- 1Department of Neurology and Stroke Center, The First Affiliated Hospital of Jinan University, Jinan University, Guangzhou, China
- 2Clinical Neuroscience Institute, The First Affiliated Hospital of Jinan University, Jinan University, Guangzhou, China
- 3Department of Neurology and Stroke Center, The Central Hospital of Shaoyang, Shaoyang, China
Background: We aimed to develop and validate a new nomogram for predicting the risk of intracranial hemorrhage (ICH) in patients with acute ischemic stroke (AIS) after intravenous thrombolysis (IVT).
Methods: A retrospective study enrolled 553 patients with AIS treated with IVT. The patients were randomly divided into two cohorts: the training set (70%, n = 387) and the testing set (30%, n = 166). The factors in the predictive nomogram were filtered using multivariable logistic regression analysis. The performance of the nomogram was assessed based on the area under the receiver operating characteristic curve (AUC-ROC), calibration plots, and decision curve analysis (DCA).
Results: After multivariable logistic regression analysis, certain factors, such as smoking, National Institutes of Health of Stroke Scale (NIHSS) score, blood urea nitrogen-to-creatinine ratio (BUN/Cr), and neutrophil-to-lymphocyte ratio (NLR), were found to be independent predictors of ICH and were used to construct a nomogram. The AUC-ROC values of the nomogram were 0.887 (95% CI: 0.842–0.933) and 0.776 (95% CI: 0.681–0.872) in the training and testing sets, respectively. The AUC-ROC of the nomogram was higher than that of the Multicenter Stroke Survey (MSS), Glucose, Race, Age, Sex, Systolic blood Pressure, and Severity of stroke (GRASPS), and stroke prognostication using age and NIH Stroke Scale-100 positive index (SPAN-100) scores for predicting ICH in both the training and testing sets (p < 0.05). The calibration plot demonstrated good agreement in both the training and testing sets. DCA indicated that the nomogram was clinically useful.
Conclusions: The new nomogram, which included smoking, NIHSS, BUN/Cr, and NLR as variables, had the potential for predicting the risk of ICH in patients with AIS after IVT.
Introduction
Stroke is the second leading cause of death and a major leading contributor to disability worldwide (1). Intravenous thrombolysis (IVT) therapy with recombinant tissue plasminogen activator (rt-PA) has proven to be a beneficial treatment for acute ischemic stroke (AIS) patients when administered within 4.5 h after stroke onset (2, 3). However, IVT therapy carries an inherent risk of complications, such as intracranial hemorrhage (ICH), that are associated with poor clinical outcomes. Furthermore, symptomatic intracranial hemorrhage (symptomatic ICH) is the most serious complication of IVT (4, 5).
Since ICH may lead to poor prognosis, a reliable scoring tool that can be used to identify the risk of post-thrombolysis ICH is essential. Several scoring systems have been applied to predict the risk of ICH after thrombolysis (6–10). However, most of these systems convert continuous variables to categorical variables, and this change might result in loss of information (11, 12). In addition, several prognostic nomograms for ICH events in patients with AIS who underwent thrombolysis have been reported, most of which were constructed based on Western populations (13–15). It was reported that the rate of ICH in Asian populations was 10.6%, while the rate of ICH in non-Asian populations was reported to be 4.74% (16). The reported ICH occurrence rate is 2.12-fold higher in Asian populations than in non-Asian populations. Two nomogram models based on Asian populations have been designed (12, 17). However, those nomogram models only include demographic and clinical factors, such as hypertension, blood glucose, blood platelet (PLT), and National Institutes of Health of Stroke Scale (NIHSS) scores, and ignore other factors that can be assessed based on laboratory examinations and that may be important risk factors for predicting ICH. We wondered whether some parameters that can be measured through laboratory examination could be risk factors for ICH.
In this study, we aimed to develop a new nomogram to predict the risk of ICH after IVT therapy and to compare the performance of our model with that of other scoring systems for predicting ICH. The nomogram we developed will help clinicians identify patients with AIS with a higher risk of ICH after IVT therapy.
Methods
Study Population and Design
The present study was a retrospective study performed in the stroke center of the Central Hospital of Shaoyang between November 2013 and January 2021. The patients met the following inclusion criteria: 1) more than 18 years of age; 2) diagnosed with AIS confirmed by MRI within 24 h after admission; 3) onset-to-treatment time for thrombolysis of <4.5 h; 4) they or their legal representatives provided written informed consent to participation in the study; and 5) treated with IVT. The exclusion criteria were as follows: 1) treated with endovascular procedures after IVT; and) not treated with IVT or refused IVT treatment; and 3) medical contraindications for IVT.
This study was approved by the Ethics Committee of the Central Hospital of Shaoyang. The Ethics Committee approval letter is available in Online Resource 1.
Baseline Data Collection
We collected demographic, clinical, and laboratory information at admission and during hospitalization. The baseline data included age, sex, current smoking status, current drinking status, NIHSS score on admission, systolic blood pressure (SBP), diastolic blood pressure (DBP), blood glucose level on admission, history of hypertension and diabetes mellitus, atrial fibrillation (AF), international normalized ratio (INR), activated partial thromboplastin time (APTT), PLT, fibrinogen, albumin (ALB), white blood cells (WBC), neutrophil-to-lymphocyte ratio (NLR), high-density lipoprotein (HDL), low-density lipoprotein (LDL), triglyceride (TG), total cholesterol (TC), blood urea nitrogen-to-creatinine ratio (BUN/Cr), and uric acid (UA).
Thrombolysis Method
All patients received rt-PA treatment within 4.5 h after the onset of stroke. Intravenous rt-PA at 0.9 mg/kg (90 mg maximum) was used; 10% of the total dose was administered as an intravenous bolus, followed by infusion of the remaining dose over 60 min (18).
Ascertainment of ICH
All patients underwent a CT scan on admission, and each patient underwent another CT scan 24 h after intravenous rt-PA administration. In cases in which the patient's neurological function deteriorated rapidly, a CT scan was immediately performed to assess the presence of ICH. According to the European Cooperative Acute Stroke Study-2 criteria (19), the discovery of blood at any site in the brain on a CT scan indicated the presence of ICH. Symptomatic ICH was defined as any type of ICH accompanied by an increase in the NIHSS score by 4 or more points from baseline or leading to death; asymptomatic ICH was defined as any type of ICH without neurological deterioration. The endpoint in our research was ICH, including symptomatic ICH and asymptomatic ICH.
Statistical Analysis
A total of 553 patients were randomly divided into two groups, the training set (n = 387) and the testing set (n = 166), at a theoretical ratio of 7:3. Categorical variables and continuous variables are expressed as frequencies (percentages, %) and means (SDs) or medians (interquartile ranges, IQRs), respectively. The differences in baseline characteristics between the training set and the testing set were assessed using Student's t-test or the non-parametric Mann–Whitney U test for continuous variables and the χ2 or Fisher's exact test for categorical variables. The least absolute shrinkage and selection operator (LASSO) method was used to screen the optimal risk factors related to ICH in the total dataset. The variables with non-zero coefficients in the LASSO regression model were selected for further analysis. In the training cohort, univariable logistic regression analysis was used to predict the probability of ICH. The variables with P-value <0.05 were added to the multivariable logistic regression analysis to screen for independent clinical predictors related to ICH. A nomogram was generated based on these risk factors in multivariable analysis. The area under the receiver operating characteristic curve (AUC-ROC) analysis was performed to assess the predictive accuracy of the nomogram, and calibration curves were drawn to compare the predicted probabilities with the observed probabilities. Decision curve analysis (DCA) was used to evaluate the clinical value of the predictive model.
The overall predictive and discriminative performance of the nomogram was compared with that of the Multicenter Stroke Survey (MSS) score, the Glucose, Race, Age, Sex, Systolic blood Pressure, and Severity of stroke (GRASPS) score, and the stroke prognostication using age and NIH Stroke Scale-100 positive index (SPAN-100) score. The AUC-ROC was used to estimate the accuracy and discrimination of the nomogram and these scoring systems in the training and testing sets. DeLong's test was performed to compare the differences in AUC-ROC between the ICH nomogram and the MSS score, GRASPS score, and SPAN-100 score.
Statistical analyses were performed using R version 3.6.3 software (http://www.R-project.org/) and SPSS version 27.0 (IBM, New York, NY, USA). Two-tailed values of p < 0.05 were considered statistically significant.
Results
Baseline Characteristics
A total of 735 patients with AIS were initially enrolled in this study between November 2013 and January 2021. Of these, 182 patients who met the exclusion criteria were removed from the study, and 553 patients were finally eligible for analysis (Figure 1).
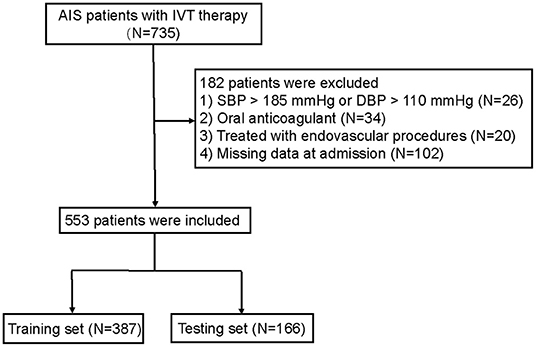
Figure 1. Flow diagram of the selection of eligible patients. AIS, acute ischemic stroke; IVT, intravenous thrombolysis. SBP, systolic blood pressure; DBP, diastolic blood pressure.
The training set consisted of 387 individuals, and the remaining 166 individuals were included in the testing set. The baseline characteristics of the patients in the training and testing sets are shown in Table 1. The median age of the patients in the training set was 69.51 ± 12.09 years, and 253 (65.37%) of them were men. The testing set consisted of 111 men (66.87%) with a median age of 68.73 ± 12.21 years. Of the total patients, the overall number with ICH was 51 (9.22%). The percentages of patients with ICH were 8.01 and 12.65% in the training set and the testing set, respectively. All variables were balanced between the two groups (p > 0.05).
Variable Selection
Through searching and analysis of the related literature, 24 potential risk factors among the demographic, clinical, and laboratory indicators of the patients were included in the LASSO regression analysis (Figure 2). The variables with non-zero coefficients in the LASSO regression model were considered to be related to ICH and were selected for further analysis; they included smoking, AF, SBP, DBP, NIHSS, PLT, ALB, HDL, LDL, BUN/Cr, and NLR (Table 2).
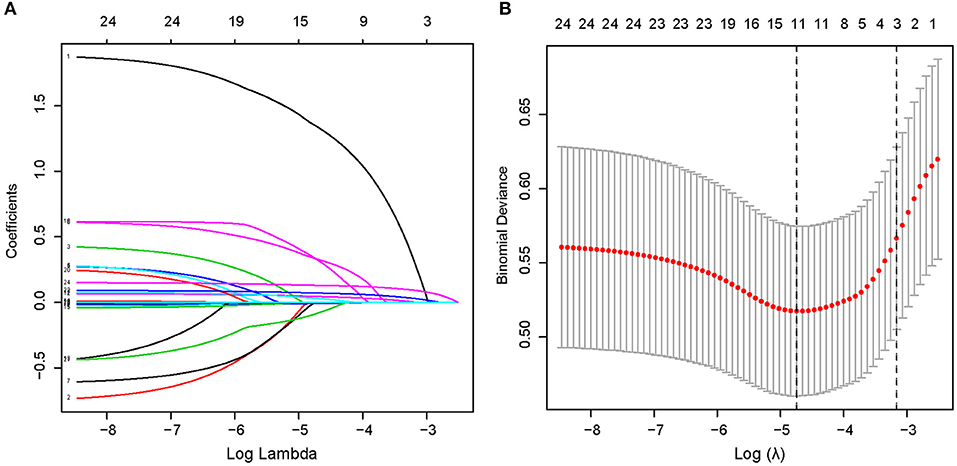
Figure 2. Demographic and clinical feature selection using the LASSO binary logistic regression model in a total of 553 patients. (A) The selection of the best parameter (lambda) in the LASSO model uses five-fold cross-validation with the lowest standard. The relationship curve between partial likelihood deviation (binomial deviation) and log(lambda) was plotted. Dotted vertical lines were drawn at the optimal values by using the minimum criteria and the 1 SE of the minimum criteria (the 1—SE criteria). (B) LASSO coefficient profiles of the 11 features. A coefficient profile plot was produced against the log(lambda) sequence. A vertical line was drawn at the value selected using five-fold cross-validation, where optimal lambda resulted in five features with non-zero coefficients. LASSO, least absolute shrinkage and selection operator; SE, standard error.
Univariable and Multivariable Analyses
A univariable analysis of the training set revealed that smoking, AF, NIHSS, BUN/Cr, and NLR were related to ICH. These factors were therefore used in multivariable logistic regression analysis for screening independent clinical predictors of ICH. Multivariable logistic regression analysis demonstrated that four variables (smoking, NIHSS, BUN/Cr, and NLR) are independently associated with ICH (p < 0.05), as shown in Table 3. The results indicated that these four variables were independent clinical predictors of ICH in patients with AIS after IVT.
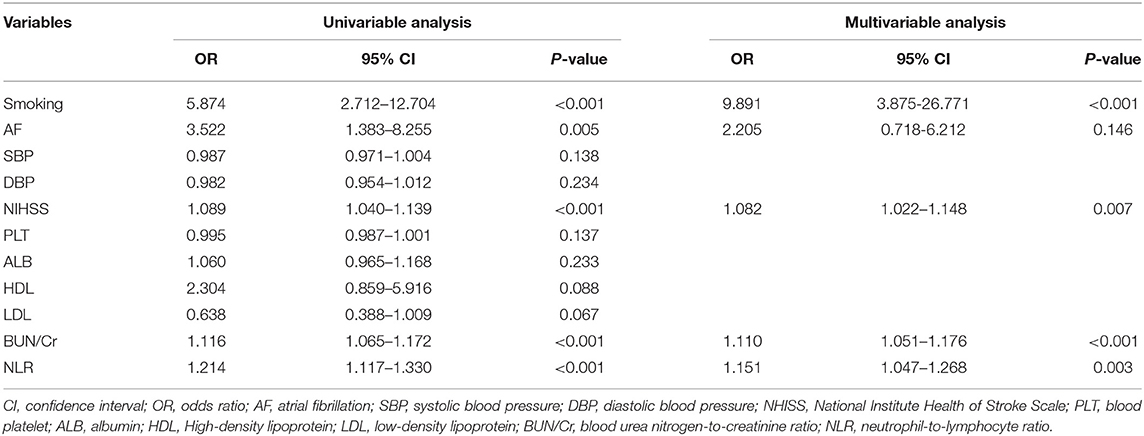
Table 3. Univariable and multivariable analyses of intracranial hemorrhage in AIS patients with Intravenous thrombolysis in the training set.
Predictive Model Development
The four variables selected by logistic regression analysis (smoking, NIHSS, BUN/Cr, and NLR) were used to build a nomogram for predicting the risk of ICH in AIS patients with IVT (Figure 3). The ratios of the calculated beta were used to evaluate the proportional prognostic effects of these variables. The projections from total points on the scales below indicated the estimated probability of ICH.
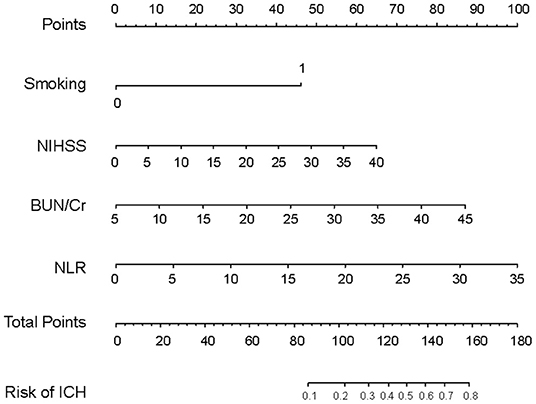
Figure 3. Nomogram model for predicting individual risk of intracranial hemorrhage in AIS patients with intravenous thrombolysis. For all patients, adding up the points identified on the points scale for all four indicators. Then, the sum is located on the “Total Points” axis. Finally, the risk of ICH according to the nomogram is the probability of “ICH” corresponding to “Total Points”. NHISS, National Institute Health of Stroke Scale; BUN/Cr, blood urea nitrogen-to-creatinine ratio; NLR, neutrophil-to-lymphocyte ratio.
Nomogram Validation
To assess the performance of the nomogram in predicting ICH, the likelihood that each patient would experience ICH was also predicted based on his or her MSS scores, GRASPS scores, and SPAN-100 scores. We compared the discrimination of our nomogram with those obtained using MSS scores, GRASPS scores, and SPAN-100 scores using AUC-ROC. As shown in Figure 4 and Table 4, the value of AUC-ROC obtained using our nomogram (0.887; 95% CI: 0.842–0.933) is greater than that obtained using the MSS scores (0.723; 95% CI: 0.637–0.808), the GRASPS scores (0.738; 95% CI: 0.646–0.831), and the SPAN-100 scores (0.588; 95% CI: 0.512–0.663) in the training set (p < 0.05). Similar to the results obtained with the training set, the value of AUC-ROC in our model (0.776; 95% CI: 0.681–0.872) was superior to the values obtained using the MSS scores (0.647; 95% CI: 0.508–0.785), the GRASPS scores (0.671; 95% CI: 0.552–0.791), and the SPAN-100 scores (0.552; 95% CI: 0.459–0.645) in the testing set (p < 0.05).
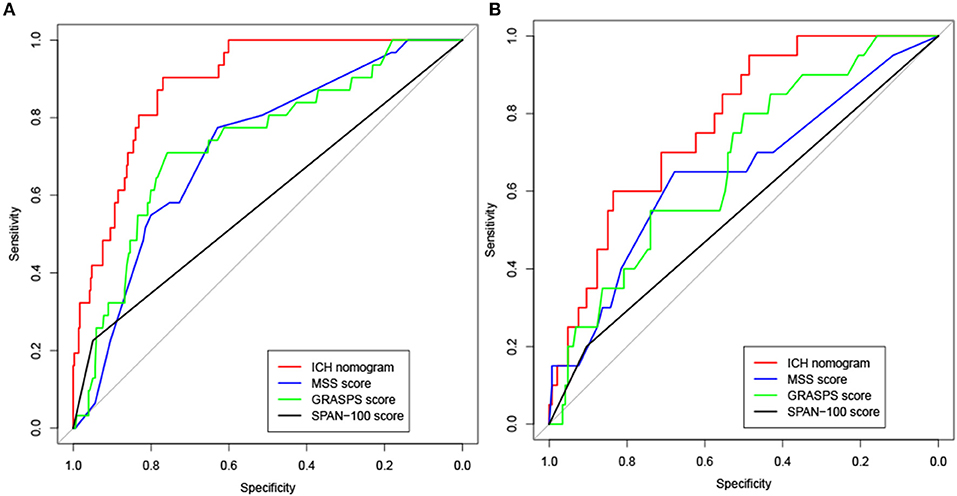
Figure 4. Receiver operating characteristic (ROC) curve analysis for the ICH nomogram, MSS scores, GRASPS scores, and SPAN-100 scores in the training set (A) and testing set (B). MSS (Multicenter Stroke Survey); GRASPS (Glucose, Race, Age, Sex, Systolic blood Pressure, and Severity of stroke); SPAN-100 (stroke prognostication using age and NIH Stroke Scale−100 positive index).
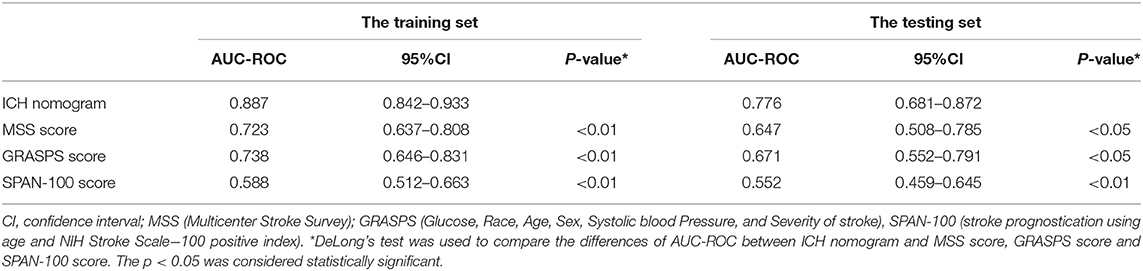
Table 4. The comparison of AUC-ROC of the ICH nomogram, MSS scores, and GRASPS scores for predicting the risk of ICH in the training set and testing set.
Figures 5A,B shows calibration plots for the training and testing sets. The calibration plot for the training set showed excellent concordance between the predicted probability of ICH and the actual observations; the mean absolute error was 0.024. The calibration plot for the testing set also showed optimal agreement; there, the mean absolute error was 0.036. As shown in Figure 6, DCA suggests that the threshold probabilities ranged from 2.5 to 57.8% in the training set and from 5.4 to 38.2% in the testing set, indicating that the nomogram is clinically useful.
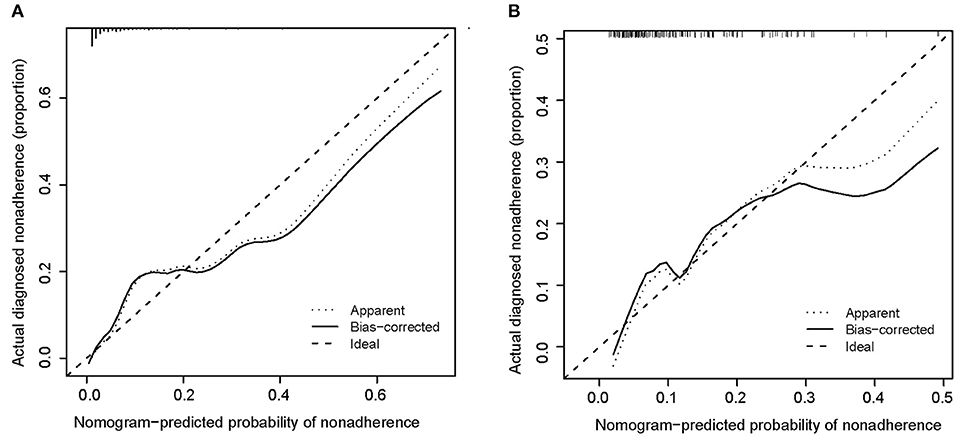
Figure 5. Calibration curve of the nomogram for the training set (A) and the testing set (B). Training set: B = 1,000 repetitions, boot, mean absolute error = 0.025, n = 387; testing set: B = 1,000 repetitions, boot, mean absolute error = 0.026, n = 166.
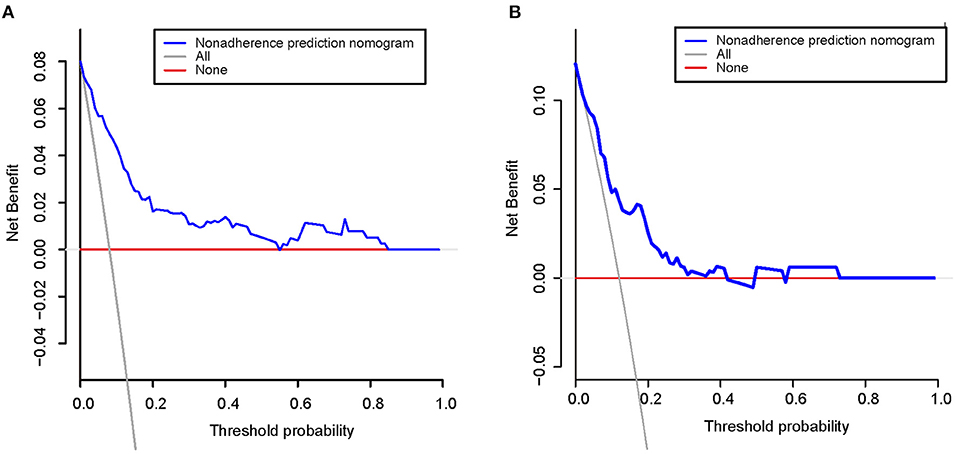
Figure 6. Decision curve analysis for the training set (A) and the testing set (B). A horizontal line indicates that all samples are negative and not treated, with a net benefit of zero. An oblique line indicates that all samples are positive. The net benefit is a backslash with a negative slope.
Discussion
We developed a new nomogram based upon smoking, NIHSS scores, BUN/Cr, and NLR for use in predicting the risk of ICH after IVT therapy. The developed nomogram demonstrated good discrimination and calibration in the training and testing sets. Furthermore, the AUC-ROC curve obtained using our nomogram showed better performance than the curves obtained using the MSS scores, the GRASPS scores, and the SPAN-100 score in both the training and testing sets. In addition, the DCA results suggested that the developed nomogram has a marked net benefit for predicting the risk of ICH.
Six scoring systems for predicting the risk of ICH in patients with AIS after IVT have been proposed. However, most of these scoring systems for individualized prediction of ICH are limited. The blood sugar, early infarct signs, hyperdense cerebral artery sign, age, NIH Stroke Scale (SEDAN) scores and the hemorrhage after thrombolysis (HAT) scores rely on CT images to detect early ischemic changes and hyperdense cerebral artery signs (6, 7). However, those scoring systems have some disadvantages. On the one hand, performing imaging tests is time-consuming, and this could delay the diagnosis and treatment of AIS with a high risk of ICH. On the other hand, hospitals in poor areas or poor communities lack imaging equipment, so these scores may not be suitable for use in patients in these situations. The Safe Implementation of Treatment in Stroke-Symptomatic IntraCerebral Hemorrhage (SITS-SICH) risk score requires data on the patient's history of antiplatelet therapy (8). Because we did not collect data on antiplatelet therapy in the present study, it is difficult to compare the performance of our nomogram with that of the SITS-SICH score. To assess the performance of the nomogram model compared to that of other scoring systems, the AUC-ROC curve was used to compare the prediction accuracy of our model with those of the MSS scores, the GRASPS scores, and the SPAN-100 scores (9, 10, 16). The AUC-ROC value of our predictive nomogram was superior to that of the MSS scores, the GRASPS scores, and the SPAN-100 scores in the training set and the testing set (p < 0.05). The better performance of our nomogram may be explained as follows. First, the three scoring systems with which it was compared converted continuous variables to dichotomization/categorization variables; this conversion is statistically inefficient and may decrease the accuracy of prediction (20). Second, the other scoring systems do not include inflammation-related indicators, such as neutrophils, lymphocytes, and NLR, despite the fact that several research studies have shown that the inflammatory response plays a key role in patients with AIS after IVT (21, 22). In addition, many studies have shown that renal dysfunction is related to the outcome of patients with AIS treated with IVT, but the other scoring systems do not take renal function into consideration (23–25).
In addition to the three scoring systems discussed above, three studies designed nomograms to predict the risk of ICH in AIS patients treated with rt-PA. STARTING-SICH (systolic blood pressure, age, onset-to-treatment time for thrombolysis, NIHSS score, glucose, aspirin alone, aspirin plus clopidogrel, anticoagulant with INR ≤1.7, current infarction sign, hyperdense artery sign) nomogram was constructed to predict ICH in stroke patients after IVT treatment in Italy (14). However, this nomogram has not been externally validated for Asian patients, for whom the risk of ICH is higher than for Caucasians. In addition, some of the risk factors included in the STARTING-SICH nomogram are not easily acquired on admission. The other two nomograms were developed in Asian populations (12, 17). Those nomograms take into account only demographic and clinical factors, such as hypertension, blood glucose, PLT, and NIHSS scores, and ignore some of the information that can be acquired from laboratory examination and may reflect risk factors for predicting ICH. In addition, in our study, the AUC-ROC value obtained using the nomogram constructed by Zhou (12) was 0.663 in the training set and 0.662 in the testing set. When the AUC-ROC is <0.700, the discriminative power of the test is not statistically significant (26–28). The other nomogram comprised three variables: AF, NIHSS scores, and glucose level (17). However, the number of cases in which this nomogram was evaluated was relatively small (n = 345). In addition, the percentage of ICH based on this nomogram was 14.5%, significantly higher than the percentage of ICH in Asian patients (29, 30). These factors reduce the credibility of this research.
In this study, smoking, NIHSS scores, BUN/Cr, and NLR were shown to be independent predictors of ICH in patients with AIS after rt-PA administration. Consistent with previous studies, smoking and NIHSS were common risk factors for predicting ICH. A previous study reported that age ≥68 years, smoking, AF, SP ≥149 mmHg 2 h after rt-PA administration, and NIHSS scores ≥17 before thrombolysis were associated with the risk of ICH after IVT (31). In Wang's study, smoking, prolongation of APTT, low fibrinogen levels, and low platelet counts were related to the risk of ICH (32). Another study also showed that age ≥70 years, cardioembolism, NIHSS ≥20, and serum glucose ≥9.0 mmol/L on admission were independent risk factors for predicting ICH in patients with AIS after rt-PA administration (33). However, few studies have focused on the BUN/Cr ratio or NLR for predicting the probability of ICH in patients with AIS after IVT. In fact, both BUN/Cr and NLR could affect the outcome of AIS. At present, BUN/Cr ≥15 is considered a biomarker of dehydration, especially in patients with normal kidney function (34). Lin's study demonstrated that BUN/Cr may be a novel predictor of early clinical deterioration in patients with AIS (35). Several studies suggested that dehydration status (BUN/Cr ratio ≥ 15) was a predictor of unfavorable long-term poor prognosis in patients with AIS treated with rt-PA (36, 37). A study showed that an increased BUN/Cr ratio indicated that patients suffered from acute kidney injury and acute heart failure (38, 39). At the same time, previous studies have demonstrated that impaired kidney function increases the risk of bleeding and hemorrhagic microangiopathy (40). Therefore, increased BUN/Cr might explain why the risk of ICH increases in AIS patients with IVT.
The NLR is another new risk factor we found that can contribute to the risk of ICH. Neuroinflammation is related to the entire pathological process of stroke. The NLR is a readily accessible and reproduces new inflammatory biomarker (41). It was reported that a high NLR (≥4.255) on admission increases the risk of ICH in patients with AIS after IVT (42). A recent meta-analysis also indicated that the NLR at admission is an independent risk factor for predicting hemorrhagic transformation after IVT (43). The underlying mechanism through which the NLR increases the risk of ICH in patients with AIS who receive IVT has not been elucidated. One reasonable explanation may be that the NLR affects the outcome because it is associated with the inflammatory destruction of neutrophils and a reduced protective effect of lymphocytes (44). Some studies have reported that peripheral neutrophils and T cells stimulated by tissue-type plasminogen activator (tPA) transmigrate to the brain vasculature, where they disrupt the blood-brain barrier (BBB) and accelerate ICH (45, 46).
Generally, the nomogram developed in this study, which includes four parameters, is convenient and efficient for use in the management of patients with AIS. First, the predictors used in our predictive nomogram are easily available at almost all medical centers, even those in poor areas. In addition, the nomogram is particularly suitable for use by non-neurologists because CT imaging is excluded. Furthermore, the discrimination and calibration performance of the nomogram was good; therefore, it could be a reliable tool for predicting the risk of ICH in patients with AIS after rt-PA treatment.
Our study has some limitations. First, our data came from a single-center retrospective analysis, and this might have limited the statistical power of the results. Second, our model has not been validated in external cohorts. Prospective, multicenter studies will be required in the future to assess the applicability of our nomogram. Third, data on onset-to-treatment time for thrombolysis or administration of oral antiplatelet drugs or anticoagulants, all of which may be risk factors for ICH, were not available in our study.
Conclusion
In conclusion, the new nomogram that includes smoking, NIHSS, BUN/Cr, and NLR may predict the risk of ICH after IVT in AIS patients in the Asian population.
Data Availability Statement
The raw data supporting the conclusions of this article will be made available by the authors, without undue reservation.
Ethics Statement
The studies involving human participants were reviewed and approved by the Ethics Committee of the Central Hospital of Shaoyang. Written informed consent for participation was not required for this study in accordance with the national legislation and the institutional requirements.
Author Contributions
A-DX, DL, Z-AW, and X-XH contributed to the study conception and design. Material preparation, data collection were performed by DD, Z-GY, S-YL, J-KZ, Y-FLi, Y-FLiu, Y-SW, T-YZ, and X-LS. Analysis and interpretation of the data were done by Z-AW. The first draft of the manuscript was written by Z-AW, X-XH, A-DX, and DL. All authors read and approved the final manuscript.
Funding
This work was supported by grants from the National Natural Science Foundation of China (81671167, 81971121, 81801150, and 82171316), the Science and Technology Planning Project of Guangdong Province, China (2017A020215049 and 2019A050513005), Natural Science Foundation of Guangdong Province (2018A0303130182 and 2020A1515010279), Postdoctoral Research Foundation of China (2018M643370 and 2021M701419), Guangzhou Science and Technology Planning Project (201508020004), Technology and People's Livelihood Major Project of Guangzhou (2014Y2-00505), and the Fundamental Research Funds for the Central Universities (21621102).
Conflict of Interest
The authors declare that the research was conducted in the absence of any commercial or financial relationships that could be construed as a potential conflict of interest.
Publisher's Note
All claims expressed in this article are solely those of the authors and do not necessarily represent those of their affiliated organizations, or those of the publisher, the editors and the reviewers. Any product that may be evaluated in this article, or claim that may be made by its manufacturer, is not guaranteed or endorsed by the publisher.
Acknowledgments
The authors thank the staff of the Department of Neurology and Stroke Center, The First Affiliated Hospital of Jinan University, and Clinical Neuroscience Institute of Jinan University, China.
Abbreviations
AF, atrial fibrillation; AIS, acute ischemic stroke; ALB, albumin; APTT, activated partial thromboplastin time; BUN/Cr, blood urea nitrogen-to-creatinine ratio; DBP, diastolic blood pressure; HDL, High-density lipoprotein; ICH, Intracranial hemorrhage; INR, International normalized ratio; IVT, intravenous thrombolysis; LASSO, The least absolute shrinkage and selection operator; LDL, low-density lipoprotein; NHISS, National Institute Health of Stroke Scale; NLR, neutrophil-to-lymphocyte ratio; PLT, blood platelet; SBP, systolic blood pressure; TC, total cholesterol; TG, triglyceride; UA, uric acid; WBC, white blood cell.
References
1. Hankey GJ. Secondary stroke prevention. Lancet Neurol. (2014) 2:178–94. doi: 10.1016/S1474-4422(13)70255-2
2. Chtaou N, Rachdi L, Midaoui AE, Souirti Z, Wahlgren N, Belahsen MF. Intravenous thrombolysis with rt-PA in stroke: experience of the moroccan stroke unit. Pan Afr Med J. (2016) 24:207. doi: 10.11604/pamj.2016.24.207.8815
3. Leng T, Xiong ZG. Treatment for ischemic stroke: From thrombolysis to thrombectomy and remaining challenges. Brain Circ. (2019) 1:8–11. doi: 10.4103/bc.bc_36_18
4. Yaghi S, Eisenberger A, Willey JZ. Symptomatic intracerebral hemorrhage in acute ischemic stroke after thrombolysis with intravenous recombinant tissue plasminogen activator: a review of natural history and treatment. JAMA Neurol. (2014) 9:1181–5. doi: 10.1001/jamaneurol.2014.1210
5. Zhao W, Wu C, Stone C, Ding Y, Ji X. Treatment of intracerebral hemorrhage: Current approaches and future directions. J Neurol Sci. (2020) 416:117020. doi: 10.1016/j.jns.2020.117020
6. Lou M, Safdar A, Mehdiratta M, Kumar S, Schlaug G, Caplan L, et al. The HAT Score: a simple grading scale for predicting hemorrhage after thrombolysis. Neurology. (2008) 18:1417–23. doi: 10.1212/01.wnl.0000330297.58334.dd
7. Strbian D, Engelter S, Michel P, Meretoja A, Sekoranja L, Ahlhelm FJ, et al. Symptomatic intracranial hemorrhage after stroke thrombolysis: the SEDAN score. Ann Neurol. (2012) 5:634–41. doi: 10.1002/ana.23546
8. Mazya M, Egido JA, Ford GA, Lees KR, Mikulik R, Toni D, et al. Predicting the risk of symptomatic intracerebral hemorrhage in ischemic stroke treated with intravenous alteplase: safe Implementation of Treatments in Stroke (SITS) symptomatic intracerebral hemorrhage risk score. Stroke. (2012) 6:1524–31. doi: 10.1161/STROKEAHA.111.644815
9. Saposnik G, Guzik AK, Reeves M, Ovbiagele B, Johnston SC. Stroke Prognostication using Age and NIH Stroke Scale: SPAN-100. Neurology. (2013) 1:21–8. doi: 10.1212/WNL.0b013e31827b1ace
10. Cucchiara B, Tanne D, Levine SR, Demchuk AM, Kasner S. A. Risk score to predict intracranial hemorrhage after recombinant tissue plasminogen activator for acute ischemic stroke. J Stroke Cerebrovasc Dis. (2008) 6:331–3. doi: 10.1016/j.jstrokecerebrovasdis.2008.03.012
11. Guo H, Xu W, Zhang X, Zhang S, Dai Z, Li S, et al. A nomogram to predict symptomatic intracranial hemorrhage after intravenous thrombolysis in chinese patients. Neuropsychiatr Dis Treat. (2021) 17:2183–90. doi: 10.2147/NDT.S320574
12. Zhou Z, Yin X, Niu Q, Liang S, Mu C, Zhang Y. Risk factors and a nomogram for predicting intracranial hemorrhage in stroke patients undergoing thrombolysis. Neuropsychiatr Dis Treat. (2020) 16:1189–97. doi: 10.2147/NDT.S250648
13. Wang A, Pednekar N, Lehrer R, Todo A, Sahni R, Marks S, Stiefel MF. DRAGON score predicts functional outcomes in acute ischemic stroke patients receiving both intravenous tissue plasminogen activator and endovascular therapy. Surg Neurol Int. (2017) 8:149. doi: 10.4103/2152-7806.210993
14. Cappellari M, Turcato G, Forlivesi S, Zivelonghi C, Bovi P, Bonetti B, et al. Nomogram to predict symptomatic intracerebral hemorrhage after intravenous thrombolysis for stroke. Stroke. (2018) 2:397–404. doi: 10.1161/STROKEAHA.117.018427
15. Cappellari M, Turcato G, Forlivesi S, Bagante F, Cervellin G, Lippi G, et al. The START nomogram for individualized prediction of the probability of unfavorable outcome after intravenous thrombolysis for stroke. Int J Stroke. (2018) 7:700–6. doi: 10.1177/1747493018765490
16. Menon BK, Saver JL, Prabhakaran S, Reeves M, Liang L, Olson DM, et al. Risk score for intracranial hemorrhage in patients with acute ischemic stroke treated with intravenous tissue-type plasminogen activator. Stroke. (2012) 9:2293–9. doi: 10.1161/STROKEAHA.112.660415
17. Yeo LLL, Chien SC, Lin JR, Liow CW, Lee JD, Peng TI, et al. Derivation and validation of a scoring system for intravenous tissue plasminogen activator use in Asian patients. J Stroke Cerebrovasc Dis. (2017) 8:1695–703. doi: 10.1016/j.jstrokecerebrovasdis.2017.03.033
18. Powers WJ, Rabinstein AA, Ackerson T, Adeoye OM, Bambakidis NC, Becker K, et al. Guidelines for the early management of patients with acute ischemic stroke: 2019 update to the 2018 guidelines for the early management of acute ischemic stroke: a guideline for healthcare professionals from the American Heart Association/American Stroke Association. Stroke. (2019) 12:e344–418. doi: 10.1161/STR.0000000000000211
19. Hacke W, Kaste M, Fieschi C, von Kummer R, Davalos A, Meier D, et al. Randomised double-blind placebo-controlled trial of thrombolytic therapy with intravenous alteplase in acute ischaemic stroke (ECASS II). Second European-Australasian Acute Stroke Study Investigators. Lancet. (1998) 9136:1245–51. doi: 10.1016/S0140-6736(98)08020-9
20. Alvarez-Sabin J, Maisterra O, Santamarina E, Kase CS. Factors influencing haemorrhagic transformation in ischaemic stroke. Lancet Neurol. (2013) 7:689–705. doi: 10.1016/S1474-4422(13)70055-3
21. Tiainen M, Meretoja A, Strbian D, Suvanto J, Curtze S, Lindsberg PJ, et al. Body temperature, blood infection parameters, and outcome of thrombolysis-treated ischemic stroke patients. Int J Stroke. (2013) 8:632–8. doi: 10.1111/ijs.12039
22. Chen J, Zhang Z, Chen L, Feng X, Hu W, Ge W, et al. Correlation of changes in leukocytes levels 24 hours after intravenous thrombolysis with prognosis in patients with acute ischemic stroke. J Stroke Cerebrovasc Dis. (2018) 10:2857–62. doi: 10.1016/j.jstrokecerebrovasdis.2018.06.014
23. Jung JM, Kim HJ, Ahn H, Ahn IM, Do Y, Choi JY, et al. Chronic kidney disease and intravenous thrombolysis in acute stroke: a systematic review and meta-analysis. J Neurol Sci. (2015) 358:345–50. doi: 10.1016/j.jns.2015.09.353
24. Zhu J, Shen X, Han C, Mei C, Zhou Y, Wang H, et al. Renal Dysfunction Associated with Symptomatic Intracranial Hemorrhage after Intravenous Thrombolysis. J Stroke Cerebrovasc Dis. (2019) 11:104363. doi: 10.1016/j.jstrokecerebrovasdis.2019.104363
25. Malhotra K, Katsanos AH, Goyal N, Tayal A, Gensicke H, Mitsias PD, et al. Intravenous thrombolysis in patients with chronic kidney disease: a systematic review and meta-analysis. Neurology. (2020) 2:e121–30. doi: 10.1212/WNL.0000000000009756
26. Aluizio CLS, Montes CG, Reis G, Nagasako CK. Risk stratification in acute variceal bleeding: far from an ideal score. Clinics (São Paulo). (2021) 76:e2921. doi: 10.6061/clinics/2021/e2921
27. Kisa NG, Kisa E, Cevik BE. Prediction of mortality in patients after oncologic gastrointestinal surgery: comparison of the ASA, APACHE II, and POSSUM Scoring Systems. Cureus. (2021) 3:e13684. doi: 10.7759/cureus.13684
28. Rau CS, Wu SC, Kuo SC, Pao-Jen K, Shiun-Yuan H, Chen YC, et al. Prediction of massive transfusion in trauma patients with shock index, modified shock index, and age shock index. Int J Environ Res Public Health. (2016) 13:683. doi: 10.3390/ijerph13070683
29. Chao AC, Liu CK, Chen CH, Lin HJ, Liu CH, Jeng JS, et al. Different doses of recombinant tissue-type plasminogen activator for acute stroke in Chinese patients. Stroke. (2014) 8:2359–65. doi: 10.1161/STROKEAHA.114.005245
30. Wu Y, Chen H, Liu X, Cai X, Kong Y, Wang H, et al. A new nomogram for individualized prediction of the probability of hemorrhagic transformation after intravenous thrombolysis for ischemic stroke patients. BMC Neurol. (2020) 1:426. doi: 10.1186/s12883-020-02002-w
31. Sun F, Liu H, Fu HX, Li CB, Geng XJ, Zhang XX, et al. Predictive factors of hemorrhage after thrombolysis in patients with acute ischemic stroke. Front Neurol. (2020) 11:551157. doi: 10.3389/fneur.2020.551157
32. Wang R, Zeng J, Wang F, Zhuang X, Chen X, Miao J. Risk factors of hemorrhagic transformation after intravenous thrombolysis with rt-PA in acute cerebral infarction. QJM. (2019) 5:323–6. doi: 10.1093/qjmed/hcy292
33. Liu M, Pan Y, Zhou L, Wang Y. Predictors of post-thrombolysis symptomatic intracranial hemorrhage in Chinese patients with acute ischemic stroke. PLoS ONE. (2017) 9:e0184646. doi: 10.1371/journal.pone.0184646
34. Gao B, Gu H, Yu W, Liu S, Zhou Q, Kang K, et al. Admission dehydration is associated with significantly lower in-hospital mortality after intracerebral hemorrhage. Front Neurol. (2021) 12:637001. doi: 10.3389/fneur.2021.637001
35. Lin LC, Yang JT, Weng HH, Hsiao CT, Lai SL, Fann WC. Predictors of early clinical deterioration after acute ischemic stroke. Am J Emerg Med. (2011) 6:577–81. doi: 10.1016/j.ajem.2009.12.019
36. Wu FF, Hung YC, Tsai YH, Yang JT, Lee TH, Liow CW, et al. The influence of dehydration on the prognosis of acute ischemic stroke for patients treated with tissue plasminogen activator. BMC Cardiovasc Disord. (2017) 1:154. doi: 10.1186/s12872-017-0590-6
37. Li SS, Yin MM, Zhou ZH, Chen HS. Dehydration is a strong predictor of long-term prognosis of thrombolysed patients with acute ischemic stroke. Brain Behav. (2017) 11:e00849. doi: 10.1002/brb3.849
38. Uchino S, Bellomo R, Goldsmith D. The meaning of the blood urea nitrogen/creatinine ratio in acute kidney injury. Clin Kidney J. (2012) 2:187–91. doi: 10.1093/ckj/sfs013
39. Matsue Y, van der Meer P, Damman K, Metra M, O'Connor CM, Ponikowski P, et al. Blood urea nitrogen-to-creatinine ratio in the general population and in patients with acute heart failure. Heart. (2017) 6:407–13. doi: 10.1136/heartjnl-2016-310112
40. Sohal AS, Gangji AS, Crowther MA, Treleaven D. Uremic bleeding: pathophysiology and clinical risk factors. Thromb Res. (2006) 3:417–22. doi: 10.1016/j.thromres.2005.03.032
41. Ma G, Pan Z, Kong L, Du G. Neuroinflammation in hemorrhagic transformation after tissue plasminogen activator thrombolysis: potential mechanisms, targets, therapeutic drugs and biomarkers. Int Immunopharmacol. (2021) 90:107216. doi: 10.1016/j.intimp.2020.107216
42. Liu YL, Lu JK, Yin HP, Xia PS, Qiu DH, Liang MQ, et al. High Neutrophil-to-Lymphocyte Ratio Predicts Hemorrhagic Transformation in Acute Ischemic Stroke Patients Treated with Intravenous Thrombolysis. Int J Hypertens. (2020) 2020:5980261. doi: 10.1155/2020/5980261
43. Wang C, Zhang Q, Ji M, Mang J, Xu Z. Prognostic value of the neutrophil-to-lymphocyte ratio in acute ischemic stroke patients treated with intravenous thrombolysis: a systematic review and meta-analysis. BMC Neurol. (2021) 1:191. doi: 10.1186/s12883-021-02222-8
44. Kim JY, Park J, Chang JY, Kim SH, Lee JE. Inflammation after ischemic stroke: the role of leukocytes and glial cells. Exp Neurobiol. (2016) 5:241–51. doi: 10.5607/en.2016.25.5.241
45. Shi K, Zou M, Jia DM, Shi S, Yang X, Liu Q, et al. tPA Mobilizes immune cells that exacerbate hemorrhagic transformation in stroke. Circ Res. (2021) 1:62–75. doi: 10.1161/CIRCRESAHA.120.317596
Keywords: acute ischemic stroke, intravenous thrombolysis, intracranial hemorrhage, nomogram, predictive model
Citation: Weng Z-A, Huang X-X, Deng D, Yang Z-G, Li S-Y, Zang J-K, Li Y-F, Liu Y-F, Wu Y-S, Zhang T-Y, Su X-L, Lu D and Xu A-D (2022) A New Nomogram for Predicting the Risk of Intracranial Hemorrhage in Acute Ischemic Stroke Patients After Intravenous Thrombolysis. Front. Neurol. 13:774654. doi: 10.3389/fneur.2022.774654
Received: 12 September 2021; Accepted: 07 February 2022;
Published: 10 March 2022.
Edited by:
Mirjam R. Heldner, University Hospital Bern, SwitzerlandReviewed by:
Wenbo Zhao, Capital Medical University, ChinaXunming Ji, Capital Medical University, China
Copyright © 2022 Weng, Huang, Deng, Yang, Li, Zang, Li, Liu, Wu, Zhang, Su, Lu and Xu. This is an open-access article distributed under the terms of the Creative Commons Attribution License (CC BY). The use, distribution or reproduction in other forums is permitted, provided the original author(s) and the copyright owner(s) are credited and that the original publication in this journal is cited, in accordance with accepted academic practice. No use, distribution or reproduction is permitted which does not comply with these terms.
*Correspondence: An-Ding Xu, tlil@jnu.edu.cn; Dan Lu, ludan@jnu.edu.cn
†These authors have contributed equally to this work and share first authorship