- 1Department of Neurology, The First Affiliated Hospital of Zhengzhou University, Zhengzhou, China
- 2Department of Neurology, Beijing Tiantan Hospital, Capital Medical University, Beijing, China
Lipids are implicated in inflammatory responses affecting acute ischaemic stroke prognosis. Therefore, we aimed to develop a predictive model that considers neutrophils and high-density lipoprotein cholesterol to predict its prognosis. This prospective study enrolled patients with acute ischaemic stroke within 24 h of onset between January 2015 and December 2017. The main outcome was a modified Rankin Scale score ≥3 at the 90th day of follow-up. Patients were divided into training and testing sets. The training set was divided into four states according to the median of neutrophils and high-density lipoprotein cholesterol levels in all patients. Through binary logistic regression analysis, the relationship between factors and prognosis was determined. A nomogram based on the results was developed; its predictive value was evaluated through internal and external validations. Altogether, 1,090 patients were enrolled with 872 (80%) and 218 (20%) in the training and testing sets, respectively. In the training set, the major outcomes occurred in 24 (10.4%), 24 (11.6%), 37 (17.2%), and 49 (22.3%) in states 1–4, respectively (P = 0.002). Validation of calibration and decision curve analyses showed that the nomogram showed better performance. The internal and external testing set receiver operating characteristics verified the predictive value [area under the curve = 0.794 (0.753–0.834), P < 0.001, and area under the curve = 0.973 (0.954–0.992), P < 0.001, respectively]. A nomogram that includes neutrophils and high-density lipoprotein cholesterol can predict the prognosis of acute ischaemic stroke, thus providing us with an effective visualization tool.
Introduction
Stroke is the leading cause of disability and the second leading cause of death worldwide (1). However, stroke is the leading cause of both death and disability in China (2). Ischaemic events are the first to occur in all stroke events, with a rate of ~70% (3). Statistics show that in 2018, 1.57 million stroke deaths were reported in China. Stroke has a significant impact on its socio-economic and family burdens. Therefore, tools that accurately predict its prognosis are needed, especially ischaemic stroke.
Many factors can affect the prognosis of ischaemic stroke, including the immune-inflammatory response, which is an essential process after stroke (4). Neutrophils are the first cells to reach the lesions after stroke and participate in a series of complex inflammatory responses (5). They are involved in the destruction and repair of local brain tissue in cerebral infarction. Studies have shown that inflammatory cells in peripheral blood after stroke also change along with local inflammatory cells (6), thus, testing patients' peripheral blood provides a clinical basis for quick judgment of their prognosis. For example, Zhu et al. (7) have shown that peripheral neutrophil count after stroke is associated with the stroke prognosis.
In addition, lipids are important factors in the inflammatory response. Recent studies have shown that high-density lipoprotein cholesterol (HDL-C) inhibits neutrophil deformation and platelet aggregation (8, 9), and inhibits the formation of neutrophil traps (10). These can prevent further damage to the infarcted tissue after promoting thrombosis. Therefore, we aimed to develop a predictive model that includes neutrophils and high-density lipoprotein cholesterol to predict the prognosis of acute ischaemic stroke (AIS).
Subjects and Methods
Study Population
This was a prospective consecutive hospital-based cohort study (11, 12). The AIS diagnosis was based on the criteria defined by the World Health Organization (13). Our study was approved by the Ethics Committee, and written informed consent was obtained from all participating patients and their families. We recruited patients with AIS who were admitted from January 2015 to December 2017.
The inclusion criteria were AIS patients admitted within 24 h of stroke onset in the database (not include patients with thrombectomy and admission with any infection). The exclusion criteria were as follows: patients who lacked blood cell count or lipid data; Immune diseases/chronic inflammatory diseases; patients with cancer or haematologic diseases or were using immunosuppressants; patients who had major trauma or surgery; patients with severe liver or kidney disease or chronic lung disease; and patients with incomplete follow-up information.
Data Collection
Baseline information was obtained from trained professionals who collected patient admission reports. Demographic characteristics included sex, age, and personal history (e.g., smoking). Clinical data included history and degree of stroke according to the National Institutes of Health Stroke Scale (NIHSS) at the time of admission. Laboratory tests included routine blood and lipid analyses obtained from the patients within 24 h of AIS onset. An automated analyser (Coulter LH 750 Hematology Analyzer; Beckman Coulter, Brea, CA, USA) was used to analyse the blood cell counts. All serum biochemical parameters were measured using a fully automatic biochemical analyser (COBAS 8000 fully automatic biochemical analyser; Roche, Basel, Switzerland). The routine blood cell count included white blood cells and neutrophils. Lipid analysis included the total cholesterol, triglycerides, HDL-C, and low-density lipoprotein.
Follow-Up and Assessment
Each patient completed 90 days of face-to-face or telephone follow-ups. The patients were assessed using the modified Rankin Scale (mRS) scale during follow-up. An mRS score ≥3 was defined as a poor prognosis, with mRS = 6 points representing death. An mRS score <3 was classified as a better prognosis. The main outcome of interest in this study was poor prognosis in patients during the 90-day follow-up.
Grouping and Design
The patients were grouped based on the admission time. The first four sessions were selected as the training set, and the last session was the testing set (with a 4:1 ratio), and the training set was compared with the testing set. The training set is used to fit the predictive model and develop the nomogram, and the testing set to validate the model externally. First, we determined the median of neutrophils and HDL-C levels in study populations and divided them into high and low groups. Afterwards, four combined neutrophils and high-density lipoprotein cholesterol states were formed: state 1, group with low neutrophils and high HDL-C; state 2, group with low neutrophils and low HDL-C; state 3, group with high neutrophils and high HDL-C; state 4, group with high neutrophils and low HDL-C. The different states were compared for poor outcomes on the 90th day of follow-up. A univariate logistic regression analysis was conducted, and variables showing significance were included in a multivariate logistic regression analysis to develop a nomogram that predicts the 90-day prognosis. Internal and external evaluations were carried out in the training and testing sets, respectively. The evaluation methods included calibration curve, decision curve analysis, and receiver operating characteristic (ROC) curve.
Statistical Analyses
All data analyses for this study were performed using SPSS Statistics version 23.0 (IBM Corp., Armonk, NY, USA). In the training set, the classification data were expressed in frequency (%), and inter-group comparison was performed using either the χ2 test or the Fisher precision test. The relationship between the variables and 90-day prognosis was analyzed using logistic regression. Nomogram development and performance evaluation were performed using R Studio based on R x64 4.0.4 (The R Project, Vienna, Austria). A two-sided P-value of <0.05 indicate significant difference and statistical significance.
Results
Comparison of the Training Set With the Testing Set
According to the research process (Figure 1), 1,090 patients met our study criteria, men, n = 738, 67.7%; age ≥60 years, n = 600, 55.0%; with major outcome, n = 165, 15.1%. The characteristics of the patients and the comparison of the training and testing sets are shown in Table 1. There were no significant differences in most variables in the group comparison between the training and testing sets, indicating high similarity between the sets. Therefore, the models fitted by the training set will be well-representative.
Baseline Characteristics of the Training Set
In the 872 patients in the training set, men, n = 588, 67.4%; age ≥60 years, n = 394, 45.2%; with major outcome, n = 134, 15.4%; states 1, 2, 3, and 4 had 230, 207, 215, and 220 patients, respectively (Table 2). The proportion of patients with poor prognosis was increasing in the 90-day follow-up, 24 (10.4%), 24 (11.6%), 37 (17.2%), and 49 (22.3%) in states 1–4, respectively (P = 0.002).
Neutrophils and High-Density Lipoprotein Cholesterol States With Prognosis
We first conducted a univariate logistic regression analysis to explore the factors that affect poor prognosis during the 90-day follow-up (Table 3). There are significant differences in those aged ≥60 years, men, those with NIHSS of >5 on admission, mRS score >1 before stroke, those with stroke, coronary heart diseases, and atrial fibrillation, and states 3 and 4. The multivariate logistic regression analysis (Table 3) revealed that age ≥60 years [odds ratio (OR) = 1.887, 95% confidence interval (95% CI): 1.205–2.955, P = 0.006], NIHSS >5 on admission (OR = 7.200, 95% CI: 4.678–11.081, P < 0.001), mRS score >1 before stroke (OR = 4.945, 95% CI: 1.593–15.348, P = 0.006), stroke (OR = 1.866, 95% CI: 1.155–3.015, P = 0.011), and state 4 (OR = 2.224, 95% CI: 1.215–4.072, P = 0.010) were significantly different.
Development and Evaluation of Nomogram
Variables with significant differences in the multivariate logistic regression analysis, including age ≥60 years, NIHSS >5 on admission, mRS score >1 before stroke, history of stroke, neutrophils, and high-density lipoprotein cholesterol states, were selected for the development of the nomogram (Figure 2). We conducted an internal effect evaluation by the training set for the nomogram. The calibration and decision curve analyses showed good consistency and practicability of the model (Figures 3A,C), and the ROC curve showed a good predictive effect [area under the curve = 0.794 (0.753–0.834), P < 0.001] (Figures 4A,C). To further confirm the accuracy of the nomogram, we conducted an external effect evaluation by the testing set that had nothing to do with the construction of the model. Similarly, the calibration curve and decision curve analysis showed good consistency and practicability of the model (Figures 3B,D), and the ROC curve showed a good prediction effect [area under the curve = 0.973 (0.954–0.992), P < 0.001] (Figures 4B,C).
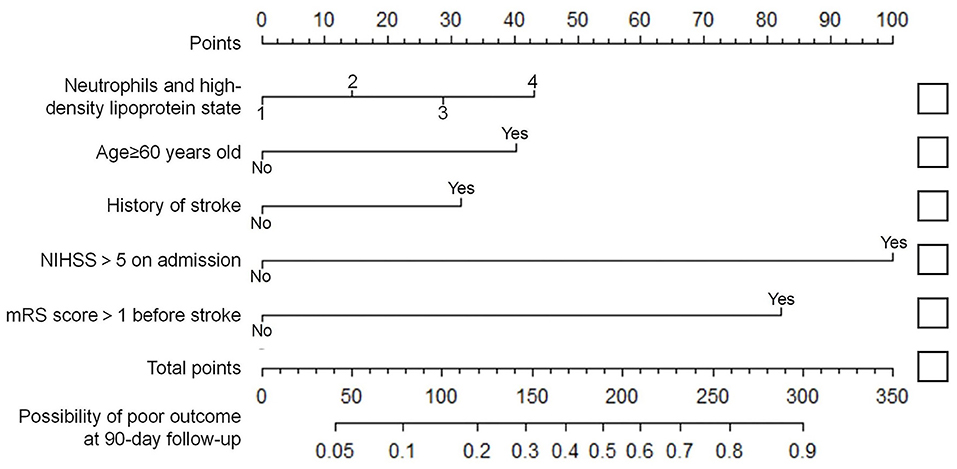
Figure 2. Nomogram for predicting short-term prognosis of acute ischemic stroke. In the neutrophils and high-density lipoprotein cholesterol states variable, state 1 scored 0 points, state 2 scored 14.3 points, state 3 scored 28.7 points, and state 4 scored 43.0 points. Age ≥60 years old, with a history of stroke, mRS >1 before the onset and NIHSS >5 at admission were recorded as 40.3 points, 31.5 points, 82.2 points and 100 points, respectively. Otherwise score 0 points (see Supplementary Materials). State 1: N <4.79 × 109 /L and HDL-C ≥1.08 mmol/L; State 2: N <4.79 × 109 /L and HDL-C <1.08 mmol/L; State 3: N ≥4.79 × 109 /L and HDL-C ≥1.08 mmol/L; State 4: N ≥4.79 × 109 /L and HDL-C <1.08 mmol/L; N, Neutrophil; HDL-C, high-density lipoprotein cholesterol.
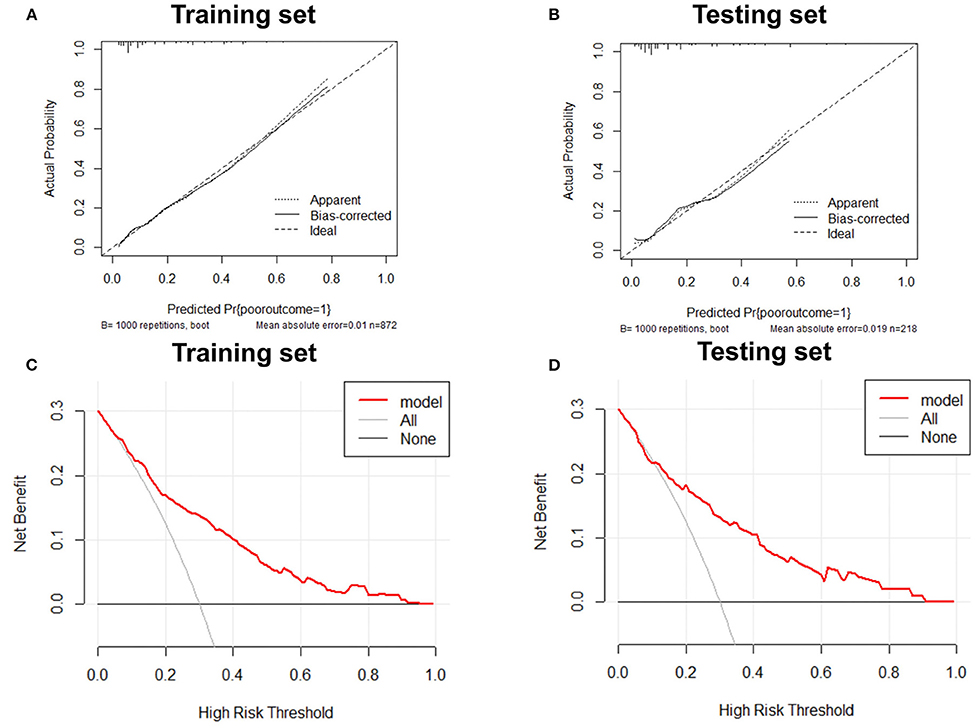
Figure 3. Calibration curve and decision curve analysis of training set and testing set. Internal and external validation of predictive models. (A) The calibration curve of the prediction model in the training set; (B) The calibration curve of the prediction model in the testing set; (C) The decision curve analysis of the prediction model in the training set; (D) The decision curve analysis of the prediction model in the testing set.
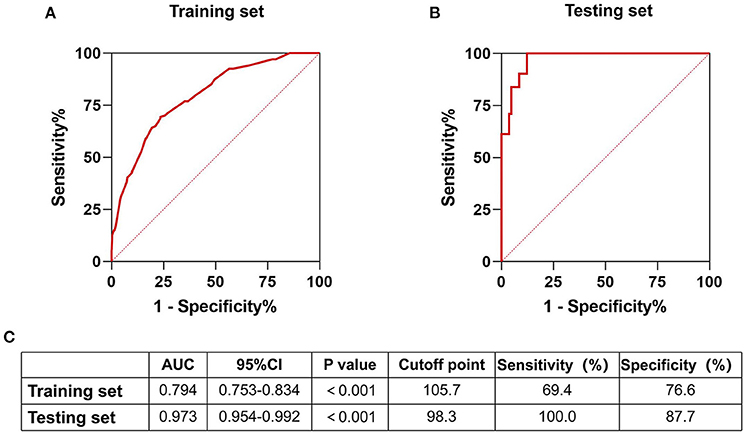
Figure 4. Receiver operating curve of training set and testing set. Internal and external validation of predictive models. (A) The receiver operating curve of the prediction model in the training set; (B) The receiver operating curve of the prediction model in the testing set; (C) Part of the result data display of the receiver operating curve of the nomograph in the training set and the test set.
Discussion
Inflammation is a new prevention target in stroke risk, and the secondary prevention of vascular risk after stroke requires new treatment methods (14). Thus, new indicators and markers as early warning signs are needed. Most predictive models currently include only traditional risk factors. Hence, the development of predictive models with inflammation and lipids is needed. Recently, Li et al. (15) examined the effects of residual inflammation risk on stroke and transient ischaemic attack (TIA) prognosis and found that those with high cholesterol levels and high-sensitivity C-reactive protein had a worse prognosis than those with only high levels of high-sensitivity C-reactive protein. Based on these ideas, the correlation of neutrophils and HDL-C states with prognosis of AIS was analyzed, and a clinically practical nomogram was developed. The results showed that the proportion of poor outcomes in the four states increased, proving the difference in prognosis in various states. The multivariate analysis revealed a significant difference and independent correlation between state 4 and poor outcome at the 90-day follow-up (OR = 2.224, 95% CI: 1.215–4.072, P = 0.010). Our nomogram, which includes neutrophils and HDL-C states, has been developed to provide clinicians and patients with visual and practical predictive tools. The evaluation of the effect for the nomogram by internal validation in training set and external validation in testing set fully demonstrated the advantages of the model.
Studies have shown a close link between inflammation and lipid media (16, 17). Few previous studies that have used the combination of inflammation and lipids to predict the prognosis of AIS have recently attracted increasing attention from scholars worldwide. For example, Yin and Kou et al. found that the neutrophil-to-HDL ratio is not only closely related to coronary artery stenosis but is also an independent predictor of severe coronary artery stenosis (18). Based on this ratio, it will be interesting to explore the relationship between the different states of neutrophils and HDL-C composition and stroke prognosis.
Numerous studies have shown that neutrophils are closely related to the prognosis of stroke. The involvement of neutrophils after stroke was confirmed in experimental animals and tissues from stroke patients who died (19). Neutrophils are involved in the acute and recovery period; thus, their effect on the AIS prognosis is significant (20). These indicate that neutrophils are involved in the infarct tissue in space and time. On a deeper level, a study has shown that the formation of neutrophil traps promotes thrombosis and further destroys lesions (21). Meanwhile, after stroke, neutrophils can bind to adhesive molecules in the inner walls of blood vessels and remain in the capillaries around the lesions (22). The above studies show that neutrophils can greatly affect functional recovery. On the other hand, Zhu et al. (7) confirmed the prognostic relationship between neutrophils in peripheral blood and AIS and TIA. This shows that although we cannot directly detect neutrophils in the infarcted area, the neutrophil count in the peripheral blood can still provide us with a meaningful reference. What's more, HDL-C, a lipid, is a recognized vascular protection factor (23). A meta-analysis of prospective cohort studies showed that high HDL-C levels reduced the incidence of stroke (24), while low HDL-C levels were associated with higher stroke severity and poor clinical prognosis (25). Studies have shown that HDL-C regulates the activity of a variety of inflammatory cells, including neutrophils and eosinophils (26). Further studies have shown that HDL-C can inhibit neutrophil activity and transformation (10). The effect of HDL-C inhibiting neutrophils is the basis of our research. We hope to see the prognosis of AIS in different neutrophils and high-density lipoprotein cholesterol states.
From a researcher's point of view, the results suggest that interventions against neutrophils and HDL-C may be potential therapeutic targets for improving the prognosis of AIS. Neutrophil retention still occurs in the capillaries after AIS revascularization, reducing blood supply to the end brain tissue. This is one of the important factors affecting the prognosis of stroke. Studies have shown that injections to remove neutrophil reagents improve stroke prognosis (27). Studies have also shown that HDL-C confers endotheliocyte protection. Using an experimental model of AIS, the researchers demonstrated the role of HDL-C receptor scavenger receptor class B type I, which is expressed by endothelial cells, in the neuroprotective effect of HDL-C. The results show that stroke volume can be reduced to improve prognosis (28). The above studies suggest that interventions for neutrophils and HDL-C and improve the prognosis of stroke still need a lot of work.
This study had some limitations. First, the sample size was relatively small and was from a single hospital, hence, there may be selection bias. Second, when the patients were grouped into the training and testing sets, they were divided according to admission time. There may be selection bias, because of the differences in medical and health conditions before and after admission time. Finally, we studied the blood from the peripheral veins of patients with ischaemic stroke who were admitted within 24 h without considering the dynamic changes in inflammatory cells and lipids in peripheral blood over time. Nevertheless, neutrophils and HDL-C states can be used as predictive indicators for the AIS prognosis, and a nomogram has been developed for clinical prediction, although it still needs to be expanded further for larger-scale verification in more centers and with larger sample sizes.
In conclusion, the study found that neutrophils and HDL-C can be used to predict the patients' prognosis 90 days after AIS. The developed nomogram, which includes neutrophils and high-density lipoprotein cholesterol, can provide clinicians and patients with visual prediction of the prognosis of AIS.
Data Availability Statement
The raw data supporting the conclusions of this article will be made available by the authors, without undue reservation.
Ethics Statement
The studies involving human participants were reviewed and approved by the Ethics Committee of the First Affiliated Hospital of Zhengzhou University. The patients/participants provided their written informed consent to participate in this study.
Author Contributions
BS and YX designed the overall study. NW designed and carried out experiments and collected data by NW, HL, MT, JL, LZ, WS, LP, and KL and so on. NW wrote the manuscript. BS and YW supervised this study, designed experiments, and edited the article. All authors contributed to the article and approved the submitted version.
Funding
This study was funded by the National Key Research and Development Program, Major Chronic Non-communicable Disease Prevention and Control Research Key Special Project (2017YFC1308202) and the Non-profit Central Research Institute Fund of Chinese Academy of Medical Sciences (2020-PT310-01).
Conflict of Interest
The authors declare that the research was conducted in the absence of any commercial or financial relationships that could be construed as a potential conflict of interest.
Publisher's Note
All claims expressed in this article are solely those of the authors and do not necessarily represent those of their affiliated organizations, or those of the publisher, the editors and the reviewers. Any product that may be evaluated in this article, or claim that may be made by its manufacturer, is not guaranteed or endorsed by the publisher.
Supplementary Material
The Supplementary Material for this article can be found online at: https://www.frontiersin.org/articles/10.3389/fneur.2022.827279/full#supplementary-material
References
1. Collaborators GDaH. Global, regional, and national disability-adjusted life-years (DALYs) for 359 diseases and injuries and healthy life expectancy (HALE) for 195 countries and territories, 1990–2017: a systematic analysis for the global burden of disease study 2017. Lancet. (2018) 392:1859–922. doi: 10.1016/S0140-6736(18)32335-3
2. Collaborators TGLRoS. Global, regional, and country-specific lifetime risks of stroke, 1990 and 2016. N Engl J Med. (2018) 379:2429–37. doi: 10.1056/NEJMoa1804492
3. Herpich F, Rincon F. Management of acute ischemic stroke. Crit Care Med. (2020) 48:1654–63. doi: 10.1097/CCM.0000000000004597
4. Anrather J, Iadecola C. Inflammation and stroke: an overview. Neurotherapeutics. (2016) 13:661–70. doi: 10.1007/s13311-016-0483-x
5. Tang C, Wang C, Zhang Y, Xue L, Li Y, Ju C, et al. Recognition, intervention, and monitoring of neutrophils in acute ischemic stroke. Nano Letters. (2019) 19:4470–7. doi: 10.1021/acs.nanolett.9b01282
6. Zera KA, Buckwalter MS. The local and peripheral immune responses to stroke: implications for therapeutic development. Neurotherapeutics. (2020) 17:414–35. doi: 10.1007/s13311-020-00844-3
7. Zhu B, Pan Y, Jing J, Meng X, Zhao X, Liu L, et al. Neutrophil counts, neutrophil ratio, and new stroke in minor ischemic stroke or TIA. Neurology. (2018) 90:e1870–8. doi: 10.1212/WNL.0000000000005554
8. Murphy AJ, Woollard KJ, Suhartoyo A, Stirzaker RA, Shaw J, Sviridov D, et al. Neutrophil activation is attenuated by high-density lipoprotein and apolipoprotein a-i in in vitro and in vivo models of inflammation. Arterioscl Thromb Vasc Biol. (2011) 31:1333–41. doi: 10.1161/ATVBAHA.111.226258
9. Marco van der Stoep SJAK, Miranda VE. High-density lipoprotein as a modulator of platelet and coagulation responses. Cardiovasc Res. (2014) 103:362–71. doi: 10.1093/cvr/cvu137
10. Curcic S, Holzer M, Frei R, Pasterk L, Schicho R, Heinemann A, et al. Neutrophil effector responses are suppressed by secretory phospholipase A2 modified HDL. Biochim Biophys Acta. (2015) 1851:184–93. doi: 10.1016/j.bbalip.2014.11.010
11. Tian M, Li Y, Wang X, Tian X, Pei LL, Wang X, et al. The hemoglobin, albumin, lymphocyte, and platelet (HALP) score is associated with poor outcome of acute ischemic stroke. Front Neurol. (2020) 11:610318. doi: 10.3389/fneur.2020.610318
12. Song B, Hu R, Pei L, Cao Y, Chen P, Sun S, et al. Dual antiplatelet therapy reduced stroke risk in high-risk patients with transient ischaemic attack assessed by ABCD3-I score. Eur J Neurol. (2019) 26:610–6. doi: 10.1111/ene.13864
13. Stroke−1989. Recommendations on stroke prevention, diagnosis, and therapy. Report of the WHO task force on stroke and other cerebrovascular disorders. Stroke. (1989) 20:1407–31. doi: 10.1161/01.STR.20.10.1407
14. Kelly PJ, Lemmens R, Tsivgoulis G. Inflammation and stroke risk: a new target for prevention. Stroke. (2021) 52:2697–706. doi: 10.1161/STROKEAHA.121.034388
15. Li J, Pan Y, Xu J, Li S, Wang M, Quan K, et al. Residual inflammatory risk predicts poor prognosis in acute ischemic stroke or transient ischemic attack patients. Stroke. (2021) 2021:STROKEAHA120033152. doi: 10.1161/STROKEAHA120033152
16. Lawrence T, Willoughby DA, Gilroy DW. Anti-inflammatory lipid mediators and insights into the resolution of inflammation. Nat Rev Immunol. (2002) 2:787–95. doi: 10.1038/nri915
17. Kasuga K, Suga T, Mano N. Bioanalytical insights into mediator lipidomics. J Pharm Biomed Anal. (2015) 113:151–62. doi: 10.1016/j.jpba.2015.02.011
18. Kou T, Luo H, Yin L. Relationship between neutrophils to HDL-C ratio and severity of coronary stenosis. BMC Cardiovasc Disord. (2021) 21:127. doi: 10.1186/s12872-020-01771-z
19. Perez-de-Puig I, Miro-Mur F, Ferrer-Ferrer M, Gelpi E, Pedragosa J, Justicia C, et al. Neutrophil recruitment to the brain in mouse and human ischemic stroke. Acta Neuropathol. (2015) 129:239–57. doi: 10.1007/s00401-014-1381-0
20. Zhu Z, Zheng L, Li Y, Huang T, Chao YC, Pan L, et al. Potential immunotherapeutic targets on myeloid cells for neurovascular repair after ischemic stroke. Front Neurosci. (2019) 13:758. doi: 10.3389/fnins.2019.00758
21. Kang L, Yu H, Yang X, Zhu Y, Bai X, Wang R, et al. Neutrophil extracellular traps released by neutrophils impair revascularization and vascular remodeling after stroke. Nat Commun. (2020) 11:2488. doi: 10.1038/s41467-020-16191-y
22. Erdener SE, Tang J, Kilic K, Postnov D, Giblin JT, Kura S, et al. Dynamic capillary stalls in reperfused ischemic penumbra contribute to injury: a hyperacute role for neutrophils in persistent traffic jams. J Cereb Blood Flow Metab. (2021) 41:236–52. doi: 10.1177/0271678X20914179
23. Luscher TF, Landmesser U, von Eckardstein A, Fogelman AM. High-density lipoprotein: vascular protective effects, dysfunction, and potential as therapeutic target. Circ Res. (2014) 114:171–82. doi: 10.1161/CIRCRESAHA.114.300935
24. Qie R, Liu L, Zhang D, Han M, Wang B, Zhao Y, et al. Dose-response association between high-density lipoprotein cholesterol and stroke: a systematic review and meta-analysis of prospective cohort studies. Prev Chronic Dis. (2021) 18:E45. doi: 10.5888/pcd18.200278
25. Yeh PS, Yang CM, Lin SH, Wang WM, Chen PS, Chao TH, et al. Low levels of high-density lipoprotein cholesterol in patients with atherosclerotic stroke: a prospective cohort study. Atherosclerosis. (2013) 228:472–7. doi: 10.1016/j.atherosclerosis.2013.03.015
26. Trakaki A, Marsche G. Current understanding of the immunomodulatory activities of high-density lipoproteins. Biomedicines. (2021) 9:587. doi: 10.3390/biomedicines9060587
27. El Amki M, Gluck C, Binder N, Middleham W, Wyss MT, Weiss T, et al. Neutrophils obstructing brain capillaries are a major cause of no-reflow in ischemic stroke. Cell Rep. (2020) 33:108260. doi: 10.1016/j.celrep.2020.108260
Keywords: stroke, neutrophil, nomogram, prognosis, high-density lipoprotein cholesterol
Citation: Wang N, Liu H, Tian M, Liang J, Sun W, Zhang L, Pei L, Liu K, Sun S, Wu J, Gao Y, Xu Y, Wang Y and Song B (2022) A Nomogram That Includes Neutrophils and High-Density Lipoprotein Cholesterol Can Predict the Prognosis of Acute Ischaemic Stroke. Front. Neurol. 13:827279. doi: 10.3389/fneur.2022.827279
Received: 22 December 2021; Accepted: 02 February 2022;
Published: 25 February 2022.
Edited by:
Wen-Jun Tu, Chinese Academy of Medical Sciences and Peking Union Medical College, ChinaReviewed by:
Miao Chen, University of Shanghai for Science and Technology, ChinaJian Xia, Central South University, China
Copyright © 2022 Wang, Liu, Tian, Liang, Sun, Zhang, Pei, Liu, Sun, Wu, Gao, Xu, Wang and Song. This is an open-access article distributed under the terms of the Creative Commons Attribution License (CC BY). The use, distribution or reproduction in other forums is permitted, provided the original author(s) and the copyright owner(s) are credited and that the original publication in this journal is cited, in accordance with accepted academic practice. No use, distribution or reproduction is permitted which does not comply with these terms.
*Correspondence: Yilong Wang, yilong528@aliyun.com; Bo Song, fccsongb@zzu.edu.cn