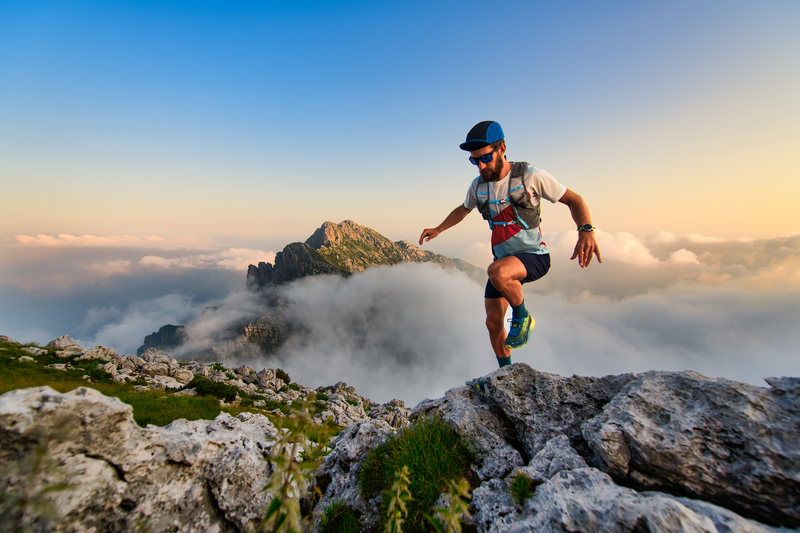
94% of researchers rate our articles as excellent or good
Learn more about the work of our research integrity team to safeguard the quality of each article we publish.
Find out more
ORIGINAL RESEARCH article
Front. Neurol. , 01 August 2022
Sec. Neurological Biomarkers
Volume 13 - 2022 | https://doi.org/10.3389/fneur.2022.942023
This article is part of the Research Topic Potential Biomarkers in Neurovascular Disorders View all 50 articles
Drug efficacy can be improved by understanding the effects of anesthesia on the neurovascular system. In this study, we used machine learning algorithms to predict the risk of infection in postoperative intensive care unit (ICU) patients who are on non-mechanical ventilation and are receiving hydromorphone analgesia. In this retrospective study, 130 patients were divided into high and low dose groups of hydromorphone analgesic pump patients admitted after surgery. The white blood cells (WBC) count and incidence rate of infection was significantly higher in the high hydromorphone dosage group compared to the low hydromorphone dosage groups (p < 0.05). Furthermore, significant differences in age (P = 0.006), body mass index (BMI) (P = 0.001), WBC count (P = 0.019), C-reactive protein (CRP) (P = 0.038), hydromorphone dosage (P = 0.014), and biological sex (P = 0.024) were seen between the infected and non-infected groups. The infected group also had a longer hospital stay and an extended stay in the intensive care unit compared to the non-infected group. We identified important risk factors for the development of postoperative infections by using machine learning algorithms, including hydromorphone dosage, age, biological sex, BMI, and WBC count. Logistic regression analysis was applied to incorporate these variables to construct infection prediction models and nomograms. The area under curves (AUC) of the model were 0.835, 0.747, and 0.818 in the training group, validation group, and overall pairwise column group, respectively. Therefore, we determined that hydromorphone dosage, age, biological sex, BMI, WBC count, and CRP are significant risk factors in developing postoperative infections.
Patients in the intensive care unit (ICU) experience varying degrees of pain, delirium, agitation, and arousal. These conditions can lead to prolonged ICU stays and increased morbidity and mortality (1–4). As a result, analgesia and sedation have become part of routine ICU care, leading to the current e-CASH (early comfort using analgesia, minimal sedatives, and maximal humane care) sedation concept (5). The development of clinical drugs used for analgesia and sedation of ICU patients has been increasing in number. Assessing the condition and determining its pharmacology can lead to better analgesia with narcotics (6–9). In the ICU, we are more likely to make better treatment decisions if we understand the different effects of anesthesia on the neurovascular system (10).
Morphine is widely used internationally as a classical neurovascular anesthetic because of its low price and significant effects (11–13). However, repeated administration of morphine can result in serious adverse events (14). Hydromorphone, a new analgesic drug, is a semi-synthetic morphine derivative. The analgesic effect is approximately 8–10 times better than morphine, and it has fast onset of action, fewer side effects, various routes of administration, low risk of hallucination addiction, low risk of gastrointestinal adverse reactions, non-toxic metabolites, and better suitability for patients with decompensated liver and kidney function (13, 15). Hydromorphone has been extensively validated for use in an emergency, day surgery, and perioperative management settings, with significant advantages in reducing respiratory depression and gastrointestinal adverse effects (15–18). However, further studies are needed to assess the effectiveness, safety, and dosage of this medication in the ICU.
In contrast to other analgesics, hydromorphone has been found to reduce the incidence of postoperative pneumonia in patients undergoing thoracic surgery; however, in general, infection remains to be a significant complication of hydromorphone (17, 19, 20). For one, an increased incidence of infective endocarditis has been associated with hydromorphone injection (20). Similarly, intrathecal targeted drug delivery of hydromorphone may lead to device infection (21). These complications may be problematic since inflammation and infection have been shown to reduce the effectiveness of some analgesics (22). Moreover, the postoperative infection has been found to be an important risk factor affecting the survival of patients (23, 24). These suggest that in clinical practice, the risk of infection needs to be predicted.
Machine learning is currently an important means of implementing artificial intelligence technologies, and the application of these algorithms in clinical diagnosis and decision-making has become a research priority in the medical field (25–27). In non-mechanically ventilated ICU, predicting the risk of hydromorphone infection is very challenging and requires an accurate biological classification model utilizing simple and effective decision rules. In relation to this, random forest models and support vector machine models are frequently used in classification tasks to predict treatment effects or complications and to screen for clinically important features related to outcomes (28). Logistic regression analysis is often used to screen for risk factors associated with adverse outcomes, and it can also be utilized in constructing a nomogram that can be easily applied clinically for the accurate detection and treatment of a disease (29, 30). In non-mechanically ventilated ICU patients after surgery, we believe that these machine learning tools can be used to predict the risk of infection with hydromorphone analgesia.
The purpose of this study is to develop a validated tool that can utilize available clinical information to predict the risk of infection in ICU patients receiving post-surgical hydromorphone analgesia. This will ultimately reduce hospital stays, medical costs, and length of hospital stays post-operatively, as well as provide guidance for future studies to improve analgesic outcomes for ICU patients.
After institutional ethics committee approval, a group of patients admitted to the ICU after surgical procedures and who received analgesic treatment was enrolled in the study. Since this study used a retrospective analysis format, patients' informed consent was waived. The inclusion criteria used were as follows: (1) age between 45 and 90; (2) American Society of Anesthesiologists (ASA) physical score I to II; and (3) cardiac left ventricular ejection fraction greater than 40%. Meanwhile, the exclusion criteria used were as follows: (1) those with significant preoperative heart, liver, or kidney disease; (2) those with neurological or psychiatric diseases; (3) those with lung conditions such as chronic obstructive pulmonary disease or chronic bronchitis; (4) those with allergies to study drugs such as opioid prescriptions; and (5) those with a body mass index of 30 kg/m2. Overall, a total of 130 patients passed the screening criteria. The general characteristics of these patients, such as biological sex, age, height, weight, BMI, etc., were collected through a clinical history data review. In addition, Acute Physiology and Chronic Health Evaluation (APACHE II) and Sepsis Related Organ Failure Assessment (SOFA) scores were collected in full at the time of patient admission to the ICU.
The pumping speed of the micropump was adjusted to achieve satisfactory analgesia. Patients were evaluated for pain and physiological indicators every 2 h. Patients whose daily hydromorphone dosage exceeded 40 mg/mL were included in the high hydromorphone dosage group, and those who did not exceed 40 mg/mL were included in the low hydromorphone dosage group.
Physiological and biochemical indices, such as heart rate (HR), mean artery pressure (MAP), respiratory rate (R), C-reactive protein (CRP), white blood cell (WBC) count, platelet (PLT), and Saturation of Pulse Oxygen (SpO2), were recorded completely after 12 h of analgesia, from the moment that the patient was admitted to ICU with self-administered hydromorphone analgesia. In addition, the occurrence of adverse reactions throughout the postoperative treatment period was recorded. In this study, the primary outcome indicator was defined as the occurrence of infection. Postoperative infection was evaluated based on the following indicators: (1) temperature >38 °C; (2) elevated WBC count; (3) positive sputum culture or blood culture showing bacteria; (4) chest X-ray showing abnormal density; and (5) diagnosis of pneumonia, etc. (31).
Firstly, a random forest model was utilized to fit this dataset so as to rank the importance of each clinical feature in terms of the infection outcome (32). Subsequently, in order to screen clinical features related to infection, Support Vector Machine-Recursive Feature Elimination (SVM-RFE) was applied to the general characteristics of patients, secondary indicators for the prediction model of infection important features screening and model construction (33). A support vector machine (SVM) is often used for non-linear classification, and they perform well on small samples. The SVM-RFE technique uses iterative iterations to remove redundant feature variables. Root Mean Squard Error (RMSE) metrics were applied to evaluate the accuracy of the SVM models and to determine the best model variables based on the RMSE minimum. Furthermore, neural networks, a common non-linear algorithmic model in machine learning, were also used to evaluate results as previous researches (34–38).
Univariate and multifactorial logistic regression analyses were also applied to assess the risk ratio of each clinical factor on infection outcome (39). In the first step, we performed univariate logistic regression analysis sequentially and analyzed the characteristics of the patients based upon P < 0.05. Those variables that were subjected to multifactor logistic regression analysis were then screened according to P < 0.2 to identify the variables obtained from logistic analysis. Based on the odds ratios (ORs) and 95% confidence intervals (95% CIs) for each variable, we calculated how much each characteristic was associated with infection risk. We then applied univariate logistic regression to determine which variables were independent risk factors.
Intersection analysis was conducted to confirm the shared characteristics between the variables screened using the Support Vector Machine-Recursive Feature Elimination (SVM-RFE) model and those screened using logistic regression analysis (40–45). To determine the amount of information that these shared features can contain about the outcomes, PCA (principle component analysis) is applied to downscale them. In addition, neural network models with multilayer network structures are continuously used in several fields. Furthermore, these shared important features are also passed into the neural network model as input features to measure the classification performance of the variables under the neural network model.
For the construction of infection prediction models, a multi-factor logistic regression model was used based on these important characteristics. By comparing the predicted probability values of the model to the actual results, the calibration curve further evaluates the accuracy of the model. A decision curve analysis (DCA) was used to evaluate the clinical safety of this clinical prediction model by measuring the yield at different prediction probability thresholds (46). The infection prediction model was then plotted using the “rms” package to obtain a clinical visualization tool to facilitate clinical translation.
Software R (version 4.0.2) was used for all analyses and graphs. Training and evaluation of the individual prediction models were conducted by dividing the entire dataset into a training and a test set. The receiver operating characteristic curve (ROC) and the area under the curve (AUC) were used to evaluate the predictive performance of the model. PROC was used to plot ROC curves (47). Pearson's chi-square test or Fisher's exact test were used for the difference test analysis on the count data. The measurement data were analyzed using the Wilcoxon rank sum test or unpaired Student's t-test. We considered a significance level of p 0.05 to be statistically significant.
In the clinical study of 130 patients admitted to the ICU after surgery, 65 patients received an average daily hydromorphone dosage of more than 40 mg/mL and were included in the high hydromorphone dosage group; the remaining 65 patients were included in the low hydromorphone dosage group. An overview of the patients' baseline clinical information is given in Tables 1, 2. Hydromorphone dosage groups did not differ statistically in terms of biological sex, age, weight, height, BMI, hypertension, diabetes, APACHE II, and SOFA scores. However, the high hydromorphone dosage group had a high WBC count (P = 0.036) and a high infection risk (P = 0.014). This suggests that high hydromorphone dosages may increase the risk of infection.
Twenty-five patients developed postoperative infections, while the other 105 did not. Table 3 summarizes the clinical characteristics of the infected and uninfected patients. The occurrence of infection was associated with age (P = 0.006), BMI (P = 0.001), WBC count (P = 0.019), CRP (P = 0.038), hydromorphone dosage (P = 0.014), and biological sex (P = 0.024). Infected patients were older, had a lower BMI, higher WBC counts, higher CRP, and used more hydromorphone than non-infected patients. These results suggest that together with hydromorphone use, multiple clinical characteristics can be associated with the occurrence of postoperative infections in surgical patients.
Based on a random forest model analysis, PLT, age, MAP, CRP, and HR were the top five clinical features associated with infection (Figure 1A). According to Figure 1B, the ROC curves for training and test sets had AUCs of 1.00 and 0.61, respectively, suggesting that the model was overfitted. After using the SVM-RFE method, we found that the model had the smallest Root Mean Square Error (RMSE) value when 16 variables were included (Figure 1C). AUCs of the SVM model based on these 16 variables were 0.822, 0.853, and 0.830, respectively, for the training, test, and overall datasets (Figure 1D). In the test set, SVM showed better classification ability than random forest model, according to the results of the appeal.
Figure 1. Feature ranking and filtering process for Random Forest and SVM-RFE models. (A) Bar chart showing a random forest model's importance ranking of each variable. PLT, age, MAP, CRP, and HR are the top five variables identified. (B) ROC curves showing the classification ability of the random forest model. (C) The feature screening process of SVM-RFE results in the model with the lowest RMSE when 16 variables are selected. (D) ROC curves showing training, test, and overall classification performances of the SVM model.
Risk factors associated with infection were identified using univariate and multifactorial logistic regression analyses. Univariate logistic regression analysis revealed an association between hydromorphone dosage, group (P = 0.018), age (P = 0.008), biological sex (P = 0.031), BMI (P = 0.002), WBC (P = 0.023), and CRP (P = 0.042). Furthermore, multifactorial logistic regression analysis revealed that age (P = 0.042), BMI (P = 0.005), and WBC (P = 0.031) were independent risk factors for infection (Table 4). Furthermore, a multifactorial logistic regression analysis with P < 0.2 as a filter identified Hydromorphone dosage group, age, biological sex, BMI, WBC count, and CRP as clinically important factors that contribute to infection risk.
According to the intersection analysis of SVM-RFE and logistic regression analysis, six variables were coinciding with the SVM-RFE and logistic regression analysis, including hydromorphone dosage group, age, biological sex, BMI, WBC count, and CRP (Figure 2A). These six variables have sufficient information to predict infection occurrence according to principal component analysis (PCA) (Figure 2B). According to ROC curve analysis, age (AUC = 0.681), biological sex (AUC = 0.620), BMI (AUC = 0.706), dosage group (AUC = 0.636), WBC count (AUC = 0.626), and CRP (AUC = 0.608) were all predictive of infection (Figures 2C–H). Supplementary figure 1 illustrates the training process of the neural network model based on these infection-related variables. In both the training and validation sets, the neural network model accurately predicted infection. However, the neural network model only performed 0.539 accurately in the test set. It is possible that this deficiency is due to the small number of patients included in this study.
Figure 2. SVM-RFE as well as logistic regression models are used for screening important clinical features. (A) Venn diagram showing six features associated with infection prediction. (B) PCA showing that based on these six characteristics, a better distinction can be made between infected and uninfected patients. (C–H) ROC curves showing the predictive performance of (C) age, (D) biological sex, (E) BMI, (F) hydromorphone concentration grouping, (G) WBC, and (H) CRP on infection.
Based on the six identified clinical variables, a multifactorial logistic regression algorithm was applied to construct a prediction model to detect the occurrence of infection in relation to the clinical use of hydromorphone. Table 4 shows the coefficients of the multifactor logistic regression model based on these six clinical characteristic variables. In the training group, validation group, and overall pairwise column, respectively, the ROC curves showed an AUC of 0.835, 0.818, and 0.747, indicating the model has good predictive ability (Figure 3A). Based on the multifactor logistic regression model, a nomogram was constructed (Figure 3B) to facilitate its clinical application. The correction curves show that the predicted values of the infection prediction model and the true label are in general agreement, further supporting the good predictive power of the model (Figure 3C). Clinical decision curves show that the predictions from the prediction model have clinical value for patients (Figure 3D). Projecting the basic characteristics of the patients and hydromorphone use onto this nomogram allows for the easy calculation of the probability of infection risk, thus guiding hydromorphone use and patient testing in the clinical setting.
Figure 3. A nomogram based on six factors is constructed and its accuracy is assessed. (A) The ROC curves for the logistic regression model constructed based on the six identified clinical factors for infection in training, validation, and overall pairwise column sets demonstrate better classification performance in all three datasets. (B) The nomogram was constructed using a logistic regression model. (C) Calibration plot showing the predicted values of the model are roughly consistent with the true labels, indicating that the model is reasonably accurate. (D) Clinical decision curve showing the prediction results in an overall pairwise column.
In addition, infected patients spent more time in the intensive care unit and hospital overall than their non-infected counterparts (Figure 4). Infections in intensive care units can prolong the stay in the unit, resulting in increased healthcare costs.
Figure 4. Compared between infected and non-infected patients, duration of ICU stay and hospitalization. In the infected group, the hospital stay and ICU stay were longer than in the non-infected group.
A machine learning approach was used to predict the risk of infection among non-mechanically ventilated ICU patients receiving hydromorphone analgesia after surgical procedures. According to the study, different factors are associated with the occurrence of postoperative infections in surgical patients based on a cohort study. We identified significant risk factors affecting the development of postoperative infections in patients based on these data, including hydromorphone dosage, age, biological sex, BMI, WBC count, and CRP. Logistic regression was used to construct a predictive model for detecting infection. To assist physicians in assessing the risk of postoperative infections in surgical patients, a nomogram was created based on this model.
In postoperative surgical patients, infections are common adverse effects of opioid analgesics, which can reduce their quality of life, and prolong their length of stay (48–51). A high hydromorphone dosage was associated with a higher incidence of postoperative infections in this study. It has been shown that patients on hydromorphone have less than normal immune system defenses, which may allow bacteria and viruses to infiltrate the body and multiply (20, 52). It has also been shown that hydromorphone, as an opioid analgesic, can cause adverse effects, such as excessive sedation and respiratory depression, pulmonary atelectasis, and infection (53–55). Additionally, hydromorphone promotes bacterial translocation by breaking endothelial tight junctions via the Toll-like receptor 2 (20). Opioids increase intestinal bacterial translocation, dysregulated immune responses, and intestinal barrier permeability, thereby increasing the risk of intestinal infections (56). We also found that the WBC count and CRP after 12 h of analgesia were higher in the high hydromorphone dosage group than in the low hydromorphone dosage group. Clinical parameters such as these have been shown to be risk factors for postoperative infection in this study. Infections are more likely to occur in the high hydromorphone dosage group, according to these findings.
Among patients admitted to the ICU after surgery and receiving analgesic treatment, old age was an independent risk factor for infection development. There may be an important correlation between this finding and low immunity in the elderly (57). Similarly, low BMI was identified as an independent risk factor for infection, possibly due to findings that low BMI correlates with worse nutritional status, postoperative recovery, and greater susceptibility to infection (58–60). Furthermore, males had a higher proportion of infections in postoperative ICU admissions than females. Different sex hormones induce different gene expression and immune responses in males and females, which may contribute to different susceptibility to infection (61). Estradiol appears to confer protective immunity, while progesterone and testosterone suppress anti-infection responses (62). Occupational differences and lifestyle differences may also play a role. Therefore, post-analgesic infections should be closely monitored in patients over the age of 65, those with low body mass indexes, and those admitted to the ICU after surgery.
According to our analysis, patients in the infected group spend more time in the ICU and in the hospital, resulting in higher costs and more resource utilization. Infection is a common adverse effect of opioid analgesics in postoperative surgical patients (48, 49). Postoperative infection can negatively impact the patient's prognosis, which negatively impacts their recovery (50, 51). Patients will benefit medically and economically from the early detection and timely treatment of infections after surgery in the ICU.
Despite the higher risk of infection associated with high doses of hydromorphone, postoperative pain control can improve patient recovery. There is evidence that timely and effective relief of postoperative pain enhances recovery, leads to fewer complications, and shortens hospital stays (63). Hydromorphone is commonly used as a bout of pain medication; however, its use also introduces a range of side effects. In order to identify infection-related factors, we used logistic regression to construct infection prediction models and developed an easy-to-use clinical infection prediction nomogram. The tool will help physicians evaluate the risk of infection in surgical patients using hydromorphone for analgesia promptly and may enable early medical intervention, as required by precision medicine. Pain control contributes to the recovery of surgical patients, and timely detection and reduction of analgesic medication use can reduce adverse effects and decrease ICU and hospital stays. A patient-centered big medical data set should be constructed in future studies by collecting all manner of basic patient information, treatment information, and outcomes indicators with the aid of various machine learning predictive models. It is important to design prospective cohort studies to further validate this model, as well as to expand the study population to include different types of surgical and postoperative infections in order to achieve precision medicine.
This study explores and clarifies hydromorphone's efficacy and safety in the ICU. Hydromorphone dosage, age, biological sex, BMI, WBC count, and CRP have been found to be significant risk factors for developing postoperative infections in non-mechanically ventilated patients in the ICU after surgery. Based on these six clinical variables, infection prediction models have good predictive power and can be used to guide hydromorphone use more safely.
The original contributions presented in the study are included in the article/Supplementary material, further inquiries can be directed to the corresponding author.
The studies involving human participants were reviewed and approved by the Ethics Committee of Shanxi Bethune Hospital. The patients/participants provided their written informed consent to participate in this study.
YD and WW: conceptualization and validation. YD: methodology. HS: software. YD and XY: data curation. YD, HS, WW, and XY: writing—original draft preparation. WW: writing—review and editing. All authors contributed to the article and approved the submitted version.
The authors declare that the research was conducted in the absence of any commercial or financial relationships that could be construed as a potential conflict of interest.
All claims expressed in this article are solely those of the authors and do not necessarily represent those of their affiliated organizations, or those of the publisher, the editors and the reviewers. Any product that may be evaluated in this article, or claim that may be made by its manufacturer, is not guaranteed or endorsed by the publisher.
The Supplementary Material for this article can be found online at: https://www.frontiersin.org/articles/10.3389/fneur.2022.942023/full#supplementary-material
Supplementary Figure 1. The training process for the neural network prediction model based on the six identified clinical features. The line graphs illustrate the (A) loss of training and validation, and (B) changes in AUC values for the prediction models in the training and validation sets during training.
1. Skrobik Y, Chanques G. The pain, agitation, and delirium practice guidelines for adult critically ill patients: a post-publication perspective. Ann Intensive Care. (2013) 3:9. doi: 10.1186/2110–5820-3–9
2. Wong IMJ, Thangavelautham S, Loh SCH, Ng SY, Murfin B, Shehabi Y. Sedation and delirium in the intensive care unit-a practice-based approach. Ann Acad Med Singap. (2020) 49:215–25. doi: 10.47102/annals-acadmed.sg.202013
3. Chanques G, Sebbane M, Barbotte E, Viel E, Eledjam J-J, Jaber S, et al. Prospective study of pain at rest: incidence and characteristics of an unrecognized symptom in surgical and trauma versus medical intensive care unit patients. Anesthesiology. (2007) 107:858–60. doi: 10.1097/01.anes.0000287211.98642.51
4. Erstad BL, Puntillo K, Gilbert HC, Grap MJ Li D, Medina J, Mularski RA, et al. Pain management principles in the critically Ill. Chest. (2009) 135:1075–86. doi: 10.1378/chest.08–2264
5. Vincent J-L, Shehabi Y, Walsh TS, Pandharipande PP, Ball JA, Spronk P, et al. Comfort and patient-centred care without excessive sedation: the eCASH concept. Intensive Care Med. (2016) 42:962–71. doi: 10.1007/s00134–016-4297–4
6. Lee S. Dexmedetomidine: present and future directions. Korean J Anesthesiol. (2019) 72:323–30. doi: 10.4097/kja.19259
7. Sahinovic MM, Struys MMRF, Absalom AR. Clinical pharmacokinetics and pharmacodynamics of propofol. Clin Pharmacokinet. (2018) 57:1539–58. doi: 10.1007/s40262–018-0672–3
8. Hemmings HC, Riegelhaupt PM, Kelz MB, Solt K, Eckenhoff RG, Orser BA, et al. Towards a comprehensive understanding of anesthetic mechanisms of action: a decade of discovery. Trends Pharmacol Sci. (2019) 40:464–81. doi: 10.1016/j.tips.2019.05.001
9. Huang H, Jiang H, Liu J, Chen J, Qiu L, Wang J, et al. Facial nerve monitoring under different levels of neuromuscular blockade with cisatracurium besilate in parotid tumour surgery. Biomed Res Int. (2021) 2021:5655061. doi: 10.1155/2021/5655061
10. Masamoto K, Kanno I. Anesthesia and the quantitative evaluation of neurovascular coupling. J Cereb Blood Flow Metab. (2012) 32:1233–47. doi: 10.1038/jcbfm.2012.50
11. Richards-Belle A, Canter RR, Power GS, Robinson EJ, Reschreiter H, Wunsch H, et al. National survey and point prevalence study of sedation practice in UK critical care. Crit Care. (2016) 20:355. doi: 10.1186/s13054–016-1532-x
12. Borne J, Riascos R, Cuellar H, Vargas D, Rojas R. Neuroimaging in drug and substance abuse part II: opioids and solvents. Topics Magn Reson Imag. (2005) 16:239–45. doi: 10.1097/01.rmr.0000192154.34563.6b
13. Solis E, Afzal A, Kiyatkin EA. Opposing mechanisms underlying differential changes in brain oxygen and temperature induced by intravenous morphine. J Neurophysiol. (2018) 120:2513–21. doi: 10.1152/jn.00445.2018
14. McDonald DD, Srisopa P. Predictors of serious adverse drug events from opioids: results from the food and drug administration adverse events reporting system. J Am Assoc Nurse Pract. (2021) 33:1207–15. doi: 10.1097/JXX.0000000000000545
15. Li Y, Ma J, Lu G, Dou Z, Knaggs R, Xia J, Zhao S, Dong S, Yang L. Hydromorphone for cancer pain. Cochrane Database Syst Rev. (2021) 8:CD011108. doi: 10.1002/14651858.CD011108.pub3
16. Nascimento R, Poklis A, Wolf CE. Evaluation of a newly formulated enzyme immunoassay for the detection of hydrocodone and hydromorphone in pain management compliance testing. J Anal Toxicol. (2016) 40:583–7. doi: 10.1093/jat/bkw080
17. Yan G, Chen J, Yang G, Duan G, Du Z, Yu Z, et al. Effects of patient-controlled analgesia with hydromorphone or sufentanil on postoperative pulmonary complications in patients undergoing thoracic surgery: a quasi-experimental study. BMC Anesthesiol. (2018) 18:192. doi: 10.1186/s12871–018-0657–7
18. Alhabardi S, Almodaimegh H, Alammari M. Pattern of hydromorphone use in King Abdulaziz Medical City-Central Region (KAMC-CR). Sci Rep. (2021) 11:8760. doi: 10.1038/s41598–021-88276–7
19. Wasiura J, Segal BH, Mullin KM. Cluster of sphingomonas paucimobilis bacteremias linked to diversion of intravenous hydromorphone. N Engl J Med. (2019) 381:584–5. doi: 10.1056/NEJMc1902973
20. Bassareo PP. Hydromorphone and risk of infective endocarditis. Lancet Infect Dis. (2020) 20:651–2. doi: 10.1016/S1473–3099(20)30269–3
21. Moman RN, Rogers JM, Pittelkow TP. High cervical intrathecal targeted drug delivery: a case report of refractory oropharyngeal cancer pain. Case Rep Oncol Med. (2019) 2019:1–3. doi: 10.1155/2019/2098921
22. Blake KJ, Baral P, Voisin T, Lubkin A, Pinho-Ribeiro FA, Adams KL, et al. Staphylococcus aureus produces pain through pore-forming toxins and neuronal TRPV1 that is silenced by QX-314. Nat Commun. (2018) 9:37. doi: 10.1038/s41467–017-02448–6
23. Baba Y, Yoshida N, Shigaki H, Iwatsuki M, Miyamoto Y, Sakamoto Y, et al. Prognostic impact of postoperative complications in 502 patients with surgically resected esophageal squamous cell carcinoma: a retrospective single-institution study. Ann Surg. (2016) 264:305–11. doi: 10.1097/SLA.0000000000001510
24. Tu R-H, Lin J-X, Li P, Xie J-W, Wang J-B, Lu J, et al. Prognostic significance of postoperative pneumonia after curative resection for patients with gastric cancer. Cancer Med. (2017) 6:2757–65. doi: 10.1002/cam4.1163
25. Pattarabanjird T, Cress C, Nguyen A, Taylor A, Bekiranov S, McNamara C, et al. Machine learning model utilizing a novel SNP shows enhanced prediction of coronary artery disease severity. Genes. (2020) 11:1446. doi: 10.3390/genes11121446
26. Silcox C, Dentzer S, Bates DW. AI-enabled clinical decision support software: a “trust and value checklist” for clinicians. NEJM Catalyst. (2020) 1:CAT.20.0212. doi: 10.1056/CAT.20.0212
27. De Cock M, Dowsley R, Nascimento ACA, Railsback D, Shen J, Todoki A. High performance logistic regression for privacy-preserving genome analysis. BMC Med Genom. (2021) 14:23. doi: 10.1186/s12920–020-00869–9
28. Hsieh MH, Hsieh MJ, Chen C-M, Hsieh C-C, Chao C-M, Lai C-C. Comparison of machine learning models for the prediction of mortality of patients with unplanned extubation in intensive care units. Sci Rep. (2018) 8:17116. doi: 10.1038/s41598–018-35582–2
29. Huang J, Liu M, He W, Liu F, Cheng J, Wang H. Use of the A2DS2 scale to predict morbidity in stroke-associated pneumonia: a systematic review and meta-analysis. BMC Neurol. (2021) 21:33. doi: 10.1186/s12883–021-02060–8
30. Liu H, Yan Y, Chen R, Zhu M, Lin J, He C, et al. Integrated nomogram based on five stage-related genes and TNM stage to predict 1-year recurrence in hepatocellular carcinoma. Cancer Cell Int. (2020) 20:140. doi: 10.1186/s12935–020-01216–9
31. Peterson JJ. Postoperative infection. Radiol Clin North Am. (2006) 44:439–50. doi: 10.1016/j.rcl.2006.01.007
32. Blanchet L, Vitale R, van Vorstenbosch R, Stavropoulos G, Pender J, Jonkers D, et al. Constructing bi-plots for random forest: tutorial. Anal Chim Acta. (2020) 1131:146–55. doi: 10.1016/j.aca.2020.06.043
33. Huang M-L, Hung Y-H, Lee WM Li RK, Jiang B-R. SVM-RFE based feature selection and Taguchi parameters optimization for multiclass SVM classifier. Sci World J. (2014) 2014:795624. doi: 10.1155/2014/795624
34. Cao C, Liu F, Tan H, Song D, Shu W, Li W, et al. Deep learning and its applications in biomedicine. Genom Proteom Bioinform. (2018) 16:17–32. doi: 10.1016/j.gpb.2017.07.003
35. Feng X, Guan N, Xu E, Miao Y, Li C. Transcription factors leading to high expression of neuropeptide L1CAM in brain metastases from lung adenocarcinoma and clinical prognostic analysis. Dis Markers. (2021) 2021:1–15. doi: 10.1155/2021/8585633
36. Chen Y, Sun Y, Luo Z, Lin J, Qi B, Kang X, et al. Potential mechanism underlying exercise upregulated circulating blood exosome miR-215–5p to prevent necroptosis of neuronal cells and a model for early diagnosis of alzheimer's disease. Front Aging Neurosci. (2022) 14:860364. doi: 10.3389/fnagi.2022.860364
37. Xuan Z, Ma T, Qin Y, Guo Y. Role of ultrasound imaging in the prediction of TRIM67 in brain metastases from breast cancer. Front Neurol. (2022) 13:889106. doi: 10.3389/fneur.2022.889106
38. Hu C, Li Q, Shou J, Zhang F, Li X, Wu M, et al. Constructing a predictive model of depression in chemotherapy patients with non-hodgkin's lymphoma to improve medical staffs' psychiatric care. Biomed Res Int. (2021) 2021:9201235. doi: 10.1155/2021/9201235
39. Tripepi G, Jager KJ, Stel VS, Dekker FW, Zoccali C. How to deal with continuous and dichotomic outcomes in epidemiological research: linear and logistic regression analyses. Nephron Clin Pract. (2011) 118:c399–c406. doi: 10.1159/000324049
40. Jiang F, Jiang Y, Zhi H, Dong Y, Li H, Ma S, et al. Artificial intelligence in healthcare: past, present and future. Stroke Vasc Neurol. (2017) 2:230–43. doi: 10.1136/svn-2017–000101
41. Patel L, Shukla T, Huang X, Ussery DW, Wang S. Machine learning methods in drug discovery. Molecules. (2020) 25:5277. doi: 10.3390/molecules25225277
42. Buchlak QD, Esmaili N, Leveque J-C, Farrokhi F, Bennett C, Piccardi M, et al. Machine learning applications to clinical decision support in neurosurgery: an artificial intelligence augmented systematic review. Neurosurg Rev. (2020) 43:1235–53. doi: 10.1007/s10143–019-01163–8
43. Liu Z, Li C A. Predictive model for the risk of cognitive impairment in patients with gallstones. Biomed Res Int. (2021) 2021:1–13. doi: 10.1155/2021/3792407
44. Zhang Q, Yang J, Yang C, Yang X, Chen Y. Eucommia ulmoides Oliver-Tribulus terrestris L. drug pair regulates ferroptosis by mediating the neurovascular-related ligand-receptor interaction pathway- a potential drug pair for treatment hypertension and prevention ischemic stroke. Front Neurol. 13:833922. doi: 10.3389/fneur.2022.833922
45. Kang X-R, Chen B, Chen Y-S, Yi B, Yan X, Jiang C, Wang S, Lu L, Shi R. A prediction modeling based on SNOT-22 score for endoscopic nasal septoplasty: a retrospective study. PeerJ. (2020) 8:e9890. doi: 10.7717/peerj.9890
46. Steyerberg EW, Vergouwe Y. Towards better clinical prediction models: seven steps for development and an ABCD for validation. Eur Heart J. (2014) 35:1925–31. doi: 10.1093/eurheartj/ehu207
47. Robin X, Turck N, Hainard A, Tiberti N, Lisacek F, Sanchez J-C, et al. pROC: an open-source package for R and S+ to analyze and compare ROC curves. BMC Bioinform. (2011) 12:77. doi: 10.1186/1471–2105-12–77
48. Hassan Z, Nisiewicz MJ, Ueland W, Plymale MA, Plymale MC, Davenport DL, et al. Preoperative opioid use and incidence of surgical site infection after repair of ventral and incisional hernias. Surgery. (2020) 168:921–5. doi: 10.1016/j.surg.2020.05.048
49. Pirkle S, Reddy S, Bhattacharjee S, Shi LL, Lee MJ. Chronic opioid use is associated with surgical site infection after lumbar fusion. Spine. (2020) 45:837–42. doi: 10.1097/BRS.0000000000003405
50. Kato S, Chikuda H, Ohya J, Oichi T, Matsui H, Fushimi K, et al. Risk of infectious complications associated with blood transfusion in elective spinal surgery—a propensity score matched analysis. Spine J. (2016) 16:55–60. doi: 10.1016/j.spinee.2015.10.014
51. Tran CW, McGree ME, Weaver AL, Martin JR, Lemens MA, Cliby WA, et al. Surgical site infection after primary surgery for epithelial ovarian cancer: predictors and impact on survival. Gynecol Oncol. (2015) 136:278–84. doi: 10.1016/j.ygyno.2014.12.007
52. Khosrow-Khavar F, Kurteva S, Cui Y, Filion KB, Douros A. Opioids and the risk of infection: a critical appraisal of the pharmacologic and clinical evidence. Expert Opinion Drug Metabolism Toxicol. (2019) 15:565–75. doi: 10.1080/17425255.2019.1634053
53. Yang Y, Wu J, Li H, Ye S, Xu X, Cheng L, et al. Prospective investigation of intravenous patient-controlled analgesia with hydromorphone or sufentanil: impact on mood, opioid adverse effects, and recovery. BMC Anesthesiol. (2018) 18:37. doi: 10.1186/s12871–018-0500–1
54. Shanthanna H, Paul J, Lovrics P, Vanniyasingam T, Devereaux PJ, Bhandari M, Thabane L. Satisfactory analgesia with minimal emesis in day surgeries: a randomised controlled trial of morphine versus hydromorphone. Br J Anaesthesia. (2019) 122:e107–e113. doi: 10.1016/j.bja.2019.03.036
55. Stone AB, Jones MR, Rao N, Urman RD. A dashboard for monitoring opioid-related adverse drug events following surgery using a national administrative database. Am J Med Qual. (2019) 34:45–52. doi: 10.1177/1062860618782646
56. Wang F, Roy S. Gut homeostasis, microbial dysbiosis, and opioids. Toxicol Pathol. (2017) 45:150–156. doi: 10.1177/0192623316679898
57. Candore G, Caruso C, Jirillo E, Magrone T, Vasto S. Low grade inflammation as a common pathogenetic denominator in age-related diseases: novel drug targets for anti-ageing strategies and successful ageing achievement. CPD. (2010) 16:584–596. doi: 10.2174/138161210790883868
58. Cederholm T, Bosaeus I, Barazzoni R, Bauer J, Van Gossum A, Klek S, et al. Diagnostic criteria for malnutrition—an ESPEN consensus Statement. Clin Nutr. (2015) 34:335–40. doi: 10.1016/j.clnu.2015.03.001
59. Rajamanickam A, Munisankar S, Dolla CK, Babu S. Diminished systemic and mycobacterial antigen specific anti-microbial peptide responses in low body mass index–latent tuberculosis co-morbidity. Front Cell Infect Microbiol. (2020) 10:165. doi: 10.3389/fcimb.2020.00165
60. Chandrasekaran P, Saravanan N, Bethunaickan R, Tripathy S. Malnutrition: modulator of immune responses in tuberculosis. Front Immunol. (2017) 8:1316. doi: 10.3389/fimmu.2017.01316
61. McClelland EE, Smith JM. Gender specific differences in the immune response to infection. Arch Immunol Ther Exp. (2011) 59:203–13. doi: 10.1007/s00005–011-0124–3
62. Gay L, Melenotte C, Lakbar I, Mezouar S, Devaux C, Raoult D, et al. Sexual dimorphism and gender in infectious diseases. Front Immunol. (2021) 12:698121. doi: 10.3389/fimmu.2021.698121
Keywords: machine learning, post-surgical ICU, neurovascular, anesthesia, infection, hydromorphone
Citation: Du Y, Shi H, Yang X and Wu W (2022) Machine learning for infection risk prediction in postoperative patients with non-mechanical ventilation and intravenous neurotargeted drugs. Front. Neurol. 13:942023. doi: 10.3389/fneur.2022.942023
Received: 12 May 2022; Accepted: 08 July 2022;
Published: 01 August 2022.
Edited by:
Yuzhen Xu, Tongji University, ChinaReviewed by:
Jie Tao, Shanghai General Hospital, ChinaCopyright © 2022 Du, Shi, Yang and Wu. This is an open-access article distributed under the terms of the Creative Commons Attribution License (CC BY). The use, distribution or reproduction in other forums is permitted, provided the original author(s) and the copyright owner(s) are credited and that the original publication in this journal is cited, in accordance with accepted academic practice. No use, distribution or reproduction is permitted which does not comply with these terms.
*Correspondence: Weidong Wu, d2VpMTMxODEyM0AxNjMuY29t
Disclaimer: All claims expressed in this article are solely those of the authors and do not necessarily represent those of their affiliated organizations, or those of the publisher, the editors and the reviewers. Any product that may be evaluated in this article or claim that may be made by its manufacturer is not guaranteed or endorsed by the publisher.
Research integrity at Frontiers
Learn more about the work of our research integrity team to safeguard the quality of each article we publish.