- 1National Institute of Mental Health, Neurology, and Neurosurgery (NIMNN), Budapest, Hungary
- 2Oxford University Hospitals NHS Foundation Trust, Oxford, United Kingdom
- 3Radcliffe Department of Medicine, University of Oxford, Oxford, United Kingdom
- 4Brainomix Ltd., Oxford, United Kingdom
- 5Centre for Statistics in Medicine, Nuffield Department of Orthopedics, Rheumatology and Musculoskeletal Sciences, University of Oxford, Oxford, United Kingdom
- 6Faculty of Medicine, Semmelweis University, Budapest, Hungary
Background: Short- and long-term outcomes from endovascular thrombectomy (EVT) for large vessel occlusion stroke remain variable. Numerous relevant predictors have been identified, including severity of neurological deficits, age, and imaging features. The latter is typically defined as acute changes (most commonly Alberta Stroke Programme Early CT Score, ASPECTS, at presentation), but there is little information on the impact of imaging assessment of premorbid brain health as a determinant of outcome.
Aims: To examine the impact of automated measures of stroke severity and underlying brain frailty on short- and long-term outcomes in acute stroke treated with EVT.
Methods: In 215 patients with anterior circulation stroke, who subsequently underwent EVT, automated analysis of presenting non-contrast CT scans was used to determine acute ischemic volume (AIV) and e-ASPECTS as markers of stroke severity, and cerebral atrophy as a marker of brain frailty. Univariate and multivariate logistic regression were used to identify significant predictors of NIHSS improvement, modified Rankin scale (mRS) at 90 and 30 days, mortality at 90 days and symptomatic intracranial hemorrhage (sICH) following successful EVT.
Results: For long-term outcome, atrophy and presenting NIHSS were significant predictors of mRS 0–2 and death at 90 days, whereas age did not reach significance in multivariate analysis. Conversely, for short-term NIHSS improvement, AIV and age were significant predictors, unlike presenting NIHSS. The interaction between age and NIHSS was similar to the interaction of AIV and atrophy for mRS 0–2 at 90 days.
Conclusion: Combinations of automated software-based imaging analysis and clinical data can be useful for predicting short-term neurological outcome and may improve long-term prognostication in EVT. These results provide a basis for future development of predictive tools built into decision-aiding software in stroke.
Introduction
Outcomes in endovascular thrombectomy (EVT) for emergent large vessel occlusion (ELVO) stroke remain variable: only 46% of patients treated with EVT in early time-window trials achieved functional independence (modified Rankin scale, mRS 0–2) (1). In real-world registry studies, the overall functional independence has been reported to be as low as 23% after EVT (2). As such, improved patient selection criteria are required to maximize cost-effectiveness while minimizing unnecessary procedure-related risks.
Currently, identifying suitable candidates relies on clinical features (presenting severity on the National Institutes of Health Stroke Scale, NIHSS; baseline mRS), time from stroke onset (3) and imaging findings, including infarct volume (4), Alberta Stroke Programme Early CT Score (ASPECTS) (5) and volume of ischemic tissue relative to infarct (6). However, these radiological features all represent acute changes. There is increasing evidence that imaging biomarkers unrelated to the index stroke, including atrophy as an indicator of brain frailty, have a significant impact on clinical outcome (7, 8). However, there is no consensus on the best integrative approach to use these factors for long-term prognosis and poor understanding of how they interact. Furthermore, manual quantification of atrophy using visual analog scales can be subjective (9) while automated quantification usually requires separate software, which would complicate reporting workflow in the acute setting.
In this study, we sought to use machine learning based automated image analysis of routine non-contrast CT (NCCT) brain imaging, in conjunction with clinical variables, to improve prognostication for short-term neurological improvement (changes in NIHSS), long-term functional outcomes (mRS at 30 and 90 days), mortality and symptomatic intracranial hemorrhage. Our hypothesis was that automated image analysis of routine NCCT imaging markers of both stroke severity and brain frailty would be significant predictors of short- and long-term outcome alone following successful EVT.
Methods
Study design
This retrospective study was performed at the National Institute of Mental Health, Neurology and Neurosurgery (NIMNN) in Budapest, Hungary. From 1 January 2017 to 31 December 2019, we included patients receiving endovascular thrombectomy for acute ischemic stroke. All treatment decisions were made based on clinical criteria, as indicated according to first line international (3) and local guidelines. Further inclusion criteria included: age ≥18, causative middle cerebral artery M1 segment occlusion, onset to groin puncture ≤6 h, NIHSS score ≥ 6 at presentation, premorbid mRS state ≤1, ASPECTS ≥ 6, and successful recanalization (TICI ≥ 2b).
Since more than 90% of the patients are transferred from primary stroke centers to NIMNN for EVT, an NCCT scan was repeated on arrival for final decision-making regarding EVT. These preprocedural scans were defined as baseline imaging and processed using e-Stroke software (version 10; Brainomix, UK), a machine learning based decision aid tool for acute ischemic stroke, which has been validated against manual analysis by neuroradiologists in previous studies (10, 11). e-Stroke was used to estimate acute ischemic volume (AIV), automated e-ASPECTS, and atrophy (defined based on CSF volume in the lateral ventricles and surrounding brain parenchyma, relative to parenchymal volume). Patients with missing or inadequate baseline imaging were excluded from analyses.
We further collected patient demographics, baseline imaging features (including radiologist ASPECTS scores), treatment times (onset to groin, groin to recanalization, NIHSS, whether the patient received intravenous thrombolysis, TICI scores, short-term neurological outcome (NIHSS improvement at discharge or 7 days), longer term functional outcome (mRS at 30 and 90 days) and whether the patient suffered symptomatic intracranial hemorrhage [defined as European Cooperative Acute Stroke Study (ECASS) parenchymal hematoma 1 and 2 categories].
Data analysis
Data were collected into a database as part of clinical care and therefore unblinded. Patients were invited for follow-up clinic appointments where mRS was determined by the reviewing physician; for patients who did not attend appointments, trained abstractors collected mRS over the phone. Statistical analyses were performed in R version 4.1.1 (12). Primary outcomes were considered as early, defined as dichotomized NIHSS improvement at discharge (reduction of NIHSS of ≥4 points in patients with a presenting NIHSS ≥4), and late, defined as dichotomized functional outcome at 90 days (with mRS 0–2 considered as good outcome). Secondary outcomes included mRS 0–2 at 30 days, intracranial hemorrhage, and mortality at 90 days. Univariate logistic regression was performed for manually selected variables. Multivariate logistic regression was performed with our four primary predictors of interest (presenting NIHSS and AIV as clinical and radiological indicators of acute severity, and age and atrophy as clinical and radiological indicators of brain frailty), alongside variables that were significant in univariate analyses (p < 0.05). As AIV and e-ASPECTS were highly collinear, the latter was not included in multivariate models even if significant in univariate analysis. Cases with missing outcome data were excluded from analysis for that model. Descriptive analysis was undertaken to explore the interaction between stroke severity and brain frailty on outcome, using both clinical and imaging biomarkers.
Results
The study population included 215 patients; demographic details and procedural characteristics are displayed in Table 1. Overall, 104 patients (48.4%) achieved mRS 0–2 at 90 days. The significant univariate and multivariate predictors of achieving mRS 0–2 are summarized in Table 2. Briefly, univariate analyses showed that likelihood of good outcome was significantly associated with age, NIHSS on admission, NCCT AIV, e-ASPECTS, atrophy and TICI status. When adjusted in multivariate regression, however, only atrophy, NIHSS on admission and TICI 2C/3 retained statistical significance. The cumulative effect of age and NIHSS, and separately infarct volume, and atrophy on functional outcome are shown in Figure 1. The pattern of interaction between stroke severity and surrogates of baseline frailty showed a similar relationship when assessed using both clinical and imaging characteristics.
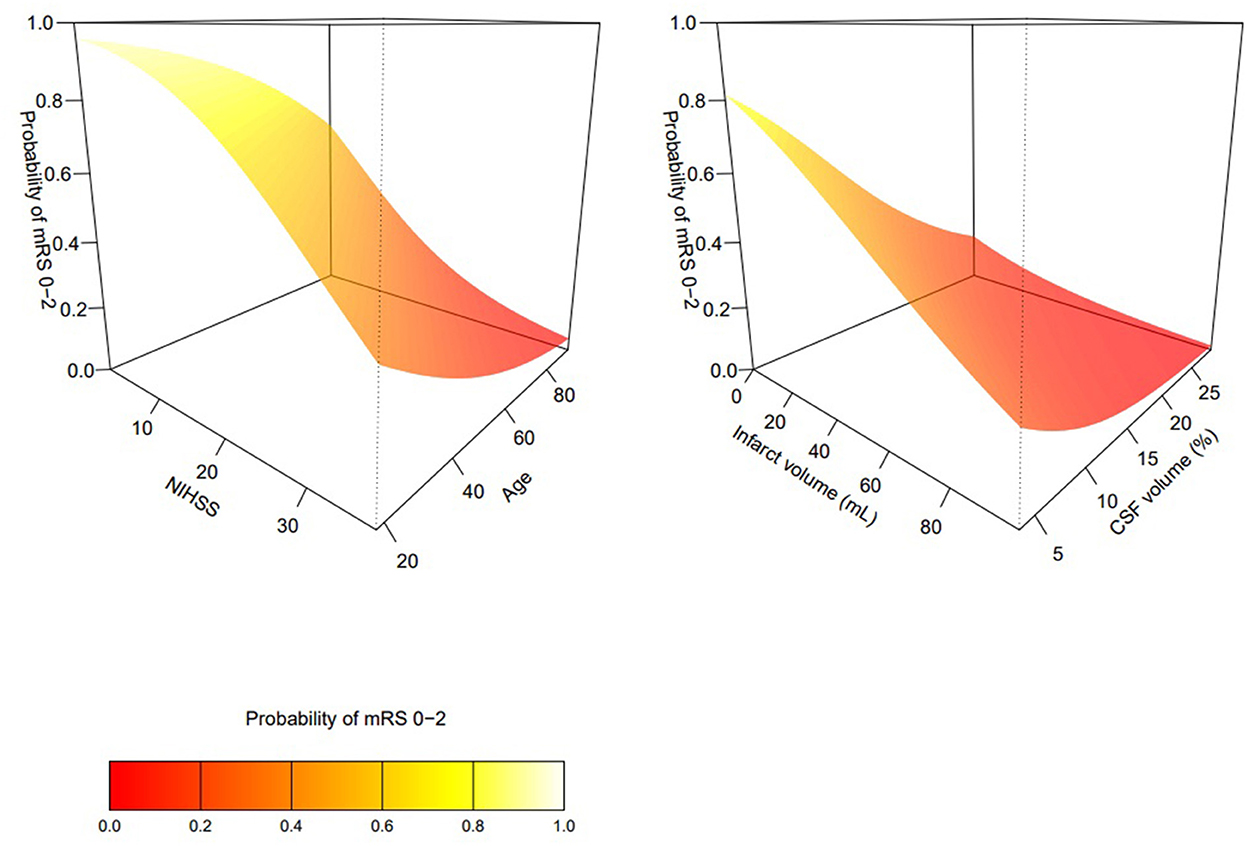
Figure 1. Interactions between age and NIHSS (left), and infarct volume and atrophy (right) in predicting mRS 0–2 at 90 days.
Using the same approach, we then looked at early neurological outcomes following thrombectomy. NIHSS improvement data were available in 203 patients, with six patients excluded due to missing follow up NIHSS and six patients excluded due to baseline severity of NIHSS < 4. NIHSS improvement was achieved by 162 patients (79.8%), albeit with a wide range of absolute NIHSS values at discharge (range 0–30, IQR 2–11). The univariate and multivariate predictors for NIHSS improvement are summarized in Table 3. In univariate analyses, only AIV and onset to recanalization time were associated with NIHSS improvement. After adjustment in multivariate analysis, age, AIV and onset to recanalization demonstrated statistical significance.
We performed the same analyses for mRS 0–2 at 30 days, achieved by 90 patients (41.9%). Univariate and multivariate predictors are displayed in Supplementary Table 1. In univariate analysis, age, AIV, NIHSS, atrophy and TICI 2C/3 status were all associated with functional independence at 30 days, similarly to the 90-day mRS; in the adjusted analysis, only age, AIV and TICI retained significance. At 90 days, 65 patients (30.2%) had died. The univariate and multivariate analyses for mortality are in Supplementary Table 2; age, admission NIHSS and atrophy were associated with death in univariate analysis, while only NIHSS and atrophy demonstrated statistical significance in multivariate analysis. We also attempted to examine post-procedural sICH, but this was limited by sample size as only 12 patients developed parenchymal hemorrhage type 1 or 2. NIHSS on admission was the only variable to show significant association to sICH in both univariate and multivariate analysis (Supplementary Table 3).
Discussion
Imaging biomarkers automatically derived from CT imaging routinely acquired at presentation provided information on both the acute injury sustained (acute infarct volume) and on the premorbid condition of the brain (brain atrophy). Both clinical and imaging markers of stroke severity, i.e., presenting NIHSS and acute infarct volume, offer potentially useful information when predicting neurological improvement. Furthermore, there is additional information to be gained from both clinical and imaging markers of brain frailty when predicting early outcomes. The interactions between markers of stroke severity and surrogates of frailty appear similar whether clinical or radiological variables are used.
Atrophy was more strongly associated with long-term outcome compared to age. Compared to age, atrophy likely provides a better estimate of brain frailty, a key determinant of vulnerability to injury (13). Metrics capturing biological age, as opposed to calendar age, might also better inform of the capacity of an individual to recover following stroke as they may better represent the brain neurological reserve and the ability to compensate for an infarct of given volume following stroke.
While long term outcome is influenced by multiple factors, such as post EVT care, rehabilitation, and comorbid factors, the early neurological outcome might be argued to be more sensitive to the impact of factors related to the index stroke severity and degree of procedural success. It is unclear why age rather than atrophy demonstrated this association in these results; hypothetically, non-neurological factors such as musculoskeletal comorbidity may contribute to the potential for early neurological compensation which is more likely to be captured by age than a neurologically specific biomarker such as atrophy.
The influence of the individual biomarkers used in this study is broadly consistent with previous observations. e-ASPECTS, which is derived from the automated AIV, has been demonstrated to predict mRS at 90 days in other cohorts (14). Similarly, automated atrophy quantification strongly predicts mRS, with an odds ratio of 0.65 per 5% increase in intracranial cerebrospinal fluid volume (15). A previous machine learning study of NCCT and CTA features using e-Stroke found that age, baseline NIHSS, occlusion side, atrophy and e-ASPECTS were the best predictors for mRS at 90 days, in keeping with the results shown here (16). The clinical variables used in this study—age and presenting NIHSS—have previously been used as a prognostic score, which was also an independent predictor of outcome in EVT (17). Other studies have also found significant predictive effects from age and NIHSS at presentation (18), and there is a combined effect between NIHSS and age in agreement with the data presented here (19). Procedurally, there is a strong correlation between treatment times and outcome, particularly in the early window (2). Excellent reperfusion (TICI 2C/3) is also highly predictive or mRS at 3 months, especially when this is achieved during the first pass (20).
There is comparatively less data on predictors of early neurological improvement which is more directly linked to the immediate impact of an intervention, and most of these focus on the earliest time windows. One study identified age, blood glucose, TICI, baseline ASPECTS and the presence of sICH as predictors for early neurological recovery (21). Others have looked at predictive factors for failure to neurologically improve, and identified variables including premorbid mRS, hyperglycemia, longer treatment times, lack of tPA bridging, and involvement of motor cortex and internal capsule in the infarct (22). Separately, there is considerable literature suggesting the utility of early neurological improvement itself as a long-term prognostic factor. In a large cohort study, absolute NIHSS at 24 h was the best predictor of mRS at 90 days, although NIHSS improvement was also strongly correlated with long-term outcome (23). Notably, in our cohort AIV was a better predictor of early neurological improvement than atrophy, illustrating the differential impact of acute and chronic brain changes to short- and long-term recovery.
Our study has limitations. First, although the group sizes of those achieving mRS 0–2 and 3–6 were similar, the overall sample size was relatively small at 215 patients. In addition, the intracerebral hemorrhage analyses were underpowered as there were only 12 cases in the cohort. Second, this was a single-center retrospective cohort study. Validation in prospective cohorts is required. Third, our hypotheses and choices of variables were clinically driven, and we cannot exclude that there are other imaging parameters that may further improve performance but were not captured in this dataset.
Overall, these data support the use of automated imaging analysis for improving the prediction of neurological recovery following EVT and demonstrate the additional information that can be captured from simple NCCT imaging. The results show the interaction between markers of stroke severity and the brain frailty of the individual and suggest an opportunity to refine estimates of an individual's capacity to recover beyond that of their chronological age. If validated in prospective cohorts, this may provide a useful adjunct tool for prognostication in large vessel occlusion ischemic stroke.
Data availability statement
The raw data supporting the conclusions of this article will be made available by the authors, without undue reservation.
Ethics statement
The studies involving human participants were reviewed and approved by National Institute of Mental Health, Neurology and Neurosurgery (NIMNN), Budapest, Hungary. Written informed consent for participation was not required for this study in accordance with the national legislation and the institutional requirements.
Author contributions
BK, ZC, AP, EC, VC, ÁS, IS, and LE designed the study and collected data. BK, AN, and SG performed data analysis. GH and OJ provided essential data analysis tools. AN, GH, and DC drafted the manuscript. All authors were involved with manuscript revisions and contributed to the article and approved the submitted version.
Conflict of interest
Authors DC, GH, OJ, and ZC have share options in and are employees of Brainomix. Author SG has received consultancy fees from Brainomix.
The remaining authors declare that the research was conducted in the absence of any commercial or financial relationships that could be construed as a potential conflict of interest.
Publisher's note
All claims expressed in this article are solely those of the authors and do not necessarily represent those of their affiliated organizations, or those of the publisher, the editors and the reviewers. Any product that may be evaluated in this article, or claim that may be made by its manufacturer, is not guaranteed or endorsed by the publisher.
Supplementary material
The Supplementary Material for this article can be found online at: https://www.frontiersin.org/articles/10.3389/fneur.2022.1056532/full#supplementary-material
References
1. Menon BK, van Zwam WH. Dippel DWJ, Mitchell PJ, Demchuk AM, et al. Endovascular thrombectomy after large-vessel ischaemic stroke: a meta-analysis of individual patient data from five randomised trials. The Lancet. (2016) 387:1723–31. doi: 10.1016/S0140-6736(16)00163-X
2. Jahan R, Saver JL, Schwamm LH, Fonarow GC, Liang L, Matsouaka RA, et al. Association between time to treatment with endovascular reperfusion therapy and outcomes in patients with acute ischemic stroke treated in clinical practice. JAMA. (2019) 322:252–63. doi: 10.1001/jama.2019.8286
3. Powers WJ, Rabinstein AA, Ackerson T, Adeoye OM, Bambakidis NC, Becker K, et al. Guidelines for the early management of patients with acute ischemic stroke: 2019 update to the 2018 guidelines for the early management of acute ischemic stroke: a guideline for healthcare professionals from the american heart association/american stroke association. Stroke. (2019) 50:e344–418. doi: 10.1161/STR.0000000000000211
4. Boers AMM, Jansen IGH, Beenen LFM, Devlin TG, San Roman L, Heo JH, et al. Association of follow-up infarct volume with functional outcome in acute ischemic stroke: a pooled analysis of seven randomized trials. J Neurointerv Surg. (2018) 10:1137–42. doi: 10.1136/neurintsurg-2017-013724
5. Cagnazzo F, Derraz I, Dargazanli C, Lefevre PH, Gascou G, Riquelme C, et al. Mechanical thrombectomy in patients with acute ischemic stroke and ASPECTS 6: a meta-analysis. J Neurointerv Surg. (2020) 12:350–5. doi: 10.1136/neurintsurg-2019-015237
6. Albers GW, Marks MP, Kemp S, Christensen S, Tsai JP, Ortega-Gutierrez S, et al. Thrombectomy for Stroke at 6 to 16 hours with selection by perfusion imaging. N Engl J Med. (2018) 378:708–18. doi: 10.1056/NEJMoa1713973
7. Lauksio I, Lindstrom I, Khan N, Sillanpaa N, Hernesniemi J, Oksala N, et al. Brain atrophy predicts mortality after mechanical thrombectomy of proximal anterior circulation occlusion. J Neurointerv Surg. (2021) 13:415–20. doi: 10.1136/neurintsurg-2020-016168
8. Pedraza MI, de Lera M, Bos D, Calleja AI, Cortijo E, Gomez-Vicente B, et al. Brain atrophy and the risk of futile endovascular reperfusion in acute ischemic stroke. Stroke. (2020) 51:1514–21. doi: 10.1161/STROKEAHA.119.028511
9. Harper L, Barkhof F, Fox NC, Schott JM. Using visual rating to diagnose dementia: a critical evaluation of MRI atrophy scales. J Neurol Neurosurg Psychiatry. (2015) 86:1225–33. doi: 10.1136/jnnp-2014-310090
10. Sundaram VK, Goldstein J, Wheelwright D, Aggarwal A, Pawha PS, Doshi A, et al. Automated ASPECTS in acute ischemic stroke: a comparative analysis with CT perfusion. AJNR Am J Neuroradiol. (2019) 40:2033–8. doi: 10.3174/ajnr.A6303
11. Wolff L, Berkhemer OA, van Es A, van Zwam WH, Dippel DWJ, Majoie C, et al. Validation of automated alberta stroke program early ct score (ASPECTS) software for detection of early ischemic changes on non-contrast brain CT scans. Neuroradiology. (2021) 63:491–8. doi: 10.1007/s00234-020-02533-6
12. R Core Team. R: A Language and Environment for Statistical Computing. Vienna: R Foundation for Statistical Computing (2021).
13. Evans NR, Todd OM, Minhas JS, Fearon P, Harston GW, Mant J, et al. Frailty and cerebrovascular disease: concepts and clinical implications for stroke medicine. Int J Stroke. (2022) 17:251–9. doi: 10.1177/17474930211034331
14. Pfaff J, Herweh C, Schieber S, Schonenberger S, Bosel J, Ringleb PA, et al. e-ASPECTS correlates with and is predictive of outcome after mechanical thrombectomy. AJNR Am J Neuroradiol. (2017) 38:1594–9. doi: 10.3174/ajnr.A5236
15. Diprose WK, Diprose JP, Wang MTM, Tarr GP, McFetridge A, Barber PA. Automated measurement of cerebral atrophy and outcome in endovascular thrombectomy. Stroke. (2019) 50:3636–8. doi: 10.1161/STROKEAHA.119.027120
16. Jabal MS, Joly O, Kallmes D, Harston G, Rabinstein A, Huynh T, et al. Interpretable machine learning modeling for ischemic stroke outcome prediction. Front Neurol. (2022) 13:884693. doi: 10.3389/fneur.2022.884693
17. Almekhlafi MA, Davalos A, Bonafe A, Chapot R, Gralla J, Pereira VM, et al. Impact of age and baseline NIHSS scores on clinical outcomes in the mechanical thrombectomy using solitaire FR in acute ischemic stroke study. AJNR Am J Neuroradiol. (2014) 35:1337–40. doi: 10.3174/ajnr.A3855
18. Reddy ST, Friedman E, Wu TC, Arevalo O, Zhang J, Rahbar MH, et al. Rapid infarct progression in anterior circulation large vessel occlusion ischemic stroke patients during inter-facility transfer. J Stroke Cerebrovasc Dis. (2020) 29:105308. doi: 10.1016/j.jstrokecerebrovasdis.2020.105308
19. Ospel JM, Brown S, Kappelhof M, van Zwam W, Jovin T, Roy D, et al. Comparing the prognostic impact of age and baseline national institutes of health stroke scale in acute stroke due to large vessel occlusion. Stroke. (2021) 52:2839–45. doi: 10.1161/STROKEAHA.120.032364
20. Yoo AJ, Soomro J, Andersson T, Saver JL, Ribo M, Bozorgchami H, et al. Benchmarking the extent and speed of reperfusion: first pass tici 2c-3 is a preferred endovascular reperfusion endpoint. Front Neurol. (2021) 12:669934. doi: 10.3389/fneur.2021.669934
21. Zhang X, Peng M, Feng C, Wang H, Gong P, Jiang T, et al. Nomogram predicting early neurological improvement in ischaemic stroke patients treated with endovascular thrombectomy. Eur J Neurol. (2021) 28:152–60. doi: 10.1111/ene.14510
22. Weyland CS, Mokli Y, Vey JA, Kieser M, Herweh C, Schonenberger S, et al. Predictors for failure of early neurological improvement after successful thrombectomy in the anterior circulation. Stroke. (2021) 52:1291–8. doi: 10.1161/STROKEAHA.120.030519
Keywords: stroke, endovascular thrombectomy, thrombolysis, neuroimaging, neuroradiology, artificial intelligence, machine learning
Citation: Kis B, Neuhaus AA, Harston G, Joly O, Carone D, Gerry S, Chadaide Z, Pánczél A, Czifrus E, Csike V, Surányi Á, Szikora I and Erőss L (2022) Automated quantification of atrophy and acute ischemic volume for outcome prediction in endovascular thrombectomy. Front. Neurol. 13:1056532. doi: 10.3389/fneur.2022.1056532
Received: 28 September 2022; Accepted: 28 November 2022;
Published: 15 December 2022.
Edited by:
Andrew Gauden, Stanford University, United StatesReviewed by:
Bin Yang, Capital Medical University, ChinaAlvin S. Das, Beth Israel Deaconess Medical Center and Harvard Medical School, United States
Copyright © 2022 Kis, Neuhaus, Harston, Joly, Carone, Gerry, Chadaide, Pánczél, Czifrus, Csike, Surányi, Szikora and Erőss. This is an open-access article distributed under the terms of the Creative Commons Attribution License (CC BY). The use, distribution or reproduction in other forums is permitted, provided the original author(s) and the copyright owner(s) are credited and that the original publication in this journal is cited, in accordance with accepted academic practice. No use, distribution or reproduction is permitted which does not comply with these terms.
*Correspondence: Balázs Kis, blazsejdoktor@gmail.com
†These authors share first authorship