- 1Faculty of Medicine Novi Sad, Department of Radiology, University of Novi Sad, Novi Sad, Serbia
- 2Vojvodina Institute of Oncology, Center for Diagnostic Imaging, Sremska Kamenica, Serbia
- 3Department for Biomedical Imaging and Image-guided Therapy, Medical University Vienna, Vienna, Austria
- 4Clinical Center of Vojvodina, Center for Radiology, Novi Sad, Serbia
- 5Department for Neuroscience, The Alfred Centre, Central Clinical School, Monash University, Melbourne, VIC, United States
- 6Imaging Genetics Center, Mark and Mary Stevens Neuroimaging and Informatics Institute, Keck School of Medicine, University of Southern California, Marina del Rey, CA, United States
- 7Faculty of Medicine Novi Sad, Department of Infectious Diseases, University of Novi Sad, Novi Sad, Serbia
- 8Clinical Center of Vojvodina, Clinic for Infectious Diseases, Novi Sad, Serbia
The current concept of brain aging proposes three gradient patterns of changes in white matter that occur during healthy brain aging: antero-posterior, supero-inferior, and the myelodegeneration-retrogenesis (or the “last-in-first-out”) concept. The aim of this study was to correlate white matter diffusivity measures (fractional anisotropy-FA, mean diffusivity-MD, radial diffusivity-RD, and axial diffusivity-AD) in healthy volunteers with chronological age and education level, in order to potentially incorporate the findings with proposed patterns of physiological brain aging. The study was performed on 75 healthy participants of both sexes, with an average age of 37.32 ± 11.91 years underwent brain magnetic resonance imaging (MRI) with diffusion tensor imaging (DTI). DTI was performed using tract-based spatial statistics (TBSS), with the analysis of four parameters: FA, MD, RD, and AD. Skeletonized measures were averaged in 29 regions of interest in white matter. Correlations between age and DTI measures and between education-level and DTI measures were performed using Pearson's correlation test. To correct for multiple comparisons, we applied a Bonferroni correction to the p-values. Significance was set at p ≤ 0.001. A significant negative correlation of FA with age was observed in posterior thalamic radiation (PTR) (p< 0.001). A significant positive correlation between age and MD was observed in sagittal stratum (SS) (p< 0.001), between age and RD in PTR, SS, and retrolenticular internal capsule (p< 0.001), and between age and AD in the body of the corpus callosum (p< 0.001). There were no significant correlations of DTI parameters with educational level. According to our study, RD showed the richest correlations with age, out of all DTI metrics. FA, MD, and RD showed significant changes in the diffusivity of projection fibers, while AD presented diffusivity changes in the commissural fibers. The observed heterogeneity in diffusivity changes across the brain cannot be explained by a single aging gradient pattern, since it seems that different patterns of degradation are true for different fiber tracts that no currently available theory can globally explain age-related changes in the brain. Additional factors, such as the effect of somatosensory decline, should be included as one of the important covariables to the existing patterns.
Introduction
Healthy brain aging occurs as a result of numerous interconnected structural, chemical, and functional brain changes, and, in turn, can lead to a decline in cognitive function. Brain aging is associated with a decline in concentration, attention, and other executive functions, as well as global cognitive information processing (1). Optimal cognitive functioning, which is vital for independent living, productivity, and overall quality of life, relies on coordinated processes in different brain regions. Disturbances in communication (i.e., disconnections) between these regions during healthy aging may result in cognitive decline (2).
Over the years, many novel techniques of magnetic resonance imaging (MRI) were introduced in order to clarify the neuropathological process that lies behind neurological and psychiatric disorders. One of the most revolutionary techniques is diffusion-weighted imaging (DWI) which provides tissue contrast based on the magnitude of diffusion differences between water molecules (3). Diffusion of the water molecules represents the random motion of the molecules (Brownian motion), and it is restricted or facilitated depending on the ongoing pathological process (4). The more advanced technique, derived from DWI is called diffusion-tensor imaging (DTI) that analyzes the three-dimensional shape of diffusion, named diffusion tensor. The diffusion tensor is actually a 3D structure defined by three principal diffusivities (eigenvalues, λ1, λ2, and λ3) that are associated with three principal directions (eigenvectors) (5). This technique requires more robust post-processing but can provide valuable information about the microstructure of the brain tissue.
The concept of cortical disconnections has been confirmed in healthy brain aging (without the manifest neurological disorder), using this technique in previous studies (6–8). Given that DTI is a non-invasive imaging method, it represents a useful tool to probe brain network integrity and functionality (9). DTI fractional anisotropy (FA), mean diffusivity (MD), radial diffusivity (RD), and axial diffusivity (AD) measures can give insight into diffusivity changes in the brain which can be driven both by physiological brain aging and pathological processes (degeneration, inflammation, neoplastic and other diseases). Most published DTI studies on the aging brain have relied on the FA, which has been shown to decrease throughout the white matter of the brain during the aging process (10–12). Age-related increases in MD and RD have also been consistently observed (13). However, findings regarding AD have been inconsistent, with both increases and decreases observed in different settings (10, 13). The studies comprehensively exploring all four DTI metrics mainly explored pathological processes in the brain (neurodegenerative and genetic disorders) (14, 15). There are several methods for the evaluation of DTI data, with tract-based spatial statistics (TBSS) being one of the most popular and also used in this study. The main idea of the TBSS approach is to project volumetric data onto a white matter skeleton, in order to gain statistical power and skip some steps regarding data processing (16).
There are three proposed gradient patterns of white matter changes during healthy brain aging. The first is the antero-posterior pattern, with more extensive changes in the anterior/frontal parts of the brain preceding the posterior lobes (17). The second pattern is the supero-inferior, with extensive changes present in the cranial aspects of the brain parenchyma (18). The third pattern is myelodegeneration-retrogenesis or, the “last-in-first-out” hypothesis, which proposes that the degeneration of myelin observed during the aging process occurs in the opposite direction of myelin development and maturation (19, 20).
The aim of this study was to correlate white matter DTI anisotropy and diffusivity measures (FA, MD, RD, and AD) using TBSS in healthy volunteers, with chronological age and formal education level (expressed in years of education). The second aim was to determine whether the gradient pattern of white matter associations was consistent with any proposed patterns of physiological brain aging.
Materials and Methods
Study Population
Of a total of 81 healthy volunteers who were enrolled in the study based on the initially performed power sample analysis (α = 0.05 (p-value), β = 0.2 (correspondent to the ower of 80%), and r > 0.30, the required sample was 79), 75 participants, average age 37.32 ± 11.91 years (range: 22–62 years; 53 male and 22 female participants) were included into analyses after undergoing whole brain magnetic resonance imaging (MRI) from July 2011 to April 2017 at the University of Novi Sad. Six data sets were excluded from the initial sample of 81 subjects due to technically inadequate quality. All the patients were cognitively screened using Mini-Mental State Examination (MMSE) (21).
Inclusion criteria were over 18 years of age, MMSE score over 24 (thus excluding persons eligible for the evaluation of dementia), and right-handed. MMSE is a 30-point screening test for the global cognitive assessment, used for quick exclusion of subjects with signs of cognitive impairment. Right-handedness was based on the self-report of the preferred hand and on the results of the Waterloo Handedness Questionnaire (WHQ) (22). Criteria for the exclusion from the study were acute and chronic neurologic and psychiatric disorders, presence of diffuse or focal white matter lesions in the brain (tumors, infarctions, metastases, vascular malformations, white matter hyperintensities), post-operative state, head trauma history, patients with palsy or deep paresis of the dominant hand, visual and hearing disorders, MMSE score ≤ 24, history of drug and alcohol abuse according to Drug Abuse Screening Test and Michigan Alcohol Screening Test (MAST) (23), and contraindications for MRI scanning.
Neuroimaging
All participants underwent an MRI of the brain on a 3T clinical scanner (Siemens Trio Tim, Erlangen, Germany), using an 8-channel head array. Conventional MRI of the brain consisted of T1W sagittal spin echo [time of repetition (TR)/time of echo (TE) 440 ms/3.8 ms, slice thickness 5 mm, duration 2:00 min], T2W transversal turbo spin echo (TR/TE 5150 ms/105 ms, slice thickness 5 mm, duration 2:57 min), Fluid Attenuation Inversion Recovery (FLAIR) transversal (TR/TE 8,000 ms/101 ms, slice thickness 5 mm, duration 3:30 min), diffusion-weighted imaging (DWI) (TR/TE 4100 ms/91 ms, slice thickness 5 mm, duration 2:07 min), T2W coronal turbo spin echo (TR/TE 7150 ms/111 ms, slice thickness 5 mm, duration 2:17 min), and 3D T1W MPRAGE sagittal tomograms (TR/TE 1530 ms/2.97 ms, slice thickness 1 mm, duration 5:12 min).
Conventional MRI was necessary for obtaining anatomic information and detection of potential focal or diffuse brain lesions.
Diffusion tensor imaging was performed using MDDWI sequence (multidirectional diffusion weighted imaging) with two diffusion shells (b-values of 1,000 s/mm2 and 1,500 s/mm2) from 64 diffusion-weighted directions each and two non-diffusion weighted volumes (at b0). The data were acquired at 2 mm isotropic resolution; the fold over direction was A-P with a P shift. Field-of-view was 230. DWI images were denoised using the LPCA filter and corrected for motion by linearly aligning all DWI volumes to the b 0 image. T1-weighted images were denoised using the non-local means filter and underwent N3 intensity inhomogeneity normalization, and brain extraction. Data sets that did not fulfill the quality control criteria were excluded from the analyses (three due to extreme EPI distortion and three due to skeleton misregistration). T1 images were linearly aligned to diffusion images, and diffusion images were then non-linearly warped to their respective T1-weighted scans to correct for echo-planar imaging (EPI) induced susceptibility artifacts.
Diffusion gradient directions were rotated to accommodate linear registrations. DTI fractional anisotropy (FA), mean diffusivity (MD), radial diffusivity (RD), and axial diffusivity (AD) scalar maps were generated from corrected images (24).
Diffusion tensor imaging analysis was performed using the tract-based spatial statistics (TBSS) technique from the FSL software package (24) and publicly available ENIGMA-DTI protocols (http://enigma.usc.edu/protocols/dti-protocols/) (25). Each subject's FA map was warped to the ENIGMA-DTI FA template with ANTs (26) and the transformations were applied to all respective DTI maps. DTI measures were then projected onto the template skeleton. Skeletonized measures were averaged in a total of 29 regions of interest (ROIs) from the John Hopkins University White Matter Atlas (27) (Table 1).
Statistical Analysis
Statistical analysis was performed using the software package SPSS ver. 23.0 (IBM, Chicago, USA). Methods of descriptive and comparative statistics were used (mean, median, standard deviation, minimum, maximum, frequencies, and percentage, depending on the type of the variable). After confirmation of normal distribution, correlations between age and DTI measures and between education-level and DTI measures were performed using Pearson's correlation test. Additionally, a partial correlation was performed between age and diffusivity changes in observed DTI parameters, with education as a control variable. Permutation tests for Pearson's correlations were performed for age and localization, education and localization, and for partial correlation, using education as a control variable. Gender differences were also explored using the t-test.
To correct for multiple comparisons, we applied a Bonferroni correction to the p-values. Significance was set at p ≤ 0.001 (21 ROIs × 2 tests + 4 = 46, 0.05/42 = 0.00108).
Results
A total of 75 participants were included in the study, 53 men (70.7%) and 22 women (29.3%). No significant gender-related differences in white matter diffusivity metrics were observed. The average age of the participants was 37.32 ± 11.91 years (range 22–62). The average education level was 13.87 ± 2.38 years of formal education. MMSE scoring was 28.87 ± 1.14 points, no person scored lower than 26.
Table 2 summarizes the changes in FA on the observed localization in the brain, in correlation with the chronological age and educational level of the participants. A significant negative correlation was observed in PTR (on the left p< 0.001 and on the right p = 0.001) (Figure 1). All correlations were negative. No significant correlations of FA with the level of education or the MMSE score were detected. Table 2A shows the results of the partial correlation summarized.
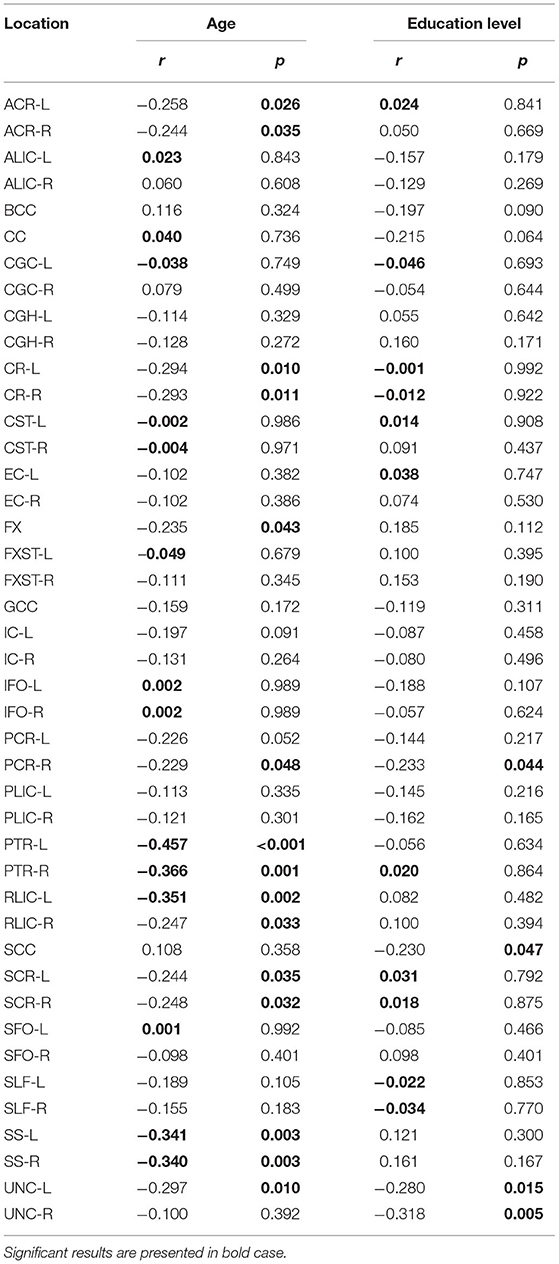
Table 2. Results of Pearson's correlation of FA with age and education level of the study participants in the observed locations (r-correlation coefficient).
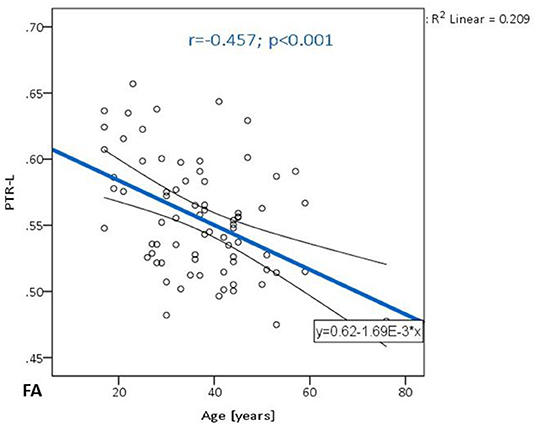
Figure 1. The trend of FA decrease with advancing age in the posterior thalamic radiation on the left.
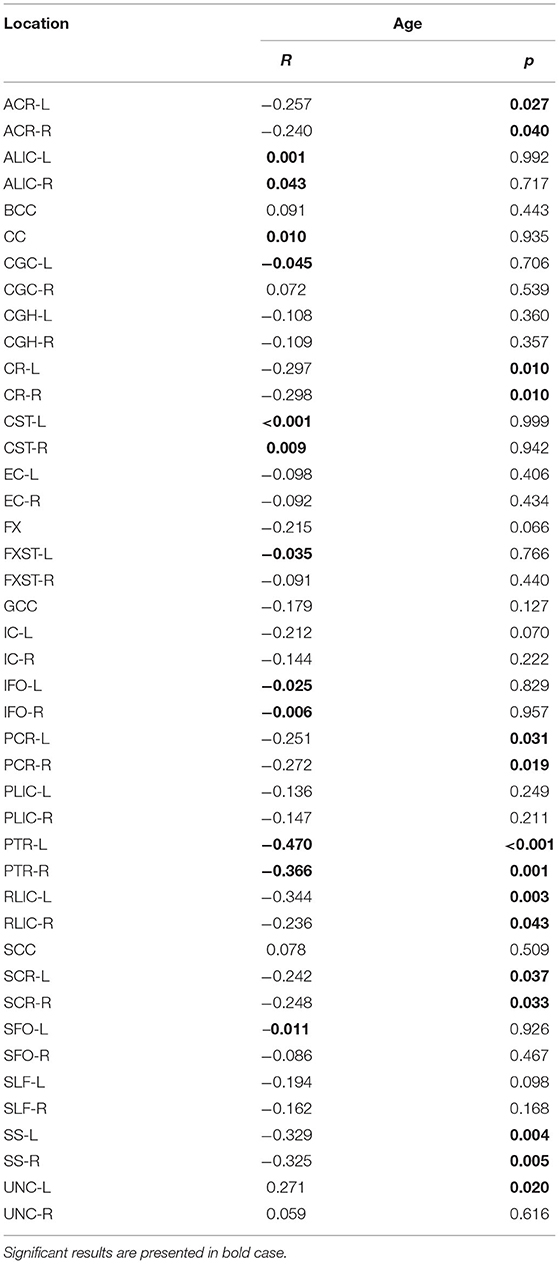
Table 2A. The results of partial correlation of FA with age, using education level as a control variable.
Table 3 shows the correlations between MD and age and between MD and educational level of the participants are presented for the analyzed locations. A significant positive correlation was confirmed only in SS on the left side (p = 0.001) (Figure 2). There were no significant correlations of this parameter with the level of formal education or the MMSE score. In Table 3A, the results of the partial correlation are summarized.
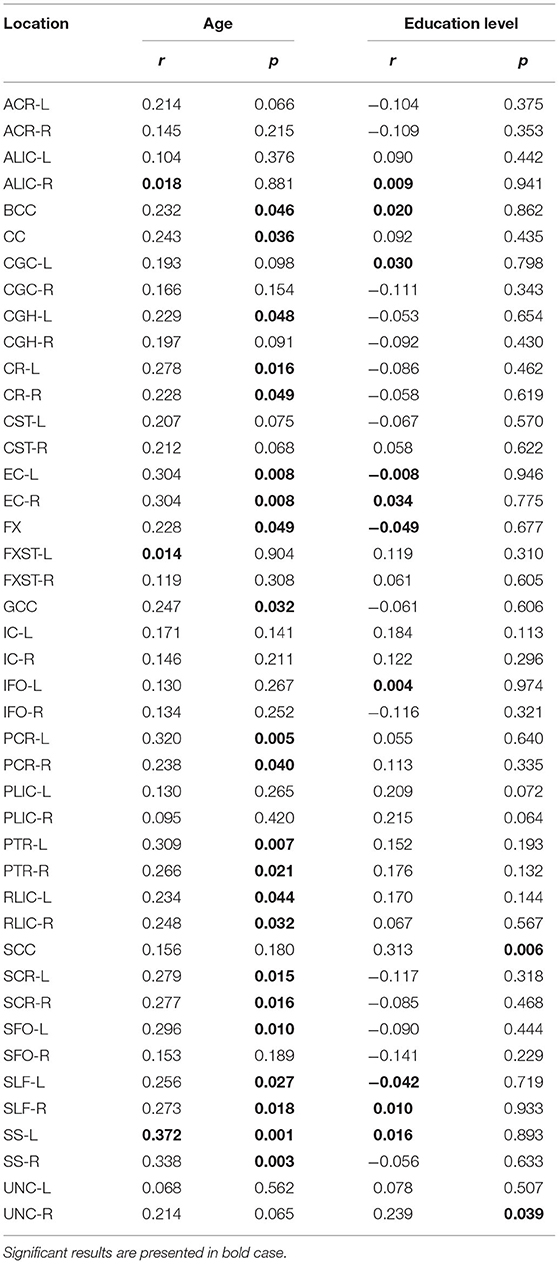
Table 3. Results of Pearson's correlation of MD with age and education level of the study participants in the observed locations (r-correlation coefficient).
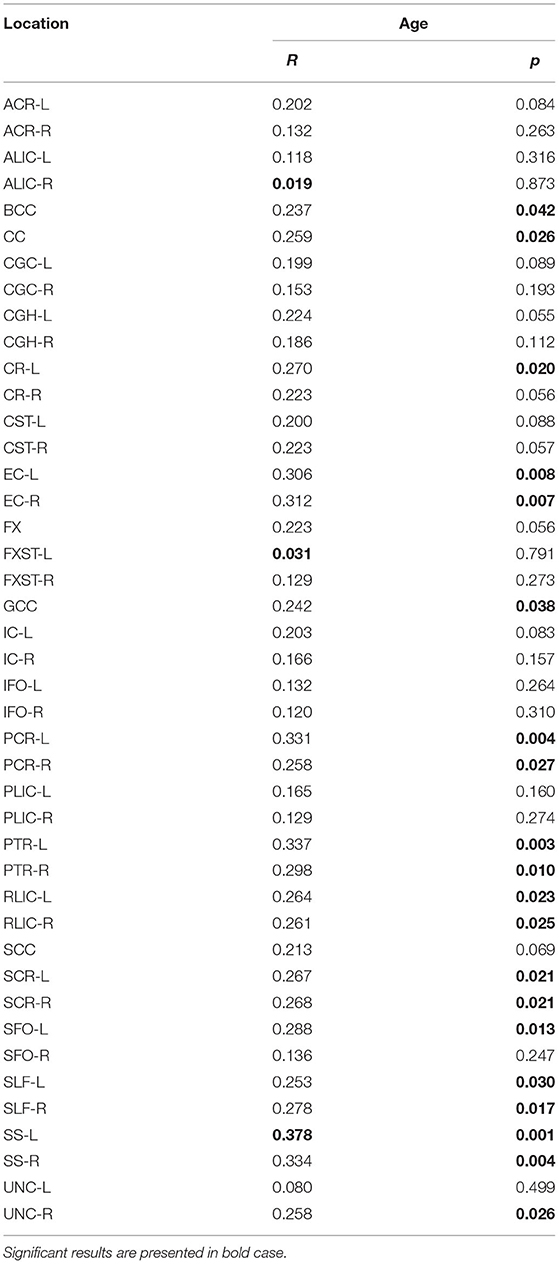
Table 3A. The results of partial correlation of MD with age, using education level as a control variable.
Significant positive correlations were detected between RD and age in PTR (on the left p< 0.001 and on the right p = 0.001, Figure 3A), SS (on the left p< 0.001, and on the right p< 0.001, Figure 3B), and in RLIC (on the left p = 0.001). There were no significant correlations of this parameter with the level of formal education or the MMSE score (Table 4). In Table 4A, the results of the partial correlation are summarized.
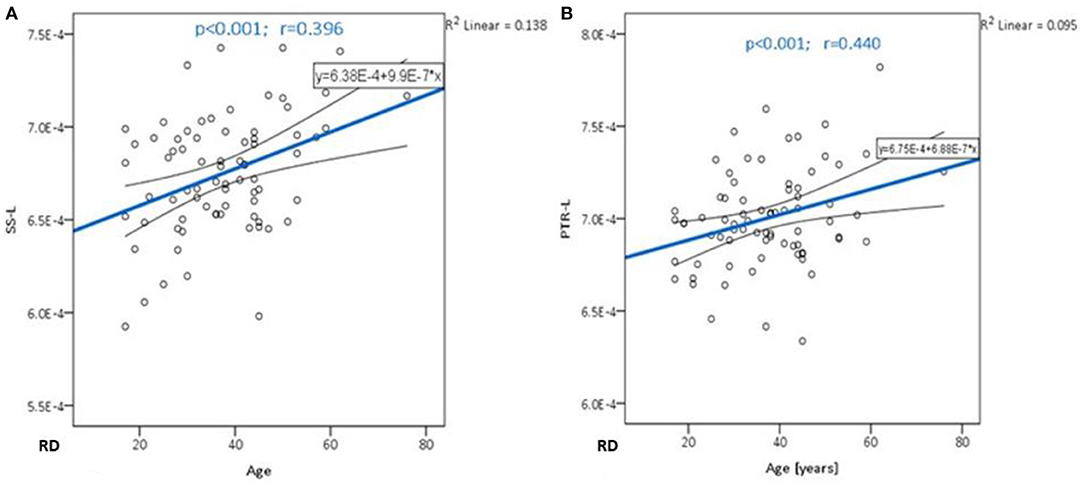
Figure 3. The trend of RD increase with advancing age in the sagittal stratum (A) and posterior thalamic radiation (B).
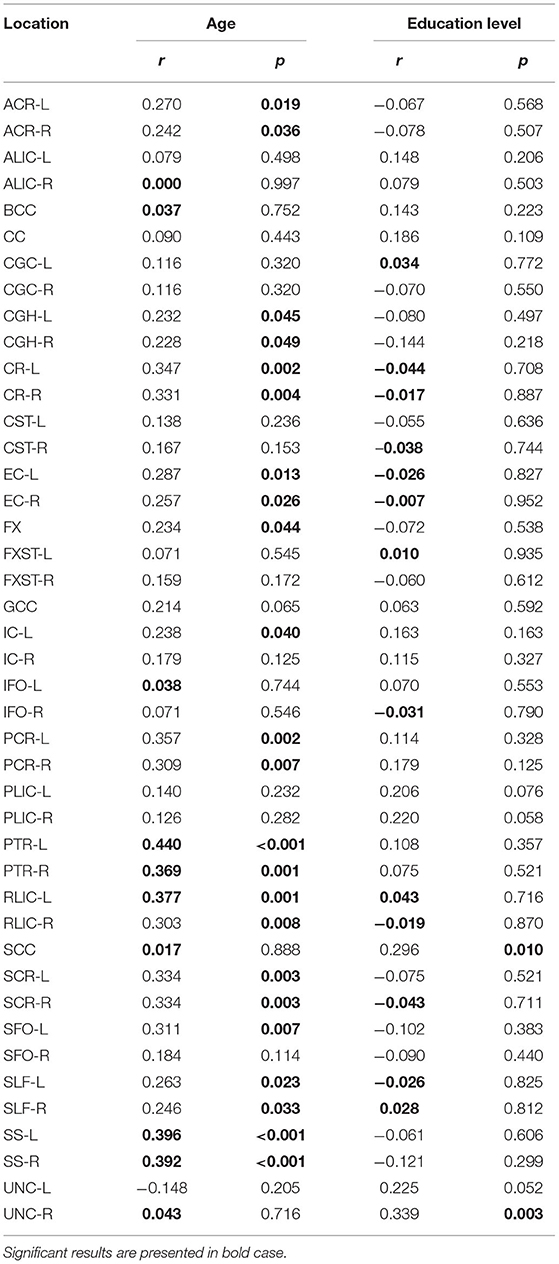
Table 4. Results of Pearson's correlation of RD with age and education level of the study participants in the observed locations (r-correlation coefficient).
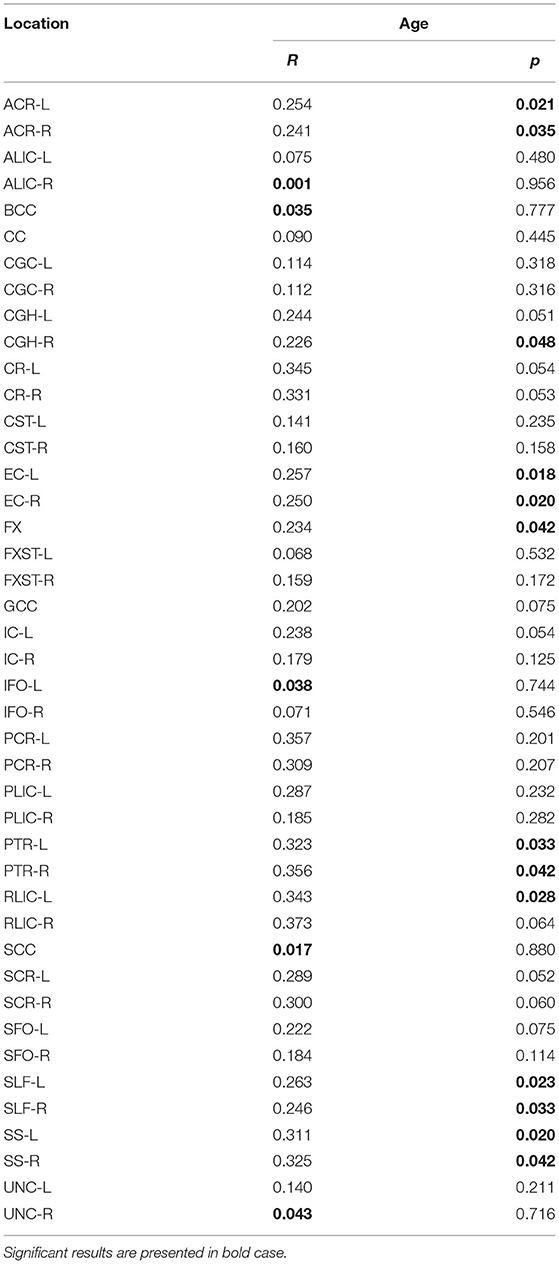
Table 4A. The results of partial correlation of RD with age, using education level as a control variable.
Significant positive correlations between AD and age were detected in BCC (p = 0.001, Figure 4). There were no significant correlations between AD and educational level (Table 5).
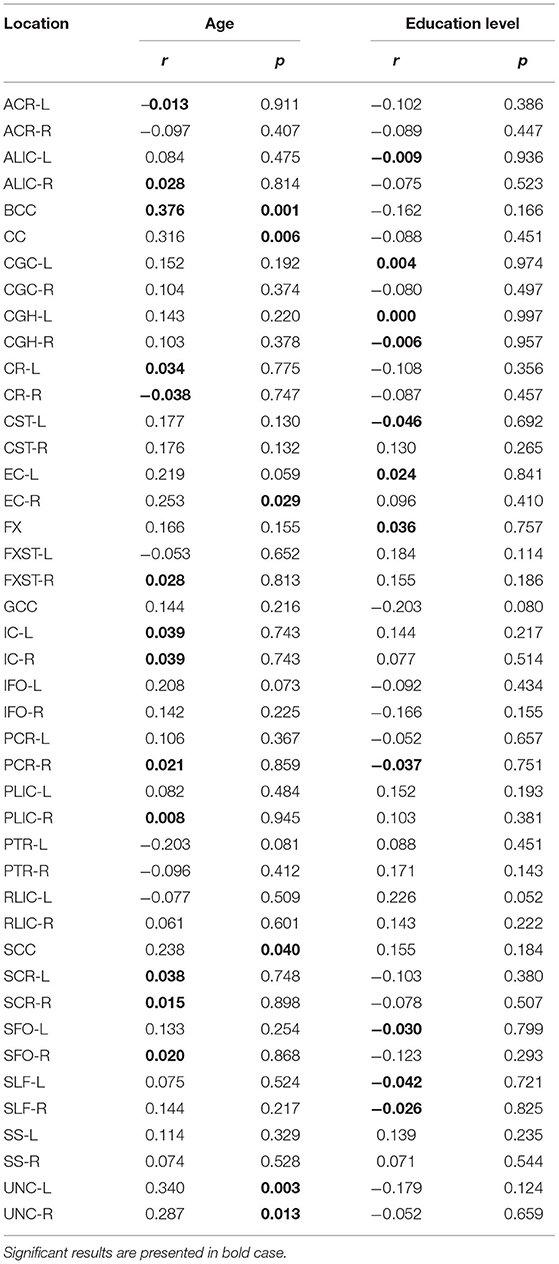
Table 5. Results of Pearson's correlation of AD with age and education level of the study participants in the observed locations (r-correlation coefficient).
However, with education level used as a control variable, the significance of the diffusivity decrease with age in the BCC was lost (Table 5A). There were no significant correlations with MMSE scores.
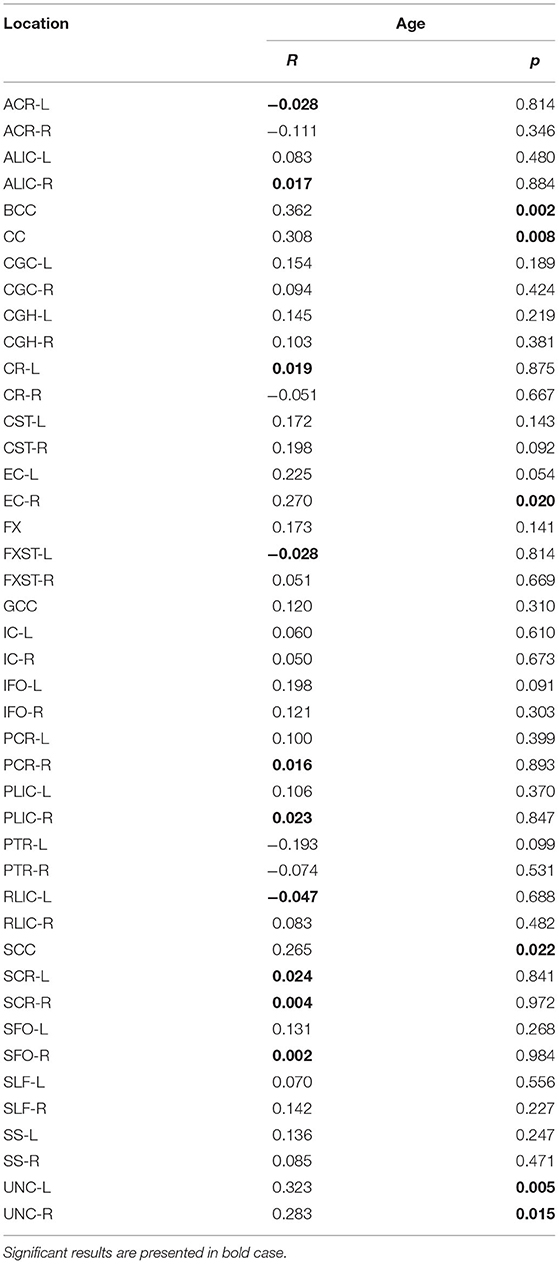
Table 5A. The results of partial correlation of AD with age, using education level as a control variable.
The results of permutation tests are provided in Supplementary Material.
Discussion
The main goal of the study was to correlate white matter DTI anisotropy and diffusivity measures (FA, MD, RD, and AD) in healthy volunteers with chronological age and formal education level. Additionally, we aimed to determine whether the gradient pattern of these associations was consistent with any of the proposed physiological brain aging patterns.
In our study, FA values showed significant reduction with advancing age in the PTR, which is the dorsal part of thalamocortical radiations connecting the thalamus with cortical centers. It consists of fibers that start from the caudal thalamic nuclei (pulvinar and lateral geniculate) via the retrolenticular part of the IC toward the parietal and occipital cortices. The function of this tract is primarily somatosensory (comprises parts of visual, gustatory, and auditory tracts) (28, 29). The relationship between the decline in perceptive and cognitive abilities is well-established in the process of aging; however, growing evidence suggests that there is a direct association between sensory deprivation-decreased processing of sensory information- and cognitive decline (30). The decrease in FA was observed in women with anorexia nervosa (31), patients with a high risk of psychosis converting to manifest psychosis (32), methamphetamine addicts (33), and children with cerebral palsy (34). An interesting recent study showed the relationship between cigarette smoking and changes in FA and MD in the PTR (35), supporting the necessity to include the daily habits as a sine-qua-non in future DTI studies (eg., nicotine and alcohol consumption, substance abuse, and pharmaceuticals).
A significant increase in MD with advancing age was observed in the SS on the left side. This tract is positioned in the deep lateral aspect of the cerebral hemisphere and organized into three layers: superficial (consisting of inferior longitudinal fasciculus and inferior part of SLF), middle (fronto- occipital fasciculus), and deep (fibers of optic radiation) (36). It is included in the information transport from parietal, occipital, cingulate, and temporal gyri to the subcortical nuclei (thalamus and pontine nuclei). In the past studies, the increase in MD was detected in patients with essential tremors and has been suggested as one of the differentials from Parkinson's disease in these patients (37). It was also observed in the mild traumatic injury of the brain (38), children with ADHD (associated with aberrant myelination) (39) but was also observed in healthy cigarette smokers (40).
A significant increase in RD with advanced age was detected in the PTR, SS, and RLIC. RLIC is positioned dorsally to the corticospinal and corticopontineus tracts but represents a functionally separate entity because it carries parts of optic radiation, proximal part of PTR, and occipito-tectal and occipito-pontine fibers. Since the increase in this parameter was observed in the PTR and RLIC, it is clear that, along with the close anatomic relationship, there is also a functional connection between these two structures, mainly in the somatosensory information transmission. SS is also functionally associated with the latter two tracts. Disturbances in the diffusivity of RLIC have been priorly observed in depression (41), bipolar disorder (36), methadone addiction (42), and traumatic injury of the brain associated with sports (43).
A significant increase in AD with advancing age was detected in BCC. The corpus callosum is the biggest commissural tract that connects corresponding regions of cerebral hemispheres. Different parts of CC are included in the transmission of various information, with premotor and supplementary motor fibers of the cortex located in the body 409. Madden et al. did not show differences in AD with advanced age (44). However, Fan et al. presented differences in FA between young (20–28 years) and elderly adults (60–75 years) primarily in the anterior portion of CC (within interhemispheric fibers), that were positively correlated with performance on the visuospatial tasks—AD was not explored in that particular study (45). Evidence supporting the “last-in-first-out” theory was also presented in the study of Davis et al. presenting greater age-related differences in RD than in AD in the CC and UNC, thus supporting the theory of myelodegeneration and retrogenesis (46). In our study, however, AD was more sensitive to the changes in these regions, thus indicating that these theoretical interpretations of the white matter diffusivity changes are in fact a simplification of the aging process. That is why we highlight the need for a deeper understanding of the age-related effect on the white matter integrity.
It is interesting to note that FA, MD, and RD were associated with the diffusivity changes in projection tracts (that connect cortical structures with the brainstem, cerebellum, and spinal medulla). On the contrary, the AD was associated with diffusivity changes in commissural tracts, connecting two hemispheres. In a recent longitudinal study, that followed healthy participants aged 50–84 years (at the moment of the first scan, the average age of women was 63.85 years and men was 67.31 years) during the follow-up period of 7 years, showed the most prominent changes in the FA and RD in commissural and projection tracts (47). It is possible that the changes in these two parameters are first observed in projection tracts, as shown in our study, and later during chronological aging, are followed by diffusivity changes in the commissural tracts as well.
Little is known about the oligodendrocyte dynamics and myelin sheath remodeling in the mature brain. However, recent studies confirmed that sensory enrichment could induce experience-dependent myelination and robustly increase the integration of oligodendrocytes (48). Reversely, the results of our study, with age-related changes detected mainly in the somatosensory tracts may imply that somatosensory deprivation during healthy aging negatively affects myelin sheaths (in the sense of myelin degradation and/ or disintegration) and results in DTI metrics changes. This effect of somatosensory deprivation on white matter integrity during the brain aging, should, in our opinion, be included in the current theories of the white matter degradation during aging process, along with the myelodegeneration-retrogenesis and gradients of degenerations (antero- posterior and supero-inferior).
It is interesting to see the overlap in the DTI metrics changes observed in specific tracts, which could potentially clear the etiology of age-related changes. We tried to group the differences in DTI metrics in the following patterns, as shown in Table 6. In our study, the observed patterns of age-related diffusivity changes were the FA-RD (detected in PTR) and the MD-RD pattern (detected in SS), speaking in favor of myleodegeneration over axonal damage in the named tracts (Figure 5 showing brain maps). In RLIC, we found the diffusivity changes reflected as RD increased, also speaking in favor of myelodegeneration. Given the absence of manifest cognitive impairment and the strict exclusion criteria that we proposed for randomizing the subjects, in our opinion, patterns found in our study sample were expected. In the cohort of healthy, fully functional, and cognitively unchallenged subjects, age-related myelin degeneration and loss seem to be the leading mechanism of white matter diffusivity changes. One recent study also tried to group the findings in DTI metrics across the brain white matter tracts. The FA–RD pattern was observed only in the posterior thalamic radiation in our study sample, while in the study of Molloy et al., it was the most observed pattern, detected in more than 30% of the brain tracts (10). The FA–RD–AD pattern in this study was observed in almost 30% of the brain (10). Other patterns were more uncommon. Burzynska et al. confirmed that FA was significantly correlated with age in more than half of the brain white matter (13). Molloy et al. showed that the highest percentage of voxels significantly correlated with age were found in the forceps minor (part of CC) (10). The FA–MD–RD pattern is related to chronic white matter degeneration, mainly dependent on the myelin loss during the aging process. The changes in AD, however, have been linked to axonal damage (in combination with FA changes representing acute axonal swelling and fragmentation) (13). FA alone changes have been suggested to reflect mild microstructural changes with the loss of fiber structure without great tissue loss. Additional MD changes, following FA changes, reflect the presence of tissue loss and atrophy (46). The changes in RD (combined with FA and, sometimes AD) were related to gliosis and subsequent increase in extracellular tissue (48). Several studies confirmed that greater changes in RD (compared to AD) were found across the brain, similar to our results, suggesting that the leading mechanism of white matter degradation is to be associated with myelin degeneration (10, 13, 17, 46). The findings in AD were inconsistent in our study, similar to other studies from the available literature, showing both positive and negative correlations with age (10, 13, 46). The explanation for this finding in our study might be that our cohort had more prevalent chronic axonal damage (related to the increase in AD) or that these changes can be related to the incoherence in the regions of crossing fibers (which is, in our opinion, a more probable explanation).
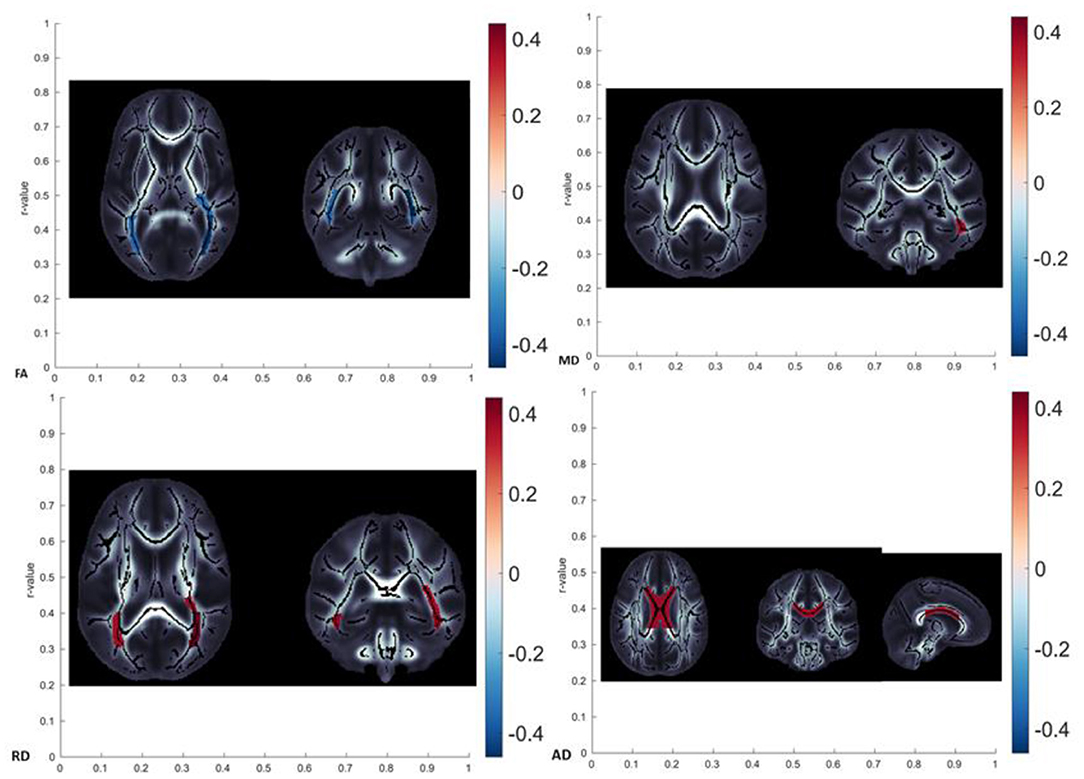
Figure 5. Brain maps showing localization with significant age-related diffusivity changes (FA showed a significant decrease in posterior thalamic radiation, MD showed a significant increase in left sagittal stratum, RD showed a significant increase in posterior thalamic radiation, and AD showed significant changes in the body of corpus callosum).
Finally, no significant gender differences related to white matter diffusivity metrics were detected (Figure 6). However, this finding might be associated with the small sample with uneven distribution of men and women (males significantly outnumbering females).
Heterogeneity in findings in diffusivity changes across the brain, in our opinion, cannot be explained by one single gradient of physiological brain aging. It seems that different patterns of degradation are true for different fiber tracts in the brain and that no currently available theory can globally explain age-related changes in the brain. Furthermore, the effect of somatosensory decline should be included as one of the important covariables in the existing patterns.
In addition, a recent study by Behler et al. showed that diffusion properties of most white matter tracts in a healthy aging brain followed a triphasic pattern (in a simplified manner, FA showed a gradual increase during early adulthood followed by a stable state during middle age, and gradual decline in senium; RD, MD, and AD showed a decline during the early adulthood, followed by the stable state in middle age and increase in senium), so that non-linear age correction should be applied to most of the tracts. Cerebellar tracts (superior and middle cerebellar pedunculi) showed basically no change over time (i.e., age correction is not applicable); optic radiation and anterior limb of the internal capsule showed linear regression with age, meaning that non-linear age correction is not necessary (49).
Study Limitations
There are some limitations to the study performed. Even though the number of participants was justified by the power analysis, it is possible that some correlations would have shown to be significant if the study sample were larger, and this might be resolved in a future study. However, it is important to highlight that the exclusion criterion for our study sample was the presence of white matter lesions including white matter hyperintensities (WMH) which represents a pertinent factor for the assessment of the changes in the white matter related to aging.
The second limitation of the study was the lack of a detailed neurocognitive assessment of our participants (only a screening cognitive test was performed as a part of the exclusion criteria). MMSE scoring test represents a screening test, of high clinical importance, but is not sensitive enough for the cases of early cognitive impairment, thus, in our opinion, suitable for the exclusion of cognitively challenged persons, but not suitable for detailed correlations. Some of the tracts we analyzed are known to be associated with affect and personality features, which means that additional assessment of depression and anxiety would be recommendable.
An additional limitation is a cross-sectional evaluation and the lack of longitudinal design, since the changes in diffusivity measurements may follow certain trajectories during chronological aging in an individual.
It is necessary to point out that the maturation of the fibers does not follow the identical dynamics in all observed tracts. Some of the tracts (IFO, AIC, PLIC, SCC, BCC, GCC, UNC, SFO, IFO, SLF) reach the full maturity (maximal FA and minimal MD values) between 20 and 40 years of age (50). In one part of our study sample, these tracts potentially did not develop their full maturity, given that the average age of the sample was around 37 years.
The final (small) limitation of the study is the lack of information on prematurity, which could also influence the changes in tract diffusivity (CC in the first place) (51).
Even though there were no significant comorbidities noted in the study sample, it will be reasonable to include additional information on metabolic function (blood glucose level, lipidemia, parameters of liver function, arterial tension, etc.) and lifestyle in the future studies (52, 53).
It is also worth mentioning that advanced techniques for evaluation of DWI data could provide more detailed information than the DTI option (54) and added to the potential of longitudinal studies in revealing the gradients of changes in the DTI metrics are two main recommendations for the future studies.
Conclusion
All four DTI metrics showed significant correlations with the advancing age of healthy participants (FA in posterior thalamic radiation, MD in left sagittal stratum, RD in the posterior thalamic radiation, sagittal stratum, and retrolenticular limb of internal capsule, and AD in body of the corpus callosum). According to our study, RD showed the richest correlations with age, out of all DTI metrics. FA, MD, and RD showed significant changes in the diffusivity of projection fibers, while AD presented diffusivity changes in the commissural fibers.
It is important to realize that all four DTI metrics are necessary for the assessment of healthy brain aging effect on white matter integrity, given that routinely analyzed parameters (FA and MD) do not allow complete insight into diffusivity changes. Since the changes in the white matter integrity that we observed during healthy aging also overlap with changes observed in several pathological conditions—psychiatric disorders, traumatic lesions, and dementia/MCI—caution is necessary when interpreting these differences in a healthy individual. In such cases, a longitudinal follow-up is necessary to track the trajectories of degenerative changes associated with the aging brain.
Finally, the observed heterogeneity in diffusivity changes across the brain, in our opinion, cannot be explained by a single aging pattern (antero-posterior, supero-inferior, or myelodegeneration- retrogenesis). It seems that different patterns of degradation are true for different fiber tracts in the brain and that no currently available theory can globally explain age-related changes in the brain. Some additional factors, such as the effect of somatosensory decline, should be included as one of the important covariables in the existing patterns.
Data Availability Statement
The raw data supporting the conclusions of this article will be made available by the authors, withoutundue reservation.
Ethics Statement
The studies involving human participants were reviewed and approved by Ethical Committee of Faculty of Medicine University of Novi Sad. The patients/participants provided their written informed consent to participate in this study.
Author Contributions
JB drafted the article. JB, MT, NB, DL, NJ, and TN were involved in acquisition, collection, and analysis of the data. DK, MT, and ML performed critical revision of intellectual content. All authors saw and approved the final version of drafted manuscript.
Funding
TN and NJ were funded, in part, by NIH grants: R01 AG059874, U54 EB020403, T32 AG058507, and U01 AG068057. The authors also received partial grant support from Biogen, Inc., for research unrelated to this work. JB, DK, and DL were funded by Provincial Secretariat for Higher Education and scientific Research, grant No. 142-451-2600/2021-01.
Conflict of Interest
The authors declare that the research was conducted in the absence of any commercial or financial relationships that could be construed as a potential conflict of interest.
Publisher's Note
All claims expressed in this article are solely those of the authors and do not necessarily represent those of their affiliated organizations, or those of the publisher, the editors and the reviewers. Any product that may be evaluated in this article, or claim that may be made by its manufacturer, is not guaranteed or endorsed by the publisher.
Supplementary Material
The Supplementary Material for this article can be found online at: https://www.frontiersin.org/articles/10.3389/fneur.2022.870909/full#supplementary-material
References
1. Ishii R, Canuet L, Aoki Y, Hata M, Iwase M, Ikeda S, et al. Healthy and pathological brain aging: from the perspective of oscillations, functional connectivity and signal complexity. Neuropsychology. (2017) 75:151–61. doi: 10.1159/000486870
2. Desai AK, Grossberg GT, Chibnall JT. Healthy brain aging: a road map. Clin Geriatr Med. (2010) 26:1–16. doi: 10.1016/j.cger.2009.12.002
3. Huisman TAGM. Diffusion-weighted and diffusion tensor imaging of the brain made easy. Cancerimaging. (2010) 10: S163–71. doi: 10.1102/1470-7330.2010.9023
4. Drake-Perez M, Boto J, Fitsiori A, Lovblad K, Vargas MI. Clinial applications of diffusion weighted imaging in neuroradiology. Insights Imaging. (2018) 9:535–47. doi: 10.1007/s13244-018-0624-3
5. O'Donnell LJ, Westin CF. An introduction to diffusion tensor image analysis. Neurosurg Clin N Am. (2011) 22:185–VII. doi: 10.1016/j.nec.2010.12.004
6. O'Sullivan M, Jones DK, Summers PE, Morris RG, Williams SC, Markus HS. Evidence for cortical “disconnection” as a mechanism of age-related cognitive decline. Neurology. (2001) 57:632–8. doi: 10.1212/WNL.57.4.632
7. Andrews-Hanna JR, Snyder AZ, Vincent JL, Lustig C, Head D, Raichle ME, Buckner RL. Disruption of large-scale brain systems in advanced aging. Neuron. (2007) 56:924–35. doi: 10.1016/j.neuron.2007.10.038
8. Salat DH. The declining infrastructure of the aging brain. Brain Connect. (2011) 1:279–93. doi: 10.1089/brain.2011.0056
9. Vinke EJ, de Groot M, Venkatraghavan V, Klien S, Niessen WJ, Ikram MA, et al. Trajectories of imaging markers in brain aging: the Rotterdam study. Neurobiol Aging. (2018) 71:32–40. doi: 10.1016/j.neurobiolaging.2018.07.001
10. Molloy CJ, Nugent S, Bokde ALW. Alterations in diffusion measures of white matter integrity associated with healthy aging. J Gerontol A Bio Sci Med Sci. (2019) 22:945–54. doi: 10.1101/540443
11. Wasenaar TM, Yaffe C, van der Werf YD, Sexton C. Associations between modifiable risk factors and white matter of the aging brain: insights from diffusion tensor imaging studies. Neurobiol Aging. (2019) 80:56–70. doi: 10.1016/j.neurobiolaging.2019.04.006
12. Brickman AM, Meier IB, Korgaonkar MS, Provenzano FA, Grieve SM, Siedlecki KL, et al. Testing the white matter retrogenesis hypothesis of cognitive aging. Neurobiol Aging. (2012) 33:1699–715. doi: 10.1016/j.neurobiolaging.2011.06.001
13. Burzynska AZ, Preuschhof C, Backman L, Nyberg L, Li SC, Lindenberger U, et al. Age-related differences in white matter microstructure: region-sepcific patterns of diffusivity. Neuroimage. (2010) 49:2104–12. doi: 10.1016/j.neuroimage.2009.09.041
14. Mayo CD, Garcia-Barrera MA, Mazerolle EL, Ritchie LJ, Fisk JD, Gawryluk JR, et al. Relationship between DTI metrics and cognitive function in Alzheimer's disease. Front Aging Neurosci. (2019) 10:436. doi: 10.3389/fnagi.2018.00436
15. Ashrafi MR, Amanat M, Garshasbi M, Kameli R, Nilipour Y, Heidari M, et al. An update on clinical, pathological, diagnostic and therapeutic perspectives of childhood leukodystrophies. Expert Rev Neurother. (2020) 20:65–84. doi: 10.1080/14737175.2020.1699060
16. Bach M, Laun FB, Leemans A, Tax CMW, Biessels GJ, Stieltjes B, et al. Methodological considerations on tract-based spatial statistics (TBSS). Neuroimage. (2014) 100:358–69. doi: 10.1016/j.neuroimage.2014.06.021
17. Bennett IJ, Madden DJ, Vaidya CJ, Howard DV, Howard JH Jr. Age-related differencesin multiple measures of white matter integrity: a diffusion tensor imaging study of healthy aging. Hum Brain Map. (2010) 31:378–90. doi: 10.1002/hbm.20872
18. Zahr NM, Rohlfing T, Pfefferbaum A, Sullivan EV. Problem solving, working memory, and motor correltaes of association and commissural fiber bundles in normal aging: a quantitative fiber tracking study. Neuroimage. (2009) 44:1050–62. doi: 10.1016/j.neuroimage.2008.09.046
19. Tian L, Ma L. Microstructural changes of the human brain from early to mid-adulthood. Front Hum Neurosci. (2017) 11:393. doi: 10.3389/fnhum.2017.00393
20. Schmierer K, Scaravilli F, Altmann DR, Barker GJ, Miller DH. Magnetization transfer ratio and myelin in postmortem multiple sclerosis brain. Ann Neurol. (2004) 56:407–15. doi: 10.1002/ana.20202
21. Folstein MF, Folstein SE, McHugh PR. “Mini-mental state”. A practical method for grading the cognitive state of patients for clinician. J Psychiatr Res. (1975) 12:189–98. doi: 10.1016/0022-3956(75)90026-6
22. Cavill S, Bryden P. Development of handedness: comparison of questionnaire and performance-based measures of preference. Brain Cogn. (2003) 53:149–51. doi: 10.1016/S0278-2626(03)00098-8
23. Lapham SC, Skipper BJ, Owen JP, Kleyboecker K, Teaf D, Thompson B, et al. Alcohol abuse screening instruments: normative test data collected from a first DWI offender screening programe. J Stud Alcohol. (1995) 56:51–9. doi: 10.15288/jsa.1995.56.51
24. Basser PJ Mattiello J, LeBihan D. MR diffusion tensor spectroscopy and imaging. Biophys J. (1994) 66:259–67. doi: 10.1016/S0006-3495(94)80775-1
25. Jahanshad N, Kochunov PV, Sprooten E, Mandl RC, Nichols TE, Almasy L, et al. Multi-site genetic analysis of diffusion images and voxelwise heritability analysis: a pilot project of the ENIGMA-DTI working group. Neuroimage. (2013) 81:455–69. doi: 10.1016/j.neuroimage.2013.04.061
26. Avants BB, Tustison NJ, Song G, Cook PA, Klein A, Gee JC. A reproducible evaluation of ANTs similarity metric performance in brain image registration. Neuroimage. (2011) 54:2033–44. doi: 10.1016/j.neuroimage.2010.09.025
27. Wakana S, Jiang H, Nagae-Poetscher LM, Van Zijl PCM, Mori S. Fiber tract-based atlas of human white matter anatomy. Radiology. (2004) 230:77–87. doi: 10.1148/radiol.2301021640
28. Hua K, Zhang J, Wakana S, Jiang H, Li X, Reich DS, et al. Tract probability maps in stereotaxic spaces: analyses of white matter anatomy and tract-specific quantification. Neuroimage. (2008) 39:336–47. doi: 10.1016/j.neuroimage.2007.07.053
29. Aralasmak A, Ulmer JL, Kocak M, Salvan CV, Hillis AE, Yousem DM. Association, commissural, and projection pathways and their functional deficit reported in literature. J Comput Assist Tomogr. (2006) 30:695–715. doi: 10.1097/01.rct.0000226397.43235.8b
30. Roberts KL, Allen HA. Perception and cognition in the ageing brain: a brief review of the short- and long-term links between perceptual and cognitive decline. Front Aging Neurosci. (2016) 8:39. doi: 10.3389/fnagi.2016.00039
31. Freiling H, Fischer J, Wilhelm J, Jiang H, Li X, Reich DS, et al. Microstructural abnormalities of the posterior thalamic radiation and the mediodorsal thalamic nuclei in females with anorexia nervosa- a voxel based diffusion tensor imaging (DTI) study. J Psychiatr Res. (2012) 46:1237–42. doi: 10.1016/j.jpsychires.2012.06.005
32. Leon-Ortiz P, Reyes-Madrigal F, Kochunov P, Gomez-Cruz G, Moncada-Habib T, Malacara M, et al. White matter alterations and the conversion to psychosis: a combined diffusion tensor imaging and glutamate 1H-MRS study. Schizofrenia Res. (2020) S0920–9964(20)30358-3. doi: 10.1016/j.schres.2020.06.006
33. Huang S, Yang W, Luo J, Yan C, Liu J. White matter abnormalities based on TBSS and its correlation with impulsivitiy behavior of methamphetamine addicts. Front Psychiatry. (2020) 11:452. doi: 10.3389/fpsyt.2020.00452
34. Hoon AH Jr, Stashinko EE, Nagae LM, Nagae LM, Lin DMD, Keller J, et al. Sensory and motor deficits in children with cerebral palsy born preterm correlate with diffusion tensor imaging abnormalities in thalamocortical pathways. Dev Med Child Neurol. (2009) 51:697–704. doi: 10.1111/j.1469-8749.2009.03306.x
35. Gray JC, Thompson M, Bachman C, Owens MM, Murphy M, Palmer R. Associations of cigarette smoking with gray and white matter in the UK Biobank. Neuropsychopharmacology. (2020) 45:1215–22. doi: 10.1038/s41386-020-0630-2
36. Di Carlo DT, Benedetto N, Duffau H, Cagnazzo F, Weiss A, Castagna M, et al. Microsurgical anatomy of the sagittal stratum. Acta Neurochir. (2019) 161:2319–27. doi: 10.1007/s00701-019-04019-8
37. Juttukonda MR, Franco G, Englot DJ, Lin YC, Petersen KJ, Trujillo P, et al. White matter differences between essential tremor and Parkinson disease. Neurology. (2019) 92:e30–9. doi: 10.1212/WNL.0000000000006694
38. Zhu J, Ling J, Ding N. Association between diffusion tensor imaging findings and cognitive outcomes following mild traumatic brain injury: a PRISMA-compliant meta-analysis. ACS Chem Neurosci. (2019) 10:4864–9. doi: 10.1021/acschemneuro.9b00584
39. Onnink AMH, Zwiers MP, Hoogman M, Mostert JC, Dammers J, Kan CC, et al. Deviant white matter structure in adults with attentiondeficit/hyperactivity disorder points to aberrant myelination and affects neuropsychological performance. Prog Neuropsychopharmacol Biol Psychiatry. (2015) 63:14–22. doi: 10.1016/j.pnpbp.2015.04.008
40. Liang H, Chang L, Chen R, Oishi K, Ernst T Independent and cobined effects of chronic HIV-infection and tobacco smoking on brain microstructure. J Neuroimmune Pharmacol. (2018) 13:509–22. doi: 10.1007/s11481-018-9810-9
41. Hyett MP, Perry A, Breakspear M, Wen W, Parker GB. White matter alterations in the internal capsule and psychomotor impairment in melancholic depression. PLoS ONE. (2018) 13:e0195672. doi: 10.1371/journal.pone.0195672
42. Bauer IE, Ouyang A, Mwangi B, Sanches M, Zunta-Soares GB, Keefe RS, et al. Reduced white matter integrity and verbal fluency impairment in young adults with bipolar disorder: a diffusion tensor imaging study. J Psychiatr Res. (2015) 62:115–22. doi: 10.1016/j.jpsychires.2015.01.008
43. Li W, Zhu J, Li Q, Ye J, Chen J, Liu J, et al. Brain white matter integrity in heroin addicts during methadonema intenance treatment is related to relapse propensity. Brain Behav. (2016) 6:e00436. doi: 10.1002/brb3.436
44. Madden DJ, Spaniol J, Costello MC, Bucur B, White LE, Cabeza R, et al. Cerebral white matter integrity mediates adult age differencesin cognitive performance. J Cogn Neurosci. (2009) 21:289–302. doi: 10.1162/jocn.2009.21047
45. Fan YT, Fang YW, Chen YP, Leshikar ED, Lin CP, Tzeng OJL, et al. Aging, cognition, and the brain: effects of age-related variation in white matter integrity on neuropsychological function. Aging Mental Health. (2019) 23:831–9. doi: 10.1080/13607863.2018.1455804
46. Zhang Y, Du AT, Hayasaka S, Jahng GH, Hlavin J, Zhan W, et al. Patterns of age-related water diffusion changes in human brain by concordance and disconcordance analysis. Neurobiol Aging. (2010) 31:1991–2001. doi: 10.1016/j.neurobiolaging.2008.10.009
47. Bender AR, Völkle MC, Raz N. Differential aging of cerebral white matter in middle-aged and older adults: a seven-year follow-up. Neuroimage. (2016) 125:74–83. doi: 10.1016/j.neuroimage.2015.10.030
48. Hughes EG, Orthmann-Murohy JL, Langseth AJ, Bergles DE. Myelin remodeling through experiencedependent oligodendrogenesis in the adult somatosensory cortex. Nat Neurosci. (2018) 21:696–706. doi: 10.1038/s41593-018-0121-5
49. Behler A, Kassubek J, Muller HP. Age-related alterations in DTI metrics in the human brainconsequences for age correction. Front Aging Neurosci. (2021) 6:94. doi: 10.3389/fnagi.2021.682109
50. Olson IR, Von Der Heide RJ, Alm KH, Vyas G. Development of the uncinate fasciculus: implicationsfor theory and developmental disorders. Dev Cogn Neurosci. (2015) 14:50–61. doi: 10.1016/j.dcn.2015.06.003
51. Shim SY, Jeong HJ, Son DW, Jeong JS, Oh SH, Park SY, et al. Altered microstructure of white matter except the corpus callosum is independent of prematurity. Neonatology. (2012) 102:309–15. doi: 10.1159/000341867
52. Hofer S, Frahm J. Topography of the human corpus callosum revisited: comprehensive fiber tractography using diffusion tensor magnetic resonance imaging. Neuroimage. (2006) 32:989–94. doi: 10.1016/j.neuroimage.2006.05.044
53. Smith JC, Lancaster MA, Nielson KA, Woodard JL, Seidenberg M, Durgerian S, et al. Interactive effects of physical activity and APO-ξ4 on white matter tract diffusivity in healthy elders. Neuroimage. (2016) 131:102–12. doi: 10.1016/j.neuroimage.2015.08.007
Keywords: diffusion-tensor imaging, brain aging, magnetic resonance imaging, physiological brain aging, pattern
Citation: Boban J, Thurnher MM, Boban N, Law M, Jahanshad N, Nir TM, Lendak DF and Kozic D (2022) Gradient Patterns of Age-Related Diffusivity Changes in Cerebral White Matter. Front. Neurol. 13:870909. doi: 10.3389/fneur.2022.870909
Received: 07 February 2022; Accepted: 25 April 2022;
Published: 02 June 2022.
Edited by:
Hans-Peter Müller, University of Ulm, GermanyReviewed by:
Jiajia Zhu, First Affiliated Hospital of Anhui Medical University, ChinaShun Takahashi, Osaka University, Japan
Copyright © 2022 Boban, Thurnher, Boban, Law, Jahanshad, Nir, Lendak and Kozic. This is an open-access article distributed under the terms of the Creative Commons Attribution License (CC BY). The use, distribution or reproduction in other forums is permitted, provided the original author(s) and the copyright owner(s) are credited and that the original publication in this journal is cited, in accordance with accepted academic practice. No use, distribution or reproduction is permitted which does not comply with these terms.
*Correspondence: Jasmina Boban, amFzbWluYS5ib2JhbkBtZi51bnMuYWMucnM=