patients' conditions. Whether high years of schooling as a protective factor can be considered to delay cognitive decline by providing patients with relevant learning and memory exercises deserves further practice and demonstration by clinicians. Finally, it is expected that this study will provide some reference for clinicians in the assessment of CSVD patients' conditions and the development of individualized disease management strategies.
Data availability statement
The raw data supporting the conclusions of this article will be made available by the authors, without undue reservation.
Ethics statement
The studies involving human participants were reviewed and approved by the Medical Ethics Committee of Gansu Provincial Hospital. The patients/participants provided their written informed consent to participate in this study. Written informed consent was obtained from the individual(s) for the publication of any potentially identifiable images or data included in this article.
Author contributions
LZ and FG contributed equally to this article, completed the study design, statistical analysis, and drafted the manuscript. YH and QS collect patient data from Hospital's Electronic Medical Record System. QZ and PH perform MoCA scale measurements for patients. YZ and YY performed imaging assessment and helped to embellish language. YZ conceived of the study and provided expert consultations and suggestions. All authors contributed to the study and approved the submitted version.
Funding
This work was supported by the Gansu Province Health Industry Scientific Research Project (GSWSKY2020-03) and the Natural Science Foundation of Gansu Province (20JR5RA152).
Acknowledgments
The authors thank all clinical patients who participated in this study.
Conflict of interest
The authors declare that the research was conducted in the absence of any commercial or financial relationships that could be construed as a potential conflict of interest.
Publisher's note
All claims expressed in this article are solely those of the authors and do not necessarily represent those of their affiliated organizations, or those of the publisher, the editors and the reviewers. Any product that may be evaluated in this article, or claim that may be made by its manufacturer, is not guaranteed or endorsed by the publisher.
References
1. Rundek T, Tolea M, Ariko T, Fagerli EA, Camargo CJ. Vascular Cognitive Impairment (VCI). Neurotherapeutics. (2021) 19:68–88. doi: 10.1007/s13311-021-01170-y
2. Li S, Shao Y, Li K, HuangFu C, Wang W, Liu Z, et al. Vascular cognitive impairment and the gut microbiota. J Alzheimers Dis. (2018) 63:1209–22. doi: 10.3233/JAD-171103
3. Cannistraro RJ, Badi M, Eidelman BH, Dickson DW, Middlebrooks EH, Meschia JF, et al. Small vessel disease: a clinical review. Neurology. (2019) 92:1146–56. doi: 10.1212/WNL.0000000000007654
4. Chen X, Wang J, Shan Y, Cai W, Liu S, Hu M, et al. Cerebral small vessel disease: neuroimaging markers and clinical implication. J Neurol. (2019) 266:2347–62. doi: 10.1007/s00415-018-9077-3
5. Pantoni L. Cerebral small vessel disease: from pathogenesis and clinical characteristics to therapeutic challenges. Lancet Neurol. (2010) 9:689–701. doi: 10.1016/S1474-4422(10)70104-6
6. Zanon Zotin MC, Sveikata L, Viswanathan A, Yilmaz P. Cerebral small vessel disease and vascular cognitive impairment: from diagnosis to management. Curr Opin Neurol. (2021) 34:246–57. doi: 10.1097/WCO.0000000000000913
7. Shi Y, Wardlaw JM. Update on cerebral small vessel disease: a dynamic whole-brain disease. Stroke Vasc Neurol. (2016) 1:83–92. doi: 10.1136/svn-2016-000035
8. Staals J, Makin SD, Doubal FN, Dennis MS, Wardlaw JM. Stroke subtype, vascular risk factors, and total MRI brain small-vessel disease burden. Neurology. (2014) 83:1228–34. doi: 10.1212/WNL.0000000000000837
9. Szcześniak D, Rymaszewska J, Zimny A, Sasiadek M, Połtyn-Zaradna K, Smith EE, et al. “Cerebral small vessel disease and other influential factors of cognitive impairment in the middle-aged: a long-term observational cohort PURE-MIND study in Poland”. Geroscience. (2021) 43:279–95. doi: 10.1007/s11357-020-00271-4
10. Evans LE, Taylor JL, Smith CJ, Pritchard HAT, Greenstein AS, Allan SM. Cardiovascular comorbidities, inflammation, and cerebral small vessel disease. Cardiovasc Res. (2021) 117:2575–88. doi: 10.1093/cvr/cvab284
11. Gong P, Zhang X, Gong Y, Liu Y, Wang S, Li Z, et al. A novel nomogram to predict early neurological deterioration in patients with acute ischaemic stroke. Eur J Neurol. (2020) 27:1996–2005. doi: 10.1111/ene.14333
12. Gittleman H, Sloan AE, Barnholtz-Sloan JS. An independently validated survival nomogram for lower-grade glioma. Neuro Oncol. (2020) 22:665–74. doi: 10.1093/neuonc/noz191
13. Gittleman H, Lim D, Kattan MW, Chakravarti A, Gilbert MR, Lassman AB, et al. An independently validated nomogram for individualized estimation of survival among patients with newly diagnosed glioblastoma: NRG oncology RTOG 0525 and 0825. Neuro Oncol. (2017) 19:669–77. doi: 10.1093/neuonc/now208
14. Chinese Chinese Society of Neurology Division Division of Cerebrovascular Diseases Chinese Chinese Society of Neurology. Chinese consensus on the diagnosis and treatment of cerebral small vessel disease. Chin J Geriatr. (2015) 48:838–44. doi: 10.3760/cma.j.issn.1006-7876.2015.10.004
15. Geriatric Neurology Group Chinese Chinese Society of Geriatrics Clinical Clinical Practice Guideline for Cognitive Impairment of Cerebral Small Vessel Disease Writing Group. Clinical practice guideline for cognitive impairment of cerebral small vessel disease of China (2019). Chin J Geriatr. (2019) 38:345–54. doi: 10.3760/cma.j.issn.0254-9026.2019.04.001
16. Chen KL, Xu Y, Chu AQ, Ding D, Liang XN, Nasreddine ZS, et al. Validation of the Chinese version of Montreal cognitive assessment basic for screening mild cognitive impairment. J Am Geriatr Soc. (2016) 64:e285–90. doi: 10.1111/jgs.14530
17. Julayanont P, Tangwongchai S, Hemrungrojn S, Tunvirachaisakul C, Phanthumchinda K, Hongsawat J, et al. The Montreal cognitive assessment-basic: a screening tool for mild cognitive impairment in illiterate and low-educated elderly adults. J Am Geriatr Soc. (2015) 63:2550–4. doi: 10.1111/jgs.13820
18. Wardlaw JM, Smith EE, Biessels GJ, Cordonnier C, Fazekas F, Frayne R, et al. Neuroimaging standards for research into small vessel disease and its contribution to ageing and neurodegeneration. Lancet Neurol. (2013) 12:822–38. doi: 10.1016/S1474-4422(13)70124-8
19. Pinter D, Enzinger C, Fazekas F. Cerebral small vessel disease, cognitive reserve and cognitive dysfunction. J Neurol. (2015) 262:2411–9. doi: 10.1007/s00415-015-7776-6
20. Rensma SP, Stehouwer CDA, Van Boxtel MPJ, Houben AJHM, Berendschot TTJM, Jansen JFA, et al. Associations of arterial stiffness with cognitive performance, and the role of microvascular dysfunction: the Maastricht study. Hypertension. (2020) 75:1607–14. doi: 10.1161/HYPERTENSIONAHA.119.14307
21. Climie RE, van Sloten TT, Bruno RM, Taddei S, Empana JP, Stehouwer CDA, et al. Macrovasculature and microvasculature at the crossroads between type 2 diabetes mellitus and hypertension. Hypertension. (2019) 73:1138–49. doi: 10.1161/HYPERTENSIONAHA.118.11769
22. Rensma SP, van Sloten TT, Houben AJHM, Köhler S, van Boxtel MPJ, Berendschot TTJM, et al. Microvascular dysfunction is associated with worse cognitive performance: the Maastricht study. Hypertension. (2020) 75:237–45. doi: 10.1161/HYPERTENSIONAHA.119.13023
23. Iadecola C, Gottesman RF. Neurovascular and cognitive dysfunction in hypertension. Circ Res. (2019) 124:1025–44. doi: 10.1161/CIRCRESAHA.118.313260
24. Jiménez-Balado J, Riba-Llena I, Abril O, Garde E, Penalba A, Ostos E, et al. Cognitive impact of cerebral small vessel disease changes in patients with hypertension. Hypertension. (2019) 73:342–9. doi: 10.1161/HYPERTENSIONAHA.118.12090
25. Liu Y, Dong YH, Lyu PY, Chen WH, Li R. Hypertension-induced cerebral small vessel disease leading to cognitive impairment. Chin Med J. (2018) 131:615–9. doi: 10.4103/0366-6999.226069
26. Gottesman RF, Albert MS, Alonso A, Coker LH, Coresh J, Davis SM, et al. Associations between midlife vascular risk factors and 25-year incident dementia in the Atherosclerosis Risk in Communities (ARIC) cohort. JAMA Neurol. (2017) 74:1246–54. doi: 10.1001/jamaneurol.2017.1658
27. Pavlovic AM, Pekmezovic T, Tomic G, Trajkovic JZ, Sternic N. Baseline predictors of cognitive decline in patients with cerebral small vessel disease. J Alzheimers Dis. (2014) 42:S37–43. doi: 10.3233/JAD-132606
28. Jiang Y, Wang Y, Yuan Z, Xu K, Zhang K, Zhu Z, et al. Total cerebral small vessel disease burden is related to worse performance on the mini-mental state examination and incident dementia: a prospective 5-year follow-up. J Alzheimers Dis. (2019) 69:253–62. doi: 10.3233/JAD-181135
29. Li X, Yuan J, Qin W, Yang L, Yang S, Li Y, et al. Higher total cerebral small vessel disease burden was associated with mild cognitive impairment and overall cognitive dysfunction: a propensity score-matched case-control study. Front Aging Neurosci. (2021) 13:695732. doi: 10.3389/fnagi.2021.695732
30. Ter Telgte A, van Leijsen EMC, Wiegertjes K, Klijn CJM, Tuladhar AM, de Leeuw FE. Cerebral small vessel disease: from a focal to a global perspective. Nat Rev Neurol. (2018) 14:387–98. doi: 10.1038/s41582-018-0014-y
31. Xu X, Lau KK, Wong YK, Mak HKF, Hui ES. The effect of the total small vessel disease burden on the structural brain network. Sci Rep. (2018) 8:7442. doi: 10.1038/s41598-018-25917-4
32. Cui L, Lu P, Li S, Pan Y, Wang M, Li Z, et al. Relationship among homocysteine, inflammation and cognitive impairment in patients with acute ischemic stroke and transient ischemic attack. Neuropsychiatr Dis Treat. (2021) 17:3607–16. doi: 10.2147/NDT.S333753
33. Hainsworth AH, Yeo NE, Weekman EM, Wilcock DM. Homocysteine, hyperhomocysteinemia and vascular contributions to cognitive impairment and dementia (VCID). Biochim Biophys Acta. (2016) 1862:1008–17. doi: 10.1016/j.bbadis.2015.11.015
34. Moretti R, Caruso P. The controversial role of homocysteine in neurology: from labs to clinical practice. Int J Mol Sci. (2019) 20:231. doi: 10.3390/ijms20010231
35. Clouston SAP, Smith DM, Mukherjee S, Zhang Y, Hou W, Link BG, et al. Education and cognitive decline: an integrative analysis of global longitudinal studies of cognitive aging. J Gerontol B Psychol Sci Soc Sci. (2020) 75:e151–60. doi: 10.1093/geronb/gbz053
36. Yahirun JJ, Vasireddy S, Hayward MD. The education of multiple family members and the life-course pathways to cognitive impairment. J Gerontol B Psychol Sci Soc Sci. (2020) 75:e113–28. doi: 10.1093/geronb/gbaa039
37. Robitaille A, van den Hout A, Machado RJM, Bennett DA, Cukić I, Deary IJ, et al. Transitions across cognitive states and death among older adults in relation to education: a multistate survival model using data from six longitudinal studies. Alzheimers Dement. (2018) 14:462–72. doi: 10.1016/j.jalz.2017.10.003
38. Hamilton OKL, Backhouse EV, Janssen E, Jochems ACC, Maher C, Ritakari TE, et al. Cognitive impairment in sporadic cerebral small vessel disease: a systematic review and meta-analysis. Alzheimers Dement. (2021) 17:665–85. doi: 10.1002/alz.12221
39. Meng X, D'Arcy C. Education and dementia in the context of the cognitive reserve hypothesis: a systematic review with meta-analyses and qualitative analyses. PLoS ONE. (2012) 7:e38268. doi: 10.1371/journal.pone.0038268
Keywords: cerebral small vessel disease, vascular dementia, cognitive dysfunction, risk factor, nomogram, predictive model
Citation: Zhang L, Gao F, Zhang Y, Hu P, Yao Y, Zhang Q, He Y, Shang Q and Zhang Y (2022) Analysis of risk factors for the development of cognitive dysfunction in patients with cerebral small vessel disease and the construction of a predictive model. Front. Neurol. 13:944205. doi: 10.3389/fneur.2022.944205
Received: 14 May 2022; Accepted: 22 July 2022;
Published: 11 August 2022.
Edited by:
Alberto Serrano-Pozo, Massachusetts General Hospital and Harvard Medical School, United StatesReviewed by:
Lukas Sveikata, Massachusetts General Hospital and Harvard Medical School, United StatesSuraj Mishra, University of Notre Dame, United States
Copyright © 2022 Zhang, Gao, Zhang, Hu, Yao, Zhang, He, Shang and Zhang. This is an open-access article distributed under the terms of the Creative Commons Attribution License (CC BY). The use, distribution or reproduction in other forums is permitted, provided the original author(s) and the copyright owner(s) are credited and that the original publication in this journal is cited, in accordance with accepted academic practice. No use, distribution or reproduction is permitted which does not comply with these terms.
*Correspondence: Yi Zhang, emhhbmd5aTkzMTBAMjFjbi5jb20=
†These authors have contributed equally to this work