- Centre for Genetic Epidemiology, Institute for Clinical Epidemiology and Applied Biometry, University of Tübingen, Tübingen, Germany
Our aim was to determine whether the genetic liability to sleep and pain-related traits have a causal effect on risk of neurodegeneration in individuals of predominantly European ancestry. We selected five neurodegenerative disorders, namely, age-related macular degeneration (AMD), Alzheimer's disease (AD), amyotrophic lateral sclerosis (ALS), multiple sclerosis (MS), and Parkinson's disease (PD). Sleep duration (SD), short sleep (SS), long sleep (LS), chronotype (CHR), morning person (MP), insomnia (INS), and multisite chronic pain (MCP) were considered as exposures. We conducted Mendelian randomization (MR) using an inverse-variance weighted (IVW) method to compute causal effect estimates using latest available GWAS data sets. The MP phenotype was observed as the strongest risk factor for genetic liability to AMD (ORIVW = 1.192; 95% CI 1.078, 1.318, P = 0.0007). We observed suggestive evidence of risky effects of CHR on AMD (P = 0.0034), SS on AD (P = 0.0044), and INS on ALS (P = 0.0123). However, we failed to observe any role of pain. The results were robust on sensitivity analyses. Our study highlighted the role of MP as a risk factor for AMD.
Introduction
Patients with neurodegenerative diseases (NDDs) often experience disruptions in circadian rhythmic activities (1, 2). Many patients with NDD and circadian disruptions also complain of painful symptoms of variable origins and intensities (3). Both sleep and pain could often be treated and, thereby, can help maintain a stable quality of life in the absence of any disease-modifying treatment for NDDs (4). A greater understanding of the etiological relationship between sleep, pain, and neurodegeneration could, thereby, enable better management of NDDs.
It is well-recognized that circadian dysfunction in old age is due to degeneration of the suprachiasmatic nucleus (SCN) in the anterior hypothalamus, directly connected to the light-sensing retina (5). Different NDDs further exhibit marked heterogeneity in manifestation of circadian disruptions, which could be attributed to loss of different neuronal subpopulations in the SCN. Clinically, patients with Alzheimer's disease (AD) often show sleep-wake rhythm disorder, and patients with PD show a reduction in the amplitude of the circadian rhythm (6, 7). A limited number of longitudinal studies have demonstrated the potential influence of circadian disruptions on predisposition to AD, PD, and related markers of neurodegeneration (8, 9).
Similar to the involvement of specific brain regions directly influencing circadian rhythms, several brain regions, also referred to as pain matrix, have been shown to be activated during pain perception (10, 11). The pain matrix comprising the primary (S1) and secondary (S2) somatosensory cortices, insula, anterior cingulate cortex (ACC), amygdala, prefrontal cortex (PFC), and thalamus, further shows differential activation during acute and chronic pain (12). Aging is specifically known to increase the likelihood of chronic pain and may amplify the neurodegeneration process (13, 14).
To date, the sparse number of large longitudinal studies and clinical trials has limited our progress in understanding the relationship between sleep, pain, and onset or progression of neurodegeneration, necessitating the need for searching alternative approaches for judging the causality. A two-sample Mendelian randomization (MR) is one such approach that employs instruments or proxy markers of risk factor in one population to judge causality of the risk factor with an outcome in an independent population (15–17).
So far, limited studies have employed a genetic instrument-based approach to judge the etiological relationship between sleep, pain, and NDDs. A recent MR study showed an absence of the role of genetic liability to sleep duration (SD) in influencing predisposition to AD (18). On the contrary, another report showed an association of genetic liability with sleep efficiency with AD (19). A couple of studies showed increased risk of ALS due to daytime sleepiness (19, 20). Considering the highly varied role of various behavioral biomarkers of circadian rhythm on neurodegeneration and potential overlapping etiology of sleep and pain, we adopted a highly comprehensive approach by exploiting the availability of genetic instruments for various markers of circadian rhythm, namely, SD (21), short sleep (SS) (21), long sleep (LS) (21), chronotype (CHR) (22), morning person (MP) (22), insomnia (INS) (23), and multisite chronic pain (MCP) (24), and NDDs, namely, AD (25), AMD (26, 27), ALS (28), MS (29), and PD (30, 31) to dissect the bi-directional relationship between sleep, pain, and neurodegeneration using two-sample MR approach.
Methods
Identification and Correlation Among Data Sets
We employed a two-sample MR study design using summary estimates to examine the lifelong effect of sleep and pain-related traits on genetic liability to neurodegeneration in European populations. We used latest available discovery cohorts of meta-analyses of GWAS data sets in the literature. We identified single nucleotide polymorphisms (SNPs) that influence circadian rhythm-related traits, including SD (21), SS (21), LS (21), CHR (22), MP (22), INS (23), and MCP (24) (Table 1). We adopted a P cutoff of 5 × 10−8 to select the genetic instruments. Concerning the outcome data sets, we used the discovery cohort of a recent meta-analysis of GWAS on AD (25), AMD (26), ALS (28), MS (29), and PD (30). Before judging the causal role of sleep and pain in predisposition to PD, we checked for any potential correlation between different sleep and pain-related traits and different NDDs. We specifically employed a cross-trait LD score regression (LDSC) method to evaluate the genome-wide correlation between traits (https://github.com/bulik/ldsc) (32).
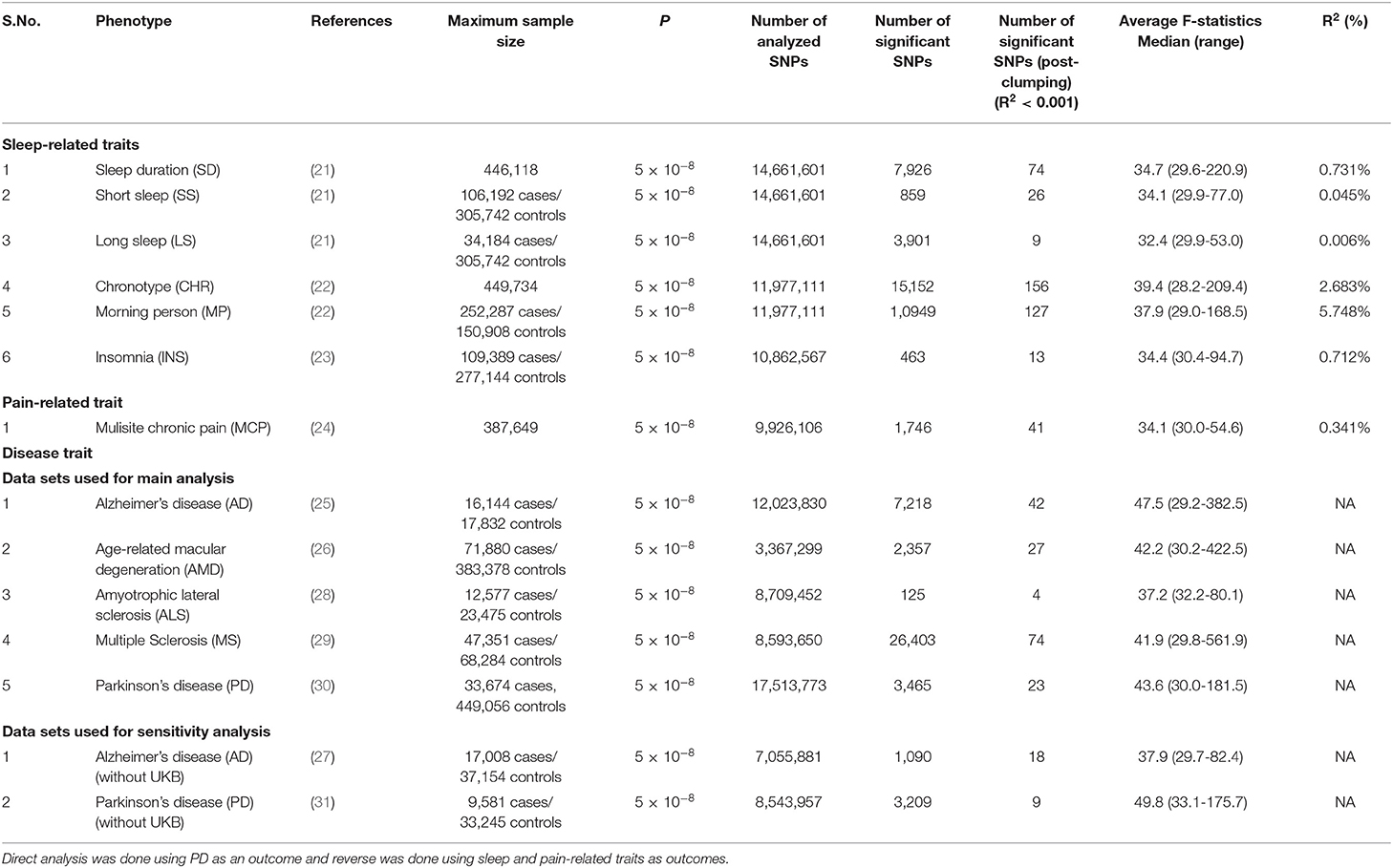
Table 1. Details of discovery GWAS datasets and prioritized instruments used for direct and reverse causal analysis in the present study.
Since the study analyzed secondary data (publicly available data) that contained information at the population-level (summary-level data), informed consent and ethical approval were waived off for this study.
Causal Effect Estimation
The prioritized SNP IDs and positions were synchronized with the NCBI GRCh37 assembly. We further checked for the validity of MR assumptions by excluding SNPs with F-statistics <10 and loci known to be directly involved in neurodegeneration based on existing evidence from previously published literature.
As the selected genetic instruments could be correlated, we performed clumping of significantly associated SNPs on each GWAS data set with the clump_data function of the TwoSampleMR package (version 0.4.25) in R (version 3.6.1). We employed a clumping window of 10,000 kb and linkage disequilibrium (LD; i.e., r2) cutoff of 0.001, and used the European population in the 1,000 Genome Phase 3v5 data set to identify the leading SNPs.
The leading SNPs were further checked for availability in the respective outcome data sets. When possible, if a specific SNP was not available, a proxy SNP (r2 > 0.8) was used. We further computed the pooled variance (R2) for the respective risk factor using effect estimates (βx) and effect allele frequencies (EAFs) of individual genetic instruments, i.e., R2 = 2*EAF*(1–EAF). Detectable risky and protective effect estimates at 80% power were computed for each NDD as an outcome at various pooled variances explained by the genetic instruments (ranging from 0.25 to 7.5%) using the Mendelian Randomisation Power Calculator (http://cnsgenomics.com/shiny/mRnd). To compute the effect estimates at specific variance for a given outcome, we employed a sample size of each outcome data set, the proportion of patients in the same data set, and a threshold P of 1.42 × 10−3.
We used the inverse variance-weighted (IVW) effect method as the primary method to compute the causal effect estimates, as used previously (17). We computed the causal estimates as odds ratio (OR) per unit of standard deviation (SD) for continuous traits and ORs for the outcome per unit log-odds of categorical traits. We employed a conservative Bonferroni correction of the significance level to account for 35 independent tests, including forward and reverse MR (threshold P = 1.42 × 10−3, i.e., 0.05/35). Heterogeneity was judged using the Cochran's Q-statistic and I2 for the IVW method along with Rucker's Q-statistic, and the intercept deviation test for the MR-Egger's method (17). All the scripts used for the primary MR analysis have been provided as part of the a R-based mrpipeline package (https://github.com/CGEatTuebingen/mrpipeline). We used a previously published data set to replicate the findings before employing the package for to this study (17). The mrpipeline package is currently under the developmental phase, with a plan to integrate external databases, including GWAS and tissue expression repositories in the future. We also performed an Mendelian Randomization Pleiotropy RESidual Sum and Outlier (MR-PRESSO) global test to evaluate horizontal pleiotropy (33). Lastly, we performed an MR Steiger test of directionality to validate the assumption that a given exposure causes an outcome using the TwoSampleMR package (version 0.4.25) in R (version 3.6.1).
Sensitivity Analysis
Several approaches were employed to rule out the influence of potential pleiotropic variants on the overall results. We used multiple modern MR methods, including the MR-Egger, weighted median (WME), and weighted mode (MBE) methods, to check the reliability of the estimates, as used in previous studies (16, 17). Since most of the recent meta-analyses of GWAS compute effect estimates by pooling UK Biobank (UKB) data sets with previously available data sets, and the existence of any overlapping samples in exposure and outcome datasets could bias the effect estimates toward the confounded observational estimates, we also used the NDD datasets without UKB samples, when required (27, 31). We conducted MR in the reverse direction to check and confirm the directionality of the observed associations.
We further employed a leave-one-out and leave-one-group-out cross-validation approach to rule out the influence of outlier variants known to be associated with confounders of the relationship between the respective exposure and outcome data sets. We specifically employed the Phenoscanner database (http://phenoscanner.medschl.cam.ac.uk) to identify genetic variants associated with potential confounders. However, in the absence of knowledge of potential confounders, we adopted a more conservative approach, and all genetic loci known to be associated with non-sleep-related traits were assumed to be pleiotropic loci. We identified such loci by searching for all genetic variants in high LD with genetic instruments prioritized for this study using r2 > 0.9 for previously reported associations in European populations. We used visual approaches, including scatter plots and funnel plots, to identify outlier variants.
We also performed a sensitivity analysis by adjusting for potential confounders using a multivariable MR method. As and when appropriate, we adjusted for quantity of sleep, sleep preference for a given time of day, and pain, the phenotypes of interest investigated in this study. As multiple, highly correlated, and overlapping traits representing both quantity of sleep (LS, SS, INS, and SD) and sleep preference (CHR, MP) were available, we performed a variable selection procedure to select the optimal variable that represented each category. Such an approach prevented us from conducting an overadjustment and avoided loss of power inherent with multiple variable regression methods. We selected SD to adjust for the quantity of sleep, as a continuous variable is more informative than a binary trait. Similarly, we selected CHR as a variable of choice representing sleep preference. Specifically, the genetic associations of instruments with respective NDDs were regressed on the genetic associations with all the risk factors (SD, sleep pattern, and pain) in a single regression model using IVW method. Genetic instruments entered into the multivariable regression model were allowed to be associated with any of the risk factor under consideration.
We further evaluated the potential biological influence of different brain regions on their respective contribution to the causal effect estimate by analyzing gene expression data for available genetic variants from the Genotype-Tissue Expression Project (https://www.gtexportal.org).
Results
Identification and Correlation Among Data Sets
Details of discovery GWAS data sets used for the causal analysis in this study are shown in Table 1. The minimum number of individuals available for a specific NDD ranged from 12,557 ALS cases to 71,880 AD cases, which are broadly in consensus with their respective prevalence.
The pairwise genetic correlation analysis of complete GWAS data sets failed to show correlation of any of the NDDs with sleep or pain-related traits (Supplementary Table 1). Expectedly, a highly significant correlation was observed among the traits representing SD (SS, LS, SD, and INS) and among those representing sleep pattern (CHR and MP). Notably, MCP was strongly correlated with all the markers of SD (rg ranging from 0.28 for LS to 0.59 for INS), suggesting a need for conducting a multivariable analysis adjusting for MCP when judging the independent association of sleep markers with NDDs or vice versa.
Causal Effect Estimation
The genetic instruments were identified that influence sleep and pain-related traits through latest publicly available meta-analysis of GWAS summary datasets (Table 1). Overall, we identified 771 genetic instruments to check the bidirectional causality between sleep, pain, and neurodegeneration, with F-statistic for individual SNPs ranging from 28.2 to 422.5. The detectable effect estimates for different NDDs as outcomes at 80% power and a type-1 error rate of 1.42 × 10−3 are further shown in Supplementary Table 2.
The data used for computation of causal effect estimates are provided in Supplementary Table 3. The causal effect estimates using various MR approaches and heterogeneity analysis measures used to judge the robustness of the estimates are provided in Table 2 for the direct causal estimates for NDDs as outcomes. We observed a highly significant causal effect of MP on genetic liability to AMD (ORIVW = 1.192; 95% CI 1.078, 1.318, P = 0.0007). Heterogeneity check confirmed the reliability of the observed association with absence of any heterogeneity in the distribution of effect estimates of individual genetic variants (I2 = 0.0%, Cochran's Q-test P = 0.9288, Rucker's Q-test P = 0.9414, MR-PRESSO global test P = 0.8420). The distribution of individual SNP-level effect estimates and the effect estimates computed with different MR methods for the effect of MP on AMD is further shown as scatter and funnel plots in Figure 1. We observed a similar directionality of causal effect estimates using the WME method (ORWME = 1.126; 95% CI = 1.044, 1.214). We also observed a similar trend using a highly correlated but continuous trait, CHR on AMD (ORIVW = 1.269; 95% CI 1.083, 1.486, P = 0.0034). The directionality of findings was further confirmed by a significantly higher variance explained by genetic instruments for MP and CHR than that explained by the respective genetic instruments for AMD (PSteiger = 2.1 × 10−98 and PSteiger = 1.65 × 10−24). In contrast, we did not observe any direct role of pain on predisposition to AMD.
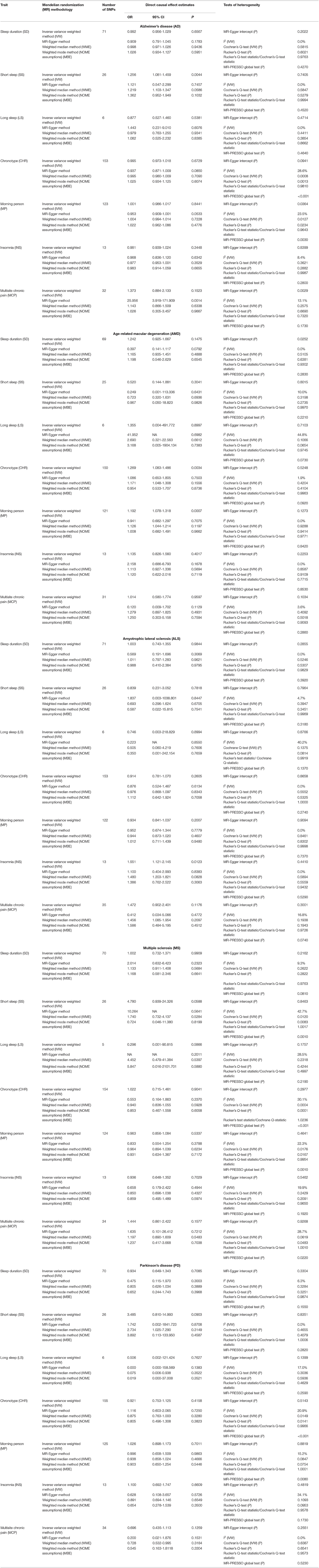
Table 2. Causal effect estimates using different Mendelian randomization (MR) methods and heterogeneity analysis of causal effect estimates for neurodegeneratice disorders (NDDs) using various sleep and pain-related traits as exposures.
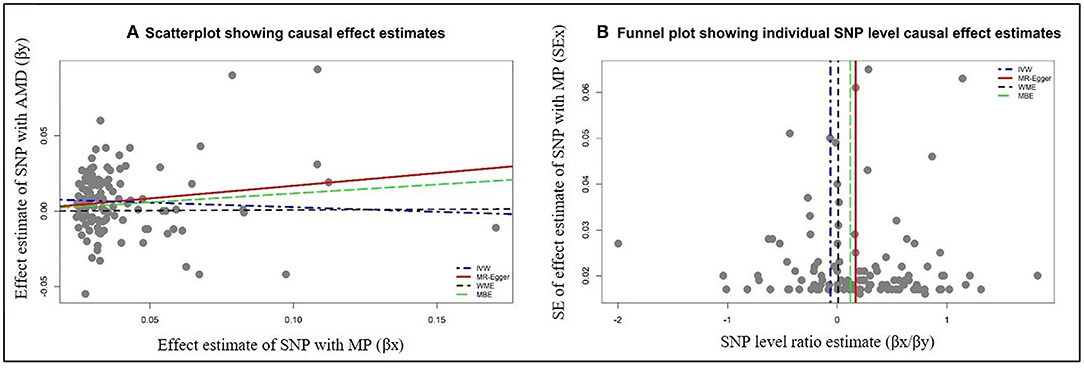
Figure 1. Graphical representation of causal association analysis and assessment of pleiotropy. (A) Scatterplot showing causal effect estimates computed using various MR methods for the association of morning person (MP) as exposure with age-related macular degeneration (AMD) as outcome. (B) Funnel plot showing the extent of heterogeneity among the individual Wald ratio estimates for morning person (MP) as exposure with age-related macular degeneration (AMD) as outcome. IVW, inverse variance-weighted method; WME, weighted median method; MBE, weighted mode method (NOME assumptions).
We further observed a suggestive risky causal effect of SS on genetic liability to AD (ORIVW = 1.256; 95% CI 1.081, 1.459, P = 0.0044). Heterogeneity check further confirmed the reliability of the observed association with absence of any heterogeneity in the distribution of effect estimates of individual genetic variants (I2 = 0%, Cochrane P =0.5847, Rucker's Q-test P = 0.5279, MR-PRESSO global test P = 0.4270). A similar directionality in the causal effect estimates was also observed using the WME method (OR = 1.121; 95% CI 1.103, 1.347). However, we did not observe any role of pain in predisposition to AD.
We also observed a suggestive risky causal effect of INS on genetic liability to ALS (ORIVW = 1.551; 95% CI 1.121, 2.145, P = 0.0123). On the other hand, we failed to observe any role of pain in predisposition to ALS.
We did not observe any direct role of sleep and pain-related traits in predisposition to MS. Similarly, our MR analysis failed to detect a role of the sleep and pain-related traits in predisposition to PD.
Sensitivity Analysis
Concerning direct MR, the association of SS with AD was lost after the exclusion of overlapping UKB samples (data not shown). In the reverse MR, PD showed suggestion of a strong protective effect against CHR and MP after the exclusion of overlapping UKB samples (data not shown). Reverse causal estimates for various sleep and pain-related traits using various NDDs as exposure are shown in Table 3. Our reverse casual check confirmed the directionality of the observed associations of MP and CHR with AMD, as we failed to observe any effect of AMD on MP and CHR. Our reverse causal check also confirmed the role of SS in predisposition to AD, as we failed to observe the causal effect of AD on SS. Interestingly, all the sleep-related traits except for SS were observed to be influenced by genetic predisposition to AD when employing non-IVW methods for judging causal effects of sleep-related traits on AD. Lastly, our reverse casual check confirmed the role of INS in predisposition to ALS. On the contrary, our findings suggested a causal role of genetic predisposition to ALS in LS with a consistent significant risk effect using the IVW, WME, and MBE methods.
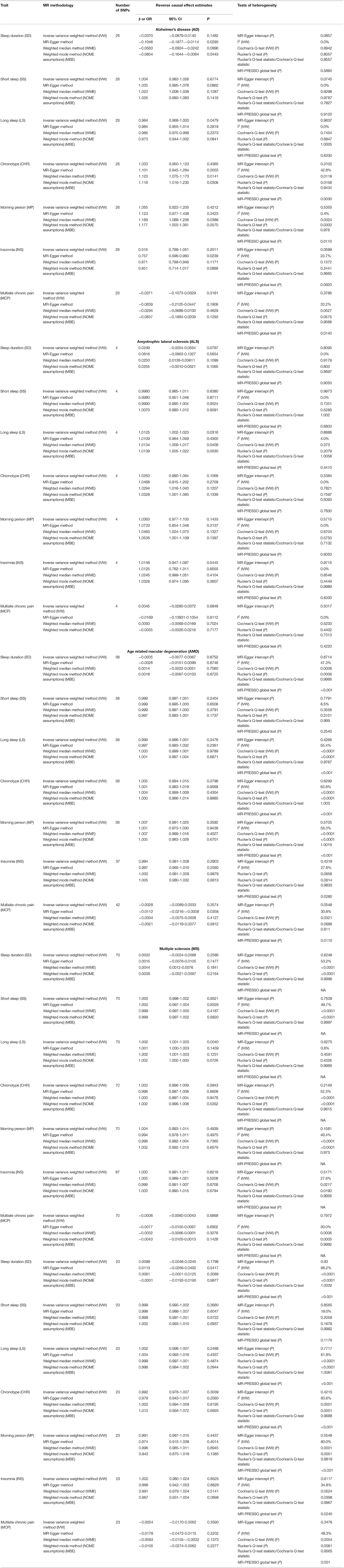
Table 3. Causal effect estimates using different Mendelian randomization methods and heterogeneity analysis of causal effect estimates for various sleep and pain-related traits using Neurodegenerative disorders (NDDs) asexposures.
We failed to observe the predominant influence of any of the single variants on causal the effect estimates of MP with AMD, as shown in Supplementary Table 4. Similarly, the observed associations of CHR with AMD, SS with AD, and INS with ALS were retained (Supplementary Table 5). Among SNPs used for causal effect estimation of MP and CHR with AMD, 46 and 51 were identified as potential pleitropic variants for respective estimations (Supplementary Table 6). However, exclusion of these SNPs did not influence the observed casual association of MP and CHR with AMD (OR = 1.202, 95% CI 1.055, 1.370; OR = 1.262, 95% CI 1.049, 1.520). On the contrary, associations of SS with AD and INS with ALS were lost, which could be attributed to the presence of a high proportion of pleiotropic SNPs in the genetic instruments for SS and INS.
The sensitivity analysis using the multivariable MR approach also yielded similar results with the retention of the association of MP and CHR with AMD (OR = 1.184, 95% CI 1.083, 1.284; OR = 1.162, 95% CI 1.060, 1.263) (Supplementary Table 7).
Concerning the influence of specific brain regions, we specifically identified a high proportion of SNPs influencing brain expression in the cerebellum and basal ganglia region (Table 4). However, exclusion of these SNPs did not affect the overall causal association of CHR and MP with AMD. Similarly, we failed to observe the effect of any of the other brain regions on the observed associations. We also failed to observe any influence of brain region-specific expression on other observed associations (data not shown).
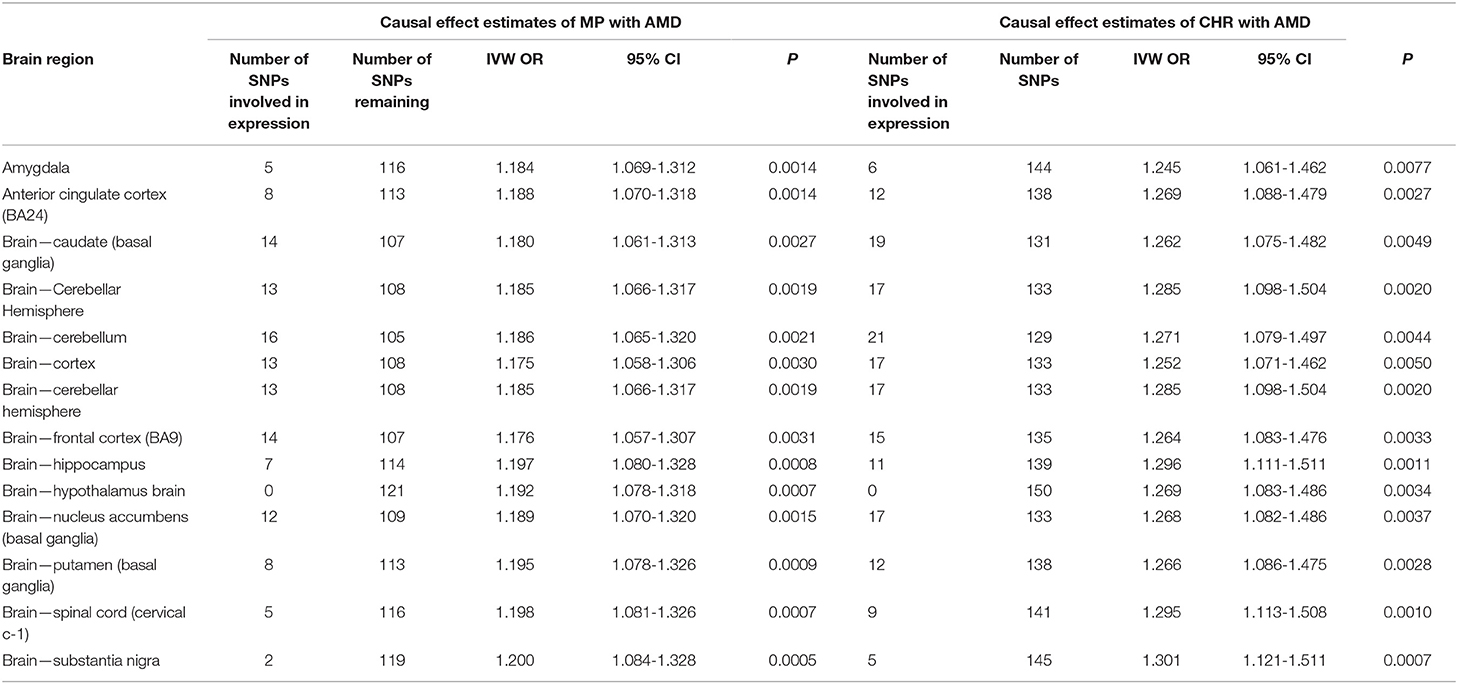
Table 4. Sensitivity analysis of causal effect estimates of sleep-related traits on neurodegeneration by exploring potential influence of specific brain region using variants involved in regional expression.
Discussion
The use of GWAS data in MR-based approaches has opened up opportunities to assess and define clinically relevant signatures for a diverse spectrum of diseases. Our study supports the role of a person's underlying circadian rhythm in genetic predisposition to neurodegeneration. We found an association of genetically predicted MP trait with AMD. The correlated trait CHR also had a suggestive risk association with AMD. We also found suggestive evidence for a possible association of genetically predicted SS with AD, and INS with ALS. Surprisingly, however, our study found no evidence to support the association between pain and NDDs.
To date, evidence from observational studies has shown a remarkable heterogeneity in the association of different circadian traits with various NDDs. A recent study investigating the incidence of AMD in 108,225 participants observed that patients with INS were 33% more likely to have subsequent AMD (HR 1.33; 95% CI 1.18, 1.48) (34). Previously, an observational study on 57 patients with neovascular AMD and 108 controls found a significantly increased risk of neovascular AMD in patients sleeping <6 h compared to those sleeping 7-8 h (OR 3.29; 95% CI 1.32, 8.27) (35). Another study failed to detect an association with LS in 316 patients with neovascular AMD compared to 500 patients without AMD (36). However, the study did find an association of LS with geographic atrophy, an advanced form of AMD, in 61 individuals (presence of a discrete area of atrophy with a diameter of ≥175 μm). A recent observational study further reported that individuals who take an afternoon nap are 60% less likely to be diagnosed with late AMD (56 with late AMD vs. 1,204 without AMD) (37). As darkness is known to stimulate the secretion of melatonin from the pineal gland, our findings are in agreement with previous studies showing that increased melatonin synthesis could play a protective role in the pathophysiology of AMD (38). However, a recent randomized controlled trial (RCT) failed to show any beneficial effect of low-level night-time light therapy on the progression of AMD (39).
In contrast to previously reported findings from epidemiological studies, we failed to observe any association of INS, SS, and LS with AMD using the genetic data in this study. However, we observed that MP is more likely to be predisposed to AMD (OR 1.19; 95% CI 1.08, 1.32). Our study suggests that more prolonged exposure to daylight in such individuals could increase the risk for AMD. Our findings are in contrast to a recent meta-analysis of observational studies demonstrating the absence of an association between sunlight exposure and AMD (OR 1.12; 95% CI 0.76, 1.67) (40). One of the possible reasons for this discrepancy could be that only one of the 14 studies included in the meta-analysis was a cohort study. The only included cohort study was a 10-year follow-up study, which demonstrated that participants exposed to summer sun for more than 5 h a day were more likely to show increased retinal pigment (RR 2.99; 95% CI 1.18, 7.6) and develop early age-related maculopathy (RR 2.2; 95% CI 1.02, 4.73) in comparison to those exposed for <2 h per day (41). It has also been suggested that excessive light exposure may induce phototoxic damage to the retinal pigmental epithelium and possibly contribute to the gradual worsening of vision in AMD (42–44).
Compared to the impact of circadian rhythms on other NDDs, the role of sleep-related traits has been well-investigated in AD but with mixed findings. Previous studies have predominantly focused on sleep-wake rhythmicity, showing higher incidence of sleep fragmentations and lower amplitude of circadian rhythmicity in patients with moderate or severe AD (1). Concerning SD, both LS and SS have been previously shown to be linked with the risk of dementia (8, 45, 46). A 17-year longitudinal study investigating sleep characteristics in 11, 247 old-aged Swedish individuals (> 65 years at baseline) observed an association of short (≤ 6 h) and extended (> 9 h) time in bed with a higher incidence of dementia (HR 1.4, 95% CI 1.06, 1.85; HR 1.11, 95% CI 1, 1.24) (8). Our results are in agreement with a previously published study (8). Indeed, we observed a strong causal role of SS in predisposition to AD (OR 1.26; 95% CI 1.08, 1.46). However, our results need to be treated with caution, as the association was lost after excluding the overlapping UKB samples from the AD data set, as demonstrated previously (18). It is also possible that the association was lost because of decrease in sample size, necessitating replication with larger AD data sets in the future.
Sleep disturbances are also frequently observed in patients with ALS. Our MR analysis also suggested a possible causal role of INS in ALS (OR 1.55; 95% CI 1.12, 2.14). A previous observational study has demonstrated decreased sleep efficiency and fragmented sleep architecture in 59 patients with ALS (47). Another study reported the presence of sleep disturbances in more than 2/3 of 40 patients with ALS. The study further reported a diagnosis of INS in 65% of the patients (48). These results are in agreement with a previous study reporting a significantly higher prevalence of INS in 90 patients with motor neuron disease compared to 96 healthy controls (48.9 vs. 31.3%, p = 0.014) (49). In summary, reports of sleep disturbance among patients with ALS in small sample-sized observational studies and the suggestive causal role of INS in ALS in this study necessitate a need for conducting large-scale epidemiological studies.
Despite the consistent findings of excessive daytime sleepiness or altered sleep timing in patients with PD, our MR findings demonstrate the absence of any causal role of sleep-related traits in predisposition to PD (1). One possible explanation could be that dopaminergic treatment might have influenced the sleeping behavior of patients with PD, as excessive daytime sleepiness is known to be one of the common side effects of dopaminergic treatment. In such a scenario, causal analysis using biological markers of circadian rhythms such as core body temperature, cortisol, and melatonin rhythms, might potentially shed light on the true relationship between sleep-related traits and PD.
We also failed to observe any causal association of sleep-related traits with MS, although sleep disturbance is a common symptom of MS (1). It is suggested that the sleep disorders observed in patients with MS could be a secondary cause of fatigue, a symptom that affects 9 of 10 patients with MS (50).
Among all NDDs, high prevalence of pain has been observed in patients with AD and PD (1). Assessment of pain in such patients of is often challenging because of associated cognitive and motor impairments (51). Nevertheless, the use of genetic instruments of pain on a general population shows that MCP does not play any causal role in AD and PD. A recent cross-sectional study investigating pain in 100 patients with PD patients showed that pain is more prevalent in patients with advanced-stage PD than patients with early-stage PD suggesting pain to be a consequence of the disease rather than a cause (52). Moreover, pain is a broad concept, and inconsistencies in the measurement of number of available pain behavior rating scales often limit their application in clinical settings.
Our study has several strengths and limitations. We adopted a highly comprehensive approach involving the exploration of several sleep-related traits and pain with commonly prevalent NDD. We further employed multiple MR methods and heterogeneity and sensitivity analysis approaches to confirm the reliability of the observed associations. Concerning limitations, previous observational studies have shown that the impact of sleep and pain-related traits may be dependent on the stage of neurodegeneration or severity of an NDD (2). However, we could not conduct such a stratified analysis because of the non-availability of an individual-level data set for respective NDD. Furthermore, pain is a highly complex trait, and the lack of genetic instruments specific for neuropathic and nociceptive pain may undermine the findings of this study. The possibility of nociceptive pain confounding the causal relationship between neuropathic pain and neurodegeneration cannot be ruled out. One critical assumption for MR is that the effect of a genetic instrument for the main exposure on disease outcome is mediated by its influence on the intermediate trait. As genetic variants associated with sleep (duration or pattern) are highly correlated with pain and other sleep-related traits (duration or pattern), we addressed the potential pleiotropic effect by conducting a multivariable analysis. Our findings of causal association between sleep pattern (CHR or MP) and AMD remained robust after adjusting for the potential pleiotropic effect of SD and pain. However, despite adopting a multivariable MR approach, the possibility of residual confounding due to our inability to simultaneously adjust for all the highly correlated SD-related traits (SD or LS or SS) cannot be ruled out.
Using genetic data, we provide strong evidence that being an MP is a causal risk factor for genetic liability to AMD. There is a necessity for conducting large-scale epidemiological cohort studies to confirm our findings. Additional research is also required to understand the biological pathways underlying these associations, including causal analysis with biochemical makers of sleep and correlated traits associated with sleep.
Data Availability Statement
The original contributions presented in the study are included in the article/Supplementary Material, further inquiries can be directed to the corresponding author.
Ethics Statement
Ethical review and approval was not required for the study on human participants in accordance with the local legislation and institutional requirements. Written informed consent from the participants' legal guardian/next of kin was not required to participate in this study in accordance with the national legislation and the institutional requirements.
Author Contributions
SG designed and conceptualized the study, conducted data extraction, analyzed the data, drafted the manuscript, and revised the final draft. MS supervised the overall study and revised the final draft. Both authors contributed to the article and approved the submitted version.
Funding
This study was, in part, supported by the EU Joint Programme-Neurodegenerative Diseases Research (JPND) project under the aegis of JPND (www.jpnd.eu) through Germany, BMBF, funding code 01ED1406. MS was further funded by the Michael J. Fox Foundation, USA Genetic Diversity in PD Program: GAP-India Grant ID: 17473 and supported by grants from the German Research Council (DFG/SH 599/6-1 to MS), and MSA Coalition.
Conflict of Interest
The authors declare that the research was conducted in the absence of any commercial or financial relationships that could be construed as a potential conflict of interest.
Publisher's Note
All claims expressed in this article are solely those of the authors and do not necessarily represent those of their affiliated organizations, or those of the publisher, the editors and the reviewers. Any product that may be evaluated in this article, or claim that may be made by its manufacturer, is not guaranteed or endorsed by the publisher.
Acknowledgments
We acknowledge the study participants and the investigators from the International Genomics of Alzheimer's Patients (IGAP), International Parkinson's Disease Genomics Consortium (IPDGC), project MinE, International Multiple Sclerosis Genetics Consortium (IMSGC), International Sleep Genetic Epidemiology Consortium (ISGEC), UK Biobank, and UK Biobank sleep and chronotype research group for sharing the summary statistics in their GWAS data sets. We also acknowledge UK biobank resources under application number 65949.
Supplementary Material
The Supplementary Material for this article can be found online at: https://www.frontiersin.org/articles/10.3389/fneur.2022.765321/full#supplementary-material
References
1. Leng Y, Musiek ES, Hu K, Cappuccio FP, Yaffe K. Association between circadian rhythms and neurodegenerative diseases. Lancet Neurol. (2019) 18:307–18. doi: 10.1016/S1474-4422(18)30461-7
2. Videnovic A, Lazar AS, Barker RA, Overeem S. The clocks that time us–circadian rhythms in neurodegenerative disorders. Nat Rev Neurol. (2014) 10:683–93. doi: 10.1038/nrneurol.2014.206
3. de Tommaso M, Arendt-Nielsen L, Defrin R, Kunz M, Pickering G, Valeriani M. Pain in neurodegenerative disease: current knowledge and future perspectives. Behav Neurol. (2016) 2016:7576292. doi: 10.1155/2016/7576292
4. Feustel AC, MacPherson A, Fergusson DA, Kieburtz K, Kimmelman J. Risks and benefits of unapproved disease-modifying treatments for neurodegenerative disease. Neurology. (2020) 94:e1–14. doi: 10.1212/WNL.0000000000008699
5. Herzog ED. Neurons and networks in daily rhythms. Nat Rev Neurosci. (2007) 8:790–802. doi: 10.1038/nrn2215
6. Hooghiemstra AM, Eggermont LH, Scheltens P, van der Flier WM, Scherder EJ. The rest-activity rhythm and physical activity in early-onset dementia. Alzheimer Dis Assoc Disord. (2015) 29:45–9. doi: 10.1097/WAD.0000000000000037
7. Videnovic A, Noble C, Reid KJ, Peng J, Turek FW, Marconi A, et al. Circadian melatonin rhythm and excessive daytime sleepiness in Parkinson disease. JAMA Neurol. (2014) 71:463–9. doi: 10.1001/jamaneurol.2013.6239
8. Bokenberger K, Ström P, Dahl Aslan AK, Johansson AL, Gatz M, Pedersen NL, et al. Association between sleep characteristics and incident dementia accounting for baseline cognitive status: a prospective population-based study. J Gerontol A Biol Sci Med Sci. (2017) 72:134–9. doi: 10.1093/gerona/glw127
9. Leng Y, Goldman SM, Cawthon PM, Stone KL, Ancoli-Israel S, Yaffe K. Excessive daytime sleepiness, objective napping and 11-year risk of Parkinson's disease in older men. Int J Epidemiol. (2018) 47:1679–86. doi: 10.1093/ije/dyy098
10. May A. Neuroimaging: visualising the brain in pain. Neurol Sci. (2007) 28(Suppl 2):S101–7. doi: 10.1007/s10072-007-0760-x
11. Pinho-Ribeiro FA, Verri WA Jr, Chiu IM. Nociceptor sensory neuron-immune interactions in pain and inflammation. Trends Immunol. (2017) 38:5–19. doi: 10.1016/j.it.2016.10.001
12. Henry DE, Chiodo AE, Yang W. Central nervous system reorganization in a variety of chronic pain states: a review. PM R. (2011) 3:1116–25. doi: 10.1016/j.pmrj.2011.05.018
13. Cravello L, Di Santo S, Varrassi G, Benincasa D, Marchettini P, de Tommaso M, et al. Chronic pain in the elderly with cognitive decline: a narrative review. Pain Ther. (2019) 8:53–65. doi: 10.1007/s40122-019-0111-7
14. Paladini A, Fusco M, Coaccioli S, Skaper SD, Varrassi G. Chronic pain in the elderly: the case for new therapeutic strategies. Pain Phys. (2015) 18:E863–76. doi: 10.36076/ppj.2015/18/E863
15. Angrist JD, Imbens GW, Rubin DB. Identification of causal effects using instrumental variables. J Am Stat Assoc. (1996) 91:12. doi: 10.2307/2291634
16. Grover S, Del Greco MF, Stein CM, Ziegler A. Mendelian randomization. Methods Mol Biol. (2017) 1666:581–628. doi: 10.1007/978-1-4939-7274-6_29
17. Grover S, Lill CM, Kasten M, Klein C, Del Greco MF, König IR. Risky behaviors and Parkinson disease: a mendelian randomization study. Neurology. (2019) 93:e1412–24. doi: 10.1212/WNL.0000000000008245
18. Henry A, Katsoulis M, Masi S, Fatemifar G, Denaxas S, Acosta D, et al. The relationship between sleep duration, cognition and dementia: a Mendelian randomization study. Int J Epidemiol. (2019) 48:849–60. doi: 10.1093/ije/dyz071
19. Cullell N, Cárcel-Márquez J, Gallego-Fábrega C, Muiño E, Llucià-Carol L, Lledós M, et al. Sleep/wake cycle alterations as a cause of neurodegenerative diseases: a Mendelian randomization study. Neurobiol Aging. (2021) 106:320.e1-320. doi: 10.1016/j.neurobiolaging.2021.05.008
20. Zhang G, Zhang L, Xia K, Zhuang Z, Huang T, Fan D. Daytime sleepiness might increase the risk of ALS: a 2-sample Mendelian randomization study. J Neurol. (2021) 268:4332–9. doi: 10.1007/s00415-021-10564-z
21. Dashti HS, Jones SE, Wood AR, Lane JM, van Hees VT, Wang H, et al. Genome-wide association study identifies genetic loci for self-reported habitual sleep duration supported by accelerometer-derived estimates. Nat Commun. (2019) 10:1100. doi: 10.1038/s41467-019-08917-4
22. Jones SE, Lane JM, Wood AR, van Hees VT, Tyrrell J, Beaumont RN, et al. Genome-wide association analyses of chronotype in 697,828 individuals provides insights into circadian rhythms. Nat Commun. (2019) 10:343. doi: 10.1038/s41467-018-08259-7
23. Jansen PR, Watanabe K, Stringer S, Skene N, Bryois J, Hammerschlag AR, et al. Genome-wide analysis of insomnia in 1,331,010 individuals identifies new risk loci and functional pathways. Nat Genet. (2019) 51:394–403. doi: 10.1038/s41588-018-0333-3
24. Johnston KJA, Adams MJ, Nicholl BI, Ward J, Strawbridge RJ, Ferguson A, et al. Genome-wide association study of multisite chronic pain in UK Biobank. PLoS Genet. (2019) 15:e1008164. doi: 10.1371/journal.pgen.1008164
25. Fritsche LG, Igl W, Bailey JN, Grassmann F, Sengupta S, Bragg-Gresham JL, et al. A large genome-wide association study of age-related macular degeneration highlights contributions of rare and common variants. Nat Genet. (2016) 48:134–43. doi: 10.1038/ng.3448
26. Jansen IE, Savage JE, Watanabe K, Bryois J, Williams DM, Steinberg S, et al. Genome-wide meta-analysis identifies new loci and functional pathways influencing Alzheimer's disease risk. Nat Genet. (2019) 51:404–13. doi: 10.1038/s41588-018-0311-9
27. Lambert JC, Ibrahim-Verbaas CA, Harold D, Naj AC, Sims R, Bellenguez C, et al. Meta-analysis of 74,046 individuals identifies 11 new susceptibility loci for Alzheimer's disease. Nat Genet. (2013) 45:1452–8. doi: 10.1038/ng.2802
28. van Rheenen W, Shatunov A, Dekker AM, McLaughlin RL, Diekstra FP, Pulit SL, et al. Genome-wide association analyses identify new risk variants and the genetic architecture of amyotrophic lateral sclerosis. Nat Genet. (2016) 48:1043–8. doi: 10.1038/ng.3622
29. International Multiple Sclerosis Genetics C. Multiple sclerosis genomic map implicates peripheral immune cells and microglia in susceptibility. Science. (2019) 365:eaav7188. doi: 10.1126/science.aav7188
30. Nalls MA, Blauwendraat C, Vallerga CL, Heilbron K, Bandres-Ciga S, Chang D, et al. Identification of novel risk loci, causal insights, and heritable risk for Parkinson's disease: a meta-analysis of genome-wide association studies. Lancet Neurol. (2019) 18:1091–102. doi: 10.1016/S1474-4422(19)30320-5
31. Nalls MA, Pankratz N, Lill CM, Do CB, Hernandez DG, Saad M, et al. Large-scale meta-analysis of genome-wide association data identifies six new risk loci for Parkinson's disease. Nat Genet. (2014) 46:989–93. doi: 10.1038/ng.3043
32. Bulik-Sullivan BK, Loh PR, Finucane HK, Ripke S, Yang J, Patterson N, et al. LD Score regression distinguishes confounding from polygenicity in genome-wide association studies. Nat Genet. (2015) 47:291–5. doi: 10.1038/ng.3211
33. Verbanck M, Chen C-Y, Neale B, Do R. Detection of widespread horizontal pleiotropy in causal relationships inferred from Mendelian randomization between complex traits and diseases. Nat Genet. (2018) 50:693–8. doi: 10.1038/s41588-018-0099-7
34. Tsai DC, Chen HC, Leu HB, Chen SJ, Hsu NW, Huang CC, et al. The association between clinically diagnosed insomnia and age-related macular degeneration: a population-based cohort study. Acta Ophthalmol. (2020) 98:e238–44. doi: 10.1111/aos.14238
35. Perez-Canales JL, Rico-Sergado L, Perez-Santonja JJ. Self-reported sleep duration in patients with neovascular age-related macular degeneration. Ophthalmic Epidemiol. (2016) 23:20–6. doi: 10.3109/09286586.2015.1119288
36. Khurana RN, Porco TC, Claman DM, Boldrey EE, Palmer JD, Wieland MR. Increasing sleep duration is associated with geographic atrophy and age-related macular degeneration. Retina. (2016) 36:255–8. doi: 10.1097/IAE.0000000000000706
37. Anastasopoulos E, Haidich AB, Coleman AL, Wilson MR, Harris A, Yu F, et al. Risk factors for age-related macular degeneration in a Greek population: the Thessaloniki Eye Study. Ophthalmic Epidemiol. (2018) 25:457–69. doi: 10.1080/09286586.2018.1512634
38. Tosini G, Baba K, Hwang CK, Iuvone PM. Melatonin: an underappreciated player in retinal physiology and pathophysiology. Exp Eye Res. (2012) 103:82–9. doi: 10.1016/j.exer.2012.08.009
39. Robinson DG, Margrain TH, Dunn MJ, Bailey C, Binns AM. Low-level nighttime light therapy for age-related macular degeneration: a randomized clinical trial. Invest Ophthalmol Vis Sci. (2018) 59:4531–41. doi: 10.1167/iovs.18-24284
40. Zhou H, Zhang H, Yu A, Xie J. Association between sunlight exposure and risk of age-related macular degeneration: a meta-analysis. BMC Ophthalmol. (2018) 18:331. doi: 10.1186/s12886-018-1004-y
41. Tomany SC, Cruickshanks KJ, Klein R, Klein BE, Knudtson MD. Sunlight and the 10-year incidence of age-related maculopathy: the Beaver Dam Eye Study. Arch Ophthalmol. (2004) 122:750–7. doi: 10.1001/archopht.122.5.750
42. Augustin AJ, Dick HB, Offermann I, Schmidt-Erfurth U. [The significance of oxidative mechanisms in diseases of the retina]. Klin Monbl Augenheilkd. (2002) 219:631–43. doi: 10.1055/s-2002-35164
43. Roberts JE. Ocular phototoxicity. J Photochem Photobiol B. (2001) 64:136–43. doi: 10.1016/S1011-1344(01)00196-8
44. Winkler BS, Boulton ME, Gottsch JD, Sternberg P. Oxidative damage and age-related macular degeneration. Mol Vis. (1999) 5:32.
45. Ohara T, Honda T, Hata J, Yoshida D, Mukai N, Hirakawa Y, et al. Association between daily sleep duration and risk of dementia and mortality in a Japanese Community. J Am Geriatr Soc. (2018) 66:1911–8. doi: 10.1111/jgs.15446
46. Westwood AJ, Beiser A, Jain N, Himali JJ, DeCarli C, Auerbach SH, et al. Prolonged sleep duration as a marker of early neurodegeneration predicting incident dementia. Neurology. (2017) 88:1172–9. doi: 10.1212/WNL.0000000000003732
47. Lo Coco D, Mattaliano P, Spataro R, Mattaliano A, La Bella V. Sleep-wake disturbances in patients with amyotrophic lateral sclerosis. J Neurol Neurosurg Psychiatry. (2011) 82:839–42. doi: 10.1136/jnnp.2010.228007
48. Panda S, Gourie-Devi M, Sharma A. Sleep disorders in amyotrophic lateral sclerosis: a questionnaire-based study from India. Neurol India. (2018) 66:700–8. doi: 10.4103/0028-3886.232327
49. Gunther R, Richter N, Sauerbier A, Chaudhuri KR, Martinez-Martin P, Storch A, et al. Non-motor symptoms in patients suffering from motor neuron diseases. Front Neurol. (2016) 7:117. doi: 10.3389/fneur.2016.00117
50. Krupp L. Fatigue is intrinsic to multiple sclerosis (MS) and is the most commonly reported symptom of the disease. Mult Scler. (2006) 12:367–8. doi: 10.1191/135248506ms1373ed
51. de Tommaso M, Arendt-Nielsen L, Defrin R, Kunz M, Pickering G, Valeriani M. Pain assessment in neurodegenerative diseases. Behav Neurol. (2016) 2016:2949358. doi: 10.1155/2016/2949358
Keywords: Mendelian randomization, causal inference, neurodegenerative disorders, sleep, pain, chronotype
Citation: Grover S, Sharma M and International Age-related Macular Degeneration Genomics Consortium (IAMDGC) (2022) Sleep, Pain, and Neurodegeneration: A Mendelian Randomization Study. Front. Neurol. 13:765321. doi: 10.3389/fneur.2022.765321
Received: 27 August 2021; Accepted: 14 March 2022;
Published: 02 May 2022.
Edited by:
Rosanna Tortelli, University College London, United KingdomReviewed by:
Xinghao Yu, Soochow University, ChinaYiqiang Zhan, Helmholtz Association of German Research Centers (HZ), Germany
Copyright © 2022 Grover, Sharma, and International Age-related Macular Degeneration Genomics Consortium (IAMDGC). This is an open-access article distributed under the terms of the Creative Commons Attribution License (CC BY). The use, distribution or reproduction in other forums is permitted, provided the original author(s) and the copyright owner(s) are credited and that the original publication in this journal is cited, in accordance with accepted academic practice. No use, distribution or reproduction is permitted which does not comply with these terms.
*Correspondence: Manu Sharma, bWFudS5zaGFybWFAdW5pLXR1ZWJpbmdlbi5kZQ==