- 1Department of Neurosurgery, National Neuroscience Institute, Singapore, Singapore
- 2Duke-NUS Medical School, Singapore, Singapore
- 3Department of Surgery, Faculty of Medicine, University of Malaya, Kuala Lumpur, Malaysia
- 4Neurosurgical Division, Department of Clinical Neurosciences, University of Cambridge, Cambridge, United Kingdom
Background: The aim of this study was to create a simplistic taxonomy to improve transparency and consistency in, and reduce complexity of, interpreting diffusion tensor imaging (DTI) profiles in white matter disruption. Using a novel strategy of a periodic table of DTI elements, we examined if DTI profiles could demonstrate neural properties of disruption sufficient to characterize white matter changes specific for hydrocephalus vs. non-hydrocephalus, and to distinguish between cohorts of neural injury by their differing potential for reversibility.
Methods: DTI datasets from three clinical cohorts representing pathological milestones from reversible to irreversible brain injury were compared to those of healthy controls at baseline, over time and with interventions. The final dataset comprised patients vs. controls in the following groupings: mild traumatic brain injury (mTBI), n = 24 vs. 27, normal pressure hydrocephalus (NPH), n = 16 vs. 9 and Alzheimer's disease (AD), n = 27 vs. 47. We generated DTI profiles from fractional anisotropy (FA) and mean, axial and radial diffusivity measures (MD, L1 and L2 and 3 respectively), and constructed an algorithm to map changes consistently to a periodic table of elements, which fully described their diffusivity and neural properties.
Results: Mapping tissue signatures to a periodic table of DTI elements rapidly characterized cohorts by their differing patterns of injury. At baseline, patients with mTBI displayed the most preserved tracts. In NPH, the magnitude of changes was dependent on “familial” DTI neuroanatomy, i.e., potential for neural distortion from risk of ventriculomegaly. With time, patients with Alzheimer's disease were significantly different to controls across multiple measures. By contrast, patients with mTBI showed both loss of integrity and pathophysiological processes of neural repair. In NPH, some patterns of injury, such as “stretch/compression” and “compression” were more reversible following intervention than others; these neural profile properties suggested “microstructural resilience” to injury.
Conclusion: Using the novel strategy of a periodic table of DTI elements, our study has demonstrated it is possible to distinguish between different cohorts along the spectrum of brain injury by describing neural profile properties of white matter disruption. Further work to contribute datasets of disease toward this proposed taxonomic framework would enhance the translatability of DTI profiles to the clinical-research interface.
Introduction
Restoration of brain functions following injury requires an understanding of the resilience of neural tissue against pathological insults. Currently, we assess white matter tracts for microstructural damage but do not describe them in terms of their potential reversibility. Participants in longitudinal cohort studies and clinical trials undergo baseline imaging to document their structural integrity and functional connectivity. However, they may have white matter injury patterns with differing capacity for neural repair or recovery. Such patients may not exhibit equivalent responses to interventions, including drug therapies or application of novel neuroprostheses. Yet, there is no standard framework to describe cohorts by their differing white matter injuries. This gap in knowledge and such differential potential of patient cohorts are likely to have been major contributors to the failures of promising drug interventions in large-scale clinical trials across acute brain injury and neurodegenerative disease.
There is an urgent need to develop more precise imaging biomarkers for correlation with assessments of intervention. In addition, our lack of understanding of how to describe the potential responsiveness of white matter injury has implications for assessing the risks vs. benefits for specific interventions in high-risk groups, such as the elderly population. The emerging field of aging neurosurgery represents such an evolving challenge. Despite the growth of the cohort of patients above 65 years presenting for surgical intervention, a cohesive approach toward a strategy for geriatric surgical needs is lacking. Elderly patients are considered high risk and yet, demonstrate potential for recovery. Specific conditions, such as chronic subdural haematoma, spinal degenerative myeloradiculopathy and hydrocephalus, have excellent results with surgical intervention (1–3). Good outcomes may be further supported with the use of risk stratification, such as frailty and comorbidity scoring. However, continuing neurological improvement may be dependent on the capacity of white matter injury for microstructural resilience. A key gap in our approach is the paucity of tools to describe the potential for neural injury to recover from pathological insults, with or without intervention. In this regard, it may be helpful to study Normal pressure hydrocephalus (NPH) as a model of reversible brain injury.
Classically described by Adams et al. (4) as a triad of gait disturbance, cognitive decline and urinary incontinence, NPH attracts up to 96% chance subjective improvement and 83% chance improvement on timed walk test at 6 months (3). Although increasing age is not a prognostic factor, various factors limit consistently good outcomes across patient groups. Challenges include developing more reliable non-invasive imaging methods for higher diagnostic certainty, with a greater capacity for predicting shunt-responsiveness (5). Symptoms attributed to the NPH triad also appear in other hydrocephalic conditions, in a spectrum from compensated to subacute obstructive forms, for which differing surgical strategies may be optimal (6). Whilst NPH is characterized by its distinctive gross ventriculomegaly, degree of ventricular dilatation is neither predictive of clinical nor functional improvement. In some cases, but not all, NPH is associated with defective cerebrospinal fluid (CSF) circulation and pressure-volume compensation. In such Classic NPH subtypes, interrogation of cerebral hydrodynamics via a CSF infusion test can be helpful in predicting improvement after shunting (7, 8). However, a further complication exists in the form of overlay with comorbid diseases. Distinctive radiological features in NPH, such as ventriculomegaly, may be common to hydrocephalus, brain atrophy as well as neurodegeneration. Neurodegenerative disorders, including Alzheimer's disease (AD), frequently co-present in the cohort with NPH symptoms. In our previous work, we have described a subtype we term “Complex NPH.” This challenging cohort presents with overlay from multiple co-morbidities co-existing, particularly vascular risk burden and neurodegenerative diseases; patients still show capacity for CSF responsiveness, but testing is difficult (9). Such subtypes of Complex NPH, or patients with frailty, may benefit from screening in vivo to characterize the reversibility potential of their neural injury prior to invasive CSF testing.
The study of NPH is confounded by multiple theories of pathogenesis, yet unresolved. However, many major hypotheses streams, such as tissue distortion and vascular ischaemia, converge in white matter dysfunction (5). Shunt-responsive NPH patients may be demonstrating a form of ‘microstructural resilience'; their white matter exhibits potential to recover from injury. Diffusion tensor imaging (DTI), has been established as a robust and reliable way of interrogating tissue microstructure, with both the capacity to document patterns of white matter injury in NPH, as well as reversibility post-shunting (10). Whilst this technique is well-accepted in conditions such as stroke, tumors and traumatic brain injury (11–15), and readily available in academic clinical centers, there are technical challenges to overcome. DTI metrics are “semi-quantitative”; dependent on (i) machine factors–site/scanner-specific, b values, number of diffusion directions, (ii) biological confounders–multiple pathophysiological processes co-existing, crossing fibers, ongoing insults/ recovery across timepoints and (iii) interpretations–post-processing algorithms and assumptions, handling of DTI conflicts. In response, there has been increasing development of higher scanning specifications and advanced imaging processing methodologies. However, such strategies reduce its attractiveness as a rapid, first-line screening tool at the clinical-research interface.
To address this conundrum, we have previously shown the utility of simplistic ROI-based DTI profiles in an at-risk model of white matter injury in NPH (10). DTI profiles are a methodology of distilling the complexity of changes, occurring across multiple DTI measures concurrently, into their most simplistic, graphical forms (9). DTI profiles display both the magnitude and direction of predominant changes to describe patterns of white matter injury in terms of their diffusion morphology. Here, we present a further contribution toward this concept. We found that, despite conflicts in DTI measures, we could observe recurring properties in DTI profiles of common patterns of white matter injury seen across cohorts. Inspired by the discussions surrounding the recent 150th anniversary of Mendeleev's periodic table in chemistry, we examined whether our observations would benefit from being organized into such an array. Mendeleev arranged his table by atomic weight, according to recurring chemical and physical properties of elements and confirmed the validity of the Periodic Law (16, 17). We believed it was possible to evolve from this concept to the notion of arranging recurring DTI profiles in a similar way (Figure 1). We therefore proposed a novel arrangement of white matter injury by their familial DTI neuroanatomy, rather than by functional considerations. As we based our table on the periodicity of “diffusivity” and “neural” properties of white matter in response to injury, we termed this a “periodic table of DTI elements.” The aims of this study were to (1) examine if DTI profiles could distinguish white matter injury in hydrocephalus vs. non-hydrocephalus and if so, (ii) create a simplified taxonomy of DTI interpretation by using the strategy of a “periodic table of DTI elements.”
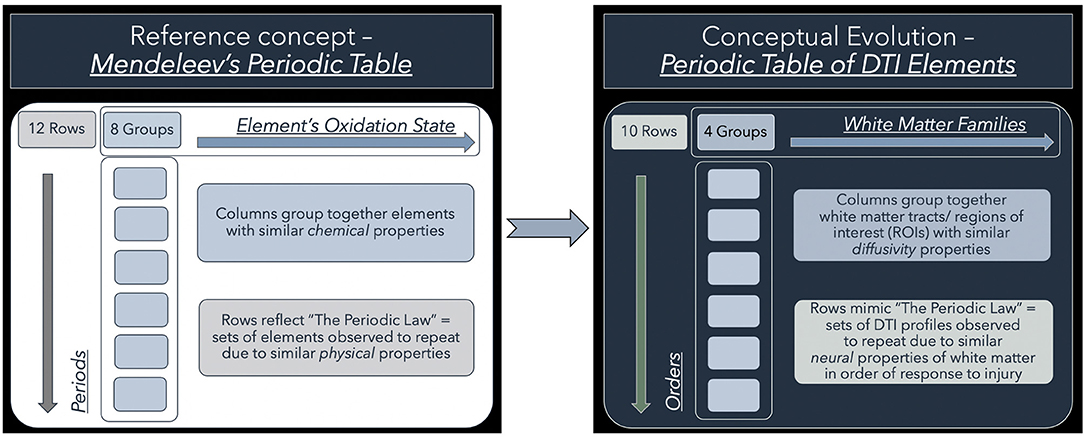
Figure 1. Conceptual evolution from Mendeleev's Periodic Table to the current work. The modern periodic table in chemistry is based on atomic number. This differs from Mendeleev's original concept, which arranged the elements according to their similar chemical and physical properties. Mendeleev sorted the elements largely by atomic mass but where there were tensions in the order, he prioritized groups by their shared properties. Similarly, we have used shared “diffusivity” properties to group white matter tracts/ regions of interest (ROIs) into columns. This grouping into “White matter families” reflects their DTI neuroanatomy, changes in diffusivity profiles due to their differential risk from progressive ventriculomegaly. Mimicking the concept of periods, we have arranged sets of DTI profiles observed to repeat due to similar “neural” properties. These rows, which we have termed “Orders,” reflect commonly occurring DTI profiles seen in white matter in response to injury. Note that the Order from I to X here reflects a predicted trend from reversible to irreversible injury. This is arbitrarily defined and could equally work in reverse order as long as the sequence is maintained.
Methods
We examined DTI profiles from a cohort of Classic NPH (ClNPH) patients, the purer form of shunt-responsive NPH, and compared them to patients with mild traumatic brain injury (mTBI; who exhibit both trajectories of recovery and persisting neurological deficits) and patients with Alzheimer's disease (AD; an exemplar cohort of irreversible brain injury due to progressive neurodegeneration). The utility of DTI examination has been shown in all three conditions; interpretations of white matter injury patterns are known (10–12, 18–23).
DTI metrics for the various clinical cohorts mTBI, ClNPH and AD–were obtained from international datasets for which methodology is well-established. Cohort demographics are described in Table 1. The mTBI cohort from the University of Malaya comprised 62 patients who presented with mTBI and 27 age-matched healthy controls (21 males and 6 females; mean age 29.0 years). In this analysis, we included only the 24 mTBI patients (20 males and 3 females; mean age 28.6 years) who had baseline DTI measures and 6-month follow-up data available. This dataset has been expanded from the previously published series (61 patients and 19 controls) (24), in which DTI profiles were not examined. Pre- and post-operative DTI measures for the ClNPH group, which included 16 patients with NPH (10 males and 6 females; mean age 74.7 years) and 9 healthy controls (4 males and 5 females; mean age 70.0 years), were derived from a cohort we have previously published from the University of Cambridge (10). We have previously demonstrated the use of DTI profiles in the ClNPH group (9, 10); we have expanded on the published data by including 6-month post-operative data in our analysis. Data for the AD cohort were obtained from the Alzheimer's Disease Neuroimaging Initiative (ADNI) database (adni.loni.usc.edu) and included 27 patients with AD baseline and 12-month follow-up DTI measures available (20 males and 7 females; mean age 75.6 years) and 47 healthy controls (22 males and 25 females; mean age 72.9 years). Using data expanded from published series was critical to testing the novel strategy of the periodic table of DTI elements as interpretation of injury patterns had already been established.
Approval for individual studies was obtained from local or institutional ethics committees. Written informed consent was obtained from all subjects or legal representatives, if appropriate, as required by local ethics committees.
DTI Acquisition
The mTBI cohort from the University of Malaya was imaged on a 3T MRI scanner (Signa HDx; General Electric, Harvey, IL) using an eight-channel head coil (24). The DTI sequence was obtained using these parameters: TR = 13,000 ms; TE = 81.2 ms; FOV = 24 mm; matrix = 128 x 128; slice thickness = 3.0 mm; 32 directions; diffusion weighted factor, b = 700 s/mm2; and image scan time of 7 min 22 s.
MRI for the NPH cohort was performed on a 3T Siemens Tim Trio using a 12 channel head matrix radio frequency receive coil (10). The DTI sequence was acquired by using a spin echo diffusion weighted echo planar imaging sequence with the following parameters: TR/TE, 8,300 ms/98 ms, matrix dimensions 96 x 96, FOV 192 x 192, slice thickness 2 mm giving a voxel size of 2 x 2 x 2 mm. Diffusion weighted images were acquired in 12 non-collinear directions each at 5 b-values of 350, 650, 1,000, 1,300 and 1,600 s/mm2, along with 4 b-0 images.
The AD cohort from the ADNI were scanned on 3T GE Medical Systems scanners at 14 acquisition sites in North America (25). Diffusion weighted images were acquired with the following parameters: 256 × 256 matrix; voxel size: 2.7 × 2.7 × 2.7 mm3; TR = 9,000 ms; scan time = 9 min. Forty-six separate images were acquired for each DTI scan: 5 T2-weighted images with no diffusion sensitization (b0 images) and 41 diffusion-weighted images (b = 1,000 s/mm2).
Pre-processing and ROI Analysis
Pre-processing, image registration, and analysis for the mTBI dataset was carried out with FSL and AFNI software packages. Initial preprocessing involved corrections for head movement and eddy currents, brain tissue extraction, and fitting of the diffusion tensor model. The FSL tool fnirt was used for non-linear special registration of each subject to the FMRIB58_FA standard-space image. Predefined ROIs for each individual subject were mapped with the AFNI 3dROIstats tool and median FA, MD, L1, and L2 and 3 values obtained for each tract (24).
In the NPH dataset, the FDT diffusion toolbox in FSL analysis tools was used for eddy current correction and DTI pre- and post-processing. Co-registration of the structural 3D volumetric image and DTI images was performed using SPM5. MPRAGE images were re-sliced to match the DTI image space using the s0 volume (the b = 0 volume with optimal signal-to-noise ratio). A DTI-based white matter tract atlas was used for anatomical identification of key fiber bundles and ROIs were placed on the structural images and applied to co-registered DTI files (10).
ADNI DTI metrics used in this paper were the UCLA DTI ROI summary measures for ADNIGO and ADNI2. Pre-processing steps for head motion and eddy current correction and removal of non-brain tissue were done using FSL. T1-weighted anatomical images were aligned to a standard brain template; skull-stripped b0 images were aligned and then registered to their respective T1-weighted scans. A study-specific template was created, each individual subject's initial FA map elastically registered to the template, and resulting deformation fields applied to diffusivity maps to align them to the same coordinate space. The mean of all voxels from each of the regions of interest from the atlas were obtained (25).
Generation of DTI Profiles
DTI region of interest (ROI) values for fractional anisotropy (FA), mean diffusivity (MD), axial diffusivity (L1), and radial diffusivity (L2 and 3) obtained using established methodology were used in this analysis (10, 24, 25). Axial and radial diffusivities are referred to by their eigenvalues (L1 and L2 and 3, respectively) to avoid confusion with acronyms for pathology, e.g., AD for Alzheimer's Disease. Previous work in the NPH study confirmed no significant differences in right- and left-sided ROIs, therefore right-sided tracts were used for this study to avoid over-averaging across multiple ROIs and within groups. However, as midline tracts were acquired as single ROIs for the NPH and mTBI groups, the averaged value of right and left body and genu of the corpus callosum in the AD group was used for comparison. We generated DTI profiles as per the workflow in Figure 2. Here, we preferred Pareto graphs; this graphical format automatically displays positive DTI measures in order of decreasing magnitude of changes, thereby allowing concurrent examination of both predominant direction and magnitude of differences. To reflect smaller, and usually negative changes in FA, we also correlated findings seen on the Pareto graphs with bar charts and standard statistical analyses (Figures 3–6, Tables 2, 3).
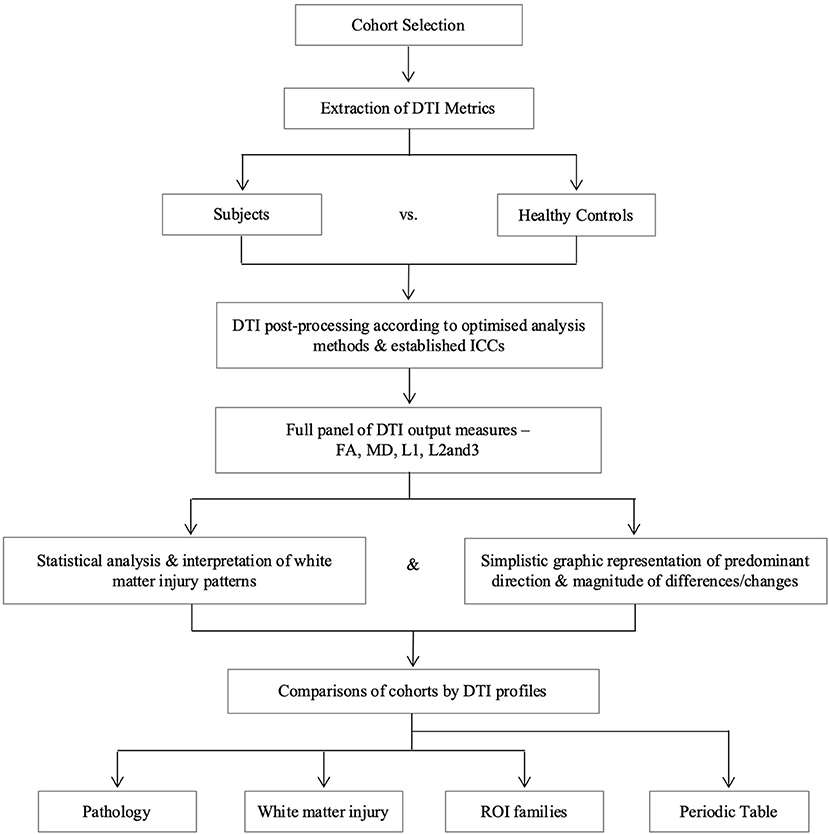
Figure 2. Overview of study methodology. We refer to axial and radial diffusivities by their eigenvalues for clarity (L1 and L2 and 3, respectively), to distinguish these measures from terms we use for pathological cohorts. DTI, diffusion tensor imaging; FA, fractional anisotropy; ICC, intraclass correlation coefficient; L1, axial diffusivity, L2 and 3, radial diffusivity; MD, mean diffusivity; ROI, region of interest.
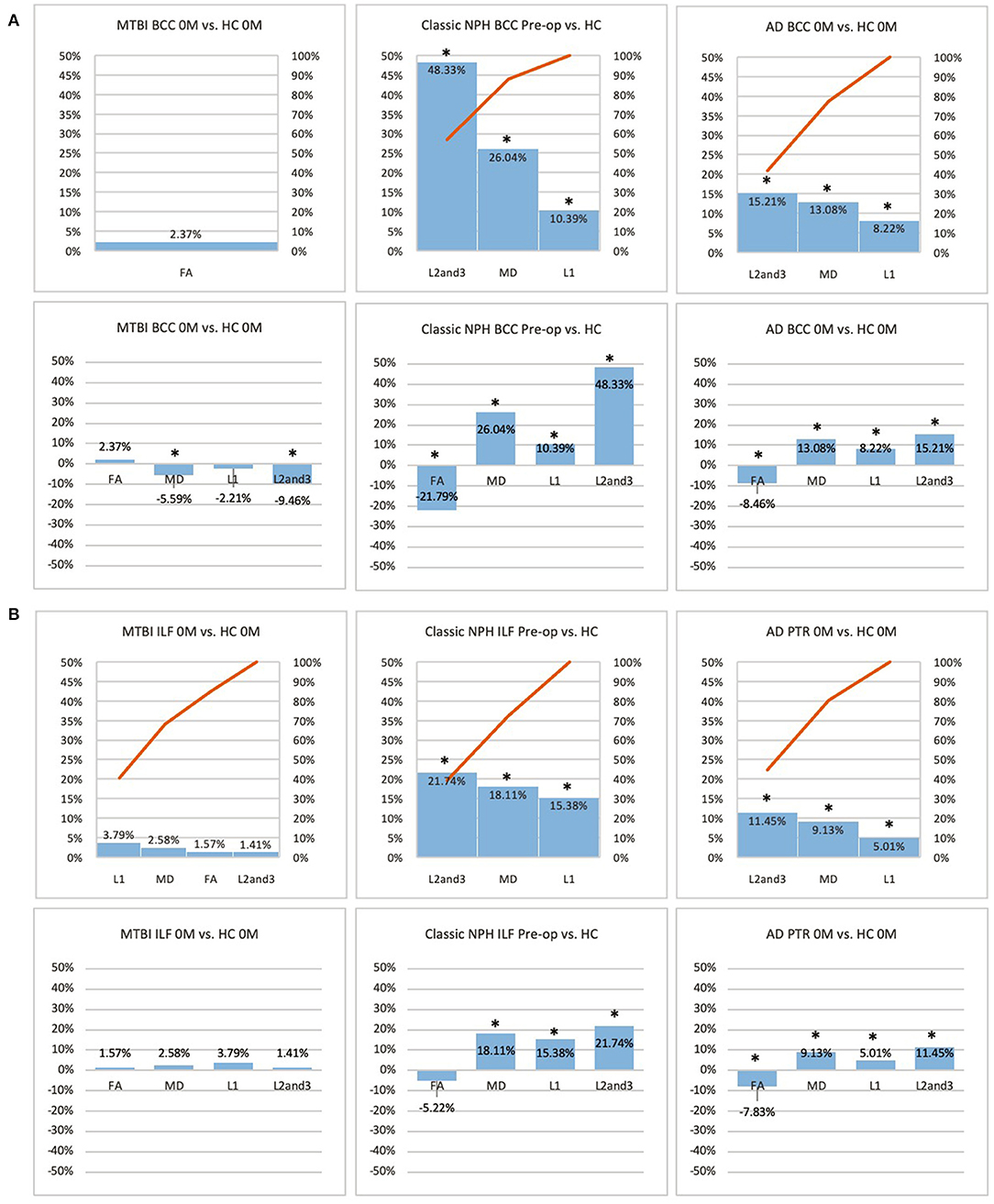
Figure 3. (A) Midline (BCC) and (B) lateral (ILF or PTR) ROI families at baseline. At baseline, percentage differences in DTI measures of clinical cohorts are compared against corresponding healthy controls, presented as Pareto graphs and bar charts. In Pareto graphs, positive values are automatically arranged sequentially, in order of highest to lowest magnitude of differences/changes. *Indicates a significant Difference between the clinical cohort and healthy controls at p < 0.05.
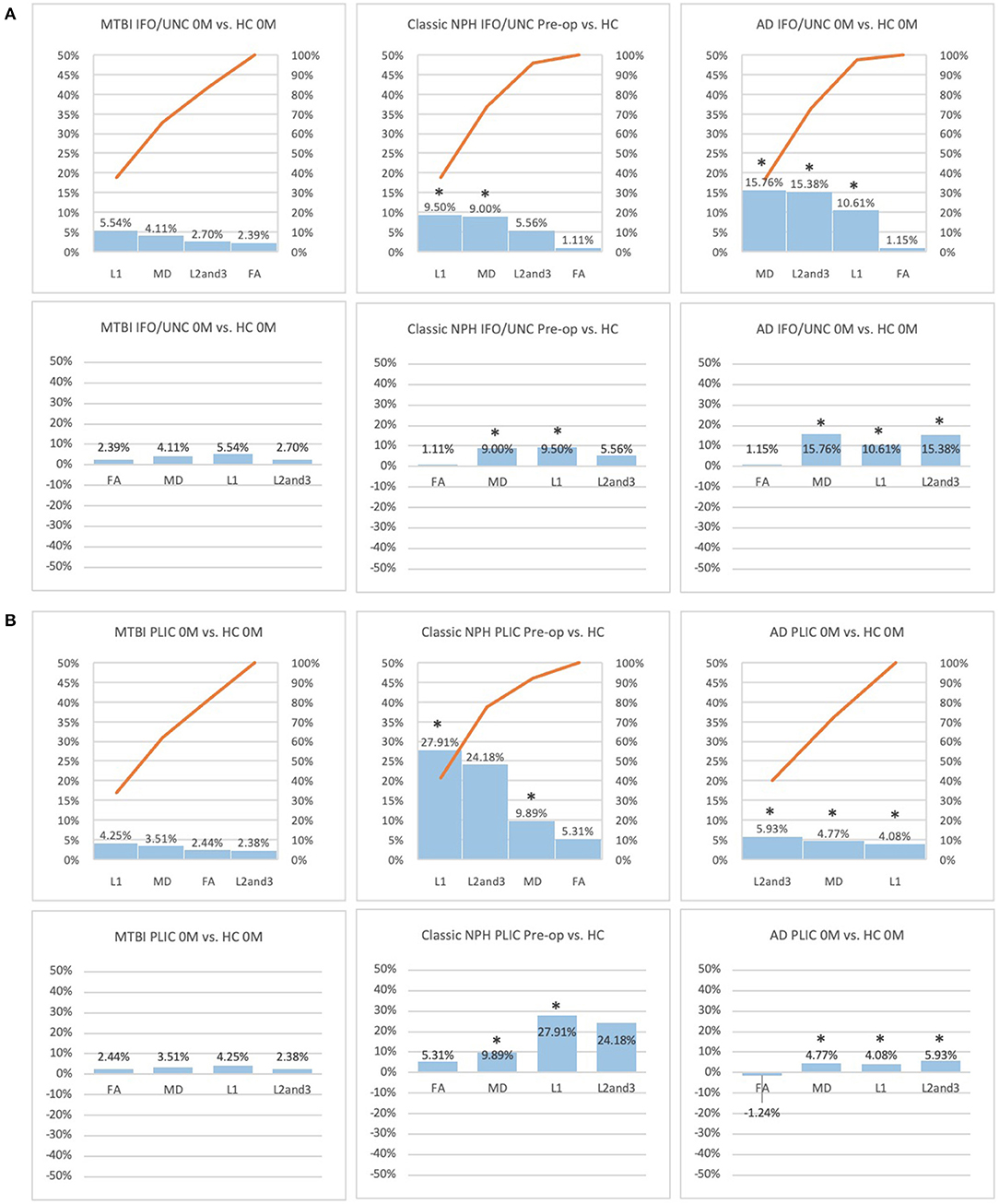
Figure 4. (A) Fronto-temporal (IFO/UNC) and (B) remote functional (PLIC) ROI families at baseline. At baseline, percentage differences in DTI measures of clinical cohorts are compared against corresponding healthy controls, presented as Pareto graphs and bar charts. In Pareto graphs, positive values are automatically arranged sequentially, in order of highest to lowest magnitude of differences/changes. * indicates a significant difference between the clinical cohort and healthy controls at p < 0.05.
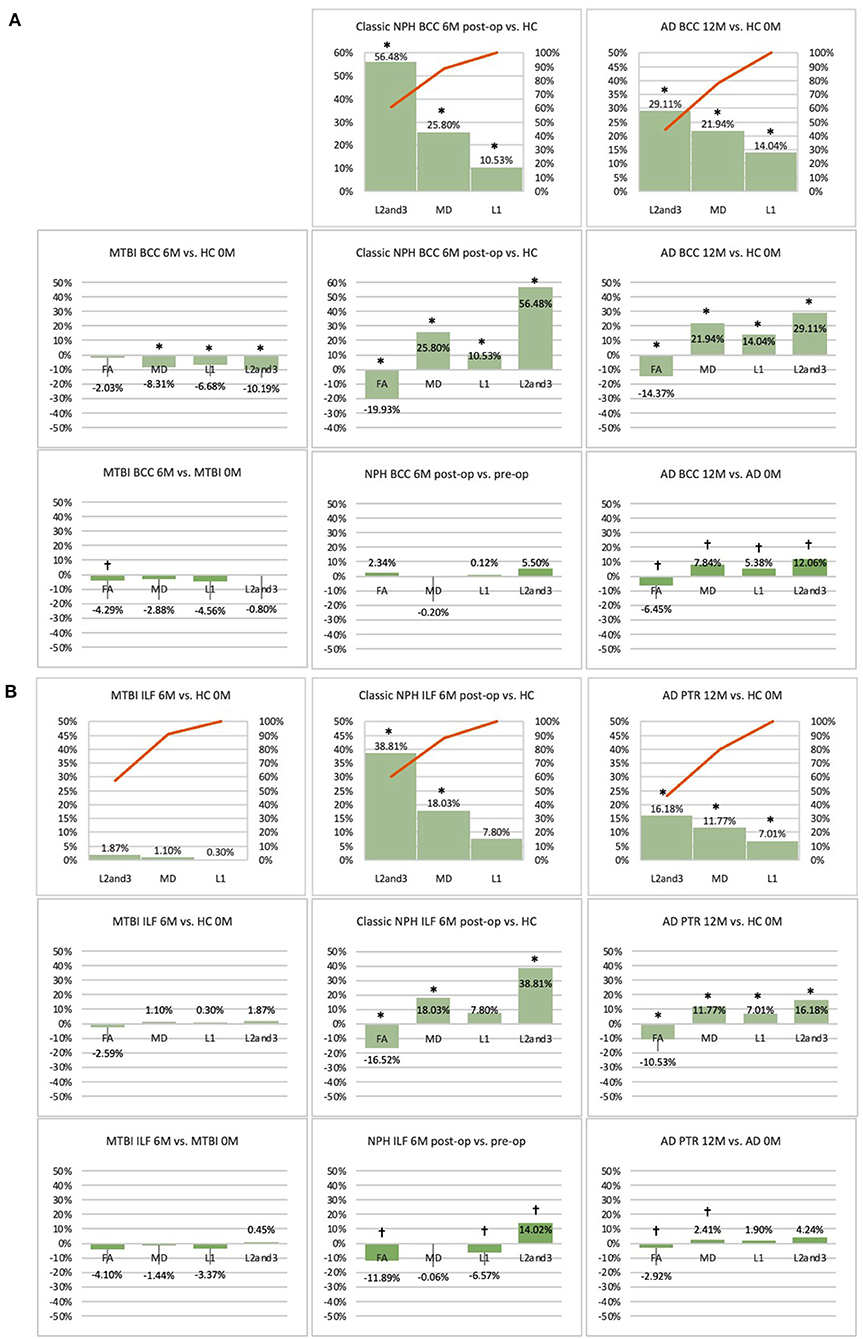
Figure 5. (A) Midline (BCC) and (B) lateral (ILF or PTR) ROI families at follow-up. At 6 or 12-month follow-up, percentage changes in DTI measures of clinical cohorts are compared against corresponding healthy controls at baseline, presented as Pareto graphs and bar charts. At this time point, patients are also compared to themselves at baseline, presented as bar charts. We observed that, in MTBI, the BCC ROI at 6-month follow-up demonstrated an unique profile (significant decreases in mean, axial and radial diffusivity measures in the context of a non-significant decrease in FA). We suggest this is possibly indicative of processes seen in the spectrum of neural repair. At this timepoint in NPH, we found changes driven by a disproportionate increase in radial diffusivity, a DTI profile reported in other subtypes of post-operative hydrocephalus. In AD at 12-month follow-up, we found global significant deterioration across mean, axial and radial diffusivity measures, consistent loss of integrity/atrophy. By contrast, we observed that, for the ILF or PTR ROI, there was preservation of white matter tracts in MTBI at 6-month follow-up. At this timepoint in NPH, we found changes indicative of improvement in compression (a significant increase in radial diffusivity but in the context of a decrease in axial diffusivity). In AD at 12-month follow-up, we observed significant worsening of white matter due to increase in loss of integrity/atrophy. *Indicates a significant Difference between the clinical cohort and healthy controls at p < 0.05. †Indicates a significant Change in the clinical cohort across timepoints at p < 0.05.
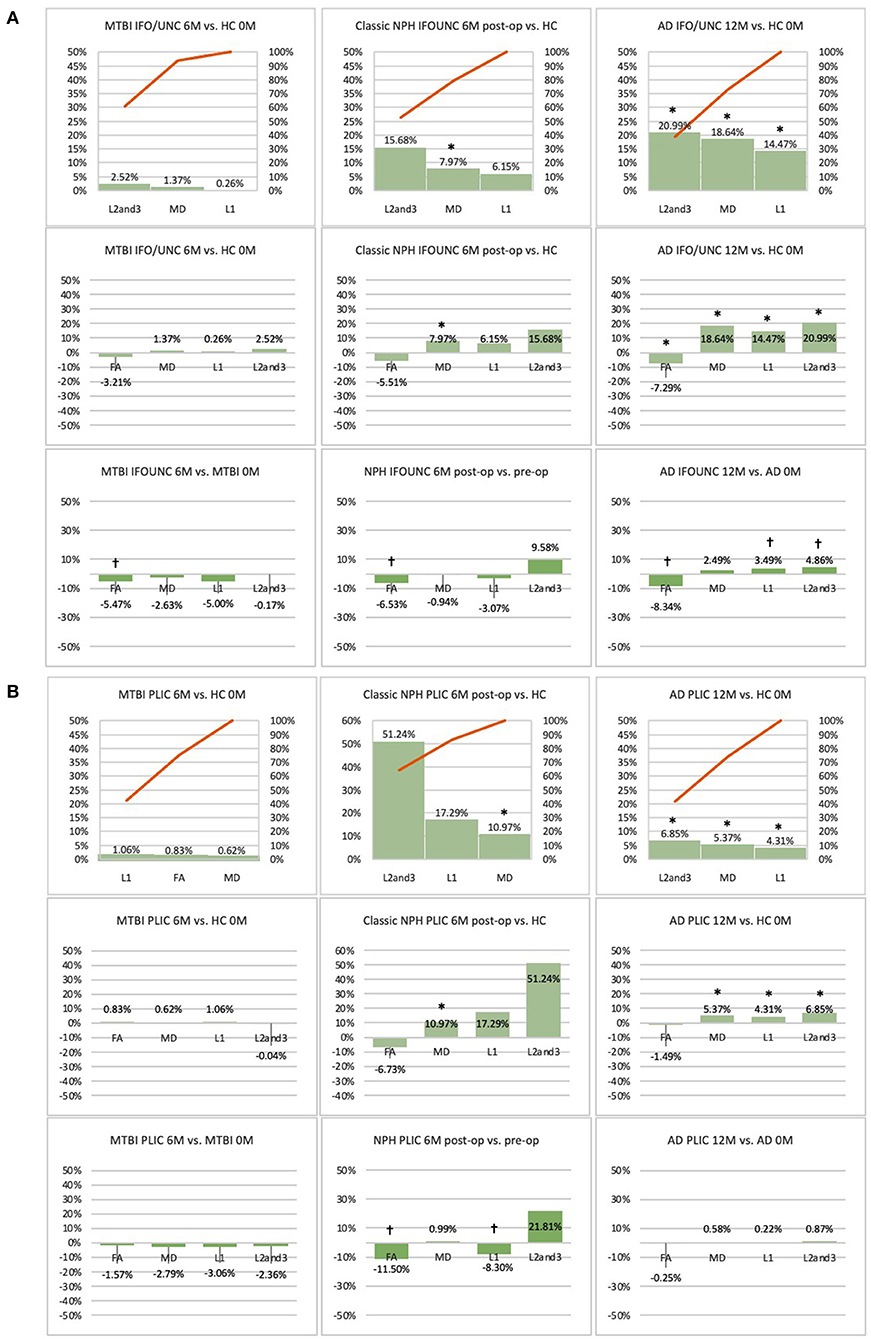
Figure 6. (A) Fronto-temporal (IFO/UNC) and (B) remote functional (PLIC) ROI families at follow-up. At 6 or 12-month follow-up, percentage changes in DTI measures of clinical cohorts are compared against corresponding healthy controls at baseline, presented as Pareto graphs and bar charts. At this time point, patients are also compared to themselves at baseline, presented as bar charts. We observed that, in MTBI, the IFO/UNC combination ROI at 6-month follow-up demonstrated a pattern of distortion/oedema (predominant increase in radial diffusivity with a significant decrease in FA), whereas in NPH, we found changes consistent with non-significant improvement in distortion of the tract (increase in radial diffusivity but in the context of decreases in axial and mean diffusivities). In AD at 12-month follow-up, we found global deterioration across all diffusivity measures (with a significant decrease in FA) consistent with progressive neurodegeneration. By contrast, we observed that, for the PLIC ROI, there was preservation of white matter tracts in MTBI at 6-month follow-up and in NPH, improvement in compression (increase in radial diffusivity but in the context of a significant decrease in axial diffusivity). In AD at 12-month follow-up, we observed non-significant worsening of white matter due to likely neurodegeneration. *Indicates a significant Difference between the clinical cohort and healthy controls at p < 0.05. †Indicates a significant Change in the clinical cohort across timepoints at p < 0.05.
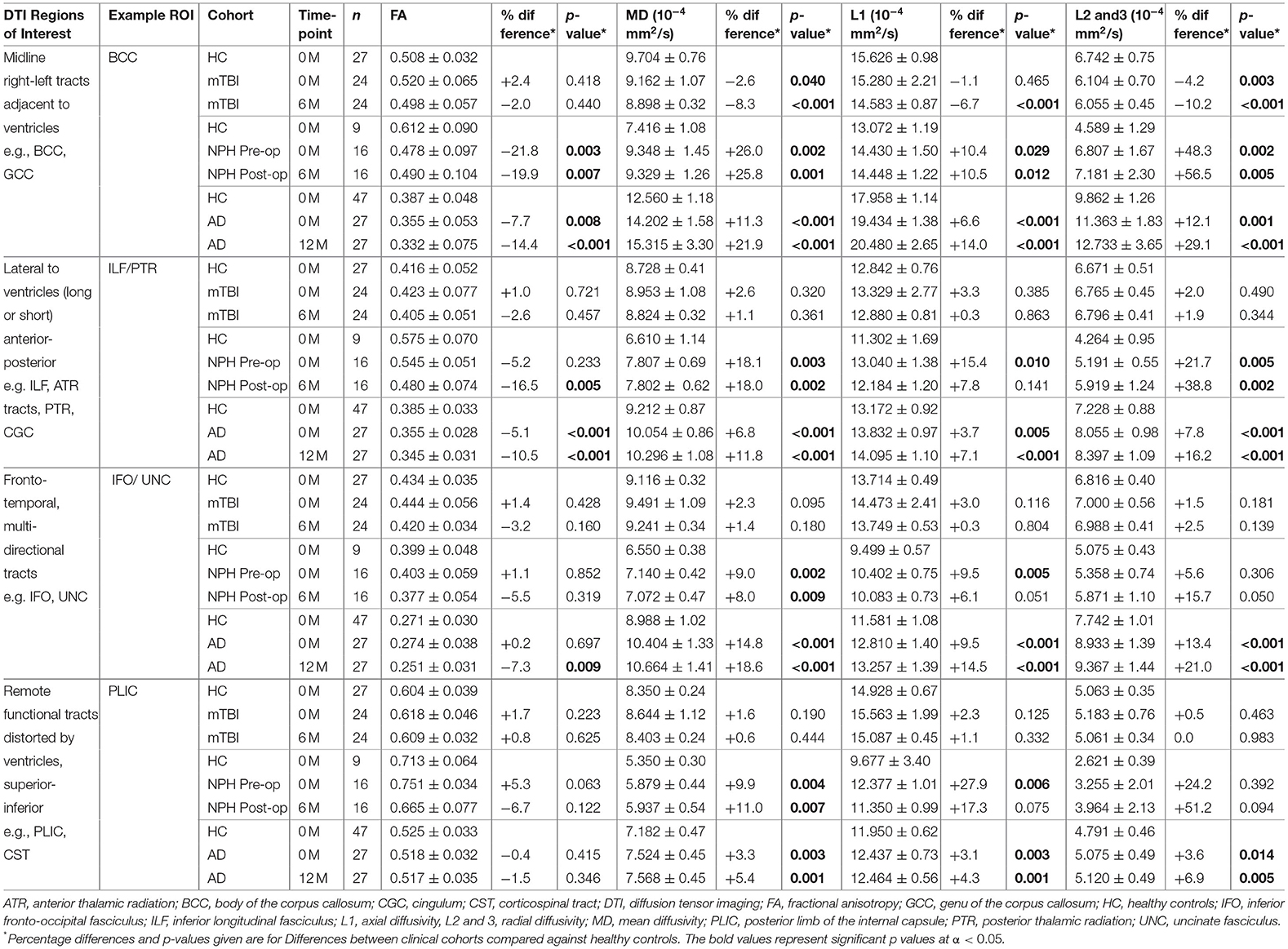
Table 2. Comparison of example DTI region of interest values between clinical cohorts and healthy controls.
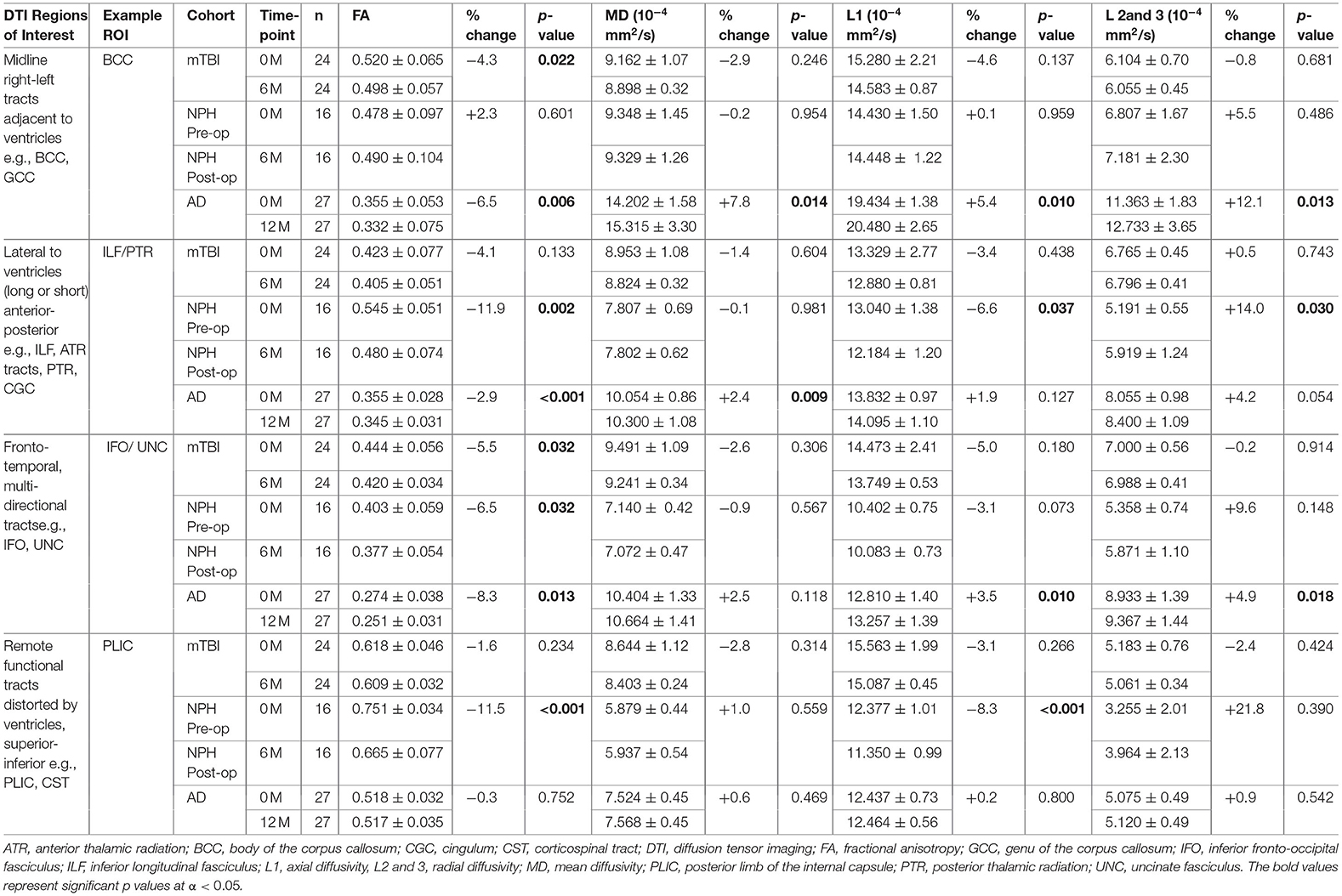
Table 3. Comparison of example DTI region of interest values within clinical cohorts at baseline and at 6 or 12-month follow-up.
Arrangement of White Matter Injury by DTI Neuroanatomy and Profile Properties
We observed recurring common properties in DTI profiles across the spectrum of brain injury and developed a novel arrangement of white matter injury as a “periodic table of DTI elements” (Figure 1, Table 4). We grouped ROIs into columns of “white matter families” according to the distortion potential to their DTI neuroanatomy from risk of ventriculomegaly (Figure 7). We then determined the “Order” of DTI profiles according to predicted reversibility of white matter injury. We reviewed the literature and considered references across multiple neurological conditions of interest that listed the full panel of DTI measures (FA, MD, L1, and L2 and 3), and where pathophysiological findings of disease correlated with DTI interpretation. Following multiple iterations of the initial prototype to accommodate findings from the literature across pathologies, we determined the final hierarchical algorithm for mapping results to the periodic table (Table 5, Figure 8).
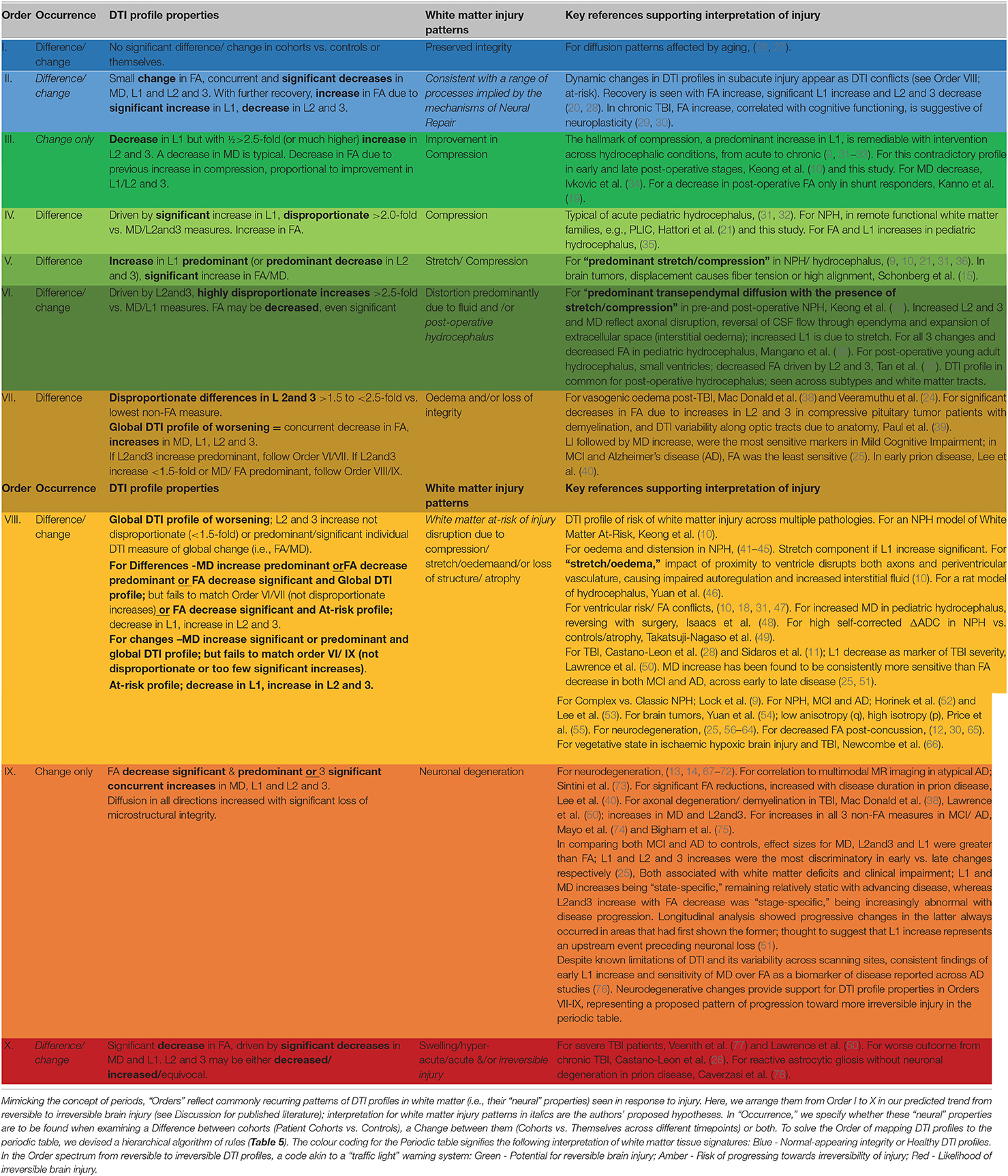
Table 4. Recurring common properties of DTI profiles, arranged by expected order of white matter reversibility.
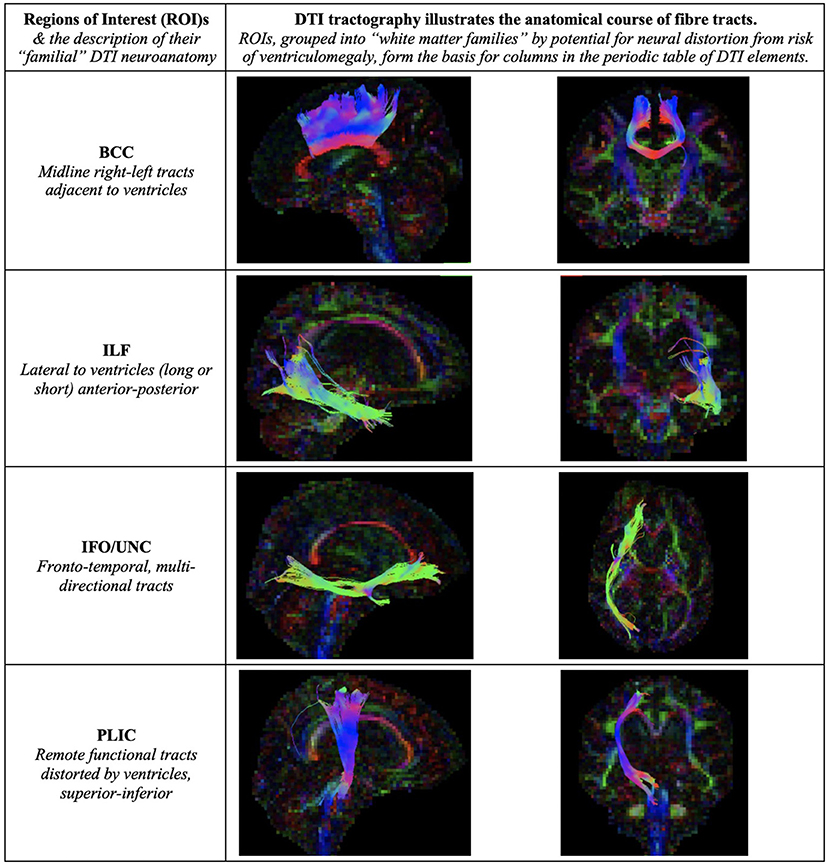
Figure 7. Illustration of Regions of Interest (ROIs). We grouped ROIs into “white matter families” by their predicted potential for neural distortion due to risk of ventriculomegaly. The familial nature of these white matter tracts can be seen in the common anatomical arrangements of their fibers, e.g., right-left or superior-inferior in direction. When grouped in this way, rather than by functional considerations, we demonstrate how tractal families share diffusivity properties. This is the basis for columns in the periodic table of DTI elements. BCC, body of the corpus callosum; ILF, inferior longitudinal fasciculus; IFO/UNC, combination ROI of the inferior fronto-occipital/ uncinate fasciculi; PLIC, posterior limb of internal capsule.
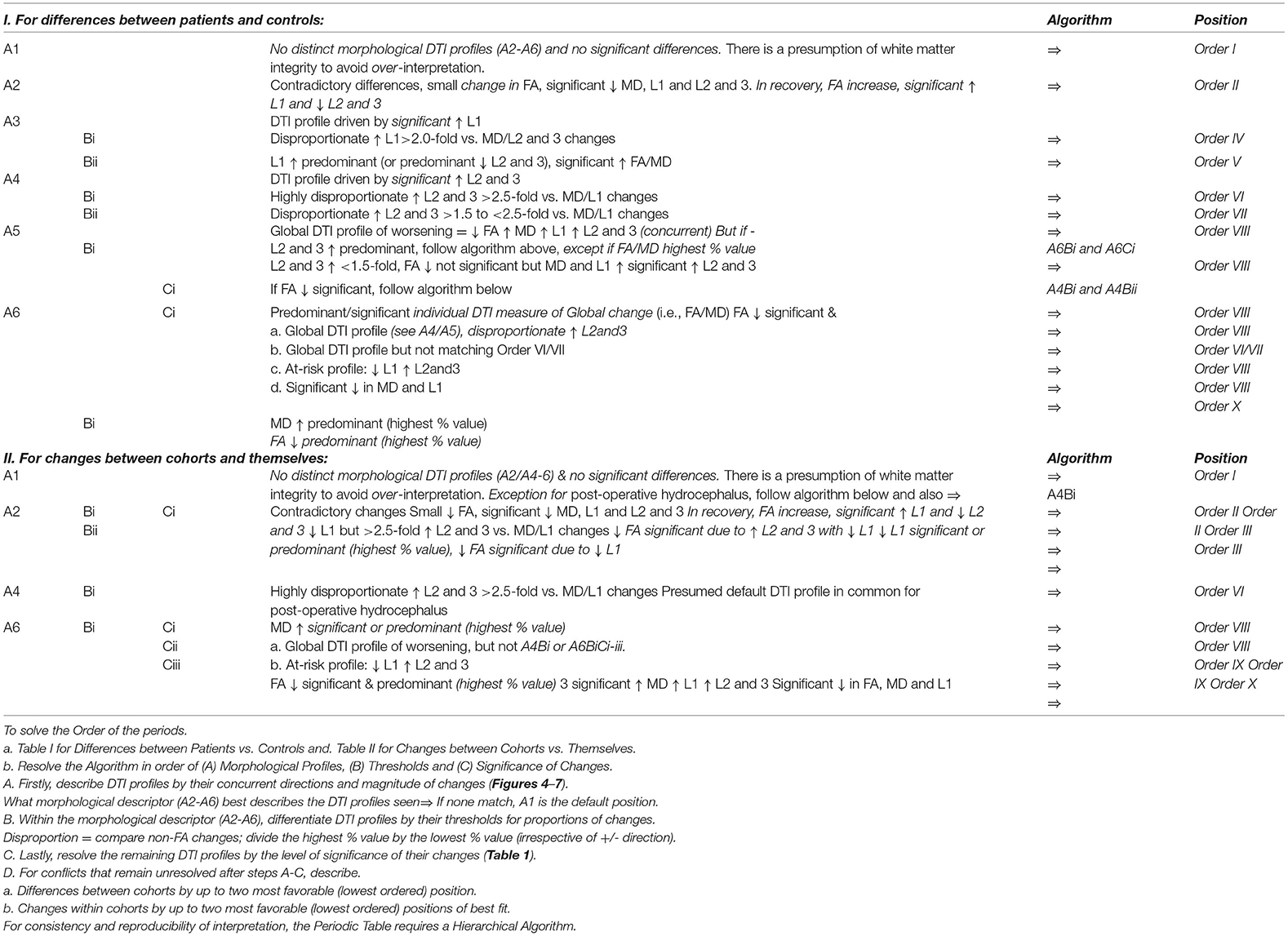
Table 5. Hierarchical algorithm for mapping DTI profiles to the Periodic Table. We mapped Differences between patient cohorts vs. healthy controls at baseline and 6- or 12-month follow-up and Changes between cohorts vs. themselves across timepoints (Tables 2, 3, Figures 3–6). For consistency and reproducibility of interpretation, the Periodic Table requires a Hierarchical Algorithm.
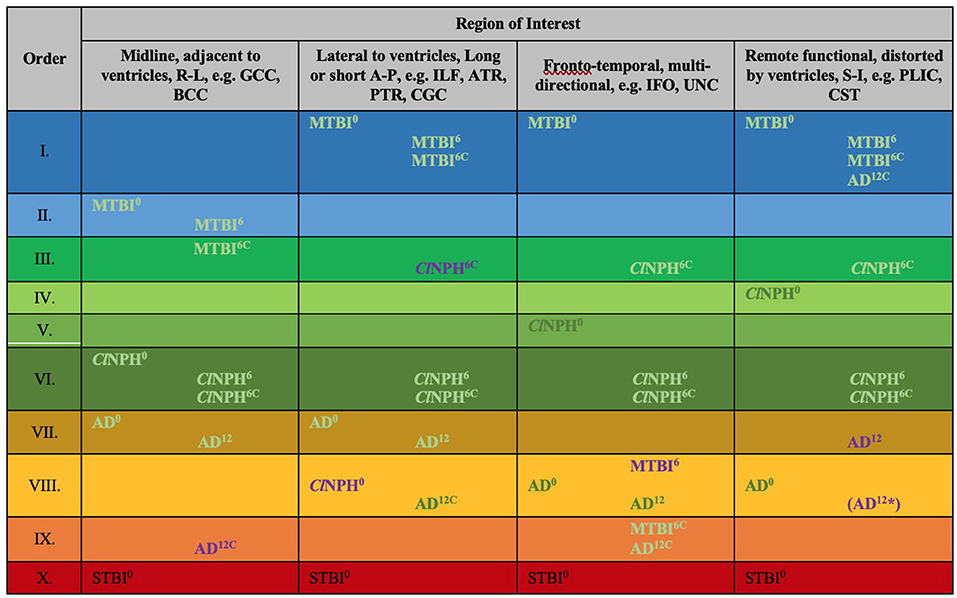
Figure 8. Periodic table of DTI elements. Recurring common properties of DTI profiles arranged vertically by predicted reversibility of white matter injury (see Table 4 and Discussion). ROIs grouped in columns of ‘white matter families' according to distortion potential from ventriculomegaly. Differences at baseline for clinical cohorts at 0 months vs. healthy controls at 0 months mapped onto the periodic table. In addition to baseline DTI profiles, cohorts mapped across timepoints; MTBI6, ClNPH6 and AD12 cohorts are mapped at 6 and 12 months respectively against healthy controls at 0 months; ClNPH6C and MTBI6C are mapped at 6 month follow-up against the respective clinical cohorts at 0 months. Order X has been derived from STBI data in a published cohort by Veenith et al. (77); coincidentally, this unrelated study was performed on the same scanner as the ClNPH cohort being described in this work. 0/6/12, DTI profiles at 0 month baseline, 6/12 month follow-up, C, denotes cohorts vs. themselves across timepoints, A-P, anterior-posterior; AD, Alzheimer's Disease; BCC, body of the corpus callosum; ClNPH, Classic NPH; DTI, diffusion tensor imaging; FA, fractional anisotropy; IFO, inferior fronto-occipital fasciculus; ILF, inferior longitudinal fasciculus; L1, axial diffusivity, L2 and 3, radial diffusivity; MD, mean diffusivity; MTBI, mild traumatic brain injury; PLIC, posterior limb of the internal capsule; PTR, posterior thalamic radiation; R-L, right-left; ROI, region of interest; S-I, superior-inferior; STBI, severe traumatic brain injury; UNC, uncinate fasciculus.
Statistical Analysis
Statistical analyses were conducted using SPSS Statistics Version 23.0 (IBM Corp., Armonk, NY, USA). Data is reported as mean ± standard deviation, unless otherwise stated. Independent samples t-tests were used to test for differences between clinical cohorts and healthy controls, and paired samples t-tests for changes in clinical cohorts over time. All statistical tests were two-tailed, and significance level was set at p < 0.05.
Results
DTI Profiles Derived at Baseline Across the Spectrum of Brain Injury
At baseline, it was possible to distinguish patient cohorts vs. healthy controls in NPH and AD using DTI profiles alone. When comparing differences in AD patients vs. controls, white matter families in the midline, lateral to the ventricles and remote functional tracts showed evidence of oedema (Figures 3, 4, Tables 2, 4; Order VII). The magnitude of differences was greatest for fronto-temporal tracts, described by Order VIII (white matter at risk) confirming its relevance toward AD pathology.
By contrast, the reverse was true for NPH, where magnitude of changes were worst for white matter tracts in the midline and lateral to the ventricles. The most preserved white matter families were remote functional, followed by fronto-temporal tracts (Figures 3, 4, Tables 2, 4). It was possible to reproduce at least three distinct patterns of white matter injury. The body of the corpus callosum was characterized by Order VI; the inferior longitudinal fasciculus showed worse changes at Order VII; stretch/oedema. By contrast, fronto-temporal and remote functional tracts displayed differences consistent with stretch/compression and compression (Orders V and IV, respectively).
Patients with mTBI displayed white matter tracts that were entirely preserved at baseline compared to healthy controls across all white matter families tested (Figures 3, 4, Tables 2, 4; Order I). By comparison, we further generated DTI profiles from a published dataset of severe TBI (sTBI) patients undergoing normobaric hyperoxia (Veenith et al. (77), an independent study coincidentally performed at the same site as the NPH cohort). We have represented this as Order X due to the life-threatening nature of acute brain injury (Table 4); this does not preclude a change to a more reversible Order with time or following intervention, using the strategy of the periodic table of DTI elements. There were no follow-up results presented in this published dataset.
The Effect of Time and Intervention on DTI Profiles After Brain Injury
Using the cohort with AD from ADNI, we found that it was possible to demonstrate the effect of progression of neurodegeneration. At 12 months' follow-up, the white matter changes progressed in all midline, lateral and fronto-temporal tracts tested (Figures 5, 6, Tables 2–4). When patients were compared to themselves, changes in the midline and fronto-temporal tracts confirmed neuronal degeneration (AD12C, Order IX; Figures 5, 6). For tracts lateral to the ventricles, the posterior thalamic radiation showed evidence of loss of integrity/ atrophy (AD12C, Order VIII. Only remote functional tracts showed no significant deterioration between cohorts (AD12C, Order I), although the cohort at 12 months remained severely disrupted compared to controls at baseline.
In NPH, at 6 months, differences driven by radial diffusivity that were highly disproportionate to other measures remained despite intervention (Order VI). This DTI profile was common to all post-operative white matter families, suggesting that it may be a DTI tissue signature of shunted hydrocephalus. In tracts midline to the ventricles, the pattern of distortion/ oedema (Order VI) was not amenable to improvement. However, white matter families with DTI profiles of stretch/oedema, stretch/compression and compression showed improvement in compression (ClNPH6C, Order III).
Interestingly, patients with mTBI, who had uniformly displayed preserved white matter tracts compared to controls at baseline, demonstrated two contrasting new patterns. Changes in fronto-temporal tracts in patients at 6 months' follow-up vs. themselves at baseline were consistent with Order IX, suggesting neuronal degeneration (Figure 6, Tables 3, 4). In the midline tracts, changes evolved at 6 months consistent with hypothesized mechanisms of neural repair (Figure 5, Tables 3, 4; Order II). Figure 8 demonstrates DTI profiles mapped onto the Periodic Table.
Discussion
In this paper, we examined the utility of creating a simplified taxonomy, to promote transparency and consistency in, and reduce complexity of, interpreting DTI profiles across a spectrum of brain injury. When we grouped white matter tracts by their familial DTI neuroanatomy, rather than their functional organization, we found recurring patterns of DTI measures across cohorts. By deconstructing these morphological patterns into DTI profiles, we were able to observe their periodicity, and so arrange white matter responses according to their diffusivity and neural properties. These properties distinguished white matter injury, when comparing cohorts of mTBI, ClNPH and AD, by the order of their potential reversibility. Using this novel strategy of a periodic table of DTI elements, we demonstrated it was possible to characterize cohorts of hydrocephalus vs. non-hydrocephalus and their varying changes over time, and with interventions. The capacity to describe white matter distortion across brain injury cohorts by their neural properties supports a higher diagnostic certainty of neural disruption and more precise evaluation of outcomes from current and emerging therapies. Taken together, the periodic table of DTI elements, and NPH as a human model of reversible disease, show how white matter can recover from varying mechanisms of injury. We term this concept of reversible injury a form of “microstructural resilience.”
At present, such a strategy is lacking; at baseline, cohorts are routinely described by their structural imaging features and less commonly, their white matter metrics, but not by the resilience of their “neural materials.” This is important because reliance on single DTI metrics alone may lead to misleading reports. In literature, fractional anisotropy (FA) is associated with structural integrity, and therefore, a drop in FA is found in most neurological disorders. This reduction in FA would be consistent with microstructural damage of fibers, such as seen in demyelination and axonal degeneration. However, we have found that in hydrocephalus, FA can be shown to both increase and decrease (10, 79). For example, Kanno et al. and our group have reported that a reduction in FA at baseline compared to healthy controls, along with an increase in mean diffusivity (MD), is compatible with cohorts of NPH shunt-responders (10, 18). However, they have also shown a decrease in post-operative FA, only in shunt-responders (19). Whilst this may appear to be an FA conflict, we were able to accommodate both findings, arranged as Order VIII pre-operatively for “white matter at-risk” and Order III post-operatively for “improvement in compression,” using the periodic table of DTI elements. Indeed, Assaf et al. originally reported both significantly increased and decreased FA in acute pediatric hydrocephalus, with significant decrease in FA (internal capsule) post-surgery (31, 32). Tan et al. also found brain-wide reductions in FA in successfully shunted young hydrocephalic patients (37). Conversely, Hattori et al. and others have also convincingly demonstrated that FA values in NPH patients were higher than in healthy controls (21). Mechanical distortion from ventriculomegaly may increase compaction of fibers along the direction of main diffusivity measures, leading to high FA values. Significant increases in FA or MD may be due to changes driven by predominant increases in L1, even if significant decreases in L2 and 3 are present (9, 10, 21, 31, 35, 36). These DTI profiles of “compression” and “stretch/compression” appear in the periodic table as neural properties differentiated by proportion; Orders IV and V, respectively.
Apparent DTI conflicts and inconsistencies reflect both the known mathematical derivation of DTI metrics (FA and MD being heavily dependent on changes occurring across other diffusivity measures), as well as specific patterns of white matter tracts and their distortions by CSF compartments described in NPH, such as ventriculomegaly and disproportionately enlarged subarachnoid space hydrocephalus (DESH) (80). Differing brain regions may respond differently to forces of pressure or deformation. The inherent “neural material properties” of white matter affected by pathological processes may determine their capacity for microstructural resilience vs. progressive neuronal degeneration. Differentiation of DTI profiles is important; it allows for multiple patterns of white matter injury to co-exist within individual cohorts. In NPH, we have shown that an a priori model of at-risk white matter tracts comprising only six key ROIs is sufficient to demonstrate at least three distinct and concurrent patterns of neural distortion (10). DTI profiles differed according to their risk of proximity to the expanding ventricles. In this study, we have used the strategy of a periodic table of DTI elements to reproduce the three known patterns of neural distortion seen in NPH, now refined as Orders IV, V and VI, respectively. We showed that some patterns of injury were more reversible than others. Early post-intervention, we found “predominant stretch/compression” to be the most amenable to reversibility; this contradictory decrease in L1 with concurrent increase in L2 and 3, now described as Order III for “improvement in compression,” preceded changes in clinical outcome (10). Here, at six months post-shunting, we found “compression” and “stretch/ compression” to be the most reversible patterns of injury (i.e., pre-operative Orders IV and V, respectively), matching findings from our previous work. Using the periodic table, this profile of improvement is described by Order III. Interestingly, by mapping cohorts to the periodic table of elements, it became apparent that, despite differing pre-operative DTI measures, all post-operative hydrocephalus tracts had a DTI profile in common. Order VI, in which FA decrease is driven by highly disproportionate increases in L2 and 3, was also observed by Tan et al. in their young adult hydrocephalic cohort without ventriculomegaly (37). This suggests Order VI may be a shared tissue DTI signature of treated, post-operative hydrocephalus. In addition, all tracts with improvement shared the DTI profile of Order III.
In this study, we sought to compare DTI profiles in hydrocephalus compared to brain injury and neurodegeneration within the spectrum of reversible to irreversible injury. Using the strategy of a periodic table of DTI elements, it has been possible to demonstrate where patterns of neural injury diverge, or where they are overlap. The cohorts in Orders VII to VIII of the periodic table match ADNI studies suggesting that ventriculomegaly may be an early imaging signature of AD and/or NPH. The progression in the AD cohort at 12 months toward neuronal degeneration (Order IX; in midline and fronto-temporal tracts), is consistent with known interpretations of ADNI data; white matter damage in AD follows neurodegenerative staging and progression of disease (75). Despite the known limitations of DTI and its variability across scanning sites, consistent DTI profiles, that of early L1 increase, sensitivity of MD over FA as a biomarker of disease and L2 and 3 increase with FA decrease being increasingly abnormal with disease progression, have been reported across AD studies (25, 51, 76). This provides further support of the morphology of DTI profiles described in Orders VII-IX, representing a proposed progression from reversible to irreversible injury in the arrangement of the periodic table of DTI elements. Our findings also support the model of acute-to-chronic traumatic brain injury with differing patterns of neural response and recovery. In the inferior longitudinal/ uncinate fasciculi, changes at 6 months suggested neuronal degeneration (Order IX). Previous work from a subset of this cohort confirmed that, patients demonstrated cognitive deficits within this timeframe (24). By contrast, at 6 months, DTI profiles seen in the midline tracts (Order II) would be consistent with expected diffusion patterns if pathophysiological processes of neural repair, such as dendritic pruning, influx of inflammatory cells, deposition of biomarker proteins and disorganized neural regeneration, were occurring. Further work is required to characterize these changes, and in particular, confirm that DTI profiles represented by Order II match reports of improvement of cognitive functioning and other deficits in the literature, suggestive of neuroplasticity (29, 30). Presently, the interpretation of DTI profiles is highly dependent upon studies of unambiguous pathophysiological processes, such as stretch distortion/compression due to tumors, variable patterns of oedema (interstitial, vasogenic, cytotoxic), demyelination and Wallerian degeneration [Orders V-IX]. Therefore, in constructing the periodic table, we also sought out further interpretations in literature for comparisons of DTI measures across pediatric to adult hydrocephalus, experimental models of injury, acute-to-chronic TBI, tumors and forms of neurodegeneration (Table 4 for key references), in order to situate DTI profiles within their appropriate context for comparisons.
Limitations
Our study has a number of limitations. Firstly, ROI methodology has known disadvantages, as compared to more advanced analysis techniques such as semi-automated tractography. However, in our work and that of others, high intraclass correlation coefficients have been reported in clinical use, confirming both its reliability as a research method and applicability to standard practice. Secondly, due to methodological considerations, inter-site DTI variability is a known concern, as diffusivity measures are highly dependent upon machine specifications and acquisition parameters. Whilst this therefore renders it challenging to directly compare DTI output across international collaborative sites, it is still possible to appreciate strong morphological features comprising specific DTI profiles of disease, such as differential diffusion metric sensitivity in early vs. late stages of AD [50, 75; see (Table 4)]. In this study, we have made comparisons across multiple international cohorts but have only compared patients against controls from their own individual sites, thereby avoiding such pitfalls of DTI variability. Furthermore, our findings in normal pressure hydrocephalus are consistent across the variety of techniques employed in literature for DTI post-processing and interpretation (manual to semi-automated methodologies, comprising voxel-based analysis, tract-based spatial statistics, deterministic fiber tractography and ROI-based analyses) (10, 25, 31, 79, 81, 82) confirming the robustness of DTI in its descriptions of microstructural injury.
In reviewing the literature, we included in the analysis sources that listed the full panel of DTI measures, and where pathophysiological findings of disease correlated with DTI interpretation. However, we were limited in that not all papers of interest published their full panel of DTI measures. As global DTI measures are influenced by disproportionate changes in individual metrics, we tried to include papers that took this into account. Additionally, for purposes of clarity, there may be a limit to the number of datasets that can be presented via a periodic table. DTI profiles may be better suited to visual representation in other ways, such as being plotted as a normal distribution curve, or as milestones along a spectrum line from reversible to irreversible brain injury. Subclassifications of DTI profiles may be needed to more accurately represent cohorts with overlapping tissue signatures. Whilst we tested three cohorts vs. controls and one exemplar within a much wider spectrum of brain injury, we expect this methodology to be translatable to other conditions comprising acute-to-chronic pathology. Crucially, the order of potential reversibility of white matter injury is determined a priori.
The concept of the periodic table is proposed as a strategy to navigate the pitfalls of DTI interpretation, rather than trying to solve its known shortcomings. This would be the first description of a taxonomy for classifying and describing DTI profiles, and we expect that this will only be its first iteration. We shall seek to address the limitations and considerations mentioned in future work; we hope that community efforts may contribute toward this concept.
Conclusion
Our study has demonstrated that it is possible to distinguish between different cohorts along the spectrum of brain injury by describing the properties of their DTI white matter profiles. We proposed a simplified taxonomy to improve the reproducibility and reduce the complexity of DTI interpretation to differentiate cohorts of hydrocephalus vs. non-hydrocephalus using a novel strategy of a periodic table of DTI elements. In this way, DTI profiles provide, both at baseline and in response to interventions, a form of rapid characterization of cohorts within the context of known tissue signatures across pathologies. As potential reversibility of white matter injury is assessed in vivo, this methodology may contribute to the understanding of the use of biomarkers to track changes in disease cohorts.
Data Availability Statement
The raw data supporting the conclusions of this article will be made available by the authors upon reasonable request.
Ethics Statement
The studies involving human participants were reviewed and approved by local or institutional Ethics Committees. Written informed consent was obtained from all subjects or legal representatives, if appropriate, as required by local Ethics Committees. The mTBI study was regulated by the University of Malaya Research Ethics Committee and Hospital Ethics Committee (UM/EC Ref: 947.15). The NPH study was approved by the local Research Ethics Committee of the Cambridge Health Authority (LREC: 06/Q0108/330). Individual ADNI sites received approval from their respective governing Institutional Review Boards. The patients/participants provided their written informed consent to participate in this study.
Author Contributions
NK conceptualized the experimental design. NK, CL, and SS analyzed the data. NK and CL wrote the manuscript, as well as provided visualizations for the data and led revisions of the work. AH contributed to data acquisition, imaging post-processing, and analysis from the UM site. ZC, MC, JP, and VN contributed to the acquisition of data used in this paper and provided comments and suggestions toward improving revisions. All authors reviewed the manuscript.
Funding
NK was supported by a National Medical Research Council Clinician Scientist Award (MOH-000303-00) and Transition Award (NMRC/TA/0024/2013) and the National Neuroscience Institute RIE2020 Centre Grant Bridging Fund (IRNMR17CBG01). NPH study imaging at the University of Cambridge was funded by a Medical Research Council Programme Grant [Wolfson Brain Imaging Centre Cooperative]. MC and ZC were supported by Revert Project, Interreg France (Channel Manche) England, funded by ERDF. JP has received a National Institute for Health Research Brain Injury Health Technology Cooperative and National Institute for Health Research Senior Investigator Award (2009–2014). MTBI data from the University of Malaya was partially funded by a University Malaya Research Grant (UMRG; RG008C-13HTM) and a High Impact Research Grant of University of Malaya (HIR-UM.C/625/1/HIRMOHE/12).
Conflict of Interest
The authors declare that the research was conducted in the absence of any commercial or financial relationships that could be construed as a potential conflict of interest.
Publisher's Note
All claims expressed in this article are solely those of the authors and do not necessarily represent those of their affiliated organizations, or those of the publisher, the editors and the reviewers. Any product that may be evaluated in this article, or claim that may be made by its manufacturer, is not guaranteed or endorsed by the publisher.
Acknowledgments
Data for the Alzheimer's Disease cohort used in this article were obtained from the Alzheimer's Disease Neuroimaging Initiative (ADNI) database (adni.loni.usc.edu). As such, the investigators within the ADNI contributed to the design and implementation of ADNI and/or provided data but did not participate in analysis or writing of this report. A complete listing of ADNI investigators can be found at: http://adni.loni.usc.edu/wp-content/uploads/how_to_apply/ADNI_Acknowledgement_List.pdf. The ADNI was launched in 2003 as a public-private partnership, led by Principal Investigator Michael W. Weiner, MD. The primary goal of ADNI has been to test whether serial magnetic resonance imaging (MRI), positron emission tomography (PET), other biological markers, and clinical and neuropsychological assessment can be combined to measure the progression of mild cognitive impairment (MCI) and early Alzheimer's disease (AD). For up-to-date information, see www.adni-info.org.
References
1. Lee L, Ker J, Ng HY, Munusamy T, King NK, Kumar D, et al. Outcomes of chronic subdural hematoma drainage in nonagenarians and centenarians: a multicenter study. J Neurosurg. (2016) 124:546–51. doi: 10.3171/2014.12.JNS142053
2. Machino M, Ando K, Kobayashi K, Ito K, Tsushima M, Matsumoto A, et al. The feature of clinical and radiographic outcomes in elderly patients with cervical spondylotic myelopathy: a prospective cohort study on 1025 patients. Spine. (2018) 43:817–23. doi: 10.1097/BRS.0000000000002446
3. Halperin JJ, Kurlan R, Schwalb JM, Cusimano MD, Gronseth G, Gloss D. Practice guideline: idiopathic normal pressure hydrocephalus: Response to shunting and predictors of response: report of the guideline development, dissemination, and implementation subcommittee of the american academy of neurology. Neurology. (2015) 85:2063–71. doi: 10.1212/WNL.0000000000002193
4. Adams RD, Fisher CM, Hakim S, Ojemann RG, Sweet WH. Symptomatic occult hydrocephalus with normal cerebrospinal-fluid pressure – a treatable syndrome. N Engl J Med. (1965) 273:117–26. doi: 10.1056/NEJM196507152730301
5. Keong NCH, Pena A, Price SJ, Czosnyka M, Czosnyka Z, Pickard JD. Imaging normal pressure hydrocephalus – theories, techniques and challenges. Neurosurg Focus. (2016) 41:E11. doi: 10.3171/2016.7.FOCUS16194
6. Lo YT, Kirollos RW, Chen MW, Cheong TM, Keong NCH. Acqueductal compression by dilated Virchow-Robin spaces in the mesencephalic-pontine region presenting with symptoms mimicking normal pressure hydrocephalus: a case report and review of literature. World Neurosurg. (2020) 138:390–7. doi: 10.1016/j.wneu.2020.03.047
7. Lalou AD, Czosnyka M, Donnelly J, Pickard JD, Nabbanja E, Keong NC, et al. Cerebral autoregulation, cerebrospinal fluid outflow resistance, and outcome following cerebrospinal fluid diversion in normal pressure hydrocephalus. J Neurosurg. (2018) 130:154–62. doi: 10.3171/2017.7.JNS17216
8. Nabbanja E, Czosnyka M, Keong NC, Garnett M, Pickard JD, Lalou DA, et al. Is there a link between ICP-derived infusion test parameters and outcome after shunting in normal pressure hydrocephalus? Acta Neurochir Suppl. (2018) 126:229–32. doi: 10.1007/978-3-319-65798-1_46
9. Lock C, Kwok J, Kumar S, Ahmad-Annuar A, Narayanan V, Ng ASL, et al. DTI profiles for rapid description of cohorts at the clinical-research interface. Front Med. (2019) 5:357. doi: 10.3389/fmed.2018.00357
10. Keong NC, Pena A, Price SJ, Czosnyka M, Czosnyka Z, DeVito EE, et al. Diffusion tensor imaging profiles reveal specific neural tract distortion in normal pressure hydrocephalus. PLoS ONE. (2017) 12:e0181624. doi: 10.1371/journal.pone.0181624
11. Sidaros A, Engberg AW, Sidaros K, Liptrot MG, Herning M, Petersen P, et al. Diffusion tensor imaging during recovery from severe traumatic brain injury and relation to clinical outcome: a longitudinal study. Brain. (2008) 131:559–72. doi: 10.1093/brain/awm294
12. Newcombe VF, Williams GB, Nortje J, Bradley PG, Harding SG, Smielewski P, et al. Analysis of acute traumatic axonal injury using diffusion tensor imaging. Br J Neurosurg. (2007) 21:340–8. doi: 10.1080/02688690701400882
13. Werring DJ, Toosy AT, Clark CA, Parker GJ, Barker GJ, Miller DH, et al. Diffusion tensor imaging can detect and quantify corticospinal tract degeneration after stroke. J Neurol Neurosurg Psychiatry. (2000) 69:269–72. doi: 10.1136/jnnp.69.2.269
14. Assaf Y, Pasternak O. Diffusion tensor imaging (DTI)-based white matter mapping in brain research: a review. J Mol Neurosci. (2008) 34:51–61. doi: 10.1007/s12031-007-0029-0
15. Schonberg T, Pianka P, Hendler T, Pasternak O, Assaf Y. Characterization of displaced white matter by brain tumors using combined DTI and fMRI. Neuroimage. (2006) 30:1100–11. doi: 10.1016/j.neuroimage.2005.11.015
16. García-Martínez J. Controversies, compromises and the common chemical language. Nat Chem. (2019) 11:853–6. doi: 10.1038/s41557-019-0336-4
18. Kanno S, Abe N, Saito M, Takagi M, Nishio Y, Hayashi A, et al. White matter involvement in idiopathic normal pressure hydrocephalus: a voxel-based diffusion tensor imaging study. J Neurol. (2011) 258:1949–57. doi: 10.1007/s00415-011-6038-5
19. Kanno S, Saito M, Kashinoura T, Nishio Y, Iizuka O, Kikuchi H, et al. A change in brain white matter after shunt surgery in idiopathic normal pressure hydrocephalus: a tract-based spatial statistics study. Fluids Barriers CNS. (2017) 14:1. doi: 10.1186/s12987-016-0048-8
20. Hattori T, Ito K, Aoki S, Yuasa T, Sato R, Ishikawa M, et al. White matter alteration in idiopathic normal pressure hydrocephalus: tract-based spatial statistics study. AJNR Am J Neuroradiol. (2012) 33:97–103. doi: 10.3174/ajnr.A2706
21. Hattori T, Yuasa T, Aoki S, Sato R, Sawaura H, Mori T, et al. Altered microstructure in corticospinal tract in idiopathic normal pressure hydrocephalus: comparison with Alzheimer disease and Parkinson disease with dementia. AJNR Am J Neurol. (2011) 32:1681–7. doi: 10.3174/ajnr.A2570
22. Iddon JL, Pickard JD, Cross JJ, Griffiths PD, Czosnyka M, Sahakian BJ. Specific patterns of cognitive impairment in patients with idiopathic normal pressure hydrocephalus and Alzheimer's disease: a pilot study. J Neurol Neurosurg Psychiatry. (1999) 67:723–32. doi: 10.1136/jnnp.67.6.723
23. Holodny AI, Waxman R, George AE, Rusinek H, Kalnin AJ, de Leon M, et al. Differential diagnosis of normal-pressure hydrocephalus and Alzheimer disease: significance of perihippocampal fissures. AJNR Am J Neuroradiol. (1998) 19:813–9.
24. Veeramuthu V, Narayanan V, Kuo TL, Delano-Wood L, Chinna K, Bondi MW, et al. Diffusion tensor imaging parameters in mild traumatic brain injury and its correlation with early neuropsychological impairment: a longitudinal study. J Neurotrauma. (2015) 32:1497–509. doi: 10.1089/neu.2014.3750
25. Nir TM, Jahanshad N, Villalon-Reina JE, Toga AW, Jack CR, Weiner MW, et al. Effectiveness of regional DTI measures in distinguishing Alzheimer's disease, MCI, and normal aging. Neuroimage Clin. (2013) 3:180–95. doi: 10.1016/j.nicl.2013.07.006
26. Chun T, Filippi CG, Zimmerman RD, Ulug AM. Diffusion changes in the aging human brain. AJNR Am Journal Neurol. (2000) 21:1078–83.
27. Bennett IJ, Madden DJ, Vaidya CJ, Howard DV, Howard JH Jr. Age-related differences in multiple measures of white matter integrity: a diffusion tensor imaging study of healthy aging. Hum Brain Mapp. (2010) 31:878–90. doi: 10.1002/hbm.20872
28. Castaño-Leon AM, Cicuendez M, Navarro B, Paredes I, Munarriz PM, Cepeda S, et al. Longitudinal analysis of corpus callosum diffusion tensor imaging metrics and its association with neurological outcome. J Neurotrauma. (2019) 36:2785–802. doi: 10.1089/neu.2018.5978
29. Chiou KS, Jiang T, Chiaravalloti N, Hoptman MJ, DeLuca J, Genova H. Longitudinal examination of the relationship between changes in white matter organization and cognitive outcome in chronic TBI. Brain Inj. (2019) 33:846–53. doi: 10.1080/02699052.2019.1606449
30. Yin B, Li DD, Huang H, Gu CH, Bai GH, Hu LX, et al. Longitudinal changes in diffusion tensor imaging following mild traumatic brain injury and correlation with outcome. Front Neural Circuits. (2019) 13:28. doi: 10.3389/fncir.2019.00028
31. Assaf Y, Ben-Sira L, Constantini S, Chang LC, Beni-Adani L. Diffusion tensor imaging in hydrocephalus: initial experience. AJNR Am J Neuroradiol. (2006) 27:1717–24.
32. Ben-Sira L, Goder N, Bassan H, Lifshits S, Assaf Y, Constantini S. Clinical benefits of diffusion tensor imaging in hydrocephalus. J Neurosurg Pediatr. (2015) 16:195–202. doi: 10.3171/2014.10.PEDS13668
33. Jurcoane A, Keil F, Szelenyi A, Pfeilschifter W, Singer OC, Hattingen E. Directional diffusion of corticospinal tract supports therapy decisions in idiopathic normal-pressure hydrocephalus. Neuroradiology. (2014) 56:5–13. doi: 10.1007/s00234-013-1289-8
34. Ivkovic M, Reiss-Zimmermann M, Katzen H, Preuss M, Kovanlikaya I, Heier L, et al. MRI assessment of the effects of acetazolamide and external lumbar drainage in idiopathic normal pressure hydrocephalus. Fluids Barriers CNS. (2015) 12:9. doi: 10.1186/s12987-015-0004-z
35. Mangano FT, Altaye M, McKinstry RC, Shimony JS, Powell SK, Phillips JM, et al. Diffusion tensor imaging study of pediatric patients with congenital hydrocephalus: 1-year postsurgical outcomes. J Neurosurg Pediatr. (2016) 18:306–19. doi: 10.3171/2016.2.PEDS15628
36. Kamiya K, Hori M, Irie R, Miyajima M, Nakajima M, Kamagata K, et al. Diffusion imaging of reversible and irreversible microstructural changes within the corticospinal tract in idiopathic normal pressure hydrocephalus. Neuroimage Clin. (2017) 14:663–71. doi: 10.1016/j.nicl.2017.03.003
37. Tan K, Meiri A, Mowrey WB, Abbott R, Goodrich JT, Sandler AL, et al. Diffusion tensor imaging and ventricle volume quantification in patients with chronic shunt-treated hydrocephalus: a matched case-control study. J Neurosurg. (2018) 129:1611–22. doi: 10.3171/2017.6.JNS162784
38. Mac Donald CL, Dikranian K, Bayly P, Holtzman D, Brody D. Diffusion tensor imaging reliably detects experimental traumatic axonal injury and indicates approximate time of injury. J Neurosci. (2007) 27:11869–76. doi: 10.1523/JNEUROSCI.3647-07.2007
39. Paul DA, Gaffin-Cahn E, Hintz EB, Adeclat GJ, Zhu T, Williams ZR, et al. White matter changes linked to visual recovery after nerve decompression. Sci Transl Med. (2014) 6:266ra173. doi: 10.1126/scitranslmed.3010798
40. Lee H, Cohen OS, Rosenmann H, Rummukainen J, Sutela A, Vanninen R, et al. Cerebral white matter disruption in Creutzfeldt-Jakob disease. AJNR Am J Neuroradiol. (2012) 33:1945–50. doi: 10.3174/ajnr.A3125
41. Leinonen V, Koivisto AM, Savolainen S, Rummukainen J, Sutela A, Vanninen R, et al. Post- mortem findings in 10 patients with presumed normal-pressure hydrocephalus and review of the literature. Neuropathol Appl Neurobiol. (2012) 38:72–86. doi: 10.1111/j.1365-2990.2011.01195.x
42. Del Bigio MR, Deck JH, Davidson GS. Glial swelling with eosinophilia in human post-mortem brains: a change indicative of plasma extravasation. Acta Neuropathol. (2000) 100:688–94. doi: 10.1007/s004010000236
43. Greitz T. Effect of brain distension on cerebral circulation. Lancet. (1969) 1:863–5. doi: 10.1016/S0140-6736(69)91903-5
44. Hakim S, Venegas JG, Burton JD. The physics of the cranial cavity, hydrocephalus and normal pressure hydrocephalus: mechanical interpretation and mathematical model. Surg Neurol. (1976) 5:187–210.
45. Akai K, Uchigasaki S, Tanaka U, Komatsu A. Normal pressure hydrocephalus. Neuropathological study Acta Pathol Jpn. (1987) 37:97–110. doi: 10.1111/j.1440-1827.1987.tb03137.x
46. Yuan W, McAllister JP 2nd, Lindquist DM, Gill N, Holland SK, Henkel D, et al. Diffusion tensor imaging of white matter injury in a rat model of infantile hydrocephalus. Childs Nerv Syst. (2012) 28:47–54. doi: 10.1007/s00381-011-1590-y
47. Eleftheriou A, Blystad I, Tisell A, Gasslander J, Lundin F. Indication of thalamo-cortical circuit dysfunction in idiopathic normal pressure hydrocephalus: a tensor imaging study. Sci Rep. (2020) 10:6148. doi: 10.1038/s41598-020-63238-7
48. Isaacs AM, Shimony JS, Morales DM, Castaneyra-Ruiz L, Hartman A, Cook M, et al. Feasibility of fast brain diffusion MRI to quantify white matter injury in pediatric hydrocephalus. J Neurosurg Pediatr. (2019) 19:1–8. doi: 10.3171/2019.5.PEDS18596
49. Takatsuji-Nagaso M, Miyati T, Ohno N, Mase M, Kasai H, Shibamoto Y, et al. Hemodynamically self-corrected ΔADC analysis in idiopathic normal pressure hydrocephalus. Br J Radiol. (2019) 92:20180553. doi: 10.1259/bjr.20180553
50. Lawrence TP, Steel A, Ezra M, Speirs M, Pretorius PM, Douaud G, et al. MRS and DTI evidence of progressive posterior cingulate cortex and corpus callosum injury in the hyper-acute phase after traumatic brain injury. Brain Inj. (2019) 33:854–68. doi: 10.1080/02699052.2019.1584332
51. Acosta-Cabronero J, Alley S, Williams GB, Pengas G, Nester PJ. Diffusion tensor metrics as biomarkers in Alzheimer's disease. PLoS ONE. (2012) 7:e49072. doi: 10.1371/journal.pone.0049072
52. Horínek D, Štěpán-Buksakowska I, Szabó N, Erickson BJ, Tóth E, Šulc V, et al. Difference in white matter microstructure in differential diagnosis of normal pressure hydrocephalus and Alzheimer's disease. Clin Neurol Neurosurg. (2016) 140:52–9. doi: 10.1016/j.clineuro.2015.11.010
53. Lee SH, Coutu JP, Wilkens P, Yendiki A, Rosas HD, Salat DH, et al. Tract-based analysis of white matter degeneration in Alzheimer's disease. Neuroscience. (2015) 301:79–89. doi: 10.1016/j.neuroscience.2015.05.049
54. Yuan W, Holland SK, Jones BV, Crone K, Mangano FT. Characterization of abnormal diffusion properties of supratentorial brain tumors: a preliminary diffusion tensor imaging study. J Neurosurg Pediatr. (2008) 1:263–9. doi: 10.3171/PED/2008/1/4/263
55. Price SJ, Peña A, Burnet NG, Jena R, Green HA, Carpenter TA, et al. Tissue signature characterisation of diffusion tensor abnormalities in cerebral gliomas. Eur Radiol. (2004) 14:1909–17. doi: 10.1007/s00330-004-2381-6
56. Wen Q, Mustafi SM Li J, Risacher SL, Tallman E, Brown SA, et al. White matter alterations in early-stage Alzheimer's disease: a tract-specific study. Alzheimer's Dement. (2019) 11:576–87. doi: 10.1016/j.dadm.2019.06.003
57. Snir JA, Bartha R, Montero-Odasso M. White matter integrity is associated with gait impairment and falls in mild cognitive impairment. Results from the gait and brain study. Neuroimage Clin. (2019) 24:101975. doi: 10.1016/j.nicl.2019.101975
58. Amlien IK, Fjell AM. Diffusion tensor imaging of white matter degeneration in Alzheimer's disease and mild cognitive impairment. Neuroscience. (2014) 276:206–15. doi: 10.1016/j.neuroscience.2014.02.017
59. Wang Y, West JD, Flashman LA, Wishart HA, Santulli RB, Rabin LA, et al. Selective changes in white matter integrity in MCI and older adults with cognitive complaints. Biochim Biophys Acta. (2012) 1822:423–30. doi: 10.1016/j.bbadis.2011.08.002
60. Kiuchi K, Kitamura S, Taoka T, Yasuno F, Tanimura M, Matsuoka K, et al. Gray and white matter changes in subjective cognitive impairment, amnestic mild cognitive impairment and Alzheimer's disease: a voxel-based analysis study. PLoS ONE. (2014) 9:e104007. doi: 10.1371/journal.pone.0104007
61. Selnes P, Fjell AM, Gjerstad L, Bjørnerud A, Wallin A, Due-Tønnessen P, et al. White matter imaging changes in subjective and mild cognitive impairment. Alzheimer's Dement. (2012) 8(5 Suppl):S112–21. doi: 10.1016/j.jalz.2011.07.001
62. Cardenas VA, Tosun D, Chao LL, Fletcher PT, Joshi S, Weiner MW, et al. Voxel-wise co-analysis of macro- and microstructural brain alteration in mild cognitive impairment and Alzheimer's disease using anatomical and diffusion MRI. J Neuroimaging. (2014) 24:435–43. doi: 10.1111/jon.12002
63. Bozzali M, Giulietti G, Basile B, Serra L, Spanò B, Perri R, et al. Damage to the cingulum contributes to Alzheimer's disease pathophysiology by deafferentation mechanism. Hum Brain Mapp. (2012) 33:1295–308. doi: 10.1002/hbm.21287
64. Pievani M, Agosta F, Pagani E, Canu E, Sala S, Absinta M, et al. Assessment of white matter tract damage in mild cognitive impairment and Alzheimer's disease. Hum Brain Mapp. (2010) 31:1862–75. doi: 10.1002/hbm.20978
65. Newcombe VF, Williams GB, Nortje J, Bradley PG, Chatfield DA, Outtrim JG, et al. Concordant biology underlies discordant imaging findings: diffusivity behaves differently in grey and white matter post acute neurotrauma. Acta Neurochir Suppl. (2008) 102:247–51. doi: 10.1007/978-3-211-85578-2_47
66. Newcombe VF, Williams GB, Scoffings D, Cross J, Carpenter TA, Pickard JD, et al. Aetiological differences in neuroanatomy of the vegetative state: insights from diffusion tensor imaging and functional implications. J Neurol Neurosurg Psychiatry. (2010) 81:552–61. doi: 10.1136/jnnp.2009.196246
67. Song SK, Sun SW, Ju WK, Lin SJ, Cross AH, Neufeld AH. Diffusion tensor imaging detects and differentiates axon and myelin degeneration in mouse optic nerve after retinal ischemia. Neuroimage. (2003) 20:1714–22. doi: 10.1016/j.neuroimage.2003.07.005
68. Sun SW, Liang HF, Cross AH, Song SK. Evolving Wallerian degeneration after transient retinal ischemia in mice characterized by diffusion tensor imaging. Neuroimage. (2008) 40:1–10. doi: 10.1016/j.neuroimage.2007.11.049
69. Thomalia G, Glauche V, Weiller C, Rother J. Time course of wallerian degeneration after ischaemic stroke revealed by diffusion tensor imaging. J Neurol Neurosurg Psychiatry. (2005) 76:266–8. doi: 10.1136/jnnp.2004.046375
70. Nitkunan A, Charlton RA, McIntyre DJ, Barrick TR, Howe FA, Markus HS. Diffusion tensor imaging and MR spectroscopy in hypertension and presumed cerebral small vessel disease. Magn Reson Med. (2008) 59:528–34. doi: 10.1002/mrm.21461
71. Song SK, Sun SW, Ramsbottom MJ, Chang C, Russell J, Cross AH. Dysmyelination revealed through MRI as increased radial (but unchanged axial) diffusion of water. Neuroimage. (2002) 17:1429–36. doi: 10.1006/nimg.2002.1267
72. Song SK, Yoshino J, Le TQ, Lin SJ, Sun SW, Cross AH, Armstrong RC. Demyelination increases radial diffusivity in corpus callosum of mouse brain. Neuroimage. (2005) 26:132–40. doi: 10.1016/j.neuroimage.2005.01.028
73. Sintini I, Schwarz CG, Martin PR, Graff-Radford J, Machulda MM, Senjem ML, et al. Regional multimodal relationships between tau, hypometabolism, atrophy, and fractional anistropy in atypical Alzheimer's disease. Hum Brain Mapp. (2019) 40:1618–31. doi: 10.1002/hbm.24473
74. Mayo CD, Garcia-Barrera MA, Mazerolle EL, Ritchie LJ, Fisk JD, Gawryluk JR. Relationship between DTI metrics and cognitive function in Alzheimer's disease. Front Aging Neurosci. (2019) 10:436. doi: 10.3389/fnagi.2018.00436
75. Bigham B, Zamanpour SA, Zemorshidi F, Boroumand F, Zare H. Alzheimer's Disease Neuroimaging Inititative. Identification of superficial white matter abnormalities in Alzheimer's disease and mild cognitive impairment using diffusion tensor imaging. J Alzheimers Dis Rep. (2020) 4:49–59. doi: 10.3233/ADR-190149
76. Acosta-Cabrenero J, Nestor PJ. Diffusion tensor imaging in Alzheimer's disease: insights into the limbic-diencephalic network and methodological considerations. Front Aging Neurosci. (2014) 6:266. doi: 10.3389/fnagi.2014.00266
77. Veenith TV, Carter EL, Grossac J, Newcombe VFJ, Outtrim JG, Nallapareddy S, et al. Normobaric hyperoxia does not improve derangements in diffusion tensor imaging found distant from visible contusions following acute traumatic brain injury. Sci Rep. (2017) 7:12419. doi: 10.1038/s41598-017-12590-2
78. Caverzasi E, Mandelli ML, DeArmond SJ, Hess CP, Vitali P, Papinutto N, et al. White matter involvement in sporadic Creutzfeldt-Jakob disease. Brain. (2014) 137:3339–54. doi: 10.1093/brain/awu298
79. Li X, Ba M, Ng KP, Mathotaarachchi S, Pascoal TA, Rosa-Neto P, et al. Characterizing biomarker features of cognitively normal individuals with ventriculomegaly. Alzheimers Dement. (2018) 10:12–21. doi: 10.1016/j.dadm.2017.08.001
80. Ishikawa M, Oowaki H, Takezawa M, Takenaka T, Yamada S, Yamamoto K, et al. Disproportionately enlarged subarachnoid space hydrocephalus in idiopathic normal-pressure hydrocephalus and its implication in pathogenesis. Acta Neurochir Suppl. (2016) 122:287–90. doi: 10.1007/978-3-319-22533-3_57
81. Douaud G, Jbabdi S, Behrens TE, Menke RA, Gass A, Monsch AU, et al. DTI measures in crossing-fibre areas: increased diffusion anisotropy reveals early white matter alteration in MCI and mild Alzheimer's disease. Neuroimage. (2011) 55:880–90. doi: 10.1016/j.neuroimage.2010.12.008
Keywords: normal pressure hydrocephalus (NPH), diffusion tensor imaging (DTI), white matter, traumatic brain injury (TBI), Alzheimer's disease, injury properties
Citation: Keong NC, Lock C, Soon S, Hernowo AT, Czosnyka Z, Czosnyka M, Pickard JD and Narayanan V (2022) Diffusion Tensor Imaging Profiles Can Distinguish Diffusivity and Neural Properties of White Matter Injury in Hydrocephalus vs. Non-hydrocephalus Using a Strategy of a Periodic Table of DTI Elements. Front. Neurol. 13:868026. doi: 10.3389/fneur.2022.868026
Received: 02 February 2022; Accepted: 01 April 2022;
Published: 06 July 2022.
Edited by:
Madoka Nakajima, Juntendo University, JapanReviewed by:
Koji Kamagata, Juntendo University, JapanNiharika Gajawelli, Children's Hospital of Los Angeles, United States
Copyright © 2022 Keong, Lock, Soon, Hernowo, Czosnyka, Czosnyka, Pickard and Narayanan. This is an open-access article distributed under the terms of the Creative Commons Attribution License (CC BY). The use, distribution or reproduction in other forums is permitted, provided the original author(s) and the copyright owner(s) are credited and that the original publication in this journal is cited, in accordance with accepted academic practice. No use, distribution or reproduction is permitted which does not comply with these terms.
*Correspondence: Nicole C. Keong, nchkeong@cantab.net