- 1Neurology Department, Allegheny Health Network, Pittsburgh, PA, United States
- 2Neurology Department at Clinical Hospital of Medical School of University of Sáo Paulo, Sáo Paulo, Brazil
- 3Medical Department of Athletes Union of Sáo Paulo, Sáo Paulo, Brazil
- 4Neurolign USA LLC, A Subsidiary of Neurolign Technologies Inc., Pittsburgh, PA, United States
Objective: An alarming proportion (>30%) of patients affected by SARS-CoV-2 (COVID-19) continue to experience neurological symptoms, including headache, dizziness, smell and/or taste abnormalities, and impaired consciousness (brain fog), after recovery from the acute infection. These symptoms are self-reported and vary from patient to patient, making it difficult to accurately diagnose and initiate a proper treatment course. Objective measures to identify and quantify neural deficits underlying the symptom profiles are lacking. This study tested the hypothesis that oculomotor, vestibular, reaction time, and cognitive (OVRT-C) testing using eye-tracking can objectively identify and measure functional neural deficits post COVID-19 infection.
Methods: Subjects diagnosed with COVID-19 (n = 77) were tested post-infection with a battery of 20 OVRT-C tests delivered on a portable eye-tracking device (Neurolign Dx100). Data from 14 tests were compared to previously collected normative data from subjects with similar demographics. Post-COVID subjects were also administered the Neurobehavioral Symptom Inventory (NSI) for symptom evaluation.
Results: A significant percentage of post COVID-19 patients (up to 86%) scored outside the norms in 12 out of 14 tests, with smooth pursuit and optokinetic responses being most severely affected. A multivariate model constructed using stepwise logistic regression identified 6 metrics as significant indicators of post-COVID patients. The area under the receiver operating characteristic curve (AUC) was 0.89, the estimated specificity was 98% (with cutoff value of 0.5) and the sensitivity was 88%. There were moderate but significant correlations between NSI domain key variables and OVRT-C tests.
Conclusions: This study demonstrates the feasibility of OVRT-C testing to provide objective measures of neural deficits in people recovering from COVID-19 infection. Such testing may serve as an efficient tool for identifying hidden neurological deficits post COVID-19, screening patients at risk of developing long COVID, and may help guide rehabilitation and treatment strategies.
Introduction
Two years into the global SARS-CoV-2 (COVID-19) pandemic, researchers and clinicians have been confronted with the long-term effects of the infection. Consistent with other coronaviruses, there is growing evidence the virus can cause damage to the central nervous system in addition to its well-known respiratory complications [(1, 2), see (3) and (4) for reviews]. This evidence became apparent early in the pandemic when clinicians worldwide reported neurological symptoms in a high proportion of infected patients; for example, up to 80% of hospitalized patients experience neurological manifestations at some point during disease progression (5, 6). Headache, dizziness, smell and/or taste abnormalities, and impaired consciousness (or “brain fog”) are the most reported neurological symptoms of COVID-19 (7).
These symptoms may be consequences of glial and neural cells in the nervous system expressing angiotensin converting enzyme-2 (ACE-2) receptors, through which the virus enters the body, rendering the brain directly vulnerable to the virus during infection (8, 9). Indeed, viral proteins and RNA have been found in the central nervous system (CNS) of deceased patients at autopsy, in additional to neuropathological findings such as notable inflammation of the brainsteam and damage to the medulla [(10, 11); but see (12) for cases of neuropathological findings in deceased patients with no viral RNA in cortical tissue]. COVID-19 infection can also affect the brain indirectly by causing encephalitis, thrombosis, stroke, coma, and/or hypoxia (6, 13–15). An emerging theme across multiple studies is that immune system hyperactivation may underly much of COVID-19's neurological effects [see (3) and (16) for reviews].
Data have shown that adverse neurological symptoms persist in a significant proportion of patients following recovery, a condition colloquially referred to as “long COVID” and categorized under names such as “post-acute sequelae of COVID-19 syndrome (PASC),” among others. Some reports put the incidence of PASC at approximately one third of infected patients (17–19). In patients that did not require hospitalization, the estimates range from 10–35%; however, in patients that required hospitalization that estimate rises to approximately 85% (20). In one study, the most common enduring symptoms included fatigue, headache, dyspnea, and anosmia (21). Another survey of 3,762 patients, taken 28 days to several months after presumed or confirmed COVID-19 infection, verified these symptoms but also revealed cognitive impairment, memory loss, sleep disruption, and dizziness/vertigo/balance issues as frequent neuropsychiatric symptoms (22).
Much like acute COVID-19 infection, these neurological symptoms of PASC are highly variable among patients, presenting a challenge for precise classification of long COVID as a specific disorder. Additionally, it is possible that neurological damage caused by COVID-19 infection may manifest in subtler neurological deficits of which patients may be unaware. Indeed, as a group, even recovered patients reporting no ongoing COVID-19 symptoms have significant cognitive deficits when compared to healthy controls (23).
As with other fatigue-related syndromes that are identified primarily by patient self-report of symptoms (e.g., chronic fatigue syndrome), objective measures add valuable quantification that otherwise very important symptom profiles lack. To address the need for such measures in COVID-19 survivors, we investigated how recovered patients perform on a comprehensive battery of oculomotor, vestibular, reaction time, and cognitive (OVRT-C) tests, as assessed by high-resolution video oculography (VOG). OVRT-C test metrics are quantifiable and objective proxies and biomarkers of neural function that have been used or investigated in patients with neurological damage due to injury or disease. For example, OVRT-C-based classification models can reliably identify concussion in high school athletes (24), and similar methods have shown promising results in adults with concussion (25). Abnormal oculomotor behaviors have also been proposed as potential biomarkers for several diseases, such as impaired convergence in Parkinson's disease (26), visual paired comparison task performance in patients with mild cognitive impairment (MCI); (27), atypical saccades in preclinical and early-stage Huntington's disease (28, 29), and multiple oculomotor deficits in multiple sclerosis [see (30) for review]. Therefore, OVRT-C testing using eye-tracking technology provides a unique tool to assess overall neural function, while objectively homing in on specific abnormalities that may be affected by COVID-19 infection.
Two recent studies tested the hypotheses that traditional oculomotor and vestibular tests, respectively, can identify neurological abnormalities in recovered COVID-19 (post-COVID) patients. Results of an eye-tracking study suggested that 9 COVID-19-recovered patients exhibited abnormal latency for centrally directed saccades, impaired memory-guided saccades, and increased latencies for antisaccades (31). In the second study, recovered patients had more asymmetries in their vestibular responses, particularly in ocular and cervical vestibular myogenic evoked potentials (oVEMP and cVEMP), and showed a low gain in the video head impulse test (vHIT), suggesting the audio-vestibular system can be damaged, at least temporarily, by COVID-19 infection (32). These reports are consistent with a longitudinal study that detected brain structure changes in previously infected patients compared to controls, including tissue damage in areas associated with the primary olfactory cortex and a greater decrease in overall brain size (33).
In this study we extended these findings, adding measures of pursuit tracking, vergence, optokinetic nystagmus, gaze stability, and reaction time to the investigation of VOG performance in recovered COVID-19 patients. We administered a battery of OVRT-C tests to 77 COVID-19 patients following their recovery from the acute infection stage of the illness. These tests allowed us to identify specific OVRT-C metrics—and the overall pattern of deficits—that are uniquely characteristic to post-COVID patients. During their visit, participants also completed the Neurobehavioral Symptom Inventory (NSI) to assess their current neurological symptoms, and to determine whether any of these symptoms were associated with specific OVRT-C metrics.
Materials and methods
Participants
All research activities were conducted according to the principles expressed in the Declaration of Helsinki and were approved by the Institutional Review Boards (IRB) at the sites where research was performed. Participants were 77 adults aged 18–45 years of age (see demographics in Table 1) from three groups recruited from three testing sites: (a) Allegheny Health Network, Pittsburgh, PA, USA (IRB#2020-367), n = 20; (b) University of São Paulo School of Medicine, São Paulo, Brazil, n = 19 and (c) São Paulo School Athletes Union, Brazil n = 38 (IRB#33787520.4.0000.0068). Subjects tested at the São Paulo School Athletes Union were primarily amateur and professional athletes (n = 38; Cohort 2), while subjects from the Allegheny Health Network and University of São Paulo School of Medicine were patients with no specific athletic categorization (n = 39; Cohort 1).
All participants reported having been diagnosed with and recovered from the acute COVID-19 infection but experienced persistent and function-impairing symptoms at least 4 weeks after initial infection. None of the subjects reported being hospitalized due to their COVID-19 infection. Each participant gave informed consent prior to testing. Exclusion criteria included those conditions and/or diseases that could influence the oculomotor or vestibular systems (e.g., a history of brain injury, vestibular disorders, blurred vision, or seizures). Special populations including children under 18 years of age, pregnant women, and individuals with diminished capacity (e.g., intellectual disability, dementia) were also excluded from this study. All three sites utilized the same inclusion/exclusion criteria, which are described in Table 2.
For the control population we used previously collected and published data (34, 35).
Battery of tests
Subjects were administered a battery of 20 OVRT-C tests (see Table 3 for a list of tests with output metrics and length for each test). Of these, the Gaze Horizontal, Spontaneous Nystagmus, Subjective Visual Vertical, and Subjective Visual Horizontal tests assess vestibular function. All stimuli were presented at high contrast on a neutral background with no distracting patterns. Tests were presented consecutively and ranged from 7 to 90 seconds in duration, for a total testing time of approximately 15 min. Subjects were seated comfortably adjacent to the test administrator throughout testing and their heads were not stabilized.
Hardware and software
OVRT-C tests were delivered via the Neurolign Dx 100 (formerly known as I-Portal Portable Assessment System - Nystagmograph; I-PAS), an FDA-cleared eye-tracking device manufactured by Neurolign USA, LLC (formerly known as Neuro Kinetics, Inc.; Pittsburgh, PA). The Neurolign Dx 100 is a portable and compact head-mounted virtual reality goggle set equipped with high-speed digital infrared cameras (940 nm, sampling rate of 100 frames/second) that capture high-resolution images of eye movements in response to light and auditory stimuli. A hand-held apparatus with response buttons recorded participants' reaction times and/or responses during tests that require manual input (i.e., subjective visual vertical/horizontal, auditory reaction time). Data were collected using Neurolign's I-Portal software, which captures time stamps (necessary for synchronization) and analyzes digital images of the eye to record horizontal and vertical eye movement data. Proprietary VEST™ software was utilized to operate the hardware, create and edit stimulus parameters, integrate I-Portal eye-tracking results, and analyze acquired data to generate a comprehensive set of desired metrics.
Treatment of artifacts and outlying samples
Data were calibrated for position by comparing eye movements to fixation locations that had a known displacement. VEST™ software identified artifacts such as blinks, recording noise, and any temporary failures in eye tracking. Along with other possible artifacts, such as shifting of goggles during testing, incorrect responses, and/or responses not related to the task, all artifacts were evaluated manually to ensure discrimination of eye movement signals from recording noise. In some cases, manual analysis was also necessary to isolate saccadic activity from pursuit activity. VEST™ software automatically reports data validity, i.e., the percentage of acceptable data available from which to calculate results. The software alerted the test administrator if data validity was below 60%, in which case VEST™ only analyzed data from a single eye as long as that eye was above the 60% validity criterion. If validity for both eyes was below 60%, data from that particular test were discarded. For some subjects, results from specific OVRT-C tests were removed from analysis when the data quality was inadequate for accurate measurement or created analytic errors.
Data analysis
Data acquired for each OVRT-C test were reviewed to ensure completion and validity, and analyzed using VEST™ software.
For each completed and validated test for every subject, measures of OVRT-C performance were collected from the VEST™ software. These measures corresponded to the “Metrics measured for each test” in Table 3, and “Metrics” in Table 4. For a given subject, each of their collected metric measurements were compared to the normative range for that metric in a clinical (FDA-approved) normative database (34, 35). We then determined the number of subjects whose measures fell within vs. outside the normative range for that metric. These are reported as percentage (%) abnormalities for each variable. Since the normative ranges were based on a 95th percentile ranking (95% of the normative database subjects were within each range), the expected percentage outside each range (% Abnormalities) would be 5% for an unaffected subject population. Our results are therefore meant to be interpreted relative to this expected 5%.
In some cases, we report % Abnormalities for a whole test (e.g., Optokinetic Nystagmus at 60 deg/sec, see Figure 1), where the whole test consists of multiple metrics. Nonetheless, testing for the significance of differences was performed per metric, not on a whole-test basis.
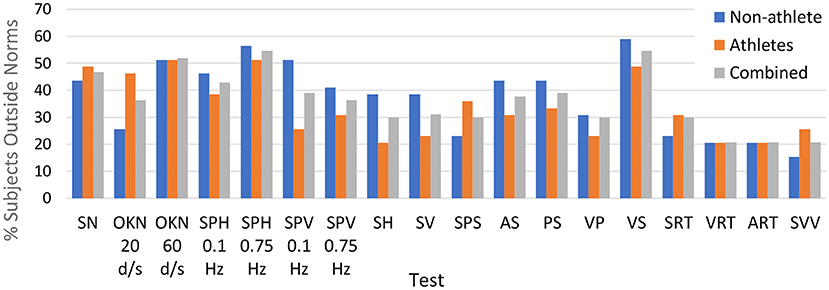
Figure 1. Percentage of subjects in each cohort that had one or more metrics outside normative ranges for each of the tests in our testing protocol.
A Two-sample t-test was used to evaluate the similarity between our two patient cohorts (TTEST2 function in MATLAB). Differences between the observed rate of metric measures that fell outside normative ranges vs. the expected (normative) rate were evaluated using a One-Proportion Z-test. The significances of these differences (given in Table 5) were computed by
where c = 1/(2N) is a correction for continuity and p is the estimated proportion of post-COVID participants whose test value is outside the normative 95% reference interval (RI) limits (column %Abn), N is the total number of post-COVID participants, and P0 = 0.05 is an expected proportion. The corresponding p-value was calculated under a two-tail hypothesis. Because we are performing multiple comparisons, each targeting essentially the same null hypothesis (the proportion of subjects with measures outside the normative range is P0), we have adjusted our acceptable significance threshold to α <0.0009 (Bonferroni correction).
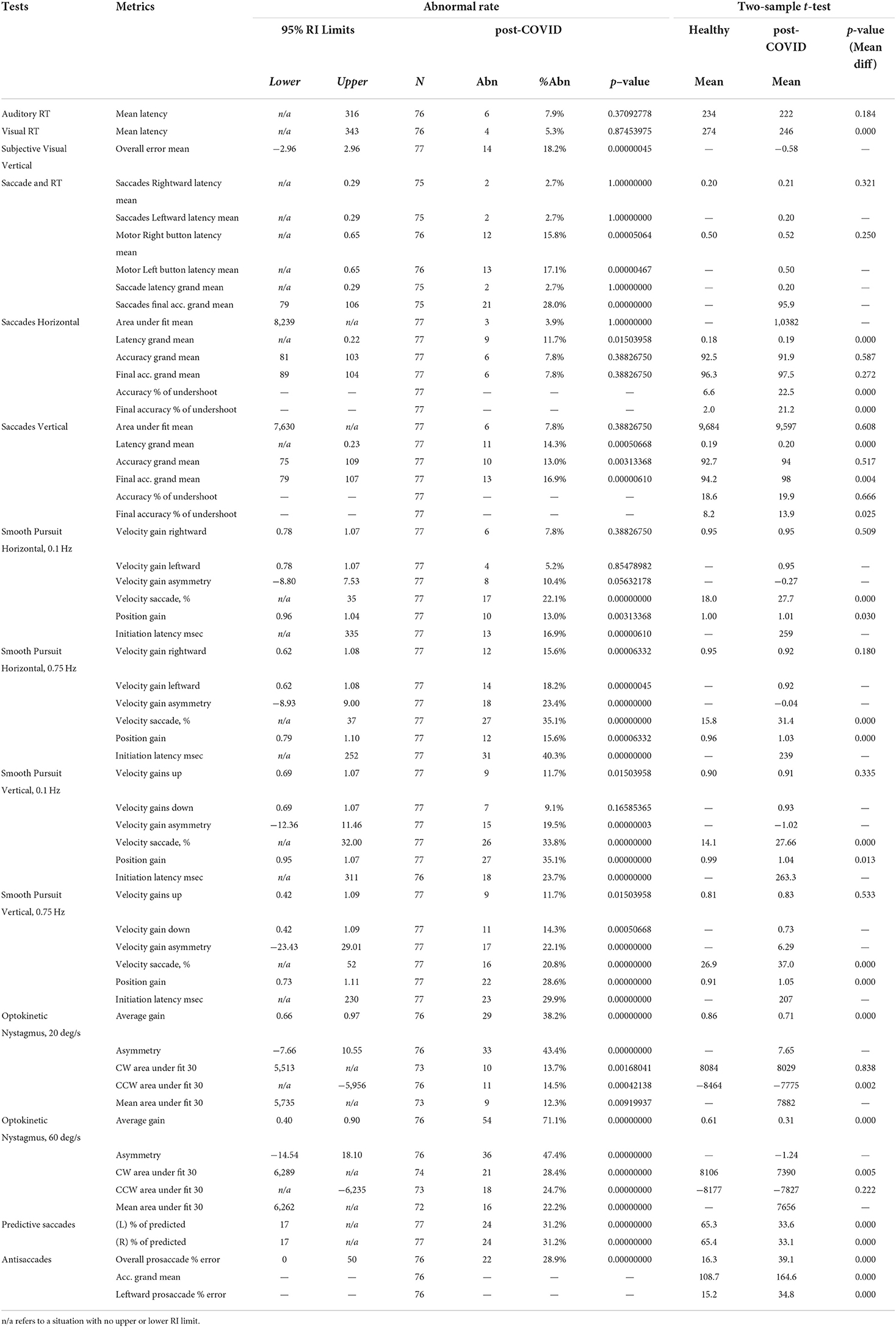
Table 5. Abnormal rates among post-COVID participants and t-test examine the mean difference between post-COVID and healthy participants.
A Two-Sample t-test was used to determine whether there is a difference between the mean (test) value of two independent groups – post-COVID 72–77 subjects and 300 healthy (control) participants. The corresponding p-value (mean difference) was calculated under a two-tail hypothesis.
We evaluated the value of our test metrics as predictors of COVID-19 status using a series of univariate and multivariate regression models. A logistic regression model was used to estimate the probability of a binary response (COVID / not COVID) as a function of one or more independent metrics. Models were generated using data from the normative database (300 normative data subjects) and 75 of our 77 subjects. We first fit logistic regression coefficients separately for each of 28 OVRT test metrics (FITGLM function in MATLAB using the method of Maximum Likelihood; see Table 6). A multivariate logistic regression model was generated and tested for its ability to distinguish between post-COVID and healthy control participants (evaluated on training data without a separate validation population). A standard stepwise procedure was used to identify those metrics that best fit the model. To evaluate the diagnostic accuracy, in addition to AUC and Somers' D, sensitivity, specificity and overall accuracy was computed with a logistic function cutoff value of 0.5.
Neurobehavioral symptom inventory
The Neurobehavioral Symptom Inventory (NSI) is a 22-item self-report questionnaire commonly administered to patients following traumatic brain injury (TBI). Each item lists a potential neurological symptom and subjects were asked to rate how much each symptom was disturbing them at time of test on a scale of 0 (none/not at all) to 4 (always/very severe), with a total possible score of 88. A recent factor structure analysis of NSI responses by US military members with mTBI and healthy National Guard members (no mTBI) revealed a 4-factor model was the best fit, grouping responses into 4 neurobehavioral domains: vestibular, somatic, cognitive, and affective (36).
Spearman's rank correlation coefficient (rho) was used to measure the relationship between the test variables and NSI symptoms (CORR function in MATLAB); see Table 8.
Results
Comparison to normative OVRT-C ranges
In this study, we examined the hypothesis that OVRT-C test responses are reliable neurological biomarkers that can be used in the identification and evaluation of post-COVID/PASC patients. Using a clinical (FDA-approved) database of normative OVRT-C values (34, 35) we tested the specific hypothesis that in this population, multiple OVRT-C measures will fall outside established normative ranges.
For each test in our protocol, we identified which subjects had abnormal (outside normative ranges) responses to one or more variables within that test. Figure 1 displays a summary of these abnormality percentages within Cohort 1 (non-athletes), Cohort 2 (athletes), and overall (pooled data). As the figure shows, OVRT-C abnormalities were distributed relatively evenly between the two cohorts. Some tests presented clear challenges for subjects of either cohort. For instance, over half of Cohort 1 and Cohort 2 had impaired (outside norms) performance on the high-speed optokinetic test.
As both cohorts showed deficits, we first evaluated whether there were differences between these two cohorts (Table 4). We found that many metrics were not significantly different (60 out of 68), with the exception of several metrics: overall mean error in the SVV test, saccade latency in all saccade tests, mean inward correlation in the vergence test, clockwise and mean area under the fit in the OKN 20deg/s test, and average gain in the OKN 60 deg/s test. Based on the lack of significant differences, we pooled the two cohorts to further evaluate the deficits by comparing them to normative data. This allowed us to increase the sample size to 77 for a more robust comparison with the 300 controls included in the normative data.
We further examined the individual variables making up each test in more detail in order to highlight where performance difficulties arose (Figure 2, Table 5).
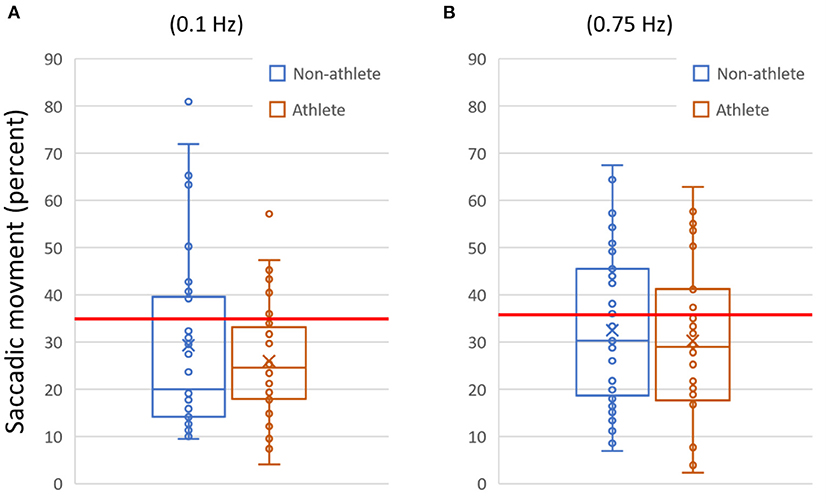
Figure 2. Example data plotted for two variables. (A) The amount of saccadic (aberrant non-pursuit) activity measured for each subject during the slow (0.1 Hz) horizontal smooth pursuit test. (B) Saccadic activity for the fast (0.75 Hz) horizontal smooth pursuit test. In (A) and (B), data are shown as quartile box and whisker plots (with outliers visible). The horizontal line indicates the 95% reference interval for the metric from the normative database (≥35% for 0.1 Hz, ≥37% for 0.75 Hz). In (A), saccadic activity above the threshold was present in 22.1% of participants (N = 17/77; 0.1 Hz). In (B), saccadic activity above the threshold was present in 35.1% (N = 27/77; 0.75 Hz).
Table 5 provides a list of multiple OVRT-C variables, the observed frequencies of measures outside respective normative ranges for our subject population (pooled data), and an indication of significance based on our acceptance threshold of α <0.0009 (by One-Proportion Z-test). This analysis revealed that a significant percentage of subjects scored outside the norms in 12 out of 14 tests and 41 out of 60 metrics. The tests showing the highest percentage of subjects scoring outside the norms for all or many test metrics were smooth pursuit and OKN. The smooth pursuit tracking function revealed multiple significant differences, especially for higher speeds (SPH, 0.75 Hz) and for vertical pursuit tracking (SPV, 0.1 Hz and 0.75 Hz). Figure 2 shows the saccadic component or the amount of saccadic activity during smooth pursuit horizontal at 0.1 Hz and 0.75 Hz for all subjects. Given that the normative thresholds represent the 95th percentile for the normative population, we would expect that approximately 5% of our subjects would exceed each threshold if patients' post-COVID status had no effect on smooth pursuit activity. However, as shown in Figure 2, the number of subjects with measures beyond the respective thresholds was significantly higher in both cases with 22.1% (N = 17/77) for 0.1 Hz (p < 0.0009 by One-Proportion Z-test) and 35.1% (N = 27/77) for 0.75 Hz (p < 0.0009). These results indicate that a significant number of post-COVID patients have abnormally increased saccadic movement, and therefore less smooth pursuit movement, compared to a normative population.
Optokinetic nystagmus (OKN) responses, which rely on both saccade-like and pursuit-like eye movements, were dramatically affected in our post-COVID subjects (Table 5). Among the OKN responses, 71.1% (N = 54/76; p < 0.0009) had abnormally low gain for the high speed OKN test, meaning that post-COVID patients were impaired in their ability to generate higher speed nystagmus movements (the slow phase of OKN was significantly slower than the normative velocity).
Notably, neither standard horizontal saccade tests nor standard manual reaction time tests revealed large differences, as shown by the low proportions of patients scoring outside normative ranges on the Auditory Reaction Time (ART), Visual Reaction Time (VRT), or Saccades Horizontal tests (Table 5). However, when saccades and manual reaction time were combined within a joint test requiring both responses in conjunction, significant differences in reaction time emerged (Saccades and Reaction Time test, Motor resp R and L button latency, Table 5). Also, in the vertical saccades test, significant differences were seen for latency and for one of the measures of accuracy (Final accuracy grand mean).
Lastly, while standard saccades were not strongly impaired, other tests showed a significantly impaired ability to generate timed, anticipatory saccades (Predictive Saccades, N = 24/77, p < 0.0009), and saccades going in the opposite direction from presented stimuli (Antisaccades, N = 22/76, p < 0.0009).
Predictive metrics
To examine the ability of our testing protocol to identify PASC in a patient population, we constructed several logistic regression models. Some of these were simple regressions of single variables (univariate), followed by a more complex model using multiple metrics as combined predictors of impairment (multivariate). We constructed the models using our combined subject cohorts (n = 77) and the controls (n = 300) from the normative database. As a measure of discriminability, for each model we computed the area under the Receiver Operating Characteristics (ROC) curve (AUC).
Univariate analyses identified 11 metrics that could discriminate between controls (healthy subjects) and post-COVID status (Table 6). These metrics largely agreed with the normative range comparison results (Table 5). As shown in Table 6, high-speed OKN gain was strongly predictive of post-COVID subject status (AUC = 0.82). For both horizontal and vertical slow and fast smooth pursuit, the amount of aberrant saccadic activity was more moderately but still significantly related to post-COVID subject status (AUC values ranging from 0.64 to 0.73). Predictive saccade metrics were also significantly predictive (AUC = 0.77 for our two predicted percent measures), as were antisaccade metrics (e.g., AUC = 0.69 for error percent). One notable difference from our normative range comparison results was that univariate models for horizontal saccade metrics were significantly predictive of subject status with, for example, an AUC of 0.79 for one measure of saccade hypometria (final accuracy % of undershoot, see Table 6), and an AUC of 0.68 for latency. This latency result suggests a correlation between saccadic timing and post-COVID health status, which is significant, but which is not revealed by comparison to normative thresholds alone.
The multivariate model was constructed using stepwise logistic regression (Table 7). The analysis identified six metrics from different tests as significant indicators of post-COVID subjects. Together, the AUC was 0.89, the estimated specificity was 98% (with cutoff value of 0.5) and the sensitivity was 88%.
Neurobehavioral symptom inventory
Of the 77 participants, 64 completed the NSI. For each NSI domain (i.e., vestibular, somatic, cognitive, and affective), we determined the maximum aggregate score per subject for each domain and overall (e.g., as the maximum score for each NSI item is 4, the aggregate score for each domain is 4*(number of NSI items in domain). We then calculated the mean score per domain for each cohort (athletes, non-athletes) and overall (pooled) (Figure 3).
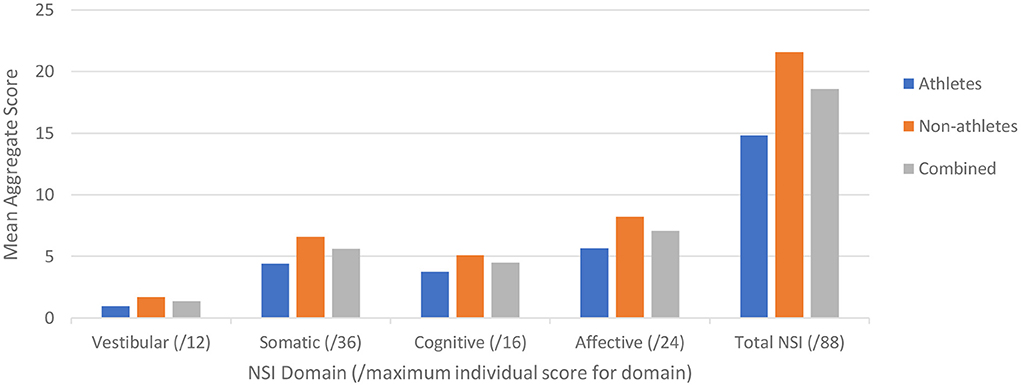
Figure 3. Neurobehavioral Symptom Inventory (NSI) scores plotted for the two cohorts (blue – Cohort 1, orange – Cohort 2, gray – combined) for each NSI domain as identified by Vanderploeg et al. (36), with the maximum score for each domain in the respective parentheses.
We then examined the relationship between symptom scores and OVRT-C metrics (Table 8). There were moderate but significant correlations between NSI domain key variables and OVRT-C tests. For example, the NSI cognitive domain correlated with Antisaccade errors (Decisions, rho = 0.36, p = 0.003), Vertical Saccades accuracy (Forgetfulness, rho = −0.33, p = 0.001), and Self-Paced Saccade rate (Decisions, rho = −0.36, p = 0.004). Vertical saccade accuracy was also correlated modestly with the NSI vestibular domain Coordination variable (% saccade undershoot, rho = 0.39, p = 0.001). Spontaneous nystagmus showed correlation with two cognitive domain measures, namely Forgetfulness (rho = −0.41, p = 0.002) and Concentration (e.g., for average slow phase velocity, rho = −0.53, p = 0.002).
Discussion
The SARS-CoV-2 pandemic has brought public and clinical attention to an area of growing concern regarding viral infections, namely that a patient's emerging from the acute phase of infection and illness does not always imply recovery. This was seen, for instance, with the SARS coronavirus infections of 2002–2004 (SARS-CoV) where a sizeable proportion of patients did not recover their pre-infection health status, and instead entered a chronic period of impairment characterized by fatigue, myalgia, chronic pain, and other factors contributing to significant disability (37). COVID-19 infection has demonstrated a similar pattern where, despite some patients showing complete acute recovery, a significant proportion of patients experience ongoing symptoms months after the acute period of infection (21, 22).
It is now clear that neurological issues feature commonly and prominently in the post-infection period for recovered COVID-19 patients. While fatigue is the most reported symptom, this is followed closely in prevalence by patient-reported impairments in concentration, memory issues, problems with mood and emotion, sleep disruption, “brain fog” and other cognitive disruptions, and dizziness (22). Indeed, our NSI data support these findings, with cognitive (e.g., concentration) and affective (e.g., anxiety) complaints being the most prevalent and severe.
The OVRT-C results reported here reinforce the conclusion that COVID-19—in addition to its post-infection sequelae—is a neurological condition as much as it is a respiratory and autonomic one [see (3, 38) for reviews], and is consistent with two recent studies (31, 32). The most important contribution of VOG measurements is that they do not simply report on the state of oculomotor systems, but rather these metrics are quantifiable and objective proxies and biomarkers for the overall state of brain health. Our results reflect clear CNS impairment in recovered COVID-19 patients and provide support for three important hypotheses. First, we observed that subjects demonstrated a diversity of impairments, for instance in smooth pursuit tracking, saccades, and optokinetic nystagmus responses. Each of these reflect different anatomical substrates, which implies COVID-19 infection can chronically affect a broad range of neuroanatomical territory. Second, there was notable heterogeneity in OVRT-C and NSI data across participants, supporting the hypothesis that chronic COVID-19 neurological effects are themselves heterogeneous, leading to an equally diverse presentation of post-infection symptoms. Lastly, the oculomotor dysfunction we observed implies that COVID-19 infection can lead to significant disability well beyond, and perhaps hidden by, the more prevalent complaints of fatigue, cognitive under-performance, and memory impairment.
A critical finding was that logistic regression analysis using six OVRT-C variables across multiple tests demonstrated excellent discrimination between recovered COVID-19 subjects and controls, with a sensitivity of 88% and specificity of 98%. These data suggest that despite the high degree of heterogeneity of our subjects' reported neurological symptoms following recovery, COVID-19 infection resulted in an identifiable and informative collection of specific OVRT-C deficits in our subjects at the group level. The strength of this pattern may vary based on factors such as disease severity, vaccination status, comorbid disorders, and/or patient age; however, recent evidence suggests that even mild COVID-19 infection is associated with structural abnormalities following recovery (33). This model can be used as a quick screening tool of post-COVID patients to identify who might be at risk of PASC/long COVID and could benefit from immediate treatment.
ME/CFS and syndromes of chronic fatigue
The mechanisms behind neurological effects of COVID-19 infection are unknown. The etiology and symptoms of recovered COVID-19 patients, however, bear a remarkable resemblance to those of another condition; namely, myalgic encephalomyelitis/chronic fatigue syndrome (ME/CFS). Not only do the presenting symptoms strongly overlap, such as fatigue, post-exertional malaise (PEM), cognitive and memory difficulties, autonomic issues, among others [see (39), for review], but it has been noted that cases of ME/CFS are frequently preceded by instances of infection (40). Equally important, post-infectious fatigue syndromes can result from several viruses, such as SARS-CoV (37), the Epstein-Barr virus (EBV), Coxiella burnetii, and Ross River virus (41). Evidence is accumulating that post-COVID symptoms and ME/CFS both result from immunological hyperactivation, whereby an overwhelming activation of immune responses during an acute infectious period leaves the immune system chronically damaged, with long-term consequences for multiple systems that are normally dependent upon proper immune system balance (16, 42, 43). Another compatible hypothesis is that both conditions correlate with brainstem dysfunction, e.g., in medullary regions that are normally responsible for respiration, vasoconstriction, and vestibular responses, among many other functions [e.g., (11); see (42) for review]. While the present study did not aim to identify etiological mechanisms or specific impaired neural targets, the similarities between disorders suggest that long COVID may share many physiological mechanisms with, is etiologically related to, or is in fact the same as ME/CFS, which are hypotheses that have been proposed or assumed by a number of researchers familiar with both conditions (39, 44, 45).
Importantly, even if post-COVID sequelae and other fatigue-dominated syndromes are eventually shown to be distinct in some of their physiological details, the results of VOG testing from one condition can still valuably inform the other, particularly with regard to fundamental relationships between fatigue, cognitive impairment, autonomic dysfunction, and quantifiable metrics of detailed brain functioning, such as those that OVRT-C/VOG testing provide (46). This may prove particularly beneficial when attempting to understand virally initiated fatigue syndromes generally. We also believe it worth noting that our results address one of the valuable lessons learned from the clinical history of ME/CFS, namely that objective measurements are a valuable complement to subjective experience in verifying the individual presence of, and even refuting doubts about the reality of, a disorder otherwise defined largely by subjective symptoms (47).
Data availability statement
The raw data supporting the conclusions of this article will be made available by the authors, without undue reservation.
Ethics statement
The studies involving human participants were reviewed and approved by Allegheny Health Network Institutional Review Board (IRB#2020-367), University of Sáo Paulo School of Medicine Institutional Review Board (IRB#33787520.4.0000.0068). The patients/participants provided their written informed consent to participate in this study.
Author contributions
KK and AKi: co-principal investigator, study design, and manuscript review. RAn: co-principal investigator, data interpretation, and neuroclinical evaluation. AKu: manuscript review and project design. RAs and LG: wrote manuscript and study design. AS: screened, recruited, and enrolled patients. LM: tested patients. AA: tested patients and organized data. RS: study design and background research. SB and RT: neuroclinical evaluation. AB: statistical analysis. All authors contributed to the article and approved the submitted version.
Funding
The authors declare that this study received funding from Neurolign International Inc. The funder was not involved in the study design, collection, analysis, interpretation of data, the writing of this article or the decision to submit it for publication.
Conflict of interest
Author AKi is the VP Technology Development, employee of, and shareholder of Neurolign USA LLC. Author RAs is an employee of and shareholder of Neurolign USA LLC. Author LG is an employee of Neurolign USA LLC. Author RAn received funding support from Neurolign USA LLC.
The remaining authors declare that the research was conducted in the absence of any commercial or financial relationships that could be construed as a potential conflict of interest.
Publisher's note
All claims expressed in this article are solely those of the authors and do not necessarily represent those of their affiliated organizations, or those of the publisher, the editors and the reviewers. Any product that may be evaluated in this article, or claim that may be made by its manufacturer, is not guaranteed or endorsed by the publisher.
References
1. Rogers JP, Chesney E, Oliver D, Pollak TA, McGuire P, Fusar-Poli P, et al. Psychiatric and neuropsychiatric presentations associated with severe coronavirus infections: a systematic review and meta-analysis with comparison to the COVID-19 pandemic. Lancet Psychiatry. (2020) 7:611–27. doi: 10.1016/S2215-0366(20)30203-0
2. Román GC, Spencer PS, Reis J, Buguet A, Faris MEA, Láinez M, et al. The neurology of COVID-19 revisited: a proposal from the environmental neurology specialty group of the world federation of neurology to implement internation neurological registries. J Neurol Sci. (2020) 414:1–12. doi: 10.1016/j.jns.2020.116884
3. Al-Ramadan A. Rabab'h O, Shah J, Gharaibeh A. Acute and post-acute neurological complications of COVID-19. Neurol Int. (2021) 13:102–19. doi: 10.3390/neurolint13010010
4. Peterson CJ, Sarangi A, Bangash F. Neurological sequelae of COVID-19: a review. Egypt J Neurol Psychiatr Neurosurg. (2021) 57:122. doi: 10.1186/s41983-021-00379-0
5. Liotta EM, Batra A, Clark JR, Shlobin NA, Hoffman SC, Orban ZS, et al. Frequent neurologic manifestations and encephalopathy-associated morbidity in COVID-19 patients. Ann Clin Transl Neurol. (2020) 7:2221–30. doi: 10.1002/acn3.51210
6. Chou SH-Y, Beghi E, Helbok R, Moro E, Sampson J, Altamirano V, et al. Global incidence of neurological manifestations among patients hospitalized with COVID-19 – a report for the GCS-NeuroCOVID Consortium and the ENERGY Constortium. JAMA Network Open. (2021) 4:e2112131.
7. Chen X, Laurent S, Onure OA, Kleineberg NN, Fink GR, Schweitzer F, et al. A systematic review of neurological symptoms and complications of COVID-19. J Neurol. (2021) 268:392–402. doi: 10.1007/s00415-020-10067-3
8. Baig AM, Khaleeq A, Ali U. Syeda H. Evidence of the COVID-19 virus targeting the CNS: tissue distribution, host-virus interaction, and proposed neurotropic mechanisms. ACS Chem Neurosci. (2020) 11:995–8. doi: 10.1021/acschemneuro.0c00122
9. Samavati L, Uhal BD. ACE2, much more than just a receptor for SARS-CoV-2. Front Cell Infect Microbiol. (2020) 10:1–9. doi: 10.3389/fcimb.2020.00317
10. Matschke J, Lütgehetmann M, Hagel C, Sperhake JP, Schröder AS, Edler C, et al. Neuropathology of patients with COVID-19 in Germany: a post-mortem case series. Lancet Neurol. (2020) 19:919–29. doi: 10.1016/S1474-4422(20)30308-2
11. Bulfamante G, Bocci T, Falleni M, Campiglio L, Coppola S, Tosi D, et al. Brainstem neuropathology in two cases of COVID-19: SARS-CoV-2 trafficking between brain and lung. J. Neurol. (2021) 268:4486–91. doi: 10.1007/s00415-021-10604-8
12. Jensen MP, Le Quesne J, Officer-Jonest L, Teodòsio A, Thaventhiran J, Ficken C, et al. Neuropathological findings in two patients with fatal COVID-19. Neuropathol Appl Neurobiol. (2020) 47:17–25. doi: 10.1111/nan.12662
13. Siow I, Lee KS, Zhang JJY, Saffari SE, Ng A. Stroke as a neurological complication of COVID-19: a systematic review and meta-analysis of incidence, outcomes and predictors. J Stroke Cerebrovasc Dis. (2021) 30:105549. doi: 10.1016/j.jstrokecerebrovasdis.2020.105549
14. Hanff TC, Mohareb AM, Giri J, Cohen JB, Chirinos JA. Thrombosis in COVID-19. Am J Hematol. (2020) 95:1578–89. doi: 10.1002/ajh.25982
15. Cavezzi A, Troiani E, Corrao S. COVID-19: hemoglobin, iron, and hypoxia beyond inflammation. A narrative review. Clin Pract. (2020) 10:1271. doi: 10.4081/cp.2020.1271
16. Andrade BS, Siqueira S, de Assis Soares WR, de Souza Rangel F, Santos NO, dos Santos Freitas A, et al. Long-COVID and post-COVID health complications: an up-to-date review on clinical conditions and their possible molecular mechanisms. Viruses. (2021) 13:700. doi: 10.3390/v13040700
17. Taquet M, Geddes JR, Husain M, Luciano S, Harrison PJ. 6-month neurological and psychiatric outcomes in 236379 survivors of COVID-19: a retrospective cohort study using electronic health records. Lancet Psychiatry. (2021) 8:416–27. doi: 10.1016/S2215-0366(21)00084-5
18. Logue JK, Franko NM, McCulloch DJ, McDonald D, Magedson A, Wolf CR, et al. Sequelae in adults at 6 months after COVID-19 infection. JAMA Network Open. (2021) 4:e210830. doi: 10.1001/jamanetworkopen.2021.0830
19. Teneforde MW, Billig Rose E, Lindsell CJ, Shapiro NI, Files DC, Gibbs KW, et al. Characteristics of adult outpatients and inpatients with COVID-19. MMWR. (2020) 69:841–6. doi: 10.15585/mmwr.mm6926e3
20. Pavli A, Theodoridou M, Maltezou HC. Post-COVID syndrome: incidence, clinical spectrum, and challenges for primary healthcare professions. Arch Med Res. (2021) 52:575–81. doi: 10.1016/j.arcmed.2021.03.010
21. Sudre CH, Murray B, Varsavsky T, Graham MS, Penfold RS, Bowyer RC, et al. Attributes and predictors of long COVID. Nat Med. (2021) 27:626–31. doi: 10.1038/s41591-021-01292-y
22. Davis HE, Assaf GS, McCorkell L, Wei H, Low RJ, Re'em Y, et al. Characterizing long COVID in an international cohort: 7 months of symptoms and their impact. EclinicalMedicine. (2021) 38:101019. doi: 10.1016/j.eclinm.2021.101019
23. Hampshire A, Trender W, Chamberlain SR, Jolly AE, Grant JE, Patrick F, et al. Cognitive deficits in people who have recovered from COVID-19. EClinicalMedicine. (2021) 39:101044. doi: 10.1016/j.eclinm.2021.101044
24. Kelly KM, Kiderman A, Akhavan S, Quigley MR, Snell ED, Happ E, et al. Oculomotor, vestibular, and reaction time effects of sports-related concussion: video-oculography in assessing sports-related concussion. J Head Trauma Rehabil. (2018) 34:176–88. doi: 10.1097/HTR.0000000000000437
25. Balaban C, Hoffer ME, Szczupak M, Snapp H, Crawford J, Murphy S, et al. Oculomotor, vestibular, and reaction time tests in mild traumatic brain injury. PLoS ONE. (2016) 11:e0162168. doi: 10.1371/journal.pone.0162168
26. Kang SL, Shaikh AG, Ghasia FF. Vergence and strabismus in neurodegenerative disorders. Front Neurol. (2018) 9:1–10. doi: 10.3389/fneur.2018.00299
27. Crutcher MD, Calhoun-Haney R, Manzanares LJJ, Levey AI, Zola SM. Eye tracking during a visual paired comparison task as a predictor of early dementia. Am J Alzheimers Dis Other Demen. (2009) 24:258–66. doi: 10.1177/1533317509332093
28. Golding CVP, Danchaivijitr TL, Hodgson SJ, Kennard TC. Identification of an oculomotor biomarker of preclinical Huntington disease. Neurology. (2006) 67:485–7. doi: 10.1212/01.wnl.0000218215.43328.88
29. Hicks SL, Robert MPA, Golding CVP, Tabrizi SJ, Kennard C. Oculomotor deficits indicate progression of Huntington's disease. Prog Brain Res. (2008) 171:555–8. doi: 10.1016/S0079-6123(08)00678-X
30. Serra A, Chisari CG, Matta M. Eye movement abnormalities in multiple sclerosis: pathogenesis, modeling, and treatment. Front Neurol. (2018) 9:31. doi: 10.3389/fneur.2018.00031
31. Cena CG, Costa MC, Saltarén Pazmiño R, Santos CP, Gómez-Andrés D, Benito-León J, et al. Eye movement alterations in post-COVID-19 condition: a proof-of-concept study. Sensors. (2022) 22:1481. doi: 10.3390/s22041481
32. Tan M, Cengiz DU, Demir I, Demirel S, Çolak SC, Karakas O, et al. Effects of COVID-19 on the audio-vestibular system. Med Surg. (2022) 43:103173. doi: 10.1016/j.amjoto.2021.103173
33. Douaud G, Lee S, Alfar-Almagro F, Arthofer C, Wang C, McCarthy P, et al. SARS-CoV-2 is associated with changes in brain structure in UK Biobank. Nature. (2022) 604:697–707. doi: 10.1101/2021.06.11.21258690
34. Kullman A, Ashmore RC, Braverman A, Mazur C, Snapp H, Williams E, et al. Portable eye-tracking as a reliable assessment of oculomotor, cognitive and reaction time function: normative data for 18-45 year old. PLoS ONE. (2021) 16:e0260351. doi: 10.1371/journal.pone.0260351
35. Kullman A, Ashmore RC, Braverman A, Mazur C, Snapp H, Willians BS, et al. Normative data for ages 18-45 for ocular and vestibular testing using eye tracking. Laryngoscope Investig Otolaryngol. (2021) 6:1116–27. doi: 10.1002/lio2.632
36. Vanderploeg RD, Silva MA, Soble JR, Curtiss G, Belanger HG, Donnell AJ, et al. The structure of postconcussion symptoms on the neurobehavioral symptom inventory: a comparison of alternative models. J Head Trauma Rehabil. (2015) 30:1–11. doi: 10.1097/HTR.0000000000000009
37. Lam MH, Wing YK Yu MW, Leung C, Ma RCW, Kong APS, et al. Mental morbidities and chronic fatigue in severe acute respiratory syndrome survivors: long-term follow-up. Arch Intern Med. (2009) 169:2142–7. doi: 10.1001/archinternmed.2009.384
38. Larsen NW, Stiles LE, Miglis MG. Preparing for the long-haul: autonomic complications of COVID-19. Auton Neurosci. (2021) 235:102841. doi: 10.1016/j.autneu.2021.102841
39. Wong TL, Weitzer DJ. Long COVID and myalgic encephalomyelitis/chronic fatigue syndrome (ME/CFS)-a systemic review and comparison of clinical presentation and symptomatology. Medicina. (2021) 57:418. doi: 10.3390/medicina57050418
40. Chu L, Valencia IJ, Garvert DW, Montoya JG. Onset patterns and course of myalgic encephalomyelitis/chronic fatigue syndrome. Front Pediatr. (2019) 7:12. doi: 10.3389/fped.2019.00012
41. Hickie I, Davenport T, Wakefield D, Vollmer-Conna U, Cameron B, Vernon SD, et al. Post-infective and chronic fatigue syndromes precipitated by viral and non-viral pathogens: prospective cohort study. BMJ. (2006) 333:575. doi: 10.1136/bmj.38933.585764.AE
42. Yong SJ. Long COVID or post-COVID-19 syndrome: putative pathophysiology, risk factors, and treatments. Infect Dis. (2021) 53:737–54. doi: 10.1080/23744235.2021.1924397
43. Montoya JG, Holmes TH, Anderson JN, Maecker HT, Rosenberg-Hasson Y, Valencia IJ, et al. Cytokine signature associated with disease severity in chronic fatigue syndrome patients. Proc Natl Acad Sci USA. (2017) 114:E7150–8. doi: 10.1073/pnas.1710519114
44. Komaroff AL, Bateman L. Will COVID-19 lead to myalgic encephalomyelitis/chronic fatigue syndrome? Front Med. (2021) 7:606824. doi: 10.3389/fmed.2020.606824
45. Mirfazeli FS, Sarabi-Jamab A, Pereira-Sanchez V, Kordi A, Shariati B, Shariat SV, et al. Chronic fatigue syndrome and cognitive deficit are associated with acute-phase neuropsychiatric manifestations of COVID-19: a 9-month follow-up study. Neurol Sci. (2022) 43:2231–9. doi: 10.1007/s10072-021-05786-y
46. Badham SP, Hutchinson CV. Characterising eye movement dysfunction in myalgic encephalomyelitis/chronic fatigue syndrome. Graefes Arch Clin Exp Ophthalmol. (2013) 251:2769–76. doi: 10.1007/s00417-013-2431-3
Keywords: oculomotor, COVID-19, biomarker, eye-tracking, Neurobehavioral Symptom Inventory (NSI)
Citation: Kelly KM, Anghinah R, Kullmann A, Ashmore RC, Synowiec AS, Gibson LC, Manfrinati L, Araújo Ad, Spera RR, Brucki SMD, Tuma RL, Braverman A and Kiderman A (2022) Oculomotor, vestibular, reaction time, and cognitive tests as objective measures of neural deficits in patients post COVID-19 infection. Front. Neurol. 13:919596. doi: 10.3389/fneur.2022.919596
Received: 13 April 2022; Accepted: 09 August 2022;
Published: 12 September 2022.
Edited by:
Pedro Gomez-Vilda, Polytechnic University of Madrid, SpainReviewed by:
Jan Johansson, Karolinska Institutet (KI), SwedenAbdulmonem Alhayani, King Abdulaziz University, Saudi Arabia
Tommaso Bocci, University of Milan, Italy
Copyright © 2022 Kelly, Anghinah, Kullmann, Ashmore, Synowiec, Gibson, Manfrinati, Araújo, Spera, Brucki, Tuma, Braverman and Kiderman. This is an open-access article distributed under the terms of the Creative Commons Attribution License (CC BY). The use, distribution or reproduction in other forums is permitted, provided the original author(s) and the copyright owner(s) are credited and that the original publication in this journal is cited, in accordance with accepted academic practice. No use, distribution or reproduction is permitted which does not comply with these terms.
*Correspondence: L. C. Gibson, laura.gibson@neurolign.com; A. Kiderman, alex.kiderman@neurolign.com