- 1Department of Neurosurgery, Affiliated Hospital of Guilin Medical University, Guilin, China
- 2Department of Neurosurgery, Huizhou Municipal Central Hospital, Huizhou, China
- 3Department of Neurosurgery, Nanxishan Hospital of Guangxi Zhuang Autonomous Region, Guilin, China
Background and Purpose: The systemic immune-inflammation index, a new index based on platelets, neutrophils and lymphocytes, has been shown to be associated with outcomes of patients with venous sinus thrombosis and cancer. However, its application in acute ischemic stroke has rarely been reported. Therefore, we examined the relationship between systemic immune-inflammation index levels at hospital admission and the outcomes of patients 3 months after onset, and plotted a nomogram to predict the probability of adverse outcomes in patients with acute ischemic stroke.
Methods: We retrospectively analyzed a total of 208 patients with acute ischemic stroke who were admitted between January 2020 and December 2020, and recorded the modified Rankin score 3 months later. A modified Rankin score ≥ 3 was defined as an adverse outcome. Age, sex, NIHSS score, SII, hypertension and coronary heart disease were included in the binary logistic regression, and the nomogram was plotted with a regression equation.
Results: Receiver operating characteristic (ROC) curve analysis indicated that the best cutoff value of the systemic immune-inflammation index was 802.8, with a sensitivity of 70.9% and specificity of 58.2% (area under the curve: 0.657, 95% confidence interval: 0.572–0.742). The nomogram had a C index of 0.802. The average error of the calibration curves of the training set and the validation set was 0.021 and 0.034, respectively.
Conclusion: The systemic immune-inflammation index is associated with short-term adverse outcomes in patients with acute ischemic stroke, and the nomograms can predict the risk of adverse outcomes in patients with acute ischemic stroke.
Introduction
Stroke is the second leading cause of death and disability worldwide (1). Eighty-seven percent of cases are ischemic stroke (2). In China, ~2.4 million new stroke cases occur each year, and the stroke mortality rate has reached 22.3% (3, 4). According to the data from China's Hospital Quality Monitoring System, in 2018, a total of 3,010,204 stoke patients were admitted to 1,853 tertiary hospitals, of whom 2,466,785 had ischemic stroke, accounting for up to 81.9% of stroke cases (4). Therefore, early risk evaluation and severity estimation in stroke patients and identification of risk factors that can be addressed with intervention would improve adverse outcomes in stroke patients (5).
Increasing studies are focusing on the inflammatory response of the brain after stroke, which is a secondary injury mechanism (6) that can indirectly aggravate brain injury (7). In an experimental stroke model, brain injury has been found to be alleviated by inhibition of inflammatory cells (6). Therefore, inflammatory factor-related immunotherapy has been suggested as a potential option to improve the outcomes of stroke patients (8).
Recently, several new leukocyte-based inflammatory indicators, such as the platelet to lymphocyte ratio (PLR), lymphocyte to monocyte ratio (LMR) and systemic immune inflammatory index (SII) have been used as prognostic predictors of certain diseases. A meta-analysis by QIANG et al. has shown that PLR is a predictor of adverse outcomes in patients with non-small cell lung cancer (9). Ha et al. have incorporated LMR into a predictive model for patients with hepatocellular carcinoma and shown that LMR can predict the survival of patients with advanced hepatocellular carcinoma (10). Ren et al. have shown that lower LMR at admission is associated with poor prognosis 3 months later in patients with AIS (3). SII, calculated as platelets × neutrophils/lymphocytes, includes lymphocytes and neutrophils—two immune inflammatory cells—and platelets, and has been reported to accurately predict the outcomes of patients with venous sinus thrombosis (11). Previous studies have suggested that SII is associated with the severity of stroke at admission (12), but this index is not widely applied to predict functional outcomes in AIS. In addition, clinicians cannot easily assess whether patients with AIS will have adverse outcomes at admission. Therefore, in this study, we aimed to discuss the relationship between SII levels at admission and the outcomes of patients with AIS 3 months after onset, and to plot nomograms to predict the probability of adverse outcomes in patients with AIS.
Methods
Patient Selection
In this study, patients with AIS admitted to the Affiliated Hospital of Guilin Medical University between January 2020 and December 2020 were retrospectively analyzed. Patients meeting the following criteria were enrolled: (1) admission to the hospital within 24 h of onset, and diagnosis by CT (completed within 24 h) or MRI (completed within 72 h), (2) symptoms and signs of neurological dysfunction, and (3) age over 18 years. Patients who met the following criteria were excluded: (1) history of cerebral infarction and MRS ≥ 2 scores, (2) history of infectious disease in the prior 2 weeks, (3) complications of hematological diseases, (4) use of immune-suppressants or steroid drugs, (5) history of malignant tumor or autoimmune disease, (6) severe liver and kidney dysfunction, and (7) death during hospitalization or the presence of respiratory infections.
This study was approved by the Ethics Committee of the Affiliated Hospital of Guilin Medical University (2021YJSLL-02) and was conducted in accordance with the Declaration of Helsinki. Because this was a retrospective study, the Ethics Committee approved the waiver of signed informed consent, in accordance with national laws and institutional requirements. In this study, the personal identity information of the patients enrolled was anonymized and replaced with a coding system.
Data Collection
In this study, baseline clinical data were collected for all patients, including age, sex and related risk factors (e.g., coronary heart disease, hypertension, diabetes, smoking and alcohol consumption, atrial fibrillation and hyperlipidemia). Hypertension was defined by a systolic blood pressure ≥140 mmHg and diastolic blood pressure ≥90 mmHg, or a history of hypertension or use of antihypertensive drugs. Diabetes was defined by a fasting blood glucose ≥7.0 mmol/L, random blood glucose ≥11.1 mmol/L, or a previous history of diabetes or use of hypoglycemic drugs. Coronary heart disease was defined by a previous history of coronary heart disease. Atrial fibrillation was defined by a previous history of atrial fibrillation or diagnosis with atrial fibrillation by electrocardiography. A white blood cell count of (4–10) × 109/L was defined as normal; other counts were considered abnormal. A neutrophil count of 2–7 × 109/L was defined as normal; other counts were considered abnormal (13). An absolute lymphocyte count in the range of 0.8–3.5 × 109/L was defined as normal; other counts were considered abnormal. The normal range of platelets was (100–300) × 109/L, and the normal range of the mean corpuscular volume (MCV) was 80–100 Fl. The normal range of mean corpuscular hemoglobin (MCH) was 26–34. LDL < 3.4 mmol/L was considered normal. HDL >1.0 mmol/L was considered normal; other values were considered abnormal. Stroke severity on admission was evaluated according to the National Institutes of Health Stroke Scale (NIHSS). According to previous studies, stroke with an NIHSS score <6 at admission was defined as mild, and stroke with an NIHSS score ≥6 was defined as moderate to severe (14, 15).
Blood samples were collected from all patients for analysis within 24 h. The white blood cell count, neutrophil count, lymphocyte count, monocyte count, platelet count, MCV, MCH, total cholesterol, triglycerides, blood creatinine and blood uric acid were measured. SII, LMR and PLR were calculated on the basis of routine blood examination results at admission. SII = platelets × neutrophils/lymphocytes, LMR = lymphocytes/monocytes and PLR = platelets/lymphocytes.
Assessment of Stroke Severity and 3-Month Outcome
All patients were scored with the NIHSS scale at admission to assess stroke severity. All enrolled patients were followed up via telephone by the same investigator. The modified Rankin scale (mRS) was used to assess the neurological outcomes of the stroke patients after 90 days. mRS score <3 was defined as a good outcome, and mRS ≥3 was defined as an adverse outcome (16).
Statistical Analysis
SPSS 23.0 (SPSS Inc., Chicago, IL) was used for data analysis, R studio (version 4.1.0) was used for nomogram plotting, and GraphPad Prism 8.3.0 was used to plot the forest map and ROC curves of the three indexes. First, the Kolmogorov-Smirnov test was used to test the normality of the data. Measurement data conforming to a normal distribution are expressed as the mean ± standard deviation, and compared between groups with single factor ANOVA. Measurement data that did not conform to a normal distribution are expressed as median and quartile, and compared between groups with the Kruskal–Wallis test. The measurement data are expressed as percentage n (%), the chi-square test was used for comparison between groups, and the Fisher test was used if the expected frequency of more than 20% of the cells was <5. The Bonferroni method was used for multiple comparisons for data found to be statistically significant with the chi-square test. The SII was divided into three groups: group 1 (SII ≤ 575.94), group 2 (SII ≤ 1096.28), and group 3 (SII > 1096.28). ROC curve analysis was used to evaluate the predictive value of SII, LMR and PLR for adverse outcomes in patients with AIS. The cut-off value of SII was determined on the basis of the ROC curve, according to which SII was divided into low SII and high SII groups. Independent predictors of adverse outcomes in stroke were evaluated with a binary logistic regression model, and odds ratios and 95% confidence intervals were calculated. The single-factor analysis results revealed P < 0.05 factors and factors related to functional endings, which were included in multi-factor analysis. All statistical results were tested with a two-sided test, and p < 0.05 indicated statistically significant differences.
Results
Baseline Characteristics
The clinical data of 299 patients with AIS were collected, 208 of whom were enrolled in the study. Figure 1 shows the exclusion process. Table 1 shows the clinical baseline data of the patients. The mean systolic blood pressure was 150.4 ± 21.2 mmHg, and the mean diastolic blood pressure was 86.9 ± 13.2 mmHg at admission. The average patient age was 63.3 ± 11.3 years, and 68.8% of the patients were male. On the basis of the SII value, the 208 patients with AIS were divided into three groups: group 1 (69 patients with SII ≤ 575.94), group 2 (70 patients with SII ≤ 1096.28), and group 3 (69 patients with SII >1096.28). No significant differences were observed in the clinical baseline data among the three groups. The leukocyte, neutrophil, SII and PLR values of the patients in group 3 were significantly higher than those in the first two groups, whereas the lymphocyte and LMR values of the patients in group 3 were significantly lower than those in the first two groups. There were no significant differences in MCV, MCH, LDL and HDL among the three SII subgroups.
Functional Outcomes
At the follow-up after 90 days, 55 patients had adverse outcomes: 11 cases in group 1 (15.9), 17 cases in group 2 (24.3%) and 27 cases in group 3 (39.1%). Significant differences were observed in adverse outcomes among the three groups (p = 0.007) (Table 2). Group 3 had a higher incidence of adverse outcomes than the first two groups, and significant differences were found between group 1 and group 3 (p = 0.002) (Table 3). No significant differences were observed between group 1 and group 2, as well as group 2 and group 3 (p = 0.22, 0.06). ROC curve analysis showed that the best cut-off value of SII was 802.8, with a sensitivity of 70.9% and specificity of 58.2% (area under the curve: 0.657, 95% confidence interval: 0.572–0.742) (Figure 2). The best cut-off value of LMR was 2.94, with a sensitivity of 65.5% and specificity of 54.5% (area under curve: 0.625 and 95% confidence interval: 0.289–0.461), and the best cut-off value of PLR was 185.66, with a sensitivity of 54.5% and specificity of 77.8% (area under curve: 0.656 and 95% confidence interval: 0.571–0.74). To further explore the relationship between SII and the clinical status of patients with AIS, we divided 208 patients with AIS into two groups according to their ROC cut-off values (SII > 802.80 × 109/L, n = 103 and SII ≤ 802.80 × 109/L, n = 105). The median SII values of the two groups were 1294.29 and 504.52, respectively.
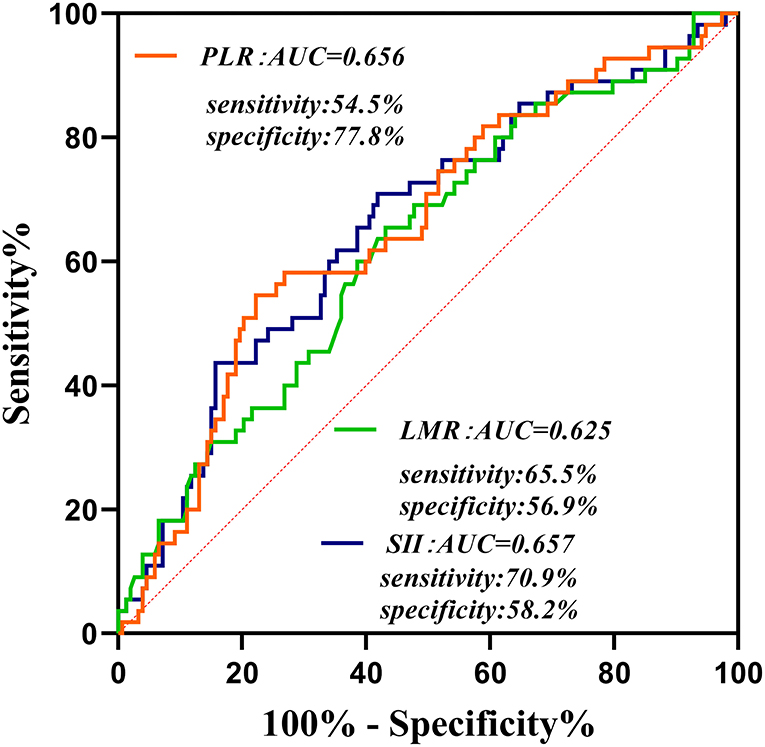
Figure 2. Receiver operating characteristic curve for composite inflammatory ratios (systemic immune inflammatory index, platelet to lymphocyte ratio, and lymphocyte to monocyte ratio) to predict the risk of adverse outcomes in patients with AIS. AUC, area under the curve; SII, systemic immune inflammatory index; PLR, platelet to lymphocyte ratio; LMR, lymphocyte to monocyte ratio.
Single-factor analysis showed that sex, age above 65 years, high NIHSS scores and SII were associated with outcomes of patients (Table 4). A multi-factor analysis including age, grade, NIHSS score, diabetes, smoking, hypertension and SII showed that high SII was an independent risk factor for 90-day adverse outcomes in stroke patients (odds ratio = 2.915, 95% confidence interval 1.420–5.985 and p = 0.004) (Table 5). Figure 3 shows the results of the multi-factor logistic regression analysis.
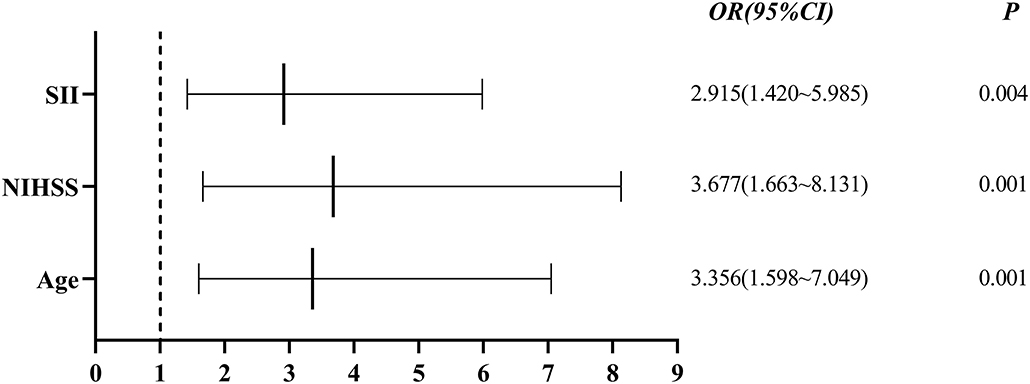
Figure 3. Forest plot for clinical factors. SII, systemic immune inflammatory index; NIHSS: National Institutes of Health Stroke Scale.
Predictive Nomogram Development
The data were randomized into training (n = 156) and validation (n = 52) sets at a ratio of 3: 1 in R (version 4.1.0). Age, sex, NIHSS score, SII, hypertension and coronary heart disease were included in the binary logistic regression, and the nomogram (Figure 4) was plotted with a regression equation in which age and NIHSS score were continuous variables, and SII, sex, hypertension and coronary heart disease were binomial variables. The numbers 0 and 1 at SII in the nomogram indicated low SII and high SII, respectively; 0 and 1 in the sex groups indicated male and female, respectively; and 0 and 1 for hypertension and coronary diseases indicated patients without and with these two diseases, respectively. The nomogram for the training set was plotted, and the C-index was calculated to plot the calibration curve and the ROC curve. Different colors are used for each scoring item in the nomogram; each color indicates a corresponding score in the right score table, and the sum of all scores indicates the corresponding risk at the bottom of the nomogram. The resulting risk was the probability of an adverse outcome in patients with AIS after 90 days.
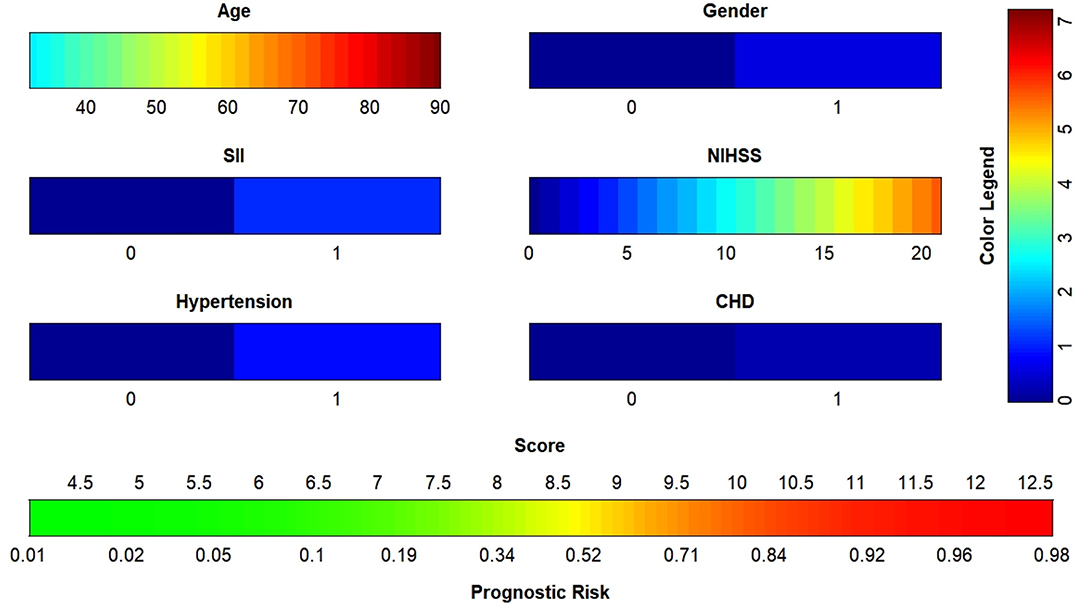
Figure 4. Nomogram to predict the risk of adverse outcome for AIS patients in 3 months. SII, systemic immune inflammatory index; NIHSS, National Institutes of Health Stroke Scale; CHD, coronary heart disease; AIS, acute ischemic stroke.
Validation of the Nomogram
The ROC curve of the training set (Figure 5) had an AUC = 0.826, and that of the validation set (Figure 5) had an AUC = 0.836. The nomogram had good predictive performance with high discrimination. The nomogram was calibrated with the calibration curve. The calibration curve of the training set (Figure 6A) revealed that the mean error between the risk of adverse outcomes predicted by the predictive model and the actual risk of adverse outcomes was 0.021. Similarly, the calibration curve of the validation set (Figure 6B) showed a mean error of 0.034, indicating consistency between the predicted and observed results. With a C index of 0.802, the nomogram had good accuracy.
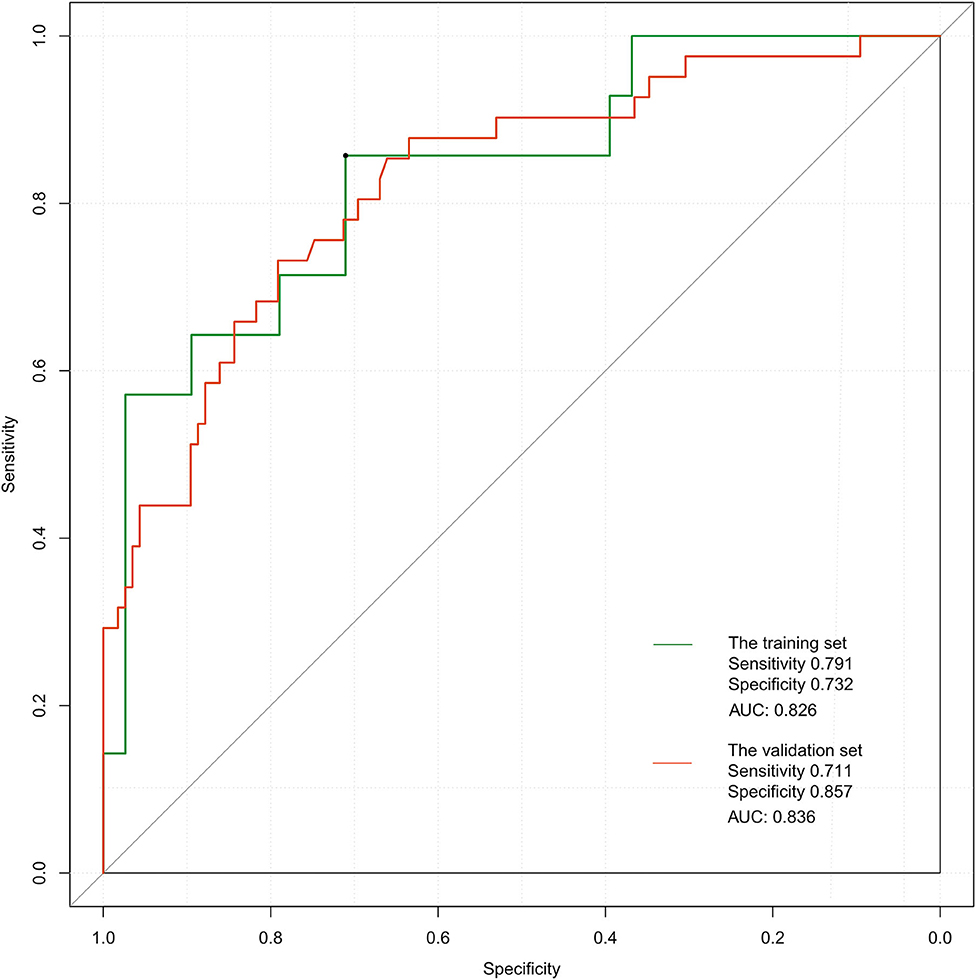
Figure 5. Receiver operating characteristic curves of the proposed nomogram in the training and validation set.
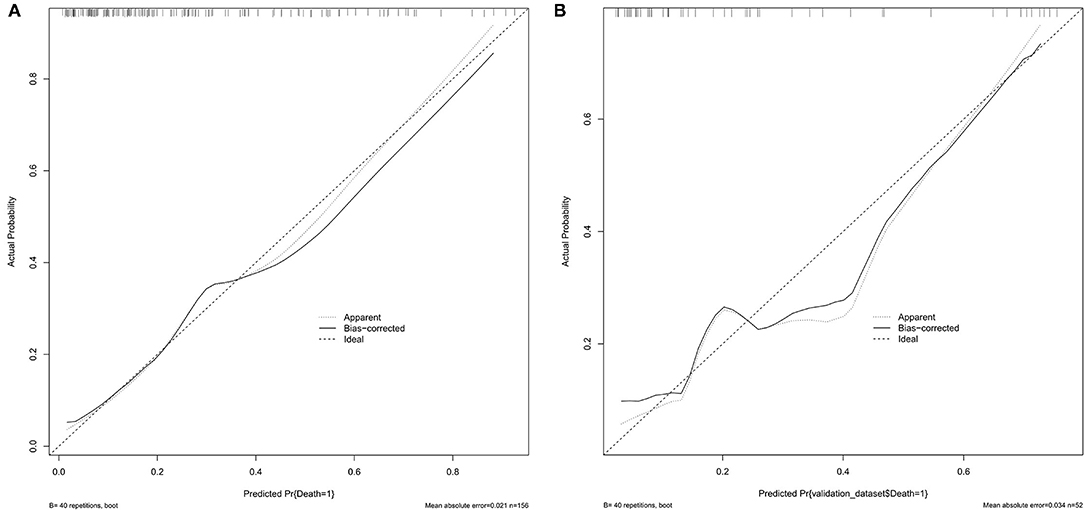
Figure 6. (A) Calibration plots of the proposed nomogram in the training set. (B) Calibration plots of the proposed nomogram in the validation set.
Discussion
In this study, the relationships among SII, LMR and PLR at admission and short and moderate term adverse outcomes in patients with AIS were explored, and their predictive values were compared. SII had a good predictive value for outcomes of patients with AIS, and high SII was closely associated with adverse outcomes in stroke patients. Therefore, SII is considered a new index for outcomes of patients with AIS. Moreover, the nomogram showed good predictive performance.
Previous studies have shown that stroke causes nerve cell death and the release of factors such as damage-related molecular patterns, thereby leading to a local inflammatory response in damaged brain regions (17–19). Mast cells are activated in early stages after the onset of AIS, and subsequently damage the blood-brain barrier and cause brain edema by releasing gelatinase (20, 21). After the destruction of the blood-brain barrier, peripheral leukocytes infiltrate into damaged brain regions and further aggravate the destruction of the blood-brain barrier by periodically releasing pro-inflammatory cytokines, reactive oxygen species and matrix metalloproteinases (22). Neutrophils infiltrate into the ischemic site within several hours after AIS and peak at 24–48 h (23). Increased neutrophil concentrations result in elevated expression of matrix metalloproteinase-9 (24), which further damages the blood brain barrier. In addition, neutrophils further aggravate brain injury by releasing inflammatory mediators (25). After AIS, the infiltration of neutrophils has been associated with injury severity (26). After AIS, blood stagnation flow produces shear stress on endothelial cells and platelets, thus resulting in the deployment of P-selectin of the adhesion molecules to the cell surface (23). Platelets interact directly with circulating leukocytes by changing the surface expression of P-selectin or CD40, thereby forming platelet-leukocyte aggregates and activating the innate immune response to ischemia (27). P-selectin of platelets also acts as a bridge molecule by binding to leukocytes, thus promoting leukocyte aggregation, leading to intravascular occlusion and further contributing to ischemic injury (28). Lymphocytes, a subtype of leukocytes, are also involved in the inflammatory response after stroke. However, the mechanism of action of lymphocytes in AIS in the literature is controversial. Some animal stroke experiments have suggested that increased lymphocytes up-regulate the levels of IL-10 and inhibit the inflammatory cytokines such as IL-6 and TNF-α, thus playing a neuroprotective role (29, 30). Previous studies have shown that lymphocytes aggravate the inflammatory response after stroke (6, 31). The specific effects of lymphocytes on AIS depend on the subtypes of lymphocytes. Furthermore, T cells and γδT CD4+ and CD8+ cells play adverse roles in AIS by producing proinflammatory cytokines such as interferon-gamma and IL-17 (32). In addition, natural killer cells aggregate brain injury by causing neuronal death (33), whereas regulatory T cells (Tregs) play a neuroprotective role by secreting IL-10 (34). Thus, the inflammatory response of AIS is highly complex.
Many studies have shown that inflammatory mechanisms play critical roles in the occurrence and development of ischemic stroke (22, 23, 35, 36). The inflammatory response aggravates ischemic brain damage and neurological dysfunction, whereas chemokines and cytokines released by ischemic tissues promote the infiltration of peripheral circulating leukocytes into ischemic sites (37, 38). The infiltration of leukocytes and the release of various inflammatory mediators cause neuronal death or apoptosis, thus resulting in adverse outcomes in patients with AIS (23). LMR and PLR at admission have been reported to have a good predictive value for the outcomes of patients with AIS. Moreover, low LMR and high PLR are closely associated with the severity of stroke (3, 39). Huang et al. (40) have found that PLR is an independent predictor of depression after a stroke. Yang et al. have found that SII is associated with many adverse events in patients with coronary heart disease (41). Given that stroke is closely associated with inflammation, SII, which combines platelet count, neutrophils and lymphocytes, is a marker of systemic immune inflammation and may be a better predictive index of outcome in AIS.
In summary, exploring the mechanism of inflammatory response after stroke is valuable for applications in immunoregulatory therapy. The SII, which combines neutrophils, lymphocytes and platelets to reflect inflammation and thrombosis, is more reliable and representative than the LMR and PLR. Furthermore, SII has the advantage of easy availability and rapidity, because routine blood analysis is essential for patients admitted to the hospital, and patients are not required to pay extra costs, thus improving compliance.
In our study, SII was an independent predictor of 3-month adverse outcomes in stroke patients. The nomogram showed good predictive performance. SII values can be calculated within several hours after admission, and a nomogram score can be derived and used by clinicians to predict the probability of short-term adverse outcomes in patients with AIS at admission. This tool should be helpful for clinicians by enabling early evaluation of patient outcomes, thereby aiding in the choice of better treatment plans.
This study has some limitations. First, this was a single center study with a small sample size (n = 208), which may have resulted in bias and inaccuracy in selection. Second, only SII at admission was calculated, and dynamic monitoring was not performed, thus potentially influencing the correlation between SII and outcomes of patients with AIS. Third, we were unable to analyze other markers of inflammation, such as C-reactive protein, IL-6, fibrinogen and ESR, because of the study's retrospective nature. Fourth, although this study divided samples into two groups—a training set and verification set—through a random grouping method, the verification set was not truly independent, thus potentially increasing the predictive value of the model to some extent. Finally, the study focused on patients admitted within 24 h after the onset of AIS. Therefore, the prognostic model in this study might not be applicable to other types of stroke.
Conclusion
In summary, our results suggest that SII at admission is a simple new index for predicting the short-tern outcomes of patients with AIS. The nomogram further improves the accuracy of adverse outcome prediction, and thus, may help clinicians determine better treatment plans. Given the limitations of the study, prospective and multicenter studies are needed to further validate the findings.
Data Availability Statement
The raw data supporting the conclusions of this article will be made available by the authors, without undue reservation.
Ethics Statement
This study was approved by the Ethics Committee of the Affiliated Hospital of Guilin Medical University (2021YJSLL-02) and was conducted in accordance with the Declaration of Helsinki. The Ethics Committee waived the requirement of written informed consent for participation.
Author Contributions
Y-XZ, W-CL, and W-BW conceived the review. CT, TX, and Y-XZ conducted data collection. W-CL, S-HX, M-JL, X-WX, J-LL, and CT analyzed and interpreted the data in this study. All authors read and approved the final manuscript.
Funding
This work was supported by the National Natural Science Foundation of China (No. 81860449) and the Natural Science Foundation of Guangxi (2018GXNSFAA050054).
Conflict of Interest
The authors declare that the research was conducted in the absence of any commercial or financial relationships that could be construed as a potential conflict of interest.
Publisher's Note
All claims expressed in this article are solely those of the authors and do not necessarily represent those of their affiliated organizations, or those of the publisher, the editors and the reviewers. Any product that may be evaluated in this article, or claim that may be made by its manufacturer, is not guaranteed or endorsed by the publisher.
Acknowledgments
We thank International Science Editing (http://www.internationalscienceediting.com) for editing this manuscript.
References
1. Wu S, Wu B, Liu M, Chen Z, Wang W, Anderson C, et al. Stroke in China: advances and challenges in epidemiology, prevention, and management. Lancet Neurol. (2019) 18:394–405. doi: 10.1016/S1474-4422(18)30500-3
2. Zhu T, Wang L, Feng Y, Sun G, Sun X. Classical active ingredients and extracts of Chinese herbal medicines: pharmacokinetics, pharmacodynamics, and molecular mechanisms for ischemic stroke. Oxid Med Cell Longev. (2021) 2021:8868941. doi: 10.1155/2021/8868941
3. Ren H, Liu X, Wang L, Gao Y. Lymphocyte-to-monocyte ratio: a novel predictor of the prognosis of acute ischemic stroke. J Stroke Cerebrovasc Dis. (2017) 26:2595–602. doi: 10.1016/j.jstrokecerebrovasdis.2017.06.019
4. Wang Y, Li Z, Gu H, Zhai Y, Jiang Y, Zhao X, et al. China stroke statistics 2019: a report from the National Center for Healthcare Quality Management in Neurological Diseases, China National Clinical Research Center for Neurological Diseases, the Chinese Stroke Association, National Center for Chronic and Non-communicable Disease Control and Prevention, Chinese Center for Disease Control and Prevention and Institute for Global Neuroscience and Stroke Collaborations. Stroke Vasc Neurol. (2020) 5:211–39. doi: 10.1136/svn-2020-000457
5. Slot K, Berge E, Dorman P, Lewis S, Dennis M, Sandercock P. Impact of functional status at six months on long term survival in patients with ischaemic stroke: prospective cohort studies. BMJ. (2008) 336:376–9. doi: 10.1136/bmj.39456.688333.BE
6. Kim J, Kawabori M, Yenari M. Innate inflammatory responses in stroke: mechanisms and potential therapeutic targets. Curr Med Chem. (2014) 21:2076–97. doi: 10.2174/0929867321666131228205146
7. Chamorro Á, Meisel A, Planas AM, Urra X, van de Beek D, Veltkamp R. The immunology of acute stroke. Nat Rev Neurol. (2012) 8:401–10. doi: 10.1038/nrneurol.2012.98
8. Pagram H, Bivard A, Lincz L, Levi C. Peripheral immune cell counts and advanced imaging as biomarkers of stroke outcome. Cerebrovasc Dis Extra. (2016) 6:120–8. doi: 10.1159/000450620
9. Qiang G, Liang C, Xiao F, Yu Q, Wen H, Song Z, et al. Prognostic significance of platelet-to-lymphocyte ratio in non-small-cell lung cancer: a meta-analysis. Oncotargets Ther. (2016) 9:869–76. doi: 10.2147/OTT.S96804
10. Ha Y, Mohamed Ali M, Petersen M, Harmsen W, Therneau T, Lee H, et al. Lymphocyte to monocyte ratio-based nomogram for predicting outcomes of hepatocellular carcinoma treated with sorafenib. Hepatol Int. (2020) 14:776–87. doi: 10.1007/s12072-020-10076-4
11. Li S, Liu K, Gao Y, Zhao L, Zhang R, Fang H, et al. Prognostic value of systemic immune-inflammation index in acute/subacute patients with cerebral venous sinus thrombosis. Stroke Vasc Neurol. (2020) 5:368–73. doi: 10.1136/svn-2020-000362
12. Hou D, Wang C, Luo Y, Ye X, Han X, Feng Y, et al. Systemic immune-inflammation index (SII) but not platelet-albumin-bilirubin (PALBI) grade is associated with severity of acute ischemic stroke (AIS). Int J Neurosci. (2020) 131:1203–8. doi: 10.1080/00207454.2020.1784166
13. Zhu B, Pan Y, Jing J, Meng X, Zhao X, Liu L, et al. Neutrophil counts, neutrophil ratio, and new stroke in minor ischemic stroke or TIA. Neurology. (2018) 90:e1870–8. doi: 10.1212/WNL.0000000000005554
14. Xue J, Huang W, Chen X, Li Q, Cai Z, Yu T, et al. Neutrophil-to-lymphocyte ratio is a prognostic marker in acute ischemic stroke. J Stroke Cerebrovasc Dis. (2017) 26:650–7. doi: 10.1016/j.jstrokecerebrovasdis.2016.11.010
15. Ducroquet A, Leys D, Al Saabi A, Richard F, Cordonnier C, Girot M, et al. Influence of chronic ethanol consumption on the neurological severity in patients with acute cerebral ischemia. Stroke. (2013) 44:2324–6. doi: 10.1161/STROKEAHA.113.001355
16. Qun S, Tang Y, Sun J, Liu Z, Wu J, Zhang J, et al. Neutrophil-to-lymphocyte ratio predicts 3-month outcome of acute ischemic stroke. Neurotoxicity Res. (2017) 31:444–52. doi: 10.1007/s12640-017-9707-z
17. Liesz A, Dalpke A, Mracsko E, Antoine D, Roth S, Zhou W, et al. DAMP signaling is a key pathway inducing immune modulation after brain injury. J Neurosci. (2015) 35:583–98. doi: 10.1523/JNEUROSCI.2439-14.2015
18. Li L, Chen C, Chang Y, Chen Y, Lee I, How C. Prognostic role of neutrophil-to-lymphocyte ratio, platelet-to-lymphocyte ratio, and systemic immune inflammation index in acute ischemic stroke: a STROBE-compliant retrospective study. Medicine. (2021) 100:e26354. doi: 10.1097/MD.0000000000026354
19. Shichita T, Ito M, Morita R, Komai K, Noguchi Y, Ooboshi H, et al. MAFB prevents excess inflammation after ischemic stroke by accelerating clearance of damage signals through MSR1. Nat Med. (2017) 23:723–32. doi: 10.1038/nm.4312
20. Mattila O, Strbian D, Saksi J, Pikkarainen T, Rantanen V, Tatlisumak T, et al. Cerebral mast cells mediate blood-brain barrier disruption in acute experimental ischemic stroke through perivascular gelatinase activation. Stroke. (2011) 42:3600–5. doi: 10.1161/STROKEAHA.111.632224
21. Strbian D, Kovanen P, Karjalainen-Lindsberg M, Tatlisumak T, Lindsberg P. An emerging role of mast cells in cerebral ischemia and hemorrhage. Ann Med. (2009) 41:438–50. doi: 10.1080/07853890902887303
22. Shi K, Tian D, Li Z, Ducruet A, Lawton M, Shi F. Global brain inflammation in stroke. Lancet Neurol. (2019) 18:1058–66. doi: 10.1016/S1474-4422(19)30078-X
23. Anrather J, Iadecola C. Inflammation and stroke: an overview. Neurotherapeutics. (2016) 13:661–70. doi: 10.1007/s13311-016-0483-x
24. Justicia C, Panés J, Solé S, Cervera A, Deulofeu R, Chamorro A, et al. Neutrophil infiltration increases matrix metalloproteinase-9 in the ischemic brain after occlusion/reperfusion of the middle cerebral artery in rats. J Cerebral Blood Flow Metab. (2003) 23:1430–40. doi: 10.1097/01.WCB.0000090680.07515.C8
25. Iadecola C, Anrather J. The immunology of stroke: from mechanisms to translation. Nat Med. (2011) 17:796–808. doi: 10.1038/nm.2399
26. Akopov S, Simonian N, Grigorian G. Dynamics of polymorphonuclear leukocyte accumulation in acute cerebral infarction and their correlation with brain tissue damage. Stroke. (1996) 27:1739–43. doi: 10.1161/01.STR.27.10.1739
27. Maugeri N, Rovere-Querini P, Evangelista V, Covino C, Capobianco A, Bertilaccio M, et al. Neutrophils phagocytose activated platelets in vivo: a phosphatidylserine, P-selectin, and {beta}2 integrin-dependent cell clearance program. Blood. (2009) 113:5254–65. doi: 10.1182/blood-2008-09-180794
28. De Meyer S, Denorme F, Langhauser F, Geuss E, Fluri F, Kleinschnitz C. Thromboinflammation in stroke brain damage. Stroke. (2016) 47:1165–72. doi: 10.1161/STROKEAHA.115.011238
29. Liesz A, Suri-Payer E, Veltkamp C, Doerr H, Sommer C, Rivest S, et al. Regulatory T cells are key cerebroprotective immunomodulators in acute experimental stroke. Nat Med. (2009) 15:192–9. doi: 10.1038/nm.1927
30. Li P, Mao L, Zhou G, Leak R, Sun B, Chen J, et al. Adoptive regulatory T-cell therapy preserves systemic immune homeostasis after cerebral ischemia. Stroke. (2013) 44:3509–15. doi: 10.1161/STROKEAHA.113.002637
31. Liesz A, Hu X, Kleinschnitz C, Offner H. Functional role of regulatory lymphocytes in stroke: facts and controversies. Stroke. (2015) 46:1422–30. doi: 10.1161/STROKEAHA.114.008608
32. Yilmaz G, Arumugam T, Stokes K, Granger D. Role of T lymphocytes and interferon-gamma in ischemic stroke. Circulation. (2006) 113:2105–12. doi: 10.1161/CIRCULATIONAHA.105.593046
33. Gan Y, Liu Q, Wu W, Yin J, Bai X, Shen R, et al. Ischemic neurons recruit natural killer cells that accelerate brain infarction. Proc Natl Acad Sci USA. (2014) 111:2704–9. doi: 10.1073/pnas.1315943111
34. Gill D, Veltkamp R. Dynamics of T cell responses after stroke. Curr Opin Pharmacol. (2016) 26:26–32. doi: 10.1016/j.coph.2015.09.009
35. Vidale S, Consoli A, Arnaboldi M, Consoli D. Postischemic inflammation in acute stroke. J Clin Neurol. (2017) 13:1–9. doi: 10.3988/jcn.2017.13.1.1
36. Rust R, Grönnert L, Schwab M. Inflammation after stroke: a local rather than systemic response? Trends Neurosci. (2018) 41:877–9. doi: 10.1016/j.tins.2018.09.011
37. Kim J, Park J, Chang J, Kim S, Lee J. Inflammation after ischemic stroke: the role of leukocytes and glial cells. Exp Neurobiol. (2016) 25:241–51. doi: 10.5607/en.2016.25.5.241
38. Macrez R, Ali C, Toutirais O, Le Mauff B, Defer G, Dirnagl U, et al. Stroke and the immune system: from pathophysiology to new therapeutic strategies. Lancet Neurol. (2011) 10:471–80. doi: 10.1016/S1474-4422(11)70066-7
39. Xu J, He X, Li Q, Liu J, Zhuang M, Huang F, et al. Higher platelet-to-lymphocyte ratio is associated with worse outcomes after intravenous thrombolysis in acute ischaemic stroke. Front Neurol. (2019) 10:1192. doi: 10.3389/fneur.2019.01192
40. Huang G, Chen H, Wang Q, Hong X, Hu P, Xiao M, et al. High platelet-to-lymphocyte ratio are associated with post-stroke depression. J Affect Disord. (2019) 246:105–11. doi: 10.1016/j.jad.2018.12.012
Keywords: acute ischemic stroke (AIS), inflammation, outcome, nomogram, systemic immune-inflammation index (SII)
Citation: Zhou Y-X, Li W-C, Xia S-H, Xiang T, Tang C, Luo J-L, Lin M-J, Xia X-W and Wang W-B (2022) Predictive Value of the Systemic Immune Inflammation Index for Adverse Outcomes in Patients With Acute Ischemic Stroke. Front. Neurol. 13:836595. doi: 10.3389/fneur.2022.836595
Received: 15 December 2021; Accepted: 16 February 2022;
Published: 18 March 2022.
Edited by:
Marios K. Georgakis, LMU Munich University Hospital, GermanyReviewed by:
Christos Savopoulos, Aristotle University of Thessaloniki, GreecePeter Kelly, University College Dublin, Ireland
Copyright © 2022 Zhou, Li, Xia, Xiang, Tang, Luo, Lin, Xia and Wang. This is an open-access article distributed under the terms of the Creative Commons Attribution License (CC BY). The use, distribution or reproduction in other forums is permitted, provided the original author(s) and the copyright owner(s) are credited and that the original publication in this journal is cited, in accordance with accepted academic practice. No use, distribution or reproduction is permitted which does not comply with these terms.
*Correspondence: Wen-Bo Wang, d3diZ3hnbEAxNjMuY29t
†These authors have contributed equally to this work