- 1Department of Neurology, University of Pittsburgh Comprehensive Epilepsy Center (UPCEC), University of Pittsburgh Medical Center (UPMC), Pittsburgh, PA, United States
- 2Division of Neurology, Department of Pediatrics, Cincinnati Children's Hospital Medical Center, Cincinnati, OH, United States
According to the latest operational 2017 ILAE classification of epileptic seizures, the generalized epileptic seizure is still conceptualized as “originating at some point within and rapidly engaging, bilaterally distributed networks.” In contrast, the focal epileptic seizure is defined as “originating within networks limited to one hemisphere.” Hence, one of the main concepts of “generalized” and “focal” epilepsy comes from EEG descriptions before the era of source localization, and a presumed simultaneous bilateral onset and bi-synchrony of epileptiform discharges remains a hallmark for generalized seizures. Current literature on the pathophysiology of generalized epilepsy supports the concept of a cortical epileptogenic focus triggering rapidly generalized epileptic discharges involving intact corticothalamic and corticocortical networks, known as the cortical focus theory. Likewise, focal epilepsy with rich connectivity can give rise to generalized spike and wave discharges resulting from widespread bilateral synchronization. Therefore, making this key distinction between generalized and focal epilepsy may be challenging in some cases, and for the first time, a combined generalized and focal epilepsy is categorized in the 2017 ILAE classification. Nevertheless, treatment options, such as the choice of antiseizure medications or surgical treatment, are the reason behind the importance of accurate epilepsy classification. Over the past several decades, plentiful scientific research on the pathophysiology of generalized epilepsy has been conducted using non–invasive neuroimaging and postprocessing of the electromagnetic neural signal by measuring the spatiotemporal and interhemispheric latency of bi-synchronous or generalized epileptiform discharges as well as network analysis to identify diagnostic and prognostic biomarkers for accurate diagnosis of the two major types of epilepsy. Among all the advanced techniques, magnetoencephalography (MEG) and multiple other methods provide excellent temporal and spatial resolution, inherently suited to analyzing and visualizing the propagation of generalized EEG activities. This article aims to provide a comprehensive literature review of recent innovations in MEG methodology using source localization and network analysis techniques that contributed to the literature of idiopathic generalized epilepsy in terms of pathophysiology and clinical prognosis, thus further blurring the boundary between focal and generalized epilepsy.
Introduction
Epilepsy is one of the most common neurological disorders affecting almost 3.5 million in the USA and 65 million worldwide and is getting increased public health attention as patients with epilepsy have a noticeable reduction in quality of life and employment prospects (1). Two major classification categories are whether an epilepsy is focal or generalized. According to the latest operational 2017 ILAE classification of epileptic seizures, the generalized seizure is still conceptualized as “originating at some point within and rapidly engaging, bilaterally distributed networks.” In contrast, the focal seizure is defined as “originating within networks limited to one hemisphere” (2). The definitions for generalized and focal seizures are retained from the 1981 ILAE classification, and the presumed simultaneous bilateral onset and bisynchrony of the epileptic discharges in electroencephalography (EEG) remains a hallmark for generalized seizures (2–4).
In 1952, Tükel and Jasper et al. reported that a mesial frontal cortical lesion could give rise to diffuse interictal spike-and-wave discharges; hence the term “secondary bilateral synchronization” and blurring the boundary of focal and generalized epilepsy (5). In addition to the frontal lobe, focal epilepsy with rich connectivity, such as posterior parietal, temporal, or even occipital lobe epilepsy, can give rise to diffuse “generalized” spike and wave discharges (GSWD) resulting from widespread bilateral synchronization, especially in the pediatric population and can be misclassified as generalized epilepsy (6–10). On the contrary, current literature validates that focal EEG features can be found in generalized epilepsy (11). Consequently, generalized epilepsy can also be misclassified as focal epilepsy. Therefore, making a distinction between generalized and focal epilepsy may be challenging in selected clinical cases (7–10). However, the 2017 ILAE classification proposes the combined generalized and focal epilepsy as one of the categories of the epilepsy classification for ambiguous cases. Nevertheless, treatment options, such as the choice of antiseizure medications (ASMs), neuromodulation, or surgical treatment alternatives, are the reason behind the importance of accurate differentiation between focal and generalized epilepsy. When epilepsy becomes drug-resistant, defined as failure to control the seizures with two appropriate ASMs, surgical resection or disruption of the epileptogenic zone (EZ) may be a way to achieve seizure freedom or reduce seizure burden in focal epilepsy, but those with generalized epilepsy are often not considered as epilepsy surgery candidates (12–16). Although neuromodulatory treatments such as vagal nerve stimulators (VNS) (17), responsive neurostimulator (RNS) (18), and deep brain stimulators (DBS) (19) are treatment alternatives for those patients who are not resective surgical candidates, the study and indications of all those neurostimulators are mostly based on focal epilepsy (20). Thus, the treatment that we could offer for drug-resistant generalized epilepsy is more limited than for focal epilepsy. There have been multiple works of literature supporting the usage of the neurostimulators, especially the RNS and DBS targeting different parts of the thalamus and cortices in patients with generalized epilepsy. However, the outcome is highly dependent upon electrode placement in relation to different thalamic nuclei, stimulation parameters, subtypes of generalized epilepsy, or even individual cortical-subcortical connectivity profiles (20, 21).
To improve the treatment options in generalized epilepsy, plentiful scientific research on its pathophysiology has been conducted over the past several decades, using advanced non–invasive investigations and postprocessing of the neuromagnetic signal by reflecting the spatiotemporal and interhemispheric latency of bi-synchronous or generalized epileptiform discharges in both animals and humans (22–24). Using invasive intracranial electroencephalographic (icEEG) data, Chen et al. reported that two hemispheres could still function independently with different focal network structures and properties under a strong global epileptic network in generalized epilepsy; the focal epileptic network from the leading hemisphere might be activating the global epileptic network. By resecting the part of the region of the leading hemisphere, five pediatric patients with generalized epilepsy with tonic/atonic and atypical absence seizures resulted in seizure freedom (24). Although the diagnosis of generalized epilepsy in the case series was disputable as all the resective brain tissue showed abnormal pathology (3 with focal cortical dysplasia type 1A, one with focal cortical dysplasia type 1B with polymicrogyria, and one with tuberous sclerosis), the conclusion was based on EEG and clinical semiology of the seizures. non-etheless, the author highlighted one of the current clinical challenges in accurately categorizing the epileptic patients into either focal or generalized epilepsy (24). In addition, accumulating evidence has shown that epilepsy is an archetypical neural network disorder. With ongoing debates, current literature on the pathophysiology of generalized epilepsy supports the concept of a cortical epileptogenic focus triggering the rapidly generalized epileptic discharges involving intact corticothalamic and corticocortical networks, which is known as the cortical focus theory (22, 23, 25).
Among all advanced non–invasive techniques, magnetoencephalography (MEG) provides excellent temporal and spatial resolution, inherently suited for analyzing the propagation of generalized EEG activities and determining whole-brain functional connectivity network patterns (26–29). The current clinical application of MEG for epilepsy in the form of magnetic source imaging (MSI) mostly uses the single equivalent current dipole (ECD) model (30, 31), especially in the United States (32). However, the traditional ECD model is restricted if the underlying assumption of focality is not fulfilled, for example, when the epileptiform activity occurs simultaneously across the various regions (33–35). Alternative source localization techniques, such as beamformer and low-resolution brain electromagnetic topography (LORETA), as well as various connectivity analyses, have played a prominent role in improving the localization of deep sources (further details in section 2) (34–40). Although there is an overall improvement in the strength of localization of the neuromagnetic activity using various source localization algorithms, there are still major limitations in analyzing deep sources with MEG (41–44). The magnetic field intensity is inversely proportional to the square of the distance between the sources and the sensors (45), and thus there is decreased signal in deeper structures of the brain, either deep cortices or the thalamus. Since GSWDs typically have very high voltages, it is postulated that MEG may be able to overcome this particular limitation in generalized epilepsy. Unfortunately, there are limitations in precise localization of the deeper structures, such as individual thalamic nuclei. Compared to MEG, functional magnetic resonance imaging (fMRI) has a better spatial but weaker temporal resolution (46). With advances in technology, there have been publications (40, 44, 47, 48) focusing on the multimodal integration of MEG with other neuroimaging techniques, mainly fMRI, to complement one modality with the other to further edify the underlying pathophysiology of the GSWDs.
In this review article, we searched PubMed, Medline, and Embase databases using the following search algorithm: “Magnetoencephalography (MEG)” and “Generalized Epilepsy” or “absence epilepsy” or “myoclonus epilepsy” or “generalized genetic epilepsy” limited to publications in English. The last date of the search was September 30th, 2021. We screened the titles, abstracts, and references of all search results to identify potentially relevant studies. We included only publications of MEG recordings in human subjects. We excluded poster publications and any study with abnormal MRI in generalized epilepsy patients. This article aimed to provide a comprehensive literature review of how the recent innovation in the MEG methodology contributed to the literature of the idiopathic generalized epilepsy in terms of physiopathology, treatment, and prognosis, thus further blurring the boundary between focal and generalized epilepsy.
Different Source Localization Models, Techniques and Connectivity Analyses
Cohen et al. were the first to record neural magnetic signals using a single-channel MEG (49). Since then, the MEG recording technique has been enhanced, and now the neural magnetic signals can be recorded using more than 200–300 sensors (31, 43). With this advancement, source estimation models have been developed to localize the neural magnetic signals (26, 30–32, 37, 39, 40, 43, 50). Source analysis usually occurs in the source space rather than sensor space, where the neural signal is acquired at each measurement sensor. Due to the various ambiguities associated with sensor level analysis, source analysis is preferred, but sensor level analysis can be performed when there are not enough sensors, e.g., analyzing 10–20 EEG neural signals, to accurately localize sources (42, 51). The goal of source localization is to correctly estimate the location and orientation of the neuromagnetic source using the inverse model (37). Multiple mathematical algorithms have been developed, but non-e is felt to be superior for every clinical situation. Each algorithm comes with its own advantages and limitations. Besides, there have been studies showing overall agreement in estimating the sources when compared to intracranial EEG (52–54).
Dipole Model
Dipole models are characterized by a single or few neural sources that are analyzed in the brain model and then sequentially moved until the projected single pattern matches the recorded pattern (30, 31). Among all, the single equivalent current dipole model (sECD) is the most well accepted, but the traditional ECD model is limited if the underlying assumption of focality is not fulfilled (30–32). Using point source analysis, the dipole modeling becomes limited and unreliable if the source is complex, multiple sources are generated over the same temporal course, or the source is generated from extended areas of the brain (26, 33–35, 55).
Multiple Signal Classification
Multiple signal classification (MUSIC) can analyze complex and asynchronous sources that typically require multiple simultaneous source localization by scanning all possible positions of the brain in three-dimensions. However, two assumptions need to be met for accurate localization, an absence of noise and an accurate head model (34, 56, 57). Unlike ECD, the recursive MUSIC (R-MUSIC) algorithm can localize multiple synchronous sources using the spatio-temporal independent topographies (IT) model (58).
Beamforming
Instead of estimating or reconstructing the source distribution, beamforming uses spatial filters to optimize predefined regions of interest or sources with a maximum signal while suppressing activity from other regions, including noise (59). Beamforming can be further divided into either linear, linearly constrained minimum variance (LCMV) (60) or non-linear, synthetic aperture magnetometry (SAM) (61). LCMV beamforming can be analyzed either in time domain using covariance metrics or in the frequency domain using cross-spectral density metrics, such as dynamic imaging of coherent sources (DICS) or accumulated spectrograms, such as accumulated source imaging (ASI) (59–63). Compared with ECD, beamforming can analyze multiple sources, either synchronous or asynchronous. Contrary to MUSIC, the neural signal analyzed with beamforming is less altered by the presence of noise. One of the limitations of beamforming, especially SAM with excess kurtosis, is performance loss when the sources are correlated. In addition, SAM with excess kurtosis [SAM(g2)] ignores frequent events, and thus it is limited in analyzing frequent discharges (59, 61).
Current Density Models
Current density models directly compute a current distribution throughout the full brain volume: minimum norm estimate (MNE), standardized low-resolution brain electromagnetic tomography (sLORETA), exact low-resolution brain electromagnetic tomography (eLORETA), sLORETA weighted accurate minimum norm (SWARM), dynamical statistical parametric mapping (dSPM), and the multiresolution focal underdetermined system solution (MR-FOCUSS) (64–67). In MNE, dipoles are analyzed simultaneously in two-dimensions by limiting the space so their strengths can be estimated as the function of time (68). MNE has an excellent spatial resolution for the superficial sources, especially complex sources, but not for deeper sources due to the model limitation (69). To improve the superficial source bias, sLORETA performs further post-processing of the current density map obtained from the MNE by replacing the noise covariance with theoretical data covariance (65). Another normalization method of the MNE current density is dSPM which computes the normalization based on the noise covariance (70, 71). In addition, the presence of biological noise has no localization bias in the source estimation of the neural signals by sLORETA (67). dSPM and LORETA improve the localization error when compared to MNE (67, 72). To improve the analysis of complex dynamic sources using the time domain, particular models are a promising technique for the ictal dynamic data, especially MR-FOCUSS (73).
Entropy Measures
Maximum entropy on the mean (MEM) is a technique to analyze synchronous sources in specific frequency bands and is sensitive to spatially extended sources using data-driven parcellation of the cortical surface into non–overlapping parcels. By maximizing the entropy of a probability distribution, the parcels that are not contributing to the measured data are excluded from the analysis (74). Pairwise MEM (pMEM), a statistical model of pairwise regional coactivation from empirical data using frequency-specific MEG resting oscillatory activity, can analyze the dynamic state's multi-stability (75, 76). The limitation of MEM is that a priori information on the number of cortical parcels is required (77).
Connectivity Measures
Over the past decades, studies have been focused on analyzing various cortical networks using diverse connectivity measures to describe the disruption of the disease state from the normal functional neural networks (78–80). In contrast to anatomical connectivity, where networks of physical white matter structural connections or synaptic connections between various (distinct) regions of the brain analyzed at the micro or macroscopic occurs, functional and effective connectivity describes the functional aspects of neural networks (81). Functional connectivity measures the temporal correlation of distinct cortical regions, whereas effective connectivity analyzes the direction of the influence of one cortical region over distinct cortex (79, 81–86). Therefore, functional connectivity analyzes whether neural activities of the two regions are linked, i.e., undirected information flow, while effective connectivity scrutinizes the direction of the communication, i.e., directed connectivity (87–89).
Correlation and coherence are the most classical measures of functional connectivity and analyze the similarity between neural signals in the time and frequency domains, respectively (90). Other functional connectivity methods are based upon quantifying the waveforms in amplitude and oscillations of neural activity, such as phase lag index, phase slope index, or phase-locking index (91, 92). If the directional interactions are pre-defined, structural equation modeling (SEM) can be used, whereas Granger causality measures the connectivity on directional interactions derived from the data (82, 89, 93). Other effective connectivity methods are directed coherence, dynamic causal modeling, linear non–Gaussian, conditional Bayes, and Bayes network methods (94–99). The main difference between functional and effective connectivity is that functional connectivity illustrates statistical dependencies, whereas effective connectivity is based on a mechanistic model of the causal effects that generated the data (87, 100).
Graph theory provides models of complex dynamic networks in the brain, allowing one to better understand the relations between and/or the processes taking place in network structures. After the connectivity matrices are calculated, these values can be used to describe features of the network using graph theory, i.e., the network is defined as a set of nodes that are connected by edges or lines. This allows the investigator to calculate measures of different graph metrics, such as degree (number of connections to a node), node strength, path length, global efficiency, clustering coefficients, between centrality, synchronizability, small world index and centrality, to identify the critical components of the network (101–105).
First Clinical MEG Recording in Generalized Epilepsy (Source Localization ERA)
Hughes et al. were the first to report a clinical MEG recording of 3 Hz generalized spike and wave discharges (GSWDs) in humans using simultaneous EEG and MEG recording (106). Interestingly, they observed that MEG was excellent in displaying the spikes and less evidence of waves when compared to the EEG. In addition, MEG waveforms were noted to precede the corresponding EEG spike activity in most patients' recordings. Ricci et al. studied the 3 Hz spike-wave using single-channel MEG with a phantom brain model and showed cortical activity was scattered bilaterally, mainly over frontal and temporal regions, often with more involvement over one hemisphere, while bilateral synchronous activity seemed to have originated from a deeper structure (107, 108). The study was the first to demonstrate evidence of primary cortical involvement in GSWDs in generalized epilepsy using neuromagnetic cortical source localization. The authors couldn't explain the relation of the cortical source localization to the deep brain structures given the limitation of the applied methodology with single-channel recordings (109). Thus, the author recommended further studies using multichannel capability with newer post-processing methodology to glean greater insights into the pathophysiology of generalized epilepsy (109).
Childhood Absence Epilepsy
Childhood absence epilepsy (CAE) is the most studied generalized epilepsy among all genetic or idiopathic generalized epilepsy subtypes. All the published study characteristics, types of post-processing signal analysis, and main results are summarized in Table 1.

Table 1. Showing all the published study characteristics and main outcomes on childhood absence epilespy.
Source Localization of GSWDs
Multiple studies were published using different source localization techniques to analyze interictal, preictal, and ictal parts of the GSWDs of CAE, as shown in Table 1. Westmijse et al. applied source analysis to ictal GSWDs of human CAE with an average seizure duration of 9s (4–22 s) using non–linear association with the beamformer technique, synthetic aperture magnetometry (SAM). At the onset, sources were localized to cortical brain regions, including left or right frontal, central and parietal, during the spike portion of GSWDs. The sources became generalized during the slow-wave phase (110). A similar finding was reported by Hu et al. using the same technique while analyzing the peak of the spike of GSWD (111). Five out of 13 CAE patients' GSWDs (38.5%) were able to source localized to bilateral frontal regions. The study findings validated the clear cortical sources of activity during the spikes of GSWDs over the bilateral frontal regions and supported the theory of the cortical focus in the generation of generalized epilepsy (111). However, no conclusion could be made regarding deep brain sources (mainly thalamus) due to the limitation of the recording and analysis technique, including the limited high frequency to 70Hz.
Similar to the findings from Rucci et al. (107, 108) and Tenney et al. reported the preictal MEG changes occurred an average of 694 ms before the initial spike of the EEG (112, 114). The same research group (112) aimed to investigate the relative timing of the cortical and thalamus activity in the generation of absence seizures by combining SAM beamformer and standardized low-resolution electromagnetic tomography (sLORETA) to analyze the preictal state, 50 milliseconds (ms) before and after the first ictal spike of ictal GSWDs, in 12 drug-naïve CAE patients. At −50 ms, the seizures were source localized to the frontal cortex, mainly the lateral inferior frontal lobe or thalamus. At the EEG onset (0 ms), focal sources were detected in the frontal cortex with decreased thalamic localization. Following the first ictal spike (50 ms), localization became more widespread. Thus, after the initial frontal and thalamic source, the ictal activity gradually recruited the remaining cortices, i.e., parietal, temporal, and occipital. Later, the same group analyzed the same ictal dataset using time-frequency analysis with different frequency bandwidths (up to 150 Hz) and source localization using sLORETA (113). Tenney et al. were the first to report the network's frequency-dependent nature in CAE (113). The high-frequency oscillations (HFO) 70–150 Hz were localized to the frontal lobe during absence seizures. At lower frequencies, sources were significantly localized to the parietal cortex. Thus, the authors proposed a hypothesis that different oscillations and frequencies favored different types of connections and/or different spatiotemporal levels of information integration. In addition, the finding suggested that the co-occurring frontal and parietal corticothalamic networks interacted to produce a pathological state that contributed to the generation of GSWDs.
The above findings were confirmed by Miao et al. using different beamformer analysis, dynamic magnetic source imaging (dMSI) (115, 116). Miao et al. validated that the source of HFOs (80–500 Hz) in the ictal stage was focal and located in the medial prefrontal cortex (MPFC) compared to the spike portions of the interictal GSWDs, which were widespread (116). In addition, Miao et al. reported that fast ripples (250–500 Hz) were associated with increased seizure frequency (115). Besides, same research group also confirmed the involvement of the default mode network, by reciprocal propagation between medial prefrontal cortex, pre-supplementary motor area, precuneus, and medial occipital cortices, through cortico-cortical pathways via medial portion of the brain or cortico-thalamus-cortical pathway via thalamus, in the ictal generation and propagation of the seizure activity in CAE (115). Compared to the ictal stage, Xiang et al. studied HFO activity during the interictal stage and compared it with age- and sex-matched healthy controls (117). The authors revealed that patients with CAE had higher odds of interictal HFO activity (either 200–1,000 or 1,000–2,000 Hz) in the parieto-occipito-temporal junction and medial frontal cortices. No significant differences in the deep brain area was reported. Thus, all the above results indicated that CAE had significantly aberrant brain connectivity activity during the interictal as well as the ictal phase, and the above electrophysiological findings could potentially serve as biomarkers for the CAE.
Connectivity
Resting-State
Resting-state connectivity of CAE is illustrated in Figure 1. Chavez et al. compared the modular organization of the brain networks' resting-state connectivity between CAE patients taking ASMs and normal healthy subjects (125). Increased connectivity with clear modular structures, subsets of units within a network, was noted in the epileptic brain at the extended alpha band (5–14 Hz). Modularity analysis revealed that nodes of epileptic brain networks were abnormally linked to different functional modules in distinct networks compared to the normal healthy subjects. To confirm whether taking ASM might be the contributing factor for altering the resting-state connectivity, Wu et al. studied resting-state connectivity in treatment naïve CAE by constructing effective connectivity (EC) using correlation and Granger causality analysis, and were the first to reveal frequency-specific alteration in EC during the resting state without 3 Hz GSWDs (8). Compared with the healthy control group, strong connectivity in the frontal lobe and weakened connectivity in the anterior-posterior pathway were noted within the delta band (1–4 Hz). In the low-frequency band (1–8 Hz), the effective network activity involving the precuneus and posterior cingulate cortex (PCC/pC) was significantly decreased in patients with CAE (8). The reduced resting functional connectivity in PCC/pC has also been reported in patients with attention deficit disorder and memory impairment (130). Thus, these particular changes may be partially responsible for behavioral and cognitive co-morbidities seen in many patients with CAE (131).
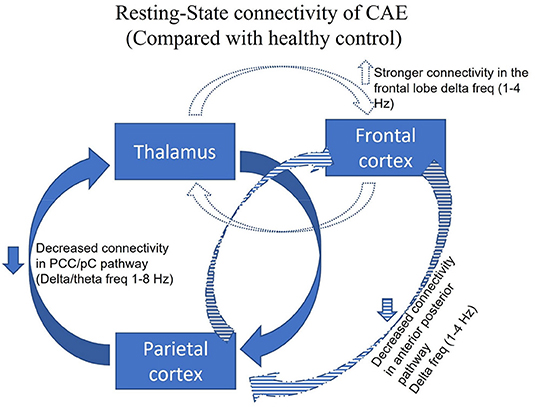
Figure 1. Adapted with permission from Tenney et al. (47). Figure showing the resting-state connectivity in CAE, without 3 Hz GSWDs, compared with the healthy control. The three major brain regions have been identified as responsible for the generalization of the childhood absence seizures (thalamus, frontal cortex, parietal cortex). Given all the data from Wu and colleagues, compared with the healthy control group, strong connectivity in the frontal lobe at 1–4 Hz (blue dotted) and a weakened connectivity in the anterior-posterior pathway was noted within the delta band 1–4 Hz (blue stripped). In the low-frequency band at 1–8 Hz (blue solid), the effective network activity involving the precuneus and posterior cingulate cortex (PCC/pC) was significantly decreased in patients with CAE (8).
Ictal Network Connectivity
Using dynamic imaging of coherent sources (DICS) beamformer, Gupta et al. studied the transitions between interictal, preictal, and ictal periods of absence seizures (within 1s of first ictal GSWD) and confirmed frequency-dependent source localization (119). The consistent appearance of low frequency 2–4 Hz frontal and occipital cortical source was noted before the first generalized spikes, and change in the connectivity networks was noted at the onset of the GSWD, suggesting the increased connectivity from preictal pathologically predisposed network toward the rapidly recruiting synchronous ictal network. Using accumulated source imaging (ASI) beamformer analysis to quantify the network connectivity changes from a preictal to an ictal state, Wu et al. demonstrated that the dynamic changes in neural networks probably resulted from the cortically initialized cortico-thalamic network and analyzed neuromagnetic data as low-frequency (1–80 Hz) and high frequency (80–200 Hz) (120). During the transition period, the predominant neuromagnetic activities were observed at low-frequency (1–80 Hz) dominantly in the frontal and parieto-occipito-temporal cortices, whereas those in the deep brain areas and medial frontal cortex were at a high-frequency band (80–500 Hz) when compared to the interictal period. The EC was mainly over the cortical regions during the interictal period, but when the ictal transition occurred, there was a strong EC between cortex and deep brain areas in both low- and high-frequency ranges. Interestingly, the direction of the EC was predominantly from the cortex to the thalamus in the early ictal period. The same research group conveyed that indeed the rhythmic ictal spiking activity of GSWDs (within 1 s of the ictal spike onset) played a dominant role in the synchronization of the CAE epileptic network at the spike of the GSWDs (at 1–4, 4–8, and 8–12 Hz) which was significantly different from that of the slow-wave of the GSWDs (123). Thus, the dynamically balanced network was distorted primarily by the increased excitatory connections subtending a spike part of the GSWDs. Yet, the connections were mostly excitatory at the high-frequency band (80–250 Hz) regardless of spikes or slow waves. Thus, the authors suggested that abnormal excitatory activity of the entire brain required a local cluster of neurons to initiate the spike discharges, which caused the synchronous hyper-excitability in the epileptic network. Using whole-brain connectivity analysis and linear constraint minimum variance (LCMV) beamformer at the broadband frequency (1–40 Hz), Youssofzadeh et al. tried to reveal the focal components of the absence seizures in effective connectivity (EC) and investigated the network contrast between ictal and preictal period (121). The highly connected brain areas or hubs in the thalami, precuneus and cingulate cortex generally supported a theory of rapidly engaging and bilaterally distributed networks responsible for seizure generation (121).
Not only the ictal transition but also the ictal termination had been studied. Jiang et al. investigated the network changes within the 2 s of ictal termination in drug-naïve CAE using beamformer (ASI) and graph theory connectivity analyses (122). At the low-frequency (1–80 Hz) bands, the activities were predominantly distributed in the frontal and parieto–occipito–temporal junction, whereas sources of HFOs (80–500 Hz) were localized to the medial frontal cortex and deep brain areas (mainly thalamus) during both interictal period and the termination transition. Furthermore, an enhanced positive cortico–thalamic EC was observed around the discharge offset with its direction primarily from various cortical regions to the thalamus (122). Sun et al. re-investigated ictal termination (within the 3 s of transition) of absence seizures and found the transition to be associated with dynamic frequency-dependent changes in the functional connectivity (124). At 1–4, 4–8, and 8–12 Hz, the magnetic source during seizure termination appeared to be consistent over the ictal period and was mainly localized in the frontal cortex and parieto-occipito-temporal junction. At ictal termination, source activity and peak source strength were significantly reduced in the frontal lobe at 12–30 and 30–80 Hz. Thus, the finding from the study, as mentioned above, suggested that the neuromagnetic activity in different frequency bands might play a role in activating or deactivating different cortical networks, such as frontal corticothalamic, parietal corticothalamic, default mode network, etc., and responsible for the pathophysiological mechanism of CAE.
To confirm the hypotheses of whether the interaction of co-occurring networks at distinct frequencies interact through cross-frequency coupling mechanism effects, Tenney et al. complemented neuromagnetic signal analysis, beamformer (LCMV), and cross-frequency canonical analyses with fMRI to increase the spatial resolution and analyze cross-frequency coupling (CFC) (48). The fMRI informed MEG effective connectivity (EC) (spatial map of the ictal network was defined using the fMRI and used as a prior for MEG connectivity) study showed beta/gamma CFC and within frequency coupling in frontoparietal and frontofrontal regions during the CAE seizures. Strong coupling between beta and gamma frequencies within the left frontal cortex, and between left frontal and right parietal regions were observed. There was also strong connectivity between left frontal and right parietal nodes within the gamma bands. Multilayer versatility analysis showed that a cluster of network hubs in the frontal regions and thus frontal cortical regions were critical for absence seizure generation (48). Thus, all the findings from the ictal connectivity studies consistently show different cross-frequency coupling or distinct frequency-dependent activation and deactivation of cortical network initiation followed by abrupt synchronization between cortical and subcortical structures in the generation, propagation, and the termination of the CAE seizure, which further supports the cortical focus theory.
Difference Between Ictal and Interictal Connectivity
Ictal and interictal GSWD connectivity were studied using ASI beamformer and correlation analyses to investigate the clinical ictal symptoms related to the ictal CAE epileptic network and illustrated in Figure 2. Shi et al. investigated the differences between the interictal GSWDs (<4 s) and ictal GSWDs (>10 s) in CAE (126). The low frequency (4–8 Hz and 8–12 Hz) magnetic sources were mainly localized within the posterior cingulate cortex and precuneus (PCC/pC) during the interictal state. The high frequency (80–250 Hz) magnetic components of the ictal GSWDs were mainly localized in the medial frontal cortex. In terms of connectivity (using posterior cingulate and precuneus (PCC/pC) as the seed), there were strong connections in the anterior-posterior pathway, mainly the frontal cortex during the ictal GSWDs. In contrast, the connections were mostly limited to the posterior cortex region at 80–250 Hz during interictal GSWDs. Thus, there were significant disparities in the source localization between ictal and interictal GSWDs. The low-frequency activation in the PCC/pC during the interictal period might be related to maintaining consciousness during the interictal GSWDs. Shi et al. concluded that weakened network connections during the interictal GSWD might be in favor of preventing overexcitability and relates to the termination of GSWDs (126). Thus, the finding concurs with the conclusion made by Wu et al. (8). There is reduced resting functional connectivity in PCC/pC patients with CAE in not only interictal but also resting state.
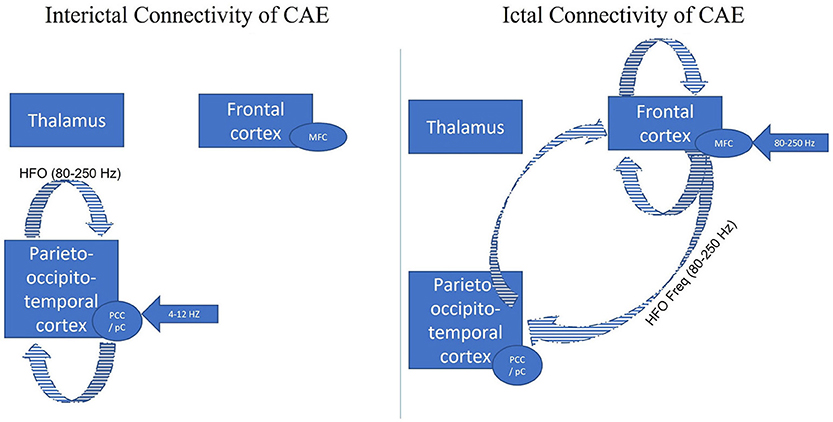
Figure 2. Adapted with permission from Tenney et al. (47). Figure comparing the interictal (GSWDs <4 s) and ictal connectivity (GSWDs > 10 s) in CAE. The three major brain regions have been identified as responsible for the generalization of the childhood absence seizures (thalamus, frontal cortex, posterior cortex). Given all the data from Shi et al. (126) the low frequency (4–8 Hz and 8–12 Hz) magnetic sources were mainly localized within the posterior cingulate cortex and precuneus (PCC/pC) during the interictal state (blue arrow). The high frequency (80–250 Hz) magnetic components of the ictal GSWDs were mainly localized in the medial frontal cortex (blue arrow). In terms of connectivity (using posterior cingulate and precuneus (PCC/pC) as the seed), there were strong connections in the anterior-posterior pathway, mainly the frontal cortex during the ictal GSWDs (blue stripped). In contrast, the connections were mostly limited to the posterior cortex region at 80–250 Hz during interictal GSWDs (blue stripped). Thus, there were significant disparities in the source localization between ictal and interictal GSWDs.
To confirm the findings, the same research group (127) studied the functional connectivity reorganization of the brain regions in both interictal without GSWD (30 s of the interictal period without GSWDs at least 60 s from the ictal period) and ictal GSWD network using two frequency band activities (1–30 Hz and 30–80 Hz). Compared to the interictal period, frontal, temporoparietal, and parietal regions were more active during seizures. On the contrary, the precuneus, the cuneus, and the thalamus were relatively silent during the ictal period compared with the interictal period. The differences in source localization between ictal and interictal states were reported, regardless of seizure duration, seizure frequency, or the age of epilepsy onset. Thus, the available data suggests the role of different frequency-dependent initial cortical involvement, most importantly in the frontal region, with predisposing hyper-excitable corticothalamic synchronization in the generation of the CAE.
Treatment and Prognostic Biomarker
Miao et al. reported that the presence of fast ripples (250–500 Hz) HFO in absence seizures was associated with increased seizure frequency (116). Similarly, Tang et al. studied whether the HFO in drug-naïve CAE was related to seizure severity and reported that the strength of ictal HFO (200–1,000 Hz) was significantly correlated with the severity of the absence seizures measured by the number of daily seizures, therefore a potential prognostic biomarker (118). Sun et al. later reported that the ictal peak source strength in the 1–4 Hz range was negatively correlated with the ictal duration of the seizure, whereas at 30–80 Hz, there was a positive correlation with the course of epilepsy (124). Yet, both studies were not able to draw major conclusions due to the limitation of a cross-sectional study (116, 118).
Thus, a couple of studies were conducted in which patients with drug-naive CAE underwent a MEG recording at the time of diagnosis (or within 1 week of diagnosis) and followed up for at least 1 year. The difference in the pretreatment ictal connectivity in patients with CAE was studied in response to ASMs treatment (responder vs. non-responder) and illustrated in Figure 3. Tenney et al. used fMRI-informed MEG effective connectivity to study prognostic biomarkers prospectively in drug-naive CAE patients with a follow-up for at least 2 years after starting the ethosuximide (ETX) (47). Pretreatment connectivity demonstrated the strongest connections in the thalamus and posterior head regions (parietal, posterior cingulate, angular gyrus, precuneus, and occipital) at low frequency (delta 3–4 Hz) and the co-occurring frontal cortical thalamic network at the high frequencies (beta/gamma 13–55 Hz). ETX non-responders' pretreatment connectivity decreased in the precuneus region and increased in the frontal cortex compared to ETX responders. This increased frontal cortical connectivity may be a potential prognostic biomarker of drug-resistance. Miao et al. also studied the responders and non–responders to the two established ASMs, lamotrigine (LTG) and valproic acid (VPA), using a beamformer (ASI) (128). In six LTG-non-responders CAE patients, ictal source locations were noted in the posterior-dorsal medial frontal cortex (post-DMFC including medial primary motor cortex and supplementary sensorimotor area) at 1–7 Hz or 8–30 Hz but not in 9 LTG responders, regardless of the age of onset and the seizure frequency. In addition, the authors suggested that ictal post-DMFC source localization could be suggestive of a biomarker for predicting LTG non–responsiveness. Zhang et al. replicated the same findings in CAE patients using the same beamformer technique (ASI) (129). The source localization of the ASMs non-responders was mainly in the frontal cortex at 8–12 and 30–80 Hz, especially the medial frontal cortex at alpha frequency. The non-responders showed strong positive local frontal connections and deficient anterior and posterior connections at 80–250 Hz. Thus, while it is likely that no one single mechanism can explain the pharmacologic responsiveness, ASM non-responders had more source localized within the dorsomedial frontal regions with decreased anterior-posterior network connectivity. At this time, the available preliminary data shows promising results in prognosticating response to ASM, but further studies with a larger sample size as well as comparing types of ASM non-responders are warranted to study the causality association.
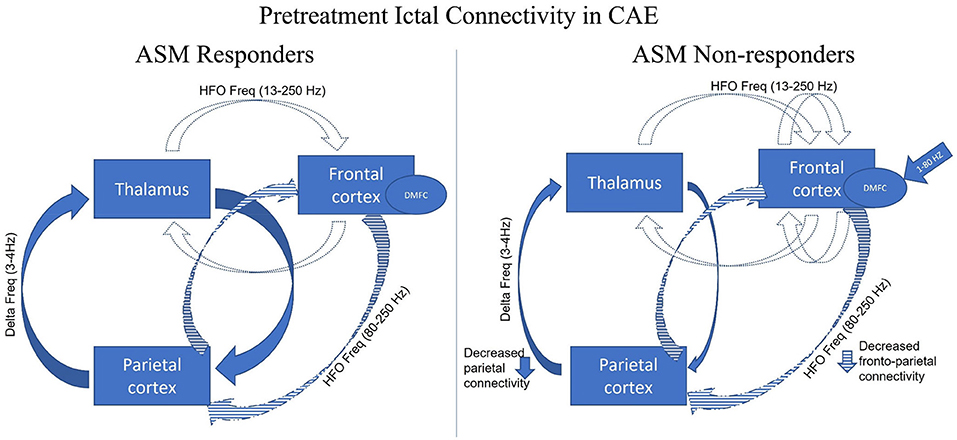
Figure 3. Adapted with permission from Tenney et al. (47). Figure showing pretreatment ictal connectivity analysis of the antiepileptic (ASM) (ethosuximide, lamotrigine, and valproic acid) responder and non-responder in CAE. The three major brain regions have been identified as responsible for the generalization of the childhood absence seizures (thalamus, frontal cortex, parietal cortex). Given all the findings collected from Tenny et al. (47), Miao et al. (128), and Zhang et al. (129), parietal cortico-thalamic network at the low frequencies (blue solid) and a co-occurring frontal corticothalamic network at higher frequency (blue dotted) and anterior-posterior/frontoparietal network at higher frequency (blue stripped). Compared to the responder, ASM non–responders have ictal neuromagnetic sources at 1–80 Hz localized to the dorsomedial frontal cortex (DMFC) (blue arrow). In addition, the ASM non–responders have decreased parietal cortico-thalamic at 3–4 Hz and frontoparietal network connectivity at 80–250 Hz with increased frontal local connectivity at 13–250 Hz.
Thus far, neuromagnetic source localization identifies three major brain areas which are thought to be responsible for the generation, propagation, and termination of CAE GSWDs: frontal cortex, parietal cortex, and thalamus with earlier cortical sources (more than 500 ms), supporting the concept of cortical focus theory. In addition, the current MEG literature suggests that the pathophysiology of GSWD in the absence seizure is a reflection of the co-occurring (excitation or inhibition) network(s) pathology rather than dysfunction in one particular brain area. For instance, frontal hyperexcitability and parietal deactivation involving intact but altered EC networks, such as corticothalamic, corticocortical and default mode networks, triggering the rapidly generalized epileptic discharges.
Juvenile Absence Epilepsy (JAE)
Although JAE and CAE share many similar clinical characteristics, CAE absence seizure has more pronounced impairment of consciousness, and tonic-clonic seizures are less common than JAE. In terms of EEG, GSWDs of JAE are usually a higher frequency at 4–5 Hz. In terms of prognosis, JAE has a slightly worse prognosis when compared to CAE (132). Studies published on JAE are illustrated in Table 2.
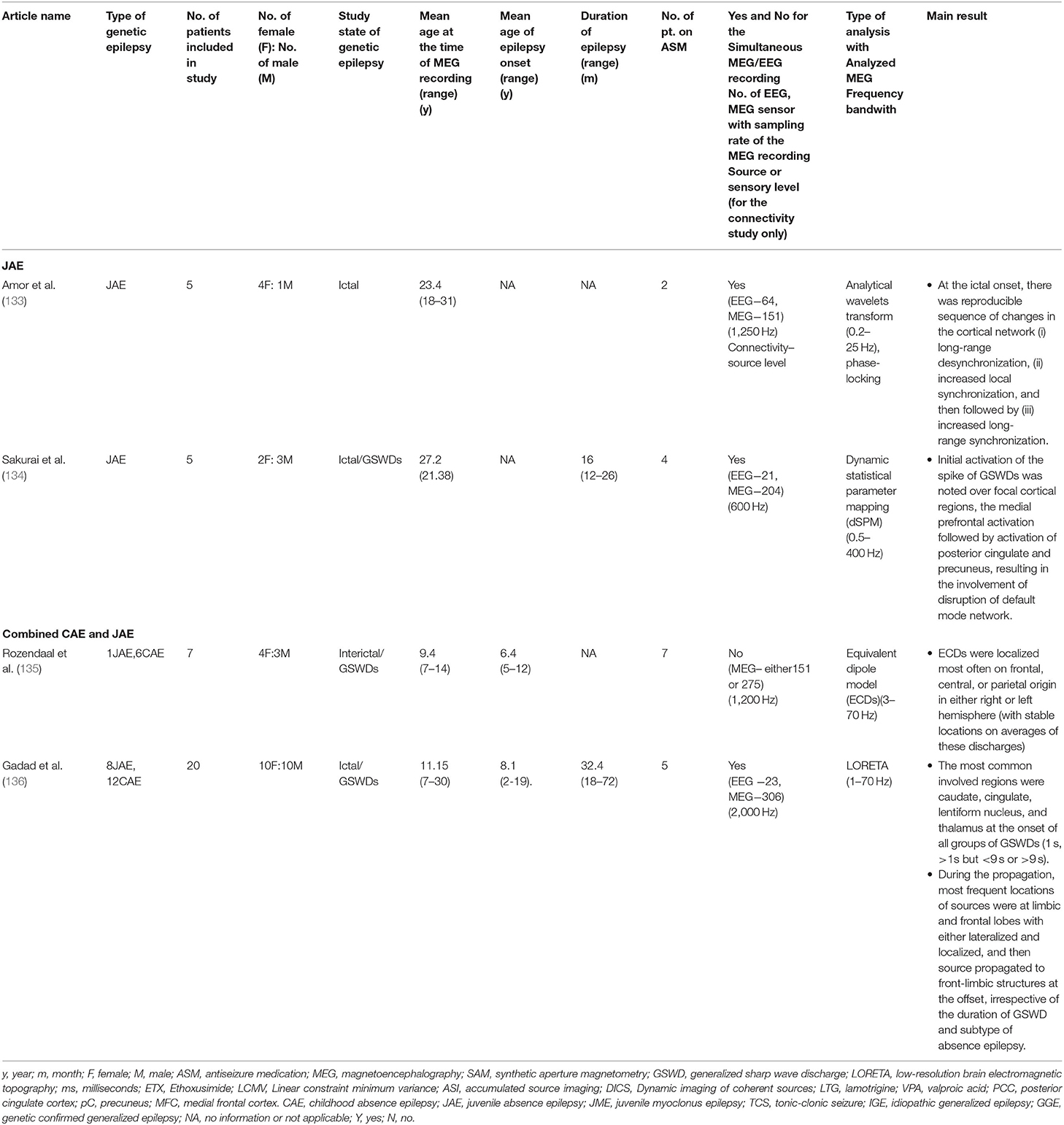
Table 2. Showing all the published study characteristics and main outcomes on juvenile absence epilepsy and combined absence epilepsy.
Amor et al. explored the spatio-temporal dynamics of interactions within and between widely distributed cortical sites using MEG in patients with JAE (133). At the ictal onset, localized phase synchronization in multiple frontal and precentral areas was recorded, and the activity preceded the first ictal EEG GSWDs by 1.5 s. The analyses revealed a reproducible sequence of changes in the cortical network: (1) long-range desynchronization, (2) increased local synchronization, and (3) increased long-range synchronization. However, both local and long-range synchronization displayed different spatio-temporal profiles, but the cortical projection within the initiation time window (500 ms before the first ictal GSWDs) overlapped multifocal fronto-central regions, such as left frontomedial, frontopolar, right orbitofrontal, and right latero-central regions. Sakurai et al. studied the source analysis of the GSWDs in 5 patients with JAE using a dynamic statistic parametric mapping (dSPM) approach (134). The researchers reported that the initial activation of the GSWDs appeared in the focal cortical region with strong activation over the medial prefrontal activation followed by posterior cingulate and precuneus in 3 out of 5 patients simultaneously right after the medial prefrontal activation (134). The area mentioned above involved the default mode network at the onset of the GSWD, and thus it wasn't random diffuse cortical involvement but rather a selective cortical network, particularly the default mode network.
Combined Absence Epilepsy (Childhood and Juvenile Absence Epilepsy)
Studies published on combined absence epilepsy (CAE and JAE) are illustrated in Table 2. Rozendaal et al. attempted to compare the interictal and ictal periods in absence epilepsy (6CAE and 1JAE) using the SECD model, and source localizations were most often frontal, central, and parietal regions in either left or right hemisphere (135). The spatiotemporal assessment of the interictal epileptiform discharges (IEDs) indicated a stable localization of the averaged discharges, indicating a single underlying cortical source. Using LORETA, Gadad et al. studied the source analysis of the GSWDs at the onset, during, and offset of the GSWDs based on the duration of GSWDs and divided into three groups: GSWDs lasting 1 s, more than 1 s but less than 9.9 s, and equal to more than 10 s (136). The authors reported that the most common involved regions were caudate, cingulate, lentiform nucleus, and thalamus at the onset of all average discharges. Thus this observation substantiated the previously documented thalamo-cortico-stratum involvement in the absence of epilepsy (22, 23). During the propagation, the most frequent localization of sources were at limbic and frontal lobes, and these sources propagated to fronto-limbic structures at the ictal offset, irrespective of the duration of GSWD and subtype of absence epilepsy. The finding indicated the restricted/sustained network circuitry in fronto-limbic network involvement in origination and propagation of GSWDs until the disruption and inhibition. No significant difference in the source localization or network involvement was noted between CAE and JAE.
Juvenile Myoclonus Epilepsy (JME)
Source Localization of GSWDs
Studies published on JME are illustrated in Table 3. Kotini et al. reported 2 adults with JME using the multiple signal characterization (MUSIC) algorithm and showed that the dipolar sources of the peak of GSWDs were localized at the cerebellar vermis with an extension up to the occipital region (137). Instead of analyzing at peak of the spike, Gadad et al. studied the source analysis of average GSWDs in three different spike phases: onset (upward phase of the spike from the baseline), peak, and offset (trailing edge of the spike) using LORETA in 20 patients with JME. At the onset of the GSWDs, the majority of the neuromagnetic sources were localized to sublobar regions (31% of localized discharges) defined as insula, caudate, claustrum, lentiform nucleus, and thalamus, followed by limbic region (22%), frontal (22%) and temporal lobe (11%). At the peak of the discharges, the sources were localized to the frontal lobe (45%), followed by sublobar regions (23%) (mainly lentiform nucleus). At the offset of the discharges, the sources were localized to the sublobar region (28%) (mainly caudate), followed by limbic (24%) and frontal regions(18%) (138). Therefore, the available evidence suggests an overall synchronous on and off interaction of cortico-subcortical structures in generating and propagating the epileptiform discharges in JME.
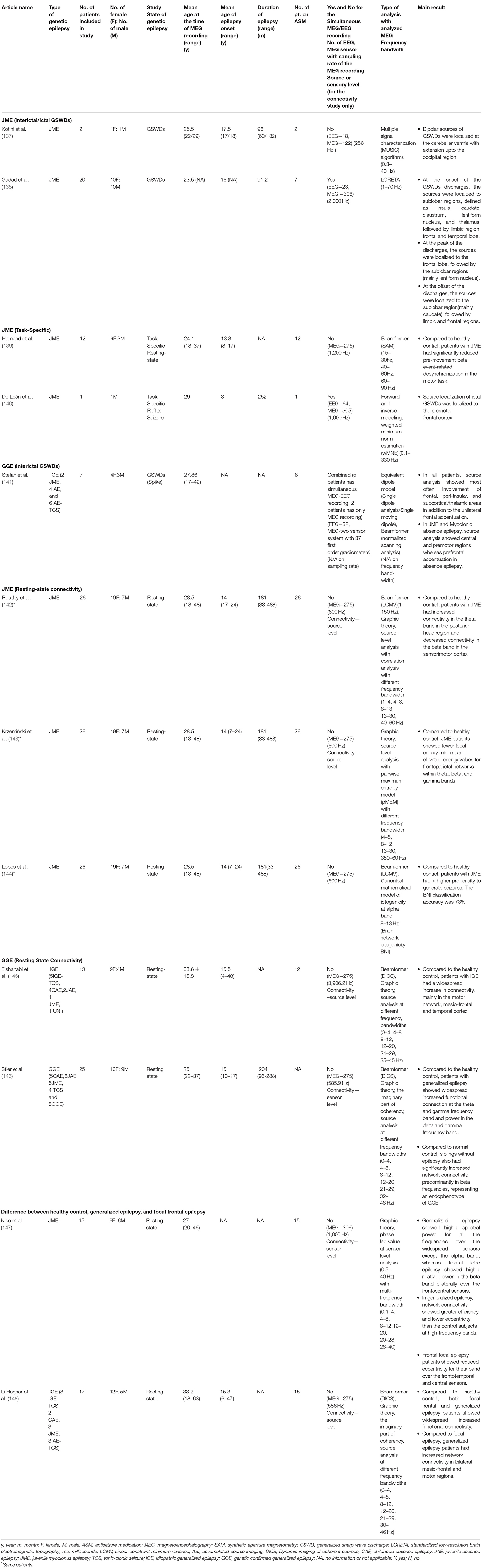
Table 3. Showing all the published study characteristics and main outcomes on juvenile myoclonic epilepsy and combined genetic epilepsy.
Network Connectivity
Resting-State
Three publications from the same research group reported and studied the 26 JME patients taking ASM using three different neuromagnetic source localization and connectivity techniques (142–144). Routley et al. studied resting-state functional connectivity in 26 patients with JME and reported that the altered resting-state connectivity could be a neuropathophysiological hallmark or potential diagnostic biomarker for JME. Compared to the healthy control group, there was overall increased connectivity in the posterior head regions in theta and alpha bands, and decreased connectivity in the pre and post-central brain region in beta bands. The reported increased connectivity in the posterior theta-frequency band might be associated with long-range connections affecting attention and arousal. The decreased beta band sensorimotor connectivity might be related to the resting state sensorimotor network and seizure-prone states in JME (142). Using a pairwise maximum entropy model, Krzeminski et al. studied the divergent oscillatory power in different networks: frontoparietal network (FPN) (ROIs: middle frontal gyrus, pars triangularis, inferior parietal gyrus, superior parietal gyrus, and angular gyrus), default mode network (ROIs: orbitofrontal cortex, precuneus, posterior cingulate, anterior cingulate and angular gyrus), and sensorimotor network (ROIs: supplementary motor area, precentral gyrus, and postcentral gyrus). Compared with the healthy control group, JME patients had fewer local energy minima and had elevated energy values for the FPN within theta, beta, and gamma bands during the resting state. No significant changes were noted between the default mode and sensorimotor networks using this method (143). Similar to the findings seen in CAE, these results highlighted the involvement of FPN in the pathophysiology of the JME.
Lopes et al. studied the same cohort of JME patients to investigate computational biomarkers using brain network ictogenicity (BNI), a computational modeling method, to generate the synthetic activity fluctuating between resting and seizure states (144). The higher values of the BNI represent a higher inherent propensity of the brain to generate seizure activity. Lopes et al. reported that patients with the JME had higher BNI values than healthy controls, and sensitivity was reported to be 0.77, and specificity was 0.58, with an area under the curve was 0.72 (144). But the model couldn't be generalized beyond JME as there was no study comparing other types of epilepsy.
Task-Specific Cortical Modulation
Hamandi et al. studied the resting state response in task-specific cortical modulation in occipital and sensorimotor cortices in JME compared to healthy control individuals (139). The authors reported that patients with JME had significantly reduced pre-movement beta event-related desynchronization in ipsi- and contralateral sensorimotor areas compared to controls, before and during the transient movement of motor tasks. There was no difference between epileptic and health patients in movement-related gamma synchronization and post-movement beta rebound. In addition to the physical motor task, De León et al. reported a case of mental calculation induced seizure in a patient with JME where the source was localized to the right premotor frontal cortex using the weighted minimum norm estimates (140). Similar to the result presented by Routley et al. and Krzeminski et al. with decreased sensorimotor connectivity, the current two task-specific JME patients suggested an abnormality in motor planning in JME likely related to the altered resting-state sensorimotor network and seizure-prone states in the JME (142, 143).
Combined Genetic/Idiopathic Generalized Epilepsy
Stefan et al. studied a total of 7 patients with various idiopathic generalized or genetic confirmed generalized epilepsy (IGE/GGE) using beamformer. After analyzing spike-wave bursts in all patients and single spikes in 6 patients, source analysis showed most frequently involved regions were the left or right frontal (mainly mesial and bilateral frontal areas), peri-insular, and subcortical/thalamic areas. In addition, all patients had unilateral frontal accentuation of the activity. In three patients, two with JME and one with myoclonic absence epilepsy, sources were mainly present in the central and premotor regions (141). Thus, the authors concluded that in contrast to pure focal epilepsy, the distribution of the GSWD is not restricted to one hemisphere but a predominant region with additional oscillating connectivity within the thalamocortical network system.
Elshahabi et al. studied the resting-state connectivity of 13 patients with various types of IGE/GGE using beamformer and graph theoretical network analysis. Compared to normal controls, the patients with IGE/GGE had more pronounced motor network connectivity, mainly superior frontal gyrus, precentral, postcentral gyri, temporal cortex, and cerebellum. The authors also found significantly increased regional connectivity in the temporal lobe (superior and inferior temporal gyri) and insula (145). However, no conclusion could be made given that the study was performed on various IGE/GGE types and the limitation of the sub-cortical localization using a particular technique.
Stier et al. studied a total of 25 patients with GGE. Compared to normal healthy individuals, there was an increased functional connectivity at the multi-frequencies level in patients with GGE. Compared to normal controls, siblings without epilepsy also had significantly increased network connectivity, predominantly in beta frequencies. Compared to the healthy siblings of GGE, the increased beta connectivity patterns in GGE patients were less concordant, followed by functional connectivity in theta and delta frequency bands. Thus, the authors proposed that increased interictal MEG power and connectivity in frontocentral and temporo-parietal cortical regions were potential hallmarks of GGE (146). In addition, changes in these network characteristics were likely driven by the genetic factor and not by the disease process or medication effect (146).
Difference in Resting-State Functional Connnectivity Between Focal (Frontal) and Generalized Epilepsy
Using the fMRI connectivity analysis, it has been reported that patient with frontal lobe epilepsy has variable connectivity, either reduced or increased, various resting-state networks when compared to healthy pediatric and adult population (149–152). Still, there is limited literature investigating the resting-state fMRI functional connectivity comparing frontal lobe epilepsy with generalized epilepsy. A few publications on MEG resting-state functional connectivity in temporal lobe epilepsy are available, but data on frontal lobe epilepsy remains scarce. Herein, we would like to describe available neuromagnetic data in comparing the resting state connectivity between focal and generalized epilepsies.
Difference Between JME and Frontal Lobe Epilepsy
Niso et al. studied the resting-state functional connectivity of patients with frontal lobe epilepsy (FLE), generalized epilepsy (JME), and healthy individuals. Using power spectral analysis and graph theory assessed by phase synchronization measured with functional connectivity, the distribution of power and topographic changes (activation or deactivations) differed among all three groups. An increased total power indicated local synchronization. Those with JME had a higher total power for all frequencies except alpha band over a widespread set of sensors, whereas the FLE group showed higher relative power in the beta band bilaterally in the frontocentral sensors; i.e., regional specific around the epileptic focus. The authors found that functional networks from generalized epilepsy had greater efficiency and lower eccentricity than control subjects for higher frequency bands without a clear topography. Functional networks in FLE exhibited only reduced eccentricity over the frontotemporal and central sensors relative to the networks from controls (147). Thus, JME and FE groups represent a characteristic pattern of changes as compared to control.
Difference Between IGE/GGE and Frontal Lobe Epilepsy
Li Hegner et al. studied functional MEG connectivity using graph theory and coherency between focal and generalized epilepsy during resting state (with the absence of spikes or GSWDs) and found significant differences in network connectivity. Increased network connectivity was noted in bilateral mesio-frontal and motor regions in patients with IGE/GGE (148). Thus, the difference in the topography of resting-state functional connectivity in the mesio-frontal region in IGE/GGE may be a specific diagnostic biomarker.
Conclusion and Future Perspectives
In summary, with the advanced signal processing techniques combined with excellent temporal resolution properties of MEG, the cerebral neuromagnetic sources of GSWDs can be recorded and analyzed with millisecond resolution (153). The recording and post-processing associated with earlier MEG recording on GSWDs, especially using the SECD model, has several limitations, including deep brain structures, signal analysis of high-frequency oscillation, frequency-dependent network changes, etc. Later recordings using various advanced methodologies (various types of the beamformer, LORETA, pMEM, mathematical brain modeling, frequency coupling, etc.) advance our understanding not only of the potential pathophysiology of generalized epilepsy but also shed light on potential diagnostic, therapeutic and prognostic biomarkers of generalized epilepsy.
This review clearly illustrates the transition from focal neuromagnetic source analysis to network-based analysis using different frequency bandwidths involved in the generation, propagation and termination of the generalized spikes in various types of GGE. Earlier neuromagnetic analysis data focused on one particular brain structure, but recent literature points out that both cortical and subcortical structures are equally important in addition to the intact connectivity between various corticocortical and cortico-subcortical networks, with the leading initial epileptogenic hubs in the cortical region, mainly frontal lobe. Overall the current neuromagnetic data in GGE shows the important role of earlier cortical involvement, mainly frontal and parietal regions, before triggering the rapid synchronization of the subcortical and cortical networks, which goes along with the concept of cortical focus theory (22, 23, 25). The hypothesis mentioned above is ascertained by the current literature listed above in Tables 1–3.
Moreover, all published data suggests that generalized epilepsy has increased focal epileptogenic hubs, i.e., uneven cortical excitability in mainly frontal or central or parietal regions depending on the types of the GGE, with rapid recruitment via cortico-thalamic oscillation to various topographic locations, rather than the diffuse involvement of the whole brain. With the availability of directed connectivity analysis, the presence of focal hyper-connectivity in the setting of the global network has been demonstrated. As described above, one of the particular challenges in the clinical setting is accurately categorizing epileptic patients into either focal epilepsy or generalized epilepsy as both have different treatment options in terms of ASM and non–pharmacological treatment (131). In some particular cases, it is very challenging to give an accurate diagnosis. In addition, one doesn't want to miss the epilepsy surgery opportunity window in focal epileptic patients with rich connectivity, especially in the pediatric population, as the patient is misclassified as generalized epilepsy. In contrast, one doesn't want to undergo expensive pre-surgical epilepsy workups in patients with generalized epilepsy. At present, there is no scientifically proven diagnostic biomarker available for these types of challenging cases, but there are some promising findings by analyzing the neuromagnetic data. As illustrated above, during the resting state, connectivity patterns are different between healthy control, focal epilepsy, and GGE. In GGE, there is a presence of disorganization in the default mode network (GGE, JME, and AE), frontoparietal network (AE), and sensorimotor network (JME) during the resting state. In contrast, in focal frontal lobe epilepsy, there is only focal hyperconnectivity in the frontal lobe. Thus, the difference between resting MEG connectivity analyses can be a promising diagnostic biomarker to differentiate between focal and generalized epilepsy. One of the well known challenges of network analysis is that there is no one method superior to the others, and thus lack of standardized methodologies will be perplexing for future research. More investigations with increased subjects are also warranted to compare GE resting connectivity with other types of focal epilepsy with high connectivity, such as posterior quadrant epilepsy, for possible diagnostic and prognostic biomarkers in epilepsy.
In the default mode network, basic network node regions are responsible for basic incoming and outgoing information, remains activated when an individual is not engaged in external tasks, whereas the default state is suspended if the individual concentrates on a task (154). Compared with controls, effective connectivity at the posterior cingulate and parietal cortex, which are part of the default mode network, is decreased in patients with CAE, suggesting PC/PCC might be crucial for consciousness (8). In addition, the reduced resting functional connectivity in PCC/pC is also reported in patients with attention-deficit disorder (130) and memory impairment. Thus, given the above finding, patients with CAE have a higher chance of attention deficit disorder (131). In JME, in addition to other networks, there is an altered resting-state sensorimotor network, and hence it may be a reason for the seizure-prone (motor) states in the JME. Thus among different GGE subtypes, there are different networks involved. In epilepsy, quality of life is dependent not only on seizure frequency but also on the presence of co-morbidities, such as learning disability, anxiety, and ADHD (131). Without a doubt, understanding the basic pathophysiology of GGE will enlighten the clinicians with more therapeutic targets to improve the quality of life in patients with GGE.
There are promising preliminary neuromagnetic data on the prognostic biomarkers for drug resistance in patients with the CAE. CAE patients with the presence of the ictal HFOs (250–1,000 Hz), localized to the medial prefrontal cortex, are associated with increased seizure frequency (115, 116, 118). Both ETX and LTG non-responders have increased pretreatment ictal local frontal connectivity and decreased anteroposterior /frontoparietal connectivity compared to non-responders (47, 128, 129). Thus, by exploring the pretreatment ictal HFO and resting-state connectivity of CAE patients, one may be able to predict whether the patient will be an ASM responder or non-responder. However, at this time, no causal assumption can be made between the ASM non-responsiveness and the ictal frontal and decreased anteroposterior connectivity due to the limited data. Further studies are needed to confirm the hypothesis using a large cohort prospective study with a longer follow-up duration.
More and more data suggest that the alterations in the connectivity of various networks in patients with GGE are more complex and maybe even more dynamic with various multi-directionality. As mentioned above, Tenney et al. (47, 48) combined MEG with fMRI, which improved the source localization over the sub-regions of the deep brain area, such as different parts of the basal ganglia, and subregions of thalamus could be explored as the different parts of the basal ganglia and thalamus has different connectivity and functionality. In addition, the same research group already presented cross-frequency coupling showing how dynamic changes occurred in the various network in the CAE at the preictal stage (47, 48). Although fMRI has better spatial resolution than MEG, it is still insufficient to accurately localize the neuromagnetic source to subnuclei of the thalamus (46). In patients with drug-resistant GGE, one currently available alternative treatment option after failing the multiple ASMs is neuromodulation. The treatment outcome of neurostimulators, mainly DBS, is highly dependent upon the locations of the electrodes placement, stimulation parameters, subtypes of generalized epilepsy, or even individual cortical-subcortical connectivity profile (20, 21, 155). Thus, further studies using multimodality analysis combining various advanced postprocessing neuromagnetic analysis and neuroimaging may enlighten the underlying pathophysiology of underlying network alteration in various ictal or interictal stages of the patients with various types of IGG in order to improve the treatment options in generalized epilepsy,
Since absence epilepsy is the most common GGE, has frequent seizures, and reduced movement artifact, most of the current literature on GSWDs has experimented on patients with AE, mainly CAE. It is unclear whether research findings for CAE can be generalized to all the various subtypes of generalized epilepsy. Given all the literature mentioned above, different subtypes of generalized epilepsy may have shared mechanisms or connectivity pathways, but this review clearly illustrates varied topographic cortical involvements in different generalized epilepsy based on their symptomatology. Hence, further studies are warranted to confirm this point of view.
Last but not least, another major limitation is how one can confirm the findings of the current non–invasive neuromagnetic data to support the concept of cortical focus theory, in which a highly connective cortical epileptogenic focus, most likely frontal hyperexcitability and parietal deactivation, triggering the rapidly generalized epileptic discharges involving intact corticothalamic or corticocortical networks. The finding has been confirmed in the animal model, with the cortical focus activation being found to be leading the thalamus activation by 500 ms (156). Although the ideal confirmation of the concept in humans should be analyzing intracranial invasive electrical activities from simultaneous cortical regions, covering bilateral multi-lobar regions, and various subcortical regions, subnuclei of bilateral thalami, it will be unethical and impractical to put multi-electrodes to cover every aspect of the thalamus and cortical regions. So far, a small study of intraoperative simultaneous invasive centromedian thalamic nuclei and scalp EEG recording had shown that generalized paroxysmal fast activity in patients with the Lennox-Gastaut syndrome appeared 75 ms later in thalamic activation when compared to the scalp frontal EEG activity, supporting a cortical driven process in generalized epilepsy (157). Another study investigated the interval relationship of the centromedian thalamus in relation to the cortical electrical activities in two patients with idiopathic generalized epilepsy (158). One of the two patients had bilateral independent discharges restricted only to the bilateral centromedian thalami, and the other had bilateral cortical discharges with the belated onset of leading thalamic discharges at the ictal onset (158). Thus, based on their symptomatology, the currently available data suggested there were different topographic cortical involvements in different subtypes of generalized epilepsy. Given the small sample size, no particular conclusion could be made. However, the findings from the CAE may likely be unable to generalize to all the subtypes of generalized epilepsy. Hence, further studies are warranted for the emerging development of responsive neurostimulation therapies for patients with generalized epilepsy.
In conclusion, current MEG literature challenges the concept of generalized epilepsy being fully generalized. Advances in recent MEG methodology contribute to the literature of idiopathic/genetic generalized epilepsy in terms of physiopathology, treatment and prognosis options, thus further blurring the boundary between focal and generalized epilepsy.
Limitations
This review is limited because only three databases were searched by one reviewer (TA) and included only the published publication in English. All the posters publications were excluded. Thus, some of the remarkable pertinent studies might be missed in the literature review.
Author Contributions
TA contributed to the conception, performed literature review, drafting the manuscript, revising the manuscript, and final approval of the version to be published. JT and AB contributed to the conception, revising the manuscript, and final approval of the version to be published. All authors contributed to the article and approved the submitted version.
Conflict of Interest
The authors declare that the research was conducted in the absence of any commercial or financial relationships that could be construed as a potential conflict of interest.
Publisher's Note
All claims expressed in this article are solely those of the authors and do not necessarily represent those of their affiliated organizations, or those of the publisher, the editors and the reviewers. Any product that may be evaluated in this article, or claim that may be made by its manufacturer, is not guaranteed or endorsed by the publisher.
Abbreviations
GSWD, generalized spikes wave discharge; IEDs, Interictal epileptiform discharges; CAE, childhood absence epilepsy; JAE, juvenile absence epilepsy; JME, juvenile myoclonus epilepsy; GGE, genetic generalized epilepsy; TCS, tonic-clonic seizure; MEG, magnetoencephalography; EC, effective connectivity; EEG, electroencephalography; fMRI, functional magnetic resonance imaging; SAM, synthetic aperture magnetometry; LORETA, standardized low-resolution brain electromagnetic topography; sLORETA, standardized low-resolution brain electromagnetic topography; ms, milliseconds; LCMV, Linear constraint minimum variance; ASI, accumulated source imaging; DICS, Dynamic imaging of coherent sources; dMSI, Dynamic magnetic source imaging; dSPM, Dynamic statistical parameter mapping; ECD, equivalent dipole model; PLV, phase-locking value; MUSIC, multiple signal characteristic; wMNE, weighted minimum-norm estimation; pMEM, pairwise maximum entropy model; CFC, cross-frequency coupling; ms, milliseconds; s, seconds; m, months; y, years; F, female; M, male; ASM, antiseizure medication.
References
1. Zack MM, Kobau R. National and state estimates of the numbers of adults and children with active epilepsy—United States, 2015. MMWR Morbid Mortal Weekly Rep. (2017) 66:821–5. doi: 10.15585/mmwr.mm6631a1
2. Fisher RS, Cross JH, D'Souza C, French JA, Haut SR, Higurashi N, et al. Instruction manual for the ILAE 2017 operational classification of seizure types. Epilepsia. (2017) 58:531–42. doi: 10.1111/epi.13671
3. Gastaut H. Clinical and Electroencephalographical Classification of Epileptic Seizures. Epilepsia. (1970) 11:102–12. doi: 10.1111/j.1528-1157.1970.tb03871.x
4. Hirsch E, Andermann F, Chauvel P, Engel J, Silva FL da, Luders H. Generalized Seizures: from clinical phenomenology to underlying systems and networks. Schweizer Archiv für Neurologie und Psychiatrie. (2007) 7:346–346. doi: 10.4414/sanp.2007.01887
5. Tükel K, Jasper H. The electroencephalogram in parasagittal lesions. Electroencephalogr Clin Neurophysiol. (1952) 4:481–94. doi: 10.1016/0013-4694(52)90079-5
6. Bancaud J, Talairach J, Morel P, Bresson M, Bonis A, Geier S, et al. ‘Generalized' epileptic seizures elicited by electrical stimulation of the frontal lobe in man. Electroencephalogr Clin Neurophysiol. (1974) 37:275–82. doi: 10.1016/0013-4694(74)90031-5
7. Wyllie E, Lachhwani DK, Gupta A, Chirla A, Cosmo G, Worley S, et al. Successful surgery for epilepsy due to early brain lesions despite generalized EEG findings. Neurology. (2007) 69:389–97. doi: 10.1212/01.wnl.0000266386.55715.3f
8. Wu C, Xiang J, Jiang W, Huang S, Gao Y, Tang L, et al. Altered effective connectivity network in childhood absence epilepsy: a multi-frequency MEG study. Brain Topogr. (2017) 30:673–84. doi: 10.1007/s10548-017-0555-1
9. Kakisaka Y, Alexopoulos AV, Gupta A, Wang ZI, Mosher JC, Iwasaki M, et al. Generalized 3- Hz spike-and-wave complexes emanating from focal epileptic activity in pediatric patients. Epilepsy Behav. (2011) 20:103–6. doi: 10.1016/j.yebeh.2010.10.025
10. Kim DW, Lee SY, Lee SK. Focal epileptogenic lesions in adult patients with epilepsy and generalized epileptiform discharges. J Epilepsy Res. (2016) 6:75–8. doi: 10.14581/jer.16014
11. Seneviratne U, Cook M, D'Souza W. Focal abnormalities in idiopathic generalized epilepsy: A critical review of the literature. Epilepsia. (2014) 55:1157–69. doi: 10.1111/epi.12688
12. Chauvel P. “Contributions of Jean Talairach and Jean Bancaud to epilepsy surgery,” in: U. Luders, Hans (Department of neurology, The Cleveland Clinic Foundation, Cleveland, Ohio and Y.G. Comair (eds) Epilepsy Surgery (2nd Edition). Philadelphia: Lippincott Williams and Wilkins. pp. 35–41. (2001). Available online at: http://books.google.com/books?hl=enandlr=andid=2x5irzPCsjwCandoi=fndandpg=PA35anddq=CONTRIBUTIONSs+OF+JEAN+TALAIRACH+AND+JEAN+BANCAUD+TO+EPILEPSY+SURGERYandots=5HllPum-Khandsig=lunHohdyMtxLqkvvM0h_W9WR1SE
13. Rosenow F, Luders H. Presurgical evaluation of epilepsy. J Pediatric Neurosci. (2008) 3:74. doi: 10.4103/1817-1745.40593
14. Kwan P, Arzimanoglou A, Berg AT, Brodie MJ, Hauser WA, Mathern G, et al. Definition of drug resistant epilepsy: consensus proposal by the ad hoc task force of the ILAE commission on therapeutic strategies. Epilepsia. (2010) 51:1069–77. doi: 10.1111/j.1528-1167.2009.02397.x
15. Chauvel P, Gonzalez-Martinez JA, Bulacio JC. Presurgical intracranial investigations in epilepsy surgery. Handbook Clinic Neurol. (2019) 19:45–71. doi: 10.1016/B978-0-444-64142-7.00040-0
16. Chauvel P. “The epileptogenic zone: a critical reconstruction,” in A Practical Approach to Stereo EEG, ed S.U. Schuele (1st ed). (New York: Springer Publishing Company) (2020). p. 105–117.
17. Englot DJ, Rolston JD, Wright CW, Hassnain KH, Chang EF. Rates and predictors of seizure freedom with vagus nerve stimulation for intractable epilepsy. Neurosurgery. (2016) 79:345–53. doi: 10.1227/NEU.0000000000001165
18. Morrell MJ, Halpern C. Responsive Direct Brain Stimulation for Epilepsy. Neurosurg Clin N Am. (2016) 27:111–21. doi: 10.1016/j.nec.2015.08.012
19. Fisher R, Salanova V, Witt T, Worth R, Henry T, Gross R, et al. Electrical stimulation of the anterior nucleus of thalamus for treatment of refractory epilepsy. Epilepsia. (2010) 51:899–908. doi: 10.1111/j.1528-1167.2010.02536.x
20. Starnes K, Miller K, Wong-Kisiel L, Lundstrom BN. A review of neurostimulation for epilepsy in pediatrics. Brain Sci. (2019) 9:283. doi: 10.3390/brainsci9100283
21. Torres Diaz CV, González-Escamilla G, Ciolac D, Navas García M, Pulido Rivas P, Sola RG, et al. Network Substrates of Centromedian Nucleus Deep Brain Stimulation in Generalized Pharmacoresistant Epilepsy. Neurotherapeutics. (2021) 18:1665–77. doi: 10.1007/s13311-021-01057-y
22. Meeren H, Van Luijtelaar G, Lopes Da Silva F, Coenen A. Evolving concepts on the pathophysiology of absence seizures: The cortical focus theory. Arch Neurol. (2005) 62:371–6. doi: 10.1001/archneur.62.3.371
23. Avoli M. A brief history on the oscillating roles of thalamus and cortex in absence seizures. Epilepsia. (2012) 53:779–89. doi: 10.1111/j.1528-1167.2012.03421.x
24. Chen PC, Castillo EM, Baumgartner J, Seo JH, Korostenskaja M, Lee KH. Identification of focal epileptogenic networks in generalized epilepsy using brain functional connectivity analysis of bilateral intracranial EEG signals. Brain Topogr. (2016) 29:728–37. doi: 10.1007/s10548-016-0493-3
25. Stefan H, Rampp S. Aktuelle klinisch-neurophysiologische befunde bei absence-epilepsien. Nervenarzt. (2009) 80:378–85. doi: 10.1007/s00115-008-2636-6
26. Hämäläinen M, Hari R, Ilmoniemi RJ, Knuutila J, Lounasmaa OV. Magnetoencephalography theory, instrumentation, and applications to non-invasive studies of the working human brain. Rev Mod Phys. (1993) 65:413–97. doi: 10.1103/RevModPhys.65.413
27. Ebersole JS. New applications of EEG/MEG in epilepsy evaluation. Epilepsy research Supplement. (1996) 11:227–37.
28. Cohen D, Halgren E. Magnetoencephalography (Neuromagnetism). Encyclopedia of Neuroscience. (2009) 9:615–22. doi: 10.1016/B978-008045046-9.02021-0
29. Knowlton RC, Shih J. Magnetoencephalography in epilepsy. Epilepsia. (2004) 45:61–71. doi: 10.1111/j.0013-9580.2004.04012.x
30. de Munck JC, van Dijk BW, Spekreijse H. Mathematical Dipoles are Adequate to Describe Realistic Generators of Human Brain Activity. IEEE Transact Biomed Eng. (1988) 35:960–6. doi: 10.1109/10.8677
31. Ebersole JS, Ebersole SM. Combining MEG and EEG source modeling in epilepsy evaluations. J Clinic Neurophysiol. (2010) 27:360–71. doi: 10.1097/WNP.0b013e318201ffc4
32. Bagić AI, Knowlton RC, Rose DF, Ebersole JS. American clinical magnetoencephalography society clinical practice guideline 1: recording and analysis of spontaneous cerebral activity. J Clinic Neurophysiol. (2011) 28:348–54. doi: 10.1097/WNP.0b013e3182272fed
33. Mosher JC, Spencer ME, Leahy RM, Lewis PS. Error bounds for EEG and MEG dipole source localization. Electroencephalogr Clin Neurophysiol. (1993) 86:303–21. doi: 10.1016/0013-4694(93)90043-U
34. Mosher JC, Baillet S, Leahy RM. EEG source localization and imaging using multiple signal classification approaches. J Clinic Neurophysiol. (1999) 16:225–38. doi: 10.1097/00004691-199905000-00004
35. Tenney JR, Fujiwara H, Rose DF. The value of source localization for clinical magnetoencephalography: beyond the equivalent current dipole. J Clinic Neurophysiol Offic Publicat Am Electroencephalograph Soc. (2020) 37:537–44. doi: 10.1097/WNP.0000000000000487
36. Huang MX, Mosher JC, Leahy RM. A sensor-weighted overlapping-sphere head model and exhaustive head model comparison for MEG. Phys Med Biol. (1999) 44:423–40. doi: 10.1088/0031-9155/44/2/010
37. Mosher JC, Leahy RM, Lewis PS. EEG and MEG: Forward solutions for inverse methods. IEEE Transact Biomed Eng. (1999) 46:245–59. doi: 10.1109/10.748978
38. Mosher JC, Baillet S, Leahy RM. Equivalence of linear approaches in bioelectromagnetic inverse solutions. IEEE Workshop Statistic Sign Process Proceed. (2003) 2003:294–297. doi: 10.1109/SSP.2003.1289402
39. Jerbi K, Mosher JC, Baillet S, Leahy RM. On MEG forward modelling using multipolar expansions. Phys Med Biol. (2002) 47:523–55. doi: 10.1088/0031-9155/47/4/301
40. Hämäläinen M, Huang M, Bowyer SM. Magnetoencephalography signal processing, forward modeling, magnetoencephalography inverse source imaging, and coherence analysis. Neuroimag Clinics North Am. (2020) 20:125–43. doi: 10.1016/j.nic.2020.02.001
41. Attal Y, Schwartz D. Assessment of subcortical source localization using deep brain activity imaging model with minimum norm operators: a MEG study. PLoS ONE. (2013) 8:e59856. doi: 10.1371/journal.pone.0059856
42. Puce A, Hämäläinen MS. A review of issues related to data acquisition and analysis in EEG/MEG studies. Brain Sci. (2017) 7:58. doi: 10.3390/brainsci7060058
43. Stefan H, Trinka E. Magnetoencephalography (MEG): Past, current and future perspectives for improved differentiation and treatment of epilepsies. Seizure. (2017) 44:121–4. doi: 10.1016/j.seizure.2016.10.028
44. Bénar CG, Velmurugan J, López-Madrona VJ, Pizzo F, Badier JM. Detection and localization of deep sources in magnetoencephalography: a review. Curr Opin Biomed Eng. (2021) 18:285. doi: 10.1016/j.cobme.2021.100285
45. Boto E, Holmes N, Leggett J, Roberts G, Shah V, Meyer SS, et al. Moving magnetoencephalography towards real-world applications with a wearable system. Nature. (2018) 555:657–61. doi: 10.1038/nature26147
46. Logothetis NK, Pauls J, Augath M, Trinath T, Oeltermann A. Neurophysiological investigation of the basis of the fMRI signal. Nature. (2001) 412:150–7. doi: 10.1038/35084005
47. Tenney JR, Kadis DS, Agler W, Rozhkov L, Altaye M, Xiang J, et al. Ictal connectivity in childhood absence epilepsy: Associations with outcome. Epilepsia. (2018) 59:971–81. doi: 10.1111/epi.14067
48. Tenney JR, Williamson BJ, Kadis DS. Cross-frequency coupling in childhood absence epilepsy. Brain Connect. (2021). doi: 10.1089/brain.2021.0119. [Epub ahead of print].
49. Cohen D. Magnetoencephalography: Evidence of magnetic fields produced by alpha-rhythm currents. Science. (1968) 161:784–6. doi: 10.1126/science.161.3843.784
50. Laohathai C, Ebersole JS, Mosher JC, Bagić AI, Sumida A, Von Allmen G, et al. Practical fundamentals of clinical MEG interpretation in epilepsy. Front Neurol. (2021) 12:986. doi: 10.3389/fneur.2021.722986
51. Schoffelen JM, Gross J. Source connectivity analysis with MEG and EEG. Hum Brain Mapp. (2009) 30:1857–65. doi: 10.1002/hbm.20745
52. Tenney JR, Fujiwara H, Horn PS, Rose DF. Comparison of magnetic source estimation to intracranial EEG, resection area, and seizure outcome. Epilepsia. (2014) 55:1854–63. doi: 10.1111/epi.12822
53. Duez L, Tankisi H, Hansen PO, Sidenius P, Sabers A, Pinborg LH, et al. Electromagnetic source imaging in presurgical workup of patients with epilepsy: a prospective study. Neurology. (2019) 92:e576–86. doi: 10.1212/WNL.0000000000006877
54. Pellegrino G, Hedrich T, Porras-Bettancourt M, Lina JM, Aydin Ü, Hall J, et al. Accuracy and spatial properties of distributed magnetic source imaging techniques in the investigation of focal epilepsy patients. Hum Brain Mapp. (2020) 41:3019–33. doi: 10.1002/hbm.24994
55. Leahy RM, Mosher JC, Spencer ME, Huang MX, Lewine JD. A study of dipole localization accuracy for MEG and EEG using a human skull phantom. Electroencephalogr Clin Neurophysiol. (1998) 107:159–73. doi: 10.1016/S0013-4694(98)00057-1
56. Hämäläinen MS. Magnetoencephalography: A tool for functional brain imaging. Brain Topogr. (1992) 5:95–102. doi: 10.1007/BF01129036
57. Mosher JC, Lewis PS, Leahy RM. Multiple dipole modeling and localization from spatio-temporal MEG Data. IEEE Transact Biomed Eng. (1992) 39:541–57. doi: 10.1109/10.141192
58. Mosher JC, Leahy RM. Recursive MUSIC: A framework for EEG and MEG source localization. IEEE Transact Biomed Eng. (1998) 45:1342–54. doi: 10.1109/10.725331
59. Van Veen BD, Buckley KM. Beamforming: a versatile approach to spatial filtering. IEEE ASSP Magazine. (1988) 5:4–24. doi: 10.1109/53.665
60. Spencer ME, Leahy RM, Mosher JC, Lewis PS. “Adaptive filters for monitoring localized brain activity from surface potential time series,” In: Conference Record—Asilomar Conference on Signals, Systems and Computers. (1992), p. 156–161.
61. Robinson SE, Vrba J. Functional neuroimaging by synthetic aperture magnetometry (SAM). Recent Advances in Biomagnetism. (1999).
62. Gross J, Kujala J, Hämäläinen M, Timmermann L, Schnitzler A, Salmelin R. Dynamic imaging of coherent sources: Studying neural interactions in the human brain. Proc Natl Acad Sci U S A. (2001) 98:694–9. doi: 10.1073/pnas.98.2.694
63. Xiang J, Luo Q, Kotecha R, Korman A, Zhang F, Luo H, et al. Accumulated source imaging of brain activity with both low and high-frequency neuromagnetic signals. Front Neuroinform. (2014) 8:57. doi: 10.3389/fninf.2014.00057
64. Hämäläinen MS, Ilmoniemi RJ. Interpreting magnetic fields of the brain: minimum norm estimates. Medical Biologic Eng Comput. (1994) 32:35–42. doi: 10.1007/BF02512476
65. Pascual-Marqui RD, Michel CM, Lehmann D. Low resolution electromagnetic tomography: a new method for localizing electrical activity in the brain. Int J Psychophysiol. (1994) 18:49–65. doi: 10.1016/0167-8760(84)90014-X
66. Pascual-Marqui RD. Low resolution brain electromagnetic tomography (LORETA). J Neurother. (2001) 4:31–3. doi: 10.1300/J184v04n04_05
67. Wagner M, Fuchs M, Kastner J. Evaluation of sLORETA in the presence of noise and multiple sources. Brain Topogr. (2004) 16:277–80. doi: 10.1023/B:BRAT.0000032865.58382.62
68. Wang JZ, Williamson SJ, Kaufman L. Magnetic source imaging based on the minimum-norm least-squares inverse. Brain Topogr. (1993) 5:365–71. doi: 10.1007/BF01128692
69. Lin FH, Witzel T, Ahlfors SP, Stufflebeam SM, Belliveau JW, Hämäläinen MS. Assessing and improving the spatial accuracy in MEG source localization by depth-weighted minimum-norm estimates. Neuroimage. (2006) 31:160–71. doi: 10.1016/j.neuroimage.2005.11.054
70. Dale AM, Sereno MI. Improved localization of cortical activity by combining EEG and MEG with MRI cortical surface reconstruction: a linear approach. J Cogn Neurosci. (1993) 5:162–76. doi: 10.1162/jocn.1993.5.2.162
71. Dale AM, Liu AK, Fischl BR, Buckner RL, Belliveau JW, Lewine JD, et al. Dynamic statistical parametric mapping: combining fMRI and MEG for high-resolution imaging of cortical activity. Neuron. (2000) 26:55–67. doi: 10.1016/S0896-6273(00)81138-1
72. Hauk O, Wakeman DG, Henson R. Comparison of noise-normalized minimum norm estimates for MEG analysis using multiple resolution metrics. Neuroimage. (2011) 54:1966–74. doi: 10.1016/j.neuroimage.2010.09.053
73. Gorodnitsky IF, George JS, Rao BD. Neuromagnetic source imaging with FOCUSS: a recursive weighted minimum norm algorithm. Electroencephalogr Clin Neurophysiol. (1995) 95:231–51. doi: 10.1016/0013-4694(95)00107-A
74. Clarke CJS, Janday BS. The solution of the biomagnetic inverse problem by maximum statistical entropy. Inverse Probl. (1989) 5:483–500. doi: 10.1088/0266-5611/5/4/005
75. Cirillo ENM, Lebowitz JL. Metastability in the two-dimensional Ising model with free boundary conditions. J Stat Phys. (1998) 90:211–26. doi: 10.1023/A:1023255802455
76. Yeh FC, Tang A, Hobbs JP, Hottowy P, Dabrowski W, Sher A, et al. Maximum entropy approaches to living neural networks. Entropy. (2010) 12:89–106. doi: 10.3390/e12010089
77. Amblard C, Lapalme E, Lina JM. Biomagnetic source detection by maximum entropy and graphical models. IEEE Transact Biomed Eng. (2004) 51:427–42. doi: 10.1109/TBME.2003.820999
78. Mesulam MM. From sensation to cognition. Brain. (1998) 121:1013–52. doi: 10.1093/brain/121.6.1013
79. Fries P. Rhythms for cognition: communication through coherence. Neuron. (2015) 88:220–35. doi: 10.1016/j.neuron.2015.09.034
80. Cetin MS, Houck JM, Rashid B, Agacoglu O, Stephen JM, Sui J, et al. Multimodal classification of schizophrenia patients with MEG and fMRI data using static and dynamic connectivity measures. Front Neurosci. (2016) 10:466. doi: 10.3389/fnins.2016.00466
81. Friston KJ. Functional and effective connectivity in neuroimaging: a synthesis. Hum Brain Mapp. (1994) 2:56–78. doi: 10.1002/hbm.460020107
82. Granger CWJ. Investigating Causal Relations by Econometric Models and Cross-spectral Methods. Econometrica. (1969) 37:424. doi: 10.2307/1912791
83. Geweke J. Measurement of linear dependence and feedback between multiple time series. J Am Stat Assoc. (1982) 77:304–13. doi: 10.1080/01621459.1982.10477803
84. Basser PJ, Mattiello J, LeBihan D. MR diffusion tensor spectroscopy and imaging. Biophys J. (1994) 66:259–67. doi: 10.1016/S0006-3495(94)80775-1
85. He B, Yang L, Wilke C, Yuan H. Electrophysiological imaging of brain activity and connectivity-challenges and opportunities. IEEE Transact Biomed Eng. (2011) 58:1918–31. doi: 10.1109/TBME.2011.2139210
86. He B, Sohrabpour A, Brown E, Liu Z. Electrophysiological source imaging: a non-invasive window to brain dynamics. Annu Rev Biomed Eng. (2018) 20:171–96. doi: 10.1146/annurev-bioeng-062117-120853
87. Friston KJ. Functional and effective connectivity: a review. Brain Connect. (2011) 1:13–36. doi: 10.1089/brain.2011.0008
88. Lehnertz K. Assessing directed interactions from neurophysiological signals—An overview. Physiol Meas. (2011) 32:1715–24. doi: 10.1088/0967-3334/32/11/R01
89. Friston K, Moran R, Seth AK. Analysing connectivity with Granger causality and dynamic causal modelling. Curr Opin Neurobiol. (2013) 23:172–8. doi: 10.1016/j.conb.2012.11.010
90. Nolte G, Bai O, Wheaton L, Mari Z, Vorbach S, Hallett M. Identifying true brain interaction from EEG data using the imaginary part of coherency. Clinic Neurophysiol. (2004) 115:2292–307. doi: 10.1016/j.clinph.2004.04.029
91. Nolte G, Müller KR. Localizing and estimating causal relations of interacting brain rhythms. Front Hum Neurosci. (2010) 4:209. doi: 10.3389/fnhum.2010.00209
92. Garcés P, Martín-Buro MC, Maestú F. Quantifying the test-retest reliability of magnetoencephalography resting-state functional connectivity. Brain Connectiv. (2016) 6:448–60. doi: 10.1089/brain.2015.0416
93. Baker GT. Purification and some properties of ATP:Arginine phosphotransferase from sea anemones, Condylactis aurantiaca. Comparativ Biochemistr Physiol Part B: Biochemistr. (1975) 52:503–6. doi: 10.1016/0305-0491(75)90225-4
94. Patel RS, Bowman FDB, Rilling JK. A Bayesian approach to determining connectivity of the human brain. Hum Brain Mapp. (2006) 27:267–76. doi: 10.1002/hbm.20182
95. Nummenmaa A, Auranen T, Hämäläinen MS, Jääskeläinen IP, Lampinen J, Sams M, et al. Hierarchical Bayesian estimates of distributed MEG sources: Theoretical aspects and comparison of variational and MCMC methods. Neuroimage. (2007) 35:669–85. doi: 10.1016/j.neuroimage.2006.05.001
96. López JD, Litvak V, Espinosa JJ, Friston K, Barnes GR. Algorithmic procedures for Bayesian MEG/EEG source reconstruction in SPM. Neuroimage. (2014) 84:476–87. doi: 10.1016/j.neuroimage.2013.09.002
97. Mumford JA, Ramsey JD. Bayesian networks for fMRI: a primer. Neuroimage. (2014) 86:573–82. doi: 10.1016/j.neuroimage.2013.10.020
98. Friston KJ, Preller KH, Mathys C, Cagnan H, Heinzle J, Razi A, et al. Dynamic causal modelling revisited. Neuroimage. (2019) 199:730–44. doi: 10.1016/j.neuroimage.2017.02.045
99. Angluin D, Aspnes J, Kontorovich A. On the learnability of shuffle ideals. Lect Notes Comput Sci. (2012) 7568:111–23. doi: 10.1007/978-3-642-34106-9_12
100. Stephan KE, Friston KJ. Analyzing effective connectivity with functional magnetic resonance imaging. Wiley Interdisciplin Rev Cogn Sci. (2010) 1:446–59. doi: 10.1002/wcs.58
101. Stam CJ. Functional connectivity patterns of human magnetoencephalographic recordings: a ‘small-world' network? Neurosci Lett. (2004) 355:25–8. doi: 10.1016/j.neulet.2003.10.063
102. Sporns O, Tononi G, Kötter R. The human connectome: A structural description of the human brain. PLoS Comput Biol. (2005) 1:0245–51. doi: 10.1371/journal.pcbi.0010042
103. Bassett DS, Bullmore E. Small-world brain networks. Neuroscientist. (2006) 12:512–23. doi: 10.1177/1073858406293182
104. Bullmore E, Sporns O. Complex brain networks: Graph theoretical analysis of structural and functional systems. Nat Rev Neurosci. (2009) 10:186–98. doi: 10.1038/nrn2575
105. Leskovec J, Horvitz E. Planetary-scale views on a large instant-messaging network. Proceed Int Conferen World Wide Web. (2008) 8:915–924. doi: 10.1145/1367497.1367620
106. Hughes JR, Cohen J, Mayman CI, Scholl ML, Hendrix DE. Relationship of the magnetoencephalogram to abnormal activity in the electroencephalogram. J Neurol. (1977) 217:79–93. doi: 10.1007/BF00312921
107. Ricci GB, Erne' SN, Del Gratta C, Peresson M, Pizzella V, Romani GL, et al. Template analysis on interictal neuromagnetic data from cases of focal and generalized epilepsy. Adv Biomagnetism. (1989) 287–290. doi: 10.1007/978-1-4613-0581-1_58
108. Ricci GB, Chapman RM, Erné SN, Narici L, Peresson M, Pizzella V, et al. Neuromagnetic topography of photoconvulsive response in man. Electroencephalograph Clinic Neurophysiol. (1990) 75:1–12. doi: 10.1016/0013-4694(90)90147-C
109. Ricci GB. The MEG in evaluation of generalized epilepsy. Physiol Measure. (1993) 14:A103–8. doi: 10.1088/0967-3334/14/4a/019
110. Westmijse I, Ossenblok P, Gunning B, van Luijtelaar G. Onset and propagation of spike and slow wave discharges in human absence epilepsy: a MEG study. Epilepsia. (2009) 50:2538–48. doi: 10.1111/j.1528-1167.2009.02162.x
111. Hu X, Dong J, Wang X, Wu T, Yang L, Lu X. Localization of epileptic foci in Children with childhood absence epilepsy by magnetoencephalography combined with synthetic aperture magnetometry. J Biomed Res. (2011) 25:259–65. doi: 10.1016/S1674-8301(11)60035-3
112. Tenney JR, Fujiwara H, Horn PS, Jacobson SE, Glauser TA, Rose DF. Focal corticothalamic sources during generalized absence seizures: a MEG study. Epilepsy Res. (2013) 106:113–22. doi: 10.1016/j.eplepsyres.2013.05.006
113. Tenney JR, Fujiwara H, Horn PS, Vannest J, Xiang J, Glauser TA, et al. Low- and high-frequency oscillations reveal distinct absence seizure networks. Ann Neurol. (2014) 76:558–67. doi: 10.1002/ana.24231
114. Jacobs-Brichford E, Horn PS, Tenney JR. Mapping preictal networks preceding childhood absence seizures using magnetoencephalography. J Child Neurol. (2014) 29:1312–9. doi: 10.1177/0883073813518107
115. Miao A, Tang L, Xiang J, Guan Q, Ge H, Liu H, et al. Dynamic magnetic source imaging of absence seizure initialization and propagation: A magnetoencephalography study. Epilepsy Res. (2014) 108:468–80. doi: 10.1016/j.eplepsyres.2014.01.006
116. Miao A, Xiang J, Tang L, Ge H, Liu H, Wu T, et al. Using ictal high-frequency oscillations (80-500 Hz) to localize seizure onset zones in childhood absence epilepsy: A MEG study. Neurosci Lett. (2014) 566:21–6. doi: 10.1016/j.neulet.2014.02.038
117. Xiang J, Tenney JR, Korman AM, Leiken K, Rose DF, Harris E, et al. Quantification of interictal neuromagnetic activity in absence epilepsy with accumulated source imaging. Brain Topogr. (2015) 28:904–14. doi: 10.1007/s10548-014-0411-5
118. Tang L, Xiang J, Huang S, Miao A, Ge H, Liu H, et al. Neuromagnetic high-frequency oscillations correlate with seizure severity in absence epilepsy. Clinic Neurophysioly. (2016) 127:1120–9. doi: 10.1016/j.clinph.2015.08.016
119. Gupta D, Ossenblok P, Van Luijtelaar G. Space-time network connectivity and cortical activations preceding spike wave discharges in human absence epilepsy: a MEG study. Med Biologic Eng Comput. (2011) 49:555–65. doi: 10.1007/s11517-011-0778-3
120. Wu C, Sun J, Huang S, Tang L, Miao A, Zhou Y, et al. Quantify neuromagnetic network changes from pre-ictal to ictal activities in absence seizures. Neuroscience. (2017) 357:134–44. doi: 10.1016/j.neuroscience.2017.05.038
121. Youssofzadeh V, Agler W, Tenney JR, Kadis DS. Whole-brain MEG connectivity-based analyses reveals critical hubs in childhood absence epilepsy. Epilepsy Res. (2018) 145:102–9. doi: 10.1016/j.eplepsyres.2018.06.001
122. Jiang W, Wu C, Xiang J, Miao A, Qiu W, Tang L, et al. Dynamic neuromagnetic network changes of seizure termination in absence epilepsy: a magnetoencephalography study. Front Neurol. (2019) 10. doi: 10.3389/fneur.2019.00703
123. Sun Y, Li Y, Shi Q, Wu C, Sun J, Chen Q, et al. Changes of ictal-onset epileptic network synchronicity in childhood absence epilepsy: a magnetoencephalography study. Front Neurol. (2020) 11. doi: 10.3389/fneur.2020.583267
124. Sun J, Gao Y, Miao A, Yu C, Tang L, Huang S, et al. Multifrequency dynamics of cortical neuromagnetic activity underlying seizure termination in absence epilepsy. Front Hum Neurosci. (2020) 14:221. doi: 10.3389/fnhum.2020.00221
125. Chavez M, Valencia M, Navarro V, Latora V, Martinerie J. Functional modularity of background activities in normal and epileptic brain networks. Phys Rev Lett. (2010) 104:118701. doi: 10.1103/physrevlett.104.118701
126. Shi Q, Zhang T, Miao A, Sun J, Sun Y, Chen Q, et al. Differences Between Interictal and Ictal Generalized Spike-Wave Discharges in Childhood Absence Epilepsy: A MEG Study. Front Neurol. (2020) 10. doi: 10.3389/fneur.2019.01359
127. Sun Y, Li Y, Sun J, Zhang K, Tang L, Wu C, et al. Functional reorganization of brain regions into a network in childhood absence epilepsy: a magnetoencephalography study. Epilepsy Behav. (2021) 122:108–17. doi: 10.1016/j.yebeh.2021.108117
128. Miao A, Wang Y, Xiang J, Liu Q, Chen Q, Qiu W, et al. Ictal source locations and cortico–thalamic connectivity in childhood absence epilepsy: associations with treatment response. Brain Topogr. (2019) 32:178–91. doi: 10.1007/s10548-018-0680-5
129. Zhang K, Sun J, Sun Y, Niu K, Wang P, Wu C, et al. Pretreatment source location and functional connectivity network correlated with therapy response in childhood absence epilepsy: a magnetoencephalography study. Front Neurol. (2021) 12:24. doi: 10.3389/fneur.2021.692126
130. Leech R, Sharp DJ. The role of the posterior cingulate cortex in cognition and disease. Brain. (2014) 137:12–32. doi: 10.1093/brain/awt162
131. Caplan R, Siddarth P, Stahl L, Lanphier E, Vona P, Gurbani S, et al. Childhood absence epilepsy: behavioral, cognitive, and linguistic comorbidities. Epilepsia. (2008) 49:1838–46. doi: 10.1111/j.1528-1167.2008.01680.x
132. Guerrini R, Marini C, Barba C. Generalized epilepsies. Handbook Clinic Neurol. (2019) 19:3–15. doi: 10.1016/B978-0-444-64142-7.00038-2
133. Amor F, Baillet S, Navarro V, Adam C, Martinerie J, Le Van Quyen M. Cortical local and long-range synchronization interplay in human absence seizure initiation. Neuroimage. (2009) 45:950–62. doi: 10.1016/j.neuroimage.2008.12.011
134. Sakurai K, Takeda Y, Tanaka N, Kurita T, Shiraishi H, Takeuchi F, et al. Generalized spike-wave discharges involve a default mode network in patients with juvenile absence epilepsy: A MEG study. Epilepsy Res. (2010) 89:176–84. doi: 10.1016/j.eplepsyres.2009.12.004
135. Rozendaal YJW, van Luijtelaar G, Ossenblok PPW. Spatiotemporal mapping of interictal epileptiform discharges in human absence epilepsy: a MEG study. Epilepsy Res. (2016) 119:67–76. doi: 10.1016/j.eplepsyres.2015.11.013
136. Gadad V, Sinha S, Mariyappa N, Velmurugan J, Chaitanya G, Saini J, et al. Source analysis of epileptiform discharges in absence epilepsy using Magnetoencephalography (MEG). Epilepsy Res. (2018) 140:46–52. doi: 10.1016/j.eplepsyres.2017.12.003
137. Kotini A, Mavraki E, Anninos P, Piperidou H, Prassopoulos P. Magnetoencephalographic findings in two cases of juvenile myoclonus epilepsy. Brain Topogr. (2010) 23:41–5. doi: 10.1007/s10548-009-0114-5
138. Gadad V, Sinha S, Mariyappa N, Chaithanya G, Jayabal V, Saini J, et al. Source localization of epileptiform discharges in juvenile myoclonic epilepsy (JME) using magnetoencephalography (MEG). Epilepsy Res. (2017) 129:67–73. doi: 10.1016/j.eplepsyres.2016.11.019
139. Hamandi K, Singh KD, Muthukumaraswamy S. Reduced movement-related beta desynchronisation in juvenile myoclonic epilepsy: a MEG study of task specific cortical modulation. Clinic Neurophysiol. (2011) 122:2128–38. doi: 10.1016/j.clinph.2011.04.017
140. de León SC G, Niso G, Canuet L, Burriel-Lobo L, Maestú F, Rodríguez-Magariños MG. Praxis-induced seizures in a patient with juvenile myoclonic epilepsy: MEG-EEG coregistration study. Epilepsy Behav Case Rep. (2016) 5:1–5. doi: 10.1016/j.ebcr.2015.10.002
141. Stefan H, Paulini-Ruf A, Hopfengärtner R, Rampp S. Network characteristics of idiopathic generalized epilepsies in combined MEG/EEG. Epilepsy Res. (2009) 85:187–98. doi: 10.1016/j.eplepsyres.2009.03.015
142. Routley B, Shaw A, Muthukumaraswamy SD, Singh KD, Hamandi K. Juvenile myoclonic epilepsy shows increased posterior theta, and reduced sensorimotor beta resting connectivity. Epilepsy Res. (2020) 163:234. doi: 10.1016/j.eplepsyres.2020.106324
143. Krzemiński D, Masuda N, Hamandi K, Singh KD, Routley B, Zhang J. Energy landscape of resting magnetoencephalography reveals fronto-parietal network impairments in epilepsy. Network Neurosci. (2019) 4:374–96. doi: 10.1162/netn_a_00125
144. Lopes MA, Krzemiński D, Hamandi K, Singh KD, Masuda N, Terry JR, et al. A computational biomarker of juvenile myoclonic epilepsy from resting-state MEG. Clinic Neurophysiol. (2021) 132:922–7. doi: 10.1016/j.clinph.2020.12.021
145. Elshahabi A, Klamer S, Sahib AK, Lerche H, Braun C, Focke NK. Magnetoencephalography reveals a widespread increase in network connectivity in idiopathic/genetic generalized epilepsy. PLoS ONE. (2015) 10:e0138119. doi: 10.1371/journal.pone.0138119
146. Stier C, Elshahabi A, Li Hegner Y, Kotikalapudi R, Marquetand J, Braun C, et al. Heritability of magnetoencephalography phenotypes among patients with genetic generalized epilepsy and their siblings. Neurology. (2021) 97:166–77. doi: 10.1212/WNL.0000000000012144
147. Niso G, Carrasco S, Gudín M, Maestú F, Del-Pozo F, Pereda E. What graph theory actually tells us about resting state interictal MEG epileptic activity. NeuroImage: Clinic. (2015) 8:503–15. doi: 10.1016/j.nicl.2015.05.008
148. Li Hegner Y, Marquetand J, Elshahabi A, Klamer S, Lerche H, Braun C, et al. Increased functional MEG connectivity as a hallmark of mri-negative focal and generalized epilepsy. Brain Topogr. (2018) 31:863–74. doi: 10.1007/s10548-018-0649-4
149. Braakman HMH, Vaessen MJ, Jansen JFA, Debeij-Van Hall MHJA, De Louw A, Hofman PAM, et al. Frontal lobe connectivity and cognitive impairment in pediatric frontal lobe epilepsy. Epilepsia. (2013) 54:446–54. doi: 10.1111/epi.12044
150. Widjaja E, Zamyadi M, Raybaud C, Snead OC, Smith ML. Abnormal functional network connectivity among resting-state networks in children with frontal lobe epilepsy. Am J Neuroradiol. (2013) 34:2386–92. doi: 10.3174/ajnr.A3608
151. Cao X, Qian Z, Xu Q, Shen J, Zhang Z, Lu G. Altered intrinsic connectivity networks in frontal lobe epilepsy: a resting-state fMRI study. Comput Math Methods Med. (2014) 2014:864979. doi: 10.1155/2014/864979
152. Klugah-Brown B, Luo C, Peng R, He H, Li J, Dong L, et al. Altered structural and causal connectivity in frontal lobe epilepsy. BMC Neurol. (2019) 19:130. doi: 10.1186/s12883-019-1300-z
153. Hari R, Salmelin R. Magnetoencephalography: from SQUIDs to neuroscience. neuroimage 20th anniversary special edition. NeuroImage. (2012) 61:386–96. doi: 10.1016/j.neuroimage.2011.11.074
154. Broyd SJ, Demanuele C, Debener S, Helps SK, James CJ, Sonuga-Barke EJS. Default-mode brain dysfunction in mental disorders: a systematic review. Neurosci Biobehav Rev. (2009) 33:279–96. doi: 10.1016/j.neubiorev.2008.09.002
155. Salanova V. Deep brain stimulation for epilepsy. Epilepsy and Behavior. (2018) 88:21–4. doi: 10.1016/j.yebeh.2018.06.041
156. Meeren HKM, Pijn JPM, Van Luijtelaar ELJM, Coenen AML, Da Silva FHL. Cortical focus drives widespread corticothalamic networks during spontaneous absence seizures in rats. J Neurosci. (2002) 22:1480–95. doi: 10.1523/JNEUROSCI.22-04-01480.2002
157. Dalic LJ, Warren AEL, Young JC, Thevathasan W, Roten A, Bulluss KJ, et al. Cortex leads the thalamic centromedian nucleus in generalized epileptic discharges in Lennox-Gastaut syndrome. Epilepsia. (2020) 61:2214–23. doi: 10.1111/epi.16657
158. Martín-López D, Jiménez-Jiménez D, Cabañés-Martínez L, Selway RP, Valentín A, Alarcón G. The Role of thalamus versus cortex in epilepsy: evidence from human ictal centromedian recordings in patients assessed for deep brain stimulation. Int J Neural Syst. (2017) 27:101. doi: 10.1142/S0129065717500101
Keywords: magnetoencephalography, source localization, generalized genetic epilepsy, absence epilepsy, myoclonus epilepsy, epilepsy classification
Citation: Aung T, Tenney JR and Bagić AI (2022) Contributions of Magnetoencephalography to Understanding Mechanisms of Generalized Epilepsies: Blurring the Boundary Between Focal and Generalized Epilepsies? Front. Neurol. 13:831546. doi: 10.3389/fneur.2022.831546
Received: 08 December 2021; Accepted: 08 March 2022;
Published: 27 April 2022.
Edited by:
Stefano Seri, Aston University, United KingdomReviewed by:
Elaine Foley, Aston University, United KingdomVahab YoussofZadeh, Medical College of Wisconsin, United States
Copyright © 2022 Aung, Tenney and Bagić. This is an open-access article distributed under the terms of the Creative Commons Attribution License (CC BY). The use, distribution or reproduction in other forums is permitted, provided the original author(s) and the copyright owner(s) are credited and that the original publication in this journal is cited, in accordance with accepted academic practice. No use, distribution or reproduction is permitted which does not comply with these terms.
*Correspondence: Thandar Aung, YXVuZy50aGFuZGFyQG91dGxvb2suY29t