- 1Institute of Neuroimmunology and Multiple Sclerosis (INIMS), Center for Molecular Neurobiology, University Medical Center Hamburg-Eppendorf, Hamburg, Germany
- 2Department of Neurology, University Medical Center Hamburg-Eppendorf, Hamburg, Germany
- 3Health Division, SINTEF, Trondheim, Norway
- 4School of Medicine, University of Nottingham, Nottingham, United Kingdom
Objective: To provide an overview of the evidence on driving ability in persons with multiple sclerosis (PwMS), specifically to (i) study the impact of MS impairment on driving ability and (ii) evaluate predictors for driving performance in MS.
Methods: To identify relevant studies, different electronic databases were screened in accordance with PRISMA guidelines; this includes reference lists of review articles, primary studies, and trial registers for protocols. Furthermore, experts in the field were contacted. Two reviewers independently screened titles, abstracts, and full-texts to identify relevant articles targeting driving in people with MS that investigated driving-related issues with a formal driving assessment (defined as either an on-road driving assessment; or naturalistic driving in a car equipped with video cameras to record the driving; or a driving simulator with a steering wheel, a brake pedal, and an accelerator).
Results: Twenty-four publications, with 15 unique samples (n = 806 PwMS), were identified. To assess driving ability, on-road tests (14 papers) and driving simulators (10 papers) were used. All studies showed moderate to high study quality in the CASP assessment. About 6 to 38% of PwMS failed the on-road tests, showing difficulties in different areas of driving. Similarly, PwMS showed several problems in driving simulations. Cognitive and visual impairment appeared to most impact driving ability, but the evidence was insufficient and inconsistent.
Conclusion: There is an urgent need for more research and standardized guidelines for clinicians as one in five PwMS might not be able to drive safely. On-road tests may be the gold standard in assessing driving ability, but on-road protocols are heterogeneous and not infallible. Driving simulators assess driving ability in a standardized way, but without standardized routes and driving outcomes, comparability between studies is difficult. Different aspects, such as cognitive impairment or vision problems, impact driving ability negatively and should be taken into consideration when making decisions about recommending driving cessation.
Systematic review registration: Identifier [10.17605/OSF.IO/WTG9J].
Introduction
Multiple sclerosis (MS) is a chronic neurodegenerative disease that is one of the most common neurological diseases in young adults (1). MS frequently causes severe disabilities related to vision, mobility in the upper and lower limbs, and cognition (e.g., slower information processing, impaired attentional functions, and visuospatial deficits) (2–5). Three out of every four people with MS (PwMS) have gait dysfunction and limited mobility (6, 7), with walking disabilities, especially leading to patients' reliance on driving. However, driving is a complex task that involves many cognitive, visual, and motor domains, and impairment in these domains has an impact on driving ability and driving safety (8–10). MS is characterized by visual impairment and cognitive deficits, which are major risk factors for drivers (11–19).
Research shows that PwMS are more likely to be involved in automobile accidents than people without MS (20), make more mistakes while driving (21), and are 3.4 times more likely to visit the emergency department because of automobile accidents (20). Therefore, a number of studies have explored the association between MS and driving in the last decades, but despite considerable evidence for reduced driving performance, there are no clear guidelines regarding fitness for driving in MS. Little is known about MS and problems with specific driving parameters, such as control of speed, tracking stability, and recognition of dangerous situations (22). A review by Krasniuk et al. (22) found only two tests to predict driving ability in MS: The Stroke Driver Screening Assessment (23) and Useful Field of View test (24, 25) (UFOV).
Measures to assess driving ability and evaluation thresholds differ across countries, regions, and are largely not evidence-based (26, 27). A review by Fragoso et al. (28) showed five different approaches to measuring driving ability in MS: (1) measuring driving ability through self-report; (2) reporting crashes and traffic violations through government or institutional data; (3) assessing crash-risk indices through computer-based assessment; (4) observing driving performance through driving simulator assessment; and (5) evaluating fitness to drive through on-road assessment. They conclude, however, that there is no specific literature on driving abilities in PwMS that assists in creating legislation (28). Even if clinical standards for suspending driving licenses exist in practice, it is frequently an arbitrary decision based on experiences and unspecific decisions of healthcare professionals. However, driving is important for maintaining independence for PwMS, and driving cessation is related to isolation, depression, and functional decline (29, 30). Therefore, there is an urgent need not only for clinicians but also for lawgivers and traffic authority personnel to have fair and reliable regulations and guidelines to evaluate driving abilities in PwMS.
Thus, the aim of this systematic review was to study (i) the impact of MS impairment on driving ability and (ii) to identify predictors for driving performance in MS. Additionally, we provide an overview of the available evidence on driving ability to determine whether the findings are sufficient to help develop standardized guidelines in future and to locate possible gaps in the literature to improve future research and clinical practice if not.
Methods
Search strategy
This systematic review was planned, conducted, and reported in accordance with PRISMA guidelines (www.prismastatement.org) and the protocol was registered with OSF Registry (10.17605/OSF.IO/WTG9J). A comprehensive search was performed on the Cochrane Library, Ovid MEDLINE, PsycInfo, Embase, and CINAHL databases to locate relevant studies up to 1 January 2022. We also screened reference lists of review articles and primary studies, checked trial registers (clinicaltrialsregister.eu, rialregister.nl, and isrctn.com) for protocols, and contacted experts in the field to identify further published or unpublished studies. The search strategy was developed by the author/co-author team and was guided by an expert on Systematic Reviews (AR and JuP).
This review is part of a project to review driving ability in people with neurodegenerative disorders. Therefore, the initial database search terms incorporated several neurological diseases, but for this study, only data about PwMS were extracted. The search terms included MeSH terms as well as the following 14 key terms: multiple sclerosis, Parkinson's disease, Alzheimer's disease, dementia, neurodegenerative disorder, Huntington, drive, accidents, traffic, simulation, on-road, car, automobile, and vehicle, as well as different spellings of those words. The search strategy for this review is shown in Supplementary material 1. Two database searches were conducted, the initial search (up to April 2020) and an update search (up to January 2022). In the second database search, the keywords for the other diseases beside MS were excluded.
Study inclusion criteria
Articles that met the following criteria for inclusion were selected for full-text review and analysis: (1) original research article published in a peer-reviewed journal; (2) English or German language; (3) human studies; (3) full-text available; (4) included drivers with a diagnosis of MS; (5) investigating driving-related issues with a formal driving assessment (formal driving assessment was defined as (a) an on-road driving assessment; (b) naturalistic driving in a car equipped with video cameras to tape the driving; or (c) a driving simulator. The driving simulator had to have a steering wheel, a brake pedal, and an accelerator to be considered); (6) used quantitative methods for data collection and reported quantitative results in the analysis. Studies were excluded if they (1) presented data in which PwMS were mixed in with other groups, or (2) were commentary articles, literature reviews, case studies, conference abstracts, and proceedings or dissertations.
Quality assessment
We used the Critical Appraisal Skills Programme (31) (CASP) tool to determine the validity and quality of the included studies. We adapted the CASP tool slightly to enhance the validity and reliability of the quality assessment. We used the CASP Case-Control Study Checklist for the assessment. Scores ranged from 0 to 6 points with higher values indicating better quality. Two reviewers (SSZ and JP) assessed the studies separately accordingly to the CASP requirements and resolved discrepancies by discussion.
Screening strategy and data extraction
After the database searches, we removed duplicates. Two researchers worked independently to screen the remaining titles and abstracts for inclusion (SSZ and EF). Any discrepancies raised during this process were resolved by discussion with the team. For the full-text screening stage, two researchers (SSZ and KW) worked independently to include/exclude studies using the selection criteria. Disagreements were resolved by discussion and consensus. Information extracted from included studies were: (1) study design (for longitudinal studies only pre-test assessments were included); (2) methodological considerations; (3) inclusion and exclusion criteria; (4) participant characteristics and demographics; (5) comparison of control group characteristics; (6) results of specific outcome measures; and (7) key findings. Data extraction was executed by two independent authors (SSZ and JP) and cross-checked.
Evidence synthesis
Only a narrative review (without meta-analyses) was undertaken due to the vastly different outcome measures, sample characteristics, and study designs of the included studies. The existing evidence is reported according to the different assessments for driving (on-road driving vs. driving in a driving simulator). MS-related disease factors influencing the assessed driving ability are summarized based on the available studies reporting driving assessment outcomes.
Results
Paper selection, characteristics of selected studies, and participants included
The database search yielded 4,081 papers (Figure 1). We included 24 papers for this review (11–19, 32–46). All included studies were published in English.
The papers covered 15 different/unique samples (some papers reported data with the same study sample) with 806 PwMS (n = 553 women; 69 %) and 280 non-MS controls (n = 146 women; 52 %). The same samples reported in more than one paper are marked with *n in the citation. Fourteen studies assessed driving ability with an on-road test, while 10 studies used a driving simulator.
Demographic and disease-specific data of all included studies are shown in Supplementary material 2. Most studies included small to moderate sample sizes: on-road studies ranged from 30 (13*2) to 218 (43) PwMS (mean: 65 PwMS included per study), while driving simulator studies ranged from 11 (36) to 38 (39*3, 40*3) PwMS (mean: 25 PwMS included per study). The age of the PwMS ranged between 35.6 years (mean, SD 8.3) (17) and 55 years (median, Q1–3: 50–59) (35). Most PwMS had relapsing-remitting MS (n = 439), 25 were classified as SPMS, 29 had PPMS, and 13 were reported as unknown. Four studies did not report the MS subtype (n = 300) (18, 41, 43, 44). The disability level of the participants was reported via EDSS in almost all samples except for three (16*5, 41, 43, 46*5), and ranged from 1.95 (mean, SD 0.91) to 6.00 (median, range 3.0–7.5). One study used the ambulation index to assess disability (16*5), and the other two studies did not assess disability level (41, 43). Six hundred nineteen PwMS (n = 429 women; 69 %) were assessed via on-road driving test (see Table 1) and 187 PwMS (n = 124 women; 66 %) in a driving simulator (see Table 2). Nine studies used healthy participants as controls (17, 18, 34–36, 39*3, 40*3, 45*4, 46*5). One study included older volunteers as controls (13*2). The studies were conducted in the US (n = 12), Canada (n = 7), Belgium (n = 2), France (n = 1), United Kingdom (n = 1), and Germany (n = 1).
Study quality assessment
Results of the CASP assessments are shown in Supplementary material 3. Quality ratings for the included studies ranged from 3/6 to two studies rated with 6/6 points (39*3, 40*3). No study was excluded for poor quality. Heterogeneity in samples, data collection, and measurement of identified variables was present between studies. All participants were screened before being included in the studies. Ten studies recruited a control group but only seven delivered a comparable number of matched controls (18, 34–36, 39*3, 40*3, 46*5). All studies performed the driving assessment (on-road or in a driving simulator) accurately (e.g., driving instructors blinded to group allocation) to minimize bias. Thirteen studies considered confounding factors appropriately (11*1, 12, 14, 16*5, 19*4, 32*1, 33*1, 39*3, 40*3, 41, 43, 45*4, 46*5). The quality of the results was evaluated as acceptable only in 11 studies (11*1, 12, 14, 15*2, 19*4, 37*2, 38*2, 39*3, 40*3, 42*2, 45*4).
Outcomes of included studies
On-road driving assessment
Fourteen studies (seven unique samples) used on-road driving to assess driving ability (Table 1). Twelve of the 14 on-road driving studies showed that 6 to 38 % of PwMS failed the on-road driving test (11*1–13*2, 15*2, 194, 32*1, 33*1, 37*2, 41, 42*2, 43, 454), with 10 out of 12 studies suggesting that between 17 and 23% of PwMS are unfit to drive (11*1–13*2, 15*2, 19*4, 32*1, 33*1, 37*2, 42*2, 45*4). Lincoln & Radford (41) reported the highest fail rate (38%, 13 out of 34 PwMS), while Ranchet et al. (43) observed a low fail rate (6 %). Akinwuntan et al. (12) had eight PwMS who initially failed the on-road assessment retake the test (seven of them trained in a driving simulator prior to the second on-road test). Five of the participants passed the second on-road assessment, while two PwMS in the training group and the one without training failed. Devos et al. (14) did not differentiate between “pass” and “fail” in their study but found that most PwMS demonstrated sub-maximal performance, with participants reaching a total on-road score of 184.15 (mean, SD 13.48) out of 196 in the Test Ride for Investigating Practical fitness-to-drive (TRIP). Adjustment to stimuli (i.e., responding to critical roadway information) and gap acceptance errors (i.e., errors in merging into a street traffic stream because the gap between two cars was miscalculated) was identified as indicators of failing the on-road test, because more PwMS who failed the on-road test had problems in those areas (13*2, 37*2, 38*2). Lane maintenance and speed regulation errors were also identified as predictors for failing the on-road test (15*2).
Assessment in a driving simulator
Ten studies (eight unique samples) assessed driving ability by a driving simulation (16*5–18, 34–36, 39*3, 40*3, 44, 46*5). Driving simulator measures and results are shown in Table 2. Eight out of 10 studies compared the driving ability of PwMS to healthy controls (HCs) (17, 18, 34–36, 39*3, 40*3, 46*5). Unlike the on-road studies, driving simulator studies did not evaluate whether PwMS passed or failed the driving assessment. Harand et al. (36) let PwMS drive in three different conditions: monotonous driving condition, divided attention (DA) condition, and urban driving condition (driving along an urban road with other cars). They found that PwMS showed significantly more difficulty in the standard deviation of lateral position (SDLP, i.e., a measure of road tracking errors, “weaving” of the car) than HC in the monotonous driving condition, as well as for the standard deviation of speed. They also had more difficulties driving in the DA condition than HC. No significant differences between groups were found in the urban driving condition, or in the number of accidents and collisions. Similarly, Marcotte et al. (18) found that PwMS had significantly greater SDLP than HC, drove significantly faster on the lane-tacking condition, and had greater variability in speed maintenance. Persons with multiple sclerosis also showed more difficulty in tracking the movements of the lead car and were slower to respond to changes in speed compared to HC. The groups did not differ in the degree to which they over- or under-compensated the distance from the lead car. Kotterba et al. (17) found that PwMS made more accidents compared to controls, and had more concentration faults (described as disregarding the speed limit, tracking errors, or disregarding traffic lights). There was no difference in distance driven in the allotted timeframe (60 min) between the two groups. Krasniuk et al. (39*3) used operational, tactical, and strategic driving maneuvers during two scripted events (i.e., traffic light changes colors and pedestrian walks out in front of the driver) and a navigational driving task to assess driving ability. PwMS made more adjustments to stimuli errors in the tactical driving maneuver than HC and had a slower response time in the pedestrian event, but they did not differ in the traffic light event (i.e., the groups did not differ in mean speed and whether they stopped or failed to stop). There were no between-group differences in the navigational driving task or reaction time in the pedestrian event. In another publication, on the same sample, Krasinuk et al. (40*3) found that PwMS had a shorter time to collision and a faster mean speed, which increased the odds of experiencing a rear-end collision than HC.
Two studies did not find any significant differences in driving performance between PwMS and HC (34, 46*5). Schultheis et al. (46*5) compared PwMS with cognitive impairment (CI), PwMS without CI (no CI), and HC using the Neurocognitive Driving Test (47) (NDT), in which one component was a driving simulation. They found that CI-PwMS performed significantly worse than both the no CI-PwMS and HC groups in the latency to perform several driving-specific functions on the NDT. No overall group differences were observed in actual errors on the NDT. They did not find any significant differences between HC and no CI-PwMS.
Impact of demographic factors
Age as a factor that impacts driving was assessed in nine samples (11*1, 14, 16*5, 17, 32*1, 34, 36, 37*2, 41, 42*2, 43) (Figure 2). No study found any relation between age and driving performance. Only one (41) found gender to have an impact on driving, with more women than men failing the driving task. In five samples (11*1, 14, 16*5, 32*1, 36, 37*2, 42*2), the impact of education on driving performance was assessed and only one (14) found a significant correlation. Four samples examined driving history, including experience, accidents/received traffic tickets/fines (11*1, 14, 32*1, 41, 43). Devos et al. (14) found a significant negative correlation between the number of traffic tickets and driving performance. Lincoln and Radford (41) found a significant difference in PwMS who passed vs. those who failed on the on-road test and the time since participants had last driven a car. In another sample (37*2, 42*2), significantly more employed participants passed the on-road test compared to participants who were unemployed at the time of testing. Participants' country of birth and ethnicity did not have any relation to driving ability.
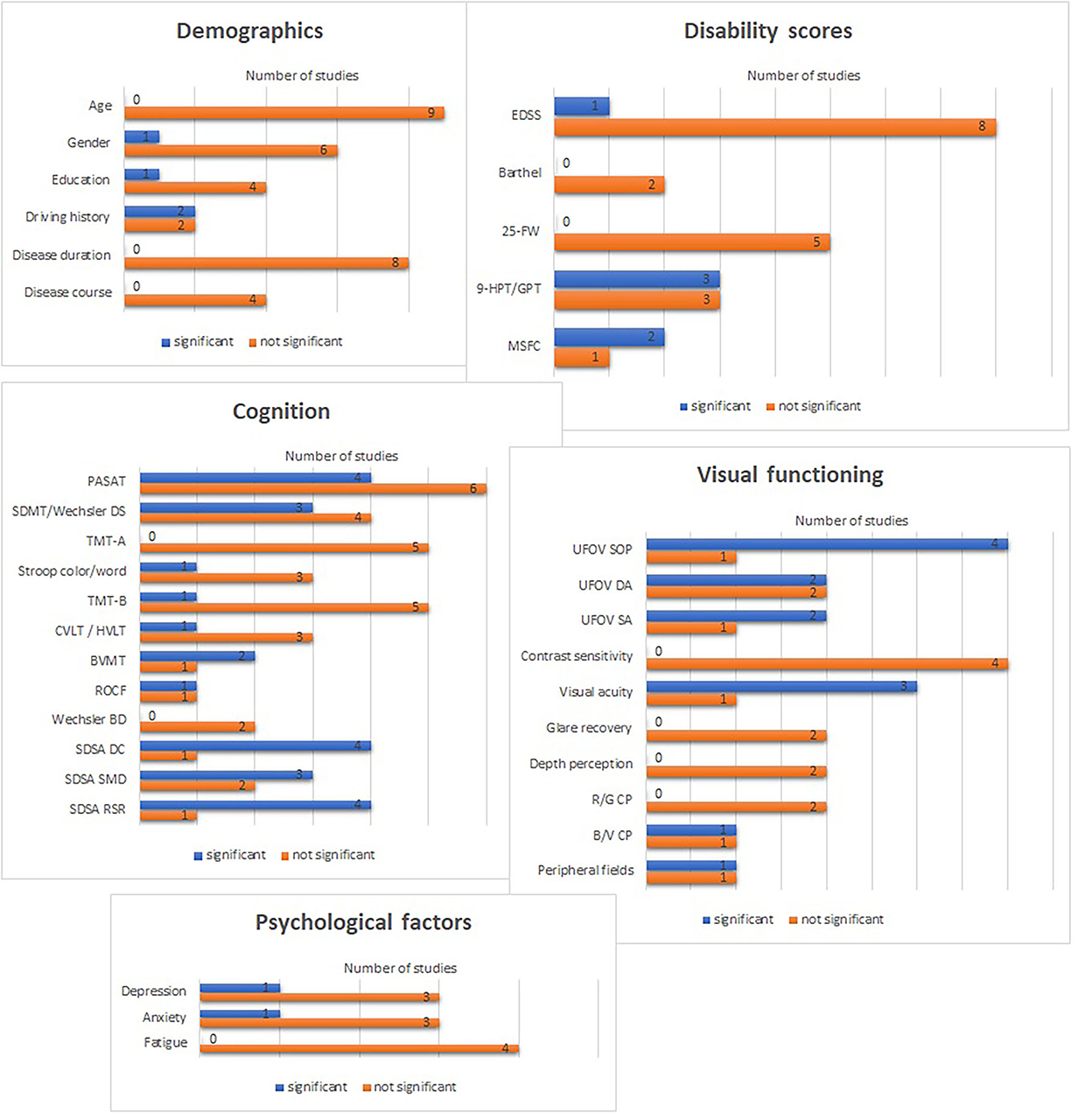
Figure 2. Impact of disease-related abilities on driving. EDSS, Expanded disability status scale; 25-FW, 25-foot walk-test; 9-HPT, 9 Hole peg test, GPT, Grooved pegboard test; MSFC, Multiple sclerosis functional composite; PASAT, The paced auditory serial addition test; SDMT, Symbol digit modalities test; DS, Digit symbol; TMT-A, Trail making test-A; TMT-B, Trail making test-B; CVLT, California verbal learning test; HVLT, Hopkins verbal learning test; BVMT, Brief visuospatial memory test; ROCF, Rey osterreith complex figure; BD, Block design; SDSA, Stroke driver screening assessment; DC, dot cancellation; SMD, Square matrices directions; RSR, Road sign recognition; UFOV, Useful field of view; SOP, Speed of processing; DA, Divided attention; SA, Selective attention; R/G CP, Red & green color perception; B/V CP, Blue & violet color perception.
Disease duration (including duration since first symptom and time since onset MS) was assessed in eight studies (11*1, 14, 16*5, 32*1, 34, 36, 37*2, 41, 422, 43), disease course in four (14, 34, 37*2, 42*2), and medication in one sample (37*2, 42*2), with no significant relations to driving found.
Impact of EDSS and motor function
In only one (19*4, 45*4) out of nine samples (11*1, 14, 17–19*4, 32*1, 34, 36, 37*2, 42*2, 44, 45*4) had a significant impact of EDSS on driving performance: patients with high EDSS failed the on-road test and performed significantly worse in driving. The Barthel index was assessed in two samples (11*1, 14, 32*1) and the 25-foot walk-Test (25FW-test) in five samples (11*1, 14, 17, 32*1, 34, 44). No significant results were found for either. The nine Hole Peg Test (9-HPT)/Grooved Pegboard Test (GPT) was used in six samples (11*1, 14, 17, 18, 32*1, 34, 44). Akinwuntan et al. (11*1) found significantly more impairment in hand functions in patients who failed the on-road test. Devos et al. (14) found significant correlations between 9-HPT and the driving performance score. Marcotte et al. (18) studied the impact of GPT on driving performance and found significant correlations between hand functions (dominant and non-dominant hand) with car following time delay. The multiple sclerosis functional composite (MSFC) was used in three samples (11*1, 16*5, 32*1, 44): one study (44) found greater variability in lane position in patients with higher impairment in MSFC and the other (16*5) found significant correlations between driving latency and hand functions measured by the MSFC.
Impact of cognition
Thirteen studies assessed cognition in relation to driving ability. We summarize the findings for each cognitive domain.
Attention and information processing
Ten studies used the PASAT to assess the capacity and rate of information processing (including sustained and divided attention). Only three of these found significant correlations between PASAT and driving performance measures (number of accidents, speed, latency) (16*5, 17, 34), and one sample found significant differences with the PASAT in PwMS who passed/failed the on-road test (11*1, 32*1). The Symbol Digit Modalities Test (SDMT)/Wechsler Digit Symbol (DS) was used in seven samples (11*1, 14, 15*2, 18, 19*4, 36, 39*3, 42*2). Devos et al. (14) found a significant correlation between SDMT performance and the driving protocol (Test Ride for Investigating Practical fitness-to-drive, TRIP test, with better performance in the SDMT relating to higher scores). Schultheis et al. (19*4) found better SDMT results in PwMS who passed the driving assessment than those who did not, and Marcotte et al. (18) found a significant correlation between the DS subtest and the Standard Deviation of Lateral Position (a measure of road tracking error) measured in a driving simulator. None found associations between Trail Making Test-A (TMT-A) and driving (11*1, 14, 18, 46*5).
Executive functions
The Stroop test was used in four studies (11*1, 12, 14, 41). Only Devos et al. (14) found significant correlations between the Stroop subtest color/word (C/W) with the TRIP. Only one (14) out of six studies (11*1, 14, 18, 19*4, 34, 46*5) found significant associations between Trail Making Test-B (TMT-B) and driving.
Learning and memory
One (18) out of four samples (15*2, 18, 19*4, 39*3, 42*2) that examined verbal learning and memory using the Hopkins Verbal Learning Test-Revised (HVLT-R)/California Verbal Learning (CVLT2) found significant correlations between lateral position and verbal learning and between car following time delay and delayed memory. Two samples (15*2, 39*3, 42*2) examined visuospatial learning and memory using the Brief Visuospatial Memory Test-Revised (BVMT-R). One found significant correlations between speed regulation errors and visuospatial memory (15*2), and one reported a significant association between impairment in immediate recall and failure on on-road driving (42*2).
Visuoconstructive functions
The Rey–Osterrieth Complex Figure (ROCF) (copy) to assess visuoconstructive abilities was used in two samples (11*1, 14, 32*1). Devos et al. (14) found significant correlations between the driving and visual-constructive functions. No significant associations between visuospatial functions (Wechsler Adult Intelligence Test [subtests BD]) and driving ability were found (11*1, 46*5).
Driving-related multi-domain measures
Four (11*1, 12, 14, 32*1, 41) out of five samples (11*1, 12, 14, 32*1, 34, 41) that examined the Stroke Driver Screening Assessment (SDSA) (subtests: dot cancellation [DC] [DC-time, DC-error, DC-false-positive], Square Matrices Directions [SMDs], Square Matrices Compass [SMC], road sign recognition [RSR]) found significant relations with driving abilities: three samples (11*1, 12, 32*1, 41) reported significant differences between passed vs. failed MS drivers in all subtests of the SDSA. Devos et al. (14) found significant correlations between on-road driving TRIP measures and the SDSA (DC-time, SMD, SMC, and RSR).
In total, 11 of the 13 studies which measured cognitive abilities did find a relation between cognition and driving performance.
Impact of psychological aspects
We found four studies examining psychological measures (depression, anxiety, fatigue) on driving (11*1, 14, 34, 36), but significant correlations were only found in one study between depression with time to collision (TTC), and anxiety with the simulation divided attention task (34). Fatigue did not significantly correlate with driving ability.
Impact of visual function
The impact of vision on driving was reported in eight studies. The UFOV (subtests: speed of processing [SOP], divided attention [DA], selective attention [SA]) was used in five studies. Two studies (11*1, 12) found significant differences in passed vs. failed participants in SOP and driving abilities. Classen et al. (13*2) reported significant correlations between SOP and gap acceptance errors. Devos et al. (14) found significant correlations between driving abilities and SOP, DA, and SA. Shawaryn et al. (16*5) used the UFOV to stratify PwMS into persons with low-risk and moderate/high-risk vision groups and found significantly lower driving ability scores in the low-risk group.
Four samples (13*2-15*2, 34, 43) examined the impact of acuity on driving performance: one study found a significant correlation between acuity and driving abilities (14), one reported a relation between binocular acuity and failing the on-road test (43), and one sample found a significant correlation between visual acuity and the on-road adjustment to stimuli measure (13*2, 15*2).
Four samples assessed the impact of contrast sensitivity on driving, and no significant associations were found (11*1, 13*2-15*2, 34). Two studies examined the impact of glare recovery on driving in MS and did not find significant associations (11*1, 14). They also examined red & green color perception (R/G CP) and blue & violet CP (B/V CP) (11*1, 14), and only one found significant differences between the driving passed vs. failed MS group in B/V CP (11*1). Depth perception was studied in two samples (11*1, 13*2, 15*2) and no significant associations were found. One study found significant correlations between the peripheral vertical measure and the on-road TRIP measure (14), but no significant associations between peripheral vision and driving ability were found in another sample (13*2, 15*2).
In total, seven of the eight studies that measured any visual aspect found a relation between at least one visual parameter and driving performance.
Predictors of driving performance
Different outcomes were investigated as possible predictors for driving performance (see Table 3). The outcomes used predicted driving ability with an accuracy ranging from 82 to 91%, with most analyses resulting in high specificity (79–98 %) but low sensitivity (25–80 %) (11*1, 12, 15*2, 19*4, 32*1, 38*2, 40*3, 41), except for Morrow et al. (42*2) who predicted pass or fail in the on-road test with 100 % sensitivity but low specificity (35.7%−53,57% depending on the combination of predictors). Commonly, the predictors tended to be related to the performance on cognitive tests (19*4, 32*1, 39*3, 41, 42*2), on predefined driving-related aspects assessed during the driving evaluation (15*2, 38*2, 40*3), or a combination of cognitive and visual tests (11*1, 12, 14). Other predictors included “having no history of driving as part of (past or current) employment” combined with cognitive tests (42*2), MSFC and education (16*5, 34), and physician recommendation on fitness to drive and binocular acuity (43). For cognitive tests, the predictors that were commonly used were SDSA (11*1, 32*1, 41), Stroop test (11*1, 14), and SDMT (19*4, 42*2). For vision, UFOV was found to be predictive of driving ability (11*1, 12).
Discussion
Our discussion is structured around three key areas in driving and MS research. After a brief discussion of the quality of the included studies, the first key aspect addresses the assessment and evaluation of driving ability. We then synthesize the results from the individual studies to consider the evidence of association between driving ability, demographic (e.g., age, gender, education, disease duration), disease characteristics (disability scores, cognition, psychological and visual impairments), and which outcomes predicted driving ability. We have organized these findings based on (i) full agreement between studies demonstrating an association between these variables and driving ability, (ii) mixed findings, and (iii) full agreement between studies demonstrating no associations. Finally, we discuss the impact of our findings on clinical practice and recommendations for further research.
Study quality
Most included studies were small cross-sectional studies with non-consecutively recruited participants. The studies were of satisfactory quality based on the CASP tool. Major problems identified were the selection of the control group, consideration of confounding factors, and presentation of study results (especially reporting of confidence intervals). Many studies assessed small, homogenous samples, which may have affected the results and limited the validity of study results.
Driving on-road vs. driving simulator
Between 6 and 38% of PwMS failed the on-road test, with the highest (41) and the lowest fail rates (43) being outliers. Most individual studies reported a fail rate between 17 and 23 %. In the study with the 38% fail rate, only seven of the 13 participants considered to have not passed actually failed the on-road test, while six others were counted as unfit to drive for not being able to participate on the on-road test for other reasons (e.g., poor eyesight, dexterity, etc.) (41). Counting only those participants who took and failed their on-road test reduces the fail rate to 25%, bringing it closer to the other studies. Ranchet et al. (43), conversely, observed a very low on-road test fail rate (6%). This study had the largest sample (n=218) in our review and was the only study in which the participants were neither volunteers nor patients in a clinic setting. Participants had to attend the on-road test for legal reasons (i.e., medical clearance for driving) (43). The large sample size and mandatory participation suggest that the fail rate may resemble the MS population more closely. However, the fail rate is comparatively very low, which might be because 74% (n=162) of the participants had been evaluated before, and had already passed the driving assessment at least once, while the people who previously failed were already removed from the total sample. Also, participants who are legally required to be assessed and research volunteers might prepare for and react to the on-road test differently (e.g., the former being more stressed because of the higher stakes for them). Furthermore, even though on-road tests are considered the gold standard for assessing driving ability, there are differences between the various on-road tests because they use differing protocols. Standardizing on-road tests is possible to a certain degree, but results may still vary depending on which route is taken, or which person is evaluating driving ability, and other factors (e.g., traffic). Furthermore, for most studies, the outcome of the on-road test is passing or failing, which shows that PwMS have impaired driving ability but fail to differentiate which domains are impaired.
Assessments using driving simulators are better suited to examine which driving domains are impaired, but show conflicting results. Some studies did not find any significant differences in driving performance between PwMS and HC (11*1, 46*5), while others did (17, 18, 36). Studies that found significant differences between both groups diverged as well. Harand et al. (36) did not find a difference in the number of accidents between PwMS and HC, while Kotterba et al. (17) showed that the accident rate was significantly higher in PwMS compared to controls. This might be in part due to the small number of PwMS assessed in most of these studies, ranging from 11 to 38 participants. In addition, Kotterba et al. (17) compared 31 PwMS with 10 HC which could have led to problems with statistical power. Overall, PwMS struggled with different aspects of the driving simulator. They performed worse in the standard deviation of lateral position (18, 36), made more adjustments to stimuli errors (39*3, 40*3), had greater variability in speed maintenance (18), and had more concentration faults (17).
Driving simulator settings could be useful in clinical practice in future to determine driving ability. However, because the driving simulators used in the studies differed, it is currently difficult to compare them not only with each other but also with on-road tests. Some presented different driving scenarios (36, 39*3, 40*3), while others evaluated more than just driving ability, e.g., pre-driving questions, reaction time task, and a visual task (16*5, 46*5). More research is needed to develop a gold standard for driving in simulators, because it allows people with severe impairments to be assessed safely. However, it is unclear how closely driving in a simulator mirrors real-world driving, with studies indicating that while driving simulators are frequently used, many are not fully validated (48), while other studies claim that performance in a driving simulator reflects real-life driving ability (49) and replicates experimental road conditions that on-road tests cannot replicate (50).
Impact of disabilities on driving ability
Different MS-related physical, cognitive, psychological, and visual impairments were found to negatively impact driving ability.
Consistent associations
The UFOV, which was used to study the impact of vision on driving ability, and the SDSA, which was used to analyze cognition in relation to driving, were two tests that stood out. This is in line with the review by Krasniuk et al. (22)who reported the UFOV and SDSA as the best measures to predict driving ability in MS. In our review, we found that for the UFOV, significant differences/correlations were reported in all five studies, albeit for different subtests (11*1, 12, 13*2, 14, 15*2, 16*5). Speed of processing (SoP) was especially found to impact driving ability. SoP was not associated with passing vs. failing in only one sample (13*2, 15*2), but did correlate with gap acceptance errors (13*2). These two papers had medium CASP ratings while the other four, with the exception of the Shawaryn et al. study (16*5), had higher quality, and might therefore be more reliable. Results regarding the UFOV are in line with prior research, reporting the UFOV as a potential predictor for driving ability (11*1, 12, 22).
The Stroke Driver Screening Assessment (SDSA) was used in five samples to assess the impact of cognition on driving ability (11*1, 12, 14, 32*1, 34, 41) and except for one study (34), all found significant results for the subtests Dot Cancellation and Road Sign Recognition. This study had medium quality in the CASP rating and a very small sample (n = 15) and might therefore have been underpowered. The quality of the other studies was rated higher. Both medium-quality studies did have a larger sample but lost points for not having a control group.
Visual acuity most consistently showed a significant impact on driving ability in multiple samples (three out of four) (13*2, 14, 15*2, 43). Devos et al. (34) did not find a significant relation, which again might be due to the small sample size.
Heterogeneous results
Most of the evidence presented in this review showed heterogeneous results. Two of the 13 studies investigating cognition did not find any relation between cognitive functioning and driving performance (39*3, 44), while another study did not find any differences in cognition between PwMS who passed vs. failed the driving task (15*2). Similarly, data was inconsistent, and in some cases contradictory, in different areas of cognition, such as learning and memory, attention and information processing, executive functions, visuo-constructive functions, and multi-domain measures. The two studies that did not find significant correlations between cognitive tests and driving ability were one of the lowest (44) and highest (39*3) quality-rated papers. However, the higher rated study did find that immediate verbal recall predicted adjustment to stimuli errors (39*3).
Physical aspects seem only partially related to driving ability, which might be due to the study samples in this review presenting PwMS with low to moderate physical disabilities, with some studies even excluding PwMS with high EDSS scores. Similarly, some visual aspects showed mixed results, with too little research to conclude. The impact of psychological aspects on driving ability was inconclusive for depression and anxiety, with each showing significant association in one out of four studies (34).
No associations
Demographic factors were consistently found to have little to no impact on driving ability. Similarly, MS-related factors like disease duration and disease course were not associated with driving ability. Other physical aspects showed no or only one significant association [e.g., EDSS in one out of nine samples (19*4, 45*4) (high CASP rating)]. One cognitive test that was shown to have no impact on driving ability was the TMT-A. For TMT-B, only one out of six studies (14) (high CASP rating) showed a significant association. Fatigue did not have a significant impact on driving ability.
Surprisingly, age and physical disability were not or only partially related to driving ability. This is a contrast to prior research on older drivers and other neurological diseases (such as Parkinson's disease), which show both aspects to be related to driving ability (51–54). Generally, prior research on driving ability in other cohorts and patient groups has shown that (1) age, (2) visual impairment, and (3) dementia or cognitive impairment were strong predictors for impaired driving (55, 56). While the results of the UFOV and the SDSA are in line with this, many other tests in both visual and cognitive functioning did not show convincing associations. This might be because the present cohort was relatively young, with mean ages from 36 to 55 years, homogeneous, and showed only low to moderate physical disability. Prior studies showed that older PwMS demonstrate more impairment in cognition than young and middle-aged PwMS (57) and that PwMS show a bigger decline in physical ability than in cognition in the first 10 years of MS (58). Therefore, the MS cohort could have been too young and not impaired enough to show significant differences. Another explanation could be that the tests used in the studies were not sensitive enough to show the differences.
Assessing driving ability in an older cohort of PwMS with more severe disabilities would thus be important to show whether age and MS-associated deficits become stronger predictors. Also, longitudinal studies on driving ability in PwMS are lacking but might be helpful to discover how driving ability changes over time. Driving simulators might be a safe alternative to assess the driving ability of this group without endangering them or other road users.
Clinical and scientific impact
The present evidence shows that one out of five (~20%) PwMS might not be able to drive safely, but there is no sure way for healthcare professionals to identify them without extensive testing. Since there is a lack of validated predictive test batteries for driving ability, not only for PwMS but for other cohorts as well (49, 59), more studies with standardized tests are needed to identify new possible predictors and to give a better idea of how strongly those predictors impact driving ability. Tests such as the UFOV and the SDSA may be helpful but can also be problematic because different studies show different subtests to be related to driving ability. For clinical practice, it is important to note that impairment in one area alone does not justify driving cessation, but when combined with other forms of impairment, it may be an indicator of unfitness to drive. The findings of this review show that while standardized guidelines for driving cessations are needed, the available evidence on driving ability in PwMS is not sufficient to develop these guidelines.
Driving simulators seem to be better suited to determine which areas in driving are impaired, as the outcomes are often more differentiated than in on-road assessments, which mostly consist of passing or failing the on-road test. Most studies presented in this review were of moderate quality in the CASP assessment, but only two studies (representing one sample) had high quality. Two key aspects that were found to be in need of improvement were the inclusion of comparable control groups and larger samples. Further high-quality research in driving in PwMS is needed to enable us to make more accurate predictions of fitness to drive, and to keep PwMS and other road users safe.
Data availability statement
The original contributions presented in the study are included in the article/Supplementary material, further inquiries can be directed to the corresponding author.
Author contributions
CB, CH, JP, and SSZ conceived the study and developed the protocol. JP and SSZ developed the search strategies, conducted the search, selected studies for the review, extracted the study data, and drafted the manuscript. CB, CH, RdN, JP, and SSZ revised the manuscript and approved the final version. All authors contributed to the article and approved the submitted version.
Funding
This study was sponsored via an independent grant by Merck Healthcare Germany GmbH, Weiterstadt, Germany (CrossRef Funder ID: 10.13039/100009945). The funder was not involved in the study design, collection, analysis, interpretation of data, the writing of this article or the decision to submit it for publication.
Acknowledgments
We would like to thank Katharina Wilkens, Elena Fricke, Anne Rahn, and Julia Peper for their assistance.
Conflict of interest
CB has received fees for advisory board participation from UCB Pharma and Zambon, as well as lecture fees from AbbVie Pharma, BIAL Pharma, TAD Pharma, UCB Pharma, and Zambon Pharma. CH received research grants from Merck, Novartis, and speaker honoraria from Merck and Roche. JP has received funding to present lectures on fatigue from GAIA AG, for investigator-initiated studies from Merck and Celgene. RdN has received funding to present lectures on cognitive screening and rehabilitation in MS (speakers' bureau) from Merck, Novartis, and Biogen. SSZ declares that the research was conducted in the absence of any commercial or financial relationships that could be construed as a potential conflict of interest.
Publisher's note
All claims expressed in this article are solely those of the authors and do not necessarily represent those of their affiliated organizations, or those of the publisher, the editors and the reviewers. Any product that may be evaluated in this article, or claim that may be made by its manufacturer, is not guaranteed or endorsed by the publisher.
Supplementary material
The Supplementary Material for this article can be found online at: https://www.frontiersin.org/articles/10.3389/fneur.2022.1056411/full#supplementary-material
References
1. Howard J, Trevick S, Younger DS. Epidemiology of multiple sclerosis. Neurologic Clin. (2016) 34:919–39. doi: 10.1016/j.ncl.2016.06.016
2. Chiaravalloti ND, DeLuca J. Cognitive impairment in multiple sclerosis. Lancet Neurology. (2008) 7:1139–51. doi: 10.1016/S1474-4422(08)70259-X
3. Coenen M, Basedow-Rajwich B, König N, Kesselring J, Cieza A. Functioning and disability in multiple sclerosis from the patient perspective. Chronic Illn. (2011) 7:291–310. doi: 10.1177/1742395311410613vspace*-2pt
4. Balcer LJ, Miller DH, Reingold SC, Cohen JA. Vision and vision-related outcome measures in multiple sclerosis. Brain. (2015) 138:11–27. doi: 10.1093/brain/awu335
5. Einarsson U, Gottberg K, Fredrikson S, Koch L, von Holmqvist LW. Activities of daily living and social activities in people with multiple sclerosis in Stockholm County. Clinical Rehabilitation. (2006) 20:543–51. doi: 10.1191/0269215506cr953oa
6. Hobart JC, Riazi A, Lamping DL, Fitzpatrick R, Thompson AJ. Measuring the impact of MS on walking ability: the 12-Item MS Walking Scale (MSWS-12). Neurology. (2003) 60:31–6. doi: 10.1212/WNL.60.1.31
7. Kister I, Chamot E, Salter AR, Cutter GR, Bacon TE, Herbert J. Disability in multiple sclerosis: a reference for patients and clinicians. Neurology. (2013) 80:1018–24. doi: 10.1212/WNL.0b013e3182872855
8. Owsley C, McGwin G. Vision and driving. Vision Res. (2010) 50:2348–61. doi: 10.1016/j.visres.2010.05.021
9. Lacherez P, Au S, Wood JM. Visual motion perception predicts driving hazard perception ability. Acta Ophthalmologica. (2014) 92:88–93. doi: 10.1111/j.1755-3768.2012.02575.x
10. Anstey KJ, Wood J. Chronological age and age-related cognitive deficits are associated with an increase in multiple types of driving errors in late life. Neuropsychology. (2011) 25:613–21. doi: 10.1037/a0023835
11. Akinwuntan AE, Devos H, Stepleman L, Casillas R, Rahn R, Smith S, et al. Predictors of driving in individuals with relapsing-remitting multiple sclerosis. Mult Scler. (2013) 19:344–50. doi: 10.1177/1352458512451944
12. Akinwuntan AE, Backus D, Grayson J, Devos H. Validation of a short cognitive battery to screen for fitness-to-drive of people with multiple sclerosis. Eur J Neurol. (2018) 25:1250–4. doi: 10.1111/ene.13690
13. Classen S, Krasniuk S, Morrow SA, Alvarez L, Monahan M, Danter T, et al. Visual correlates of fitness to drive in adults with multiple sclerosis. OTJR (Thorofare N J). (2018) 38:15–27. doi: 10.1177/1539449217718841
14. Devos H, Ranchet M, Backus D, Abisamra M, Anschutz J, Allison CD, et al. Determinants of on-road driving in multiple sclerosis. Archives Phys Med Rehabilitat. (2017) 98:1332–8.e2. doi: 10.1016/j.apmr.2016.10.008
15. Krasniuk S, Classen S, Monahan M, Danter T, He W, Rosehart H, et al. A strategic driving maneuver that predicts on-road outcomes in adults with multiple sclerosis. Transp Res F: Traffic Psychol Behav. (2019) 60:147–56. doi: 10.1016/j.trf.2018.10.014
16. Shawaryn MA, Schultheis MT, Garay E, DeLuca J. Assessing functional status: exploring the relationship between the multiple sclerosis functional composite and driving. Arch Phys Med Rehabil. (2002) 83:1123–9. doi: 10.1053/apmr.2002.33730
17. Kotterba S, Orth M, Eren E, Fangerau T, Sindern E. Assessment of driving performance in patients with relapsing-remitting multiple sclerosis by a driving simulator. ENE. (2003) 50:160–4. doi: 10.1159/000073057
18. Marcotte TD, Rosenthal TJ, Roberts E, Lampinen S, Scott JC, Allen RW, et al. The contribution of cognition and spasticity to driving performance in multiple sclerosis. Arch Phys Med Rehabil. (2008) 89:1753–8. doi: 10.1016/j.apmr.2007.12.049
19. Schultheis MT, Weisser V, Ang J, Elovic E, Nead R, Sestito N, et al. Examining the relationship between cognition and driving performance in multiple sclerosis. Arch Phys Med Rehabil. (2010) 91:465–73. doi: 10.1016/j.apmr.2009.09.026
20. Lings S. Driving accident frequency increased in patients with multiple sclerosis. Acta Neurol Scand. (2002) 105:169–73. doi: 10.1034/j.1600-0404.2002.1o165.x
21. Dehning M, Kim J, Nguyen CM, Shivapour E, Denburg NL. Neuropsychological performance, brain imaging, and driving violations in multiple sclerosis. Arch Phys Med Rehabil. (2014) 95:1818–23. doi: 10.1016/j.apmr.2014.05.022
22. Krasniuk S, Classen S, Morrow SA, Tippett M, Knott M, Akinwuntan A. Clinical determinants of fitness to drive in persons with multiple sclerosis: systematic review. Arch Phys Med Rehabil. (2019) 100:1534–55. doi: 10.1016/j.apmr.2018.12.029
23. Nouri FM, Lincoln NB. Validation of a cognitive assessment: predicting driving performance after stroke. Clin Rehabil. (1992) 6:275–81. doi: 10.1177/026921559200600402
24. Ball K, Owsley C. The useful field of view test: a new technique for evaluating age-related declines in visual function. J Am Optom Assoc. (1993) 64:71–9.
26. Bro T, Lindblom B. Strain out a gnat and swallow a camel? Vision and driving in the Nordic countries. Acta Ophthalmologica. (2018) 96:623–30. doi: 10.1111/aos.13741
27. Wood JM. 2015 Glenn A fry award lecture: driving toward a new vision: understanding the role of vision in driving. Optometry Vision Sci. (2019) 96:626–36. doi: 10.1097/OPX.0000000000001421
28. Fragoso YD, Rocha FB, Beloni TM, Orquiza B, Rosa PB, Donadel RW, et al. Driving ability among patients with multiple sclerosis: can legislation be provided on the matter? Crit Rev Phys Rehabil Med. (2016) 28:215–24. doi: 10.1615/CritRevPhysRehabilMed.2017020023
29. Marottoli RA, Mendes de Leon CF, Glass TA, Williams CS, Cooney LM, Berkman LF, et al. Driving cessation and increased depressive symptoms: prospective evidence from the New Haven EPESE established populations for epidemiologic studies of the elderly. J Am Optom Assoc. (1997) 45:202–6. doi: 10.1111/j.1532-5415.1997.tb04508.x
30. Windsor TD, Anstey KJ, Butterworth P, Luszcz MA, Andrews GR. The role of perceived control in explaining depressive symptoms associated with driving cessation in a longitudinal study. Gerontologist. (2007) 47:215–23. doi: 10.1093/geront/47.2.215
31. Critical Appraisal Skills Programme. CASP Case Control Study Checklist. (2022). Available online at: https://casp-uk.b-cdn.net/wp-content/uploads/2018/03/CASP-Case-Control-Study-Checklist-2018_fillable_form.pdf
32. Akinwuntan AE, O'Connor C, McGonegal E, Turchi K, Smith S, Williams M, et al. Prediction of driving ability in people with relapsing-remitting multiple sclerosis using the stroke driver screening assessment. Int J MS Care. (2012) 14:65–70. doi: 10.7224/1537-2073-14.2.65
33. Akinwuntan AE, Devos H, Baker K, Phillips K, Kumar V, Smith S, et al. Improvement of driving skills in persons with relapsing-remitting multiple sclerosis: a pilot study. Arch Phys Med Rehabil. (2014) 95:531–7. doi: 10.1016/j.apmr.2013.08.294
34. Devos H, Brijs T, Alders G, Wets G, Feys P. Driving performance in persons with mild to moderate symptoms of multiple sclerosis. Disabil Rehabil. (2013) 35:1387–93. doi: 10.3109/09638288.2012.731468
35. Devos H, Alissa N, Lynch S, Sadeghi M, Akinwuntan AE, Siengsukon C. Real-time assessment of daytime sleepiness in drivers with multiple sclerosis. Mult Scler Relat Disord. (2021) 47:102607. doi: 10.1016/j.msard.2020.102607
36. Harand C, Mondou A, Chevanne D, Bocca ML, Defer G. Evidence of attentional impairments using virtual driving simulation in multiple sclerosis. Mult Scler Relat Disord. (2018) 25:251–7. doi: 10.1016/j.msard.2018.08.005
37. Krasniuk S, Classen S, Morrow SA, Monahan M, Danter T, Rosehart H, et al. Driving errors that predict on-road outcomes in adults with multiple sclerosis. OTJR (Thorofare N J). (2017) 1539449217708554. doi: 10.1177/1539449217708554
38. Krasniuk S, Classen S, Morrow SA, He W. Driving environments that influence on-road outcomes in persons with Multiple Sclerosis. Transp Res F: Traffic Psychol Behav. (2020) 70:191–8. doi: 10.1016/j.trf.2020.03.003
39. Krasniuk S, Classen S, Morrow SA, Alvarez L, He W, Srinivasan S, et al. Clinical predictors of driving simulator performance in drivers with multiple sclerosis. Mult Scler. (2021) 27:2085–92. doi: 10.1177/1352458521992507
40. Krasniuk S, Classen S, Morrow SA. Driving errors that predict simulated rear-end collisions in drivers with multiple sclerosis. Traffic Inj Prev. (2021) 22:212–7. doi: 10.1080/15389588.2021.1883008
41. Lincoln NB, Radford KA. Cognitive abilities as predictors of safety to drive in people with multiple sclerosis. Mult Scler. (2008) 14:123–8. doi: 10.1177/1352458507080467
42. Morrow SA, Classen S, Monahan M, Danter T, Taylor R, Krasniuk S, et al. On-road assessment of fitness-to-drive in persons with MS with cognitive impairment: a prospective study. Mult Scler. (2018) 24:1499–506. doi: 10.1177/1352458517723991
43. Ranchet M, Akinwuntan AE, Tant M, Neal E, Devos H. Agreement between physician's recommendation and fitness-to-drive decision in multiple sclerosis. Arch Phys Med Rehabil. (2015) 96:1840–4. doi: 10.1016/j.apmr.2015.06.010
44. Raphail A-MA, Vickers KL, Leist TP, Schultheis MT. The relationship between multiple sclerosis symptom severity measures and performance on driving variability metrics in a virtual reality simulator. Am J Phys Med Rehabil. (2020) 99:278–84. doi: 10.1097/PHM.0000000000001351
45. Schultheis MT, Weisser V, Manning K, Blasco A, Ang J. Driving behaviors among community-dwelling persons with multiple sclerosis. Arch Phys Med Rehabil. (2009) 90:975–81. doi: 10.1016/j.apmr.2008.12.017
46. Schultheis MT, Garay E, DeLuca J. The influence of cognitive impairment on driving performance in multiple sclerosis. Neurology. (2001) 56:1089–94. doi: 10.1212/WNL.56.8.1089
47. Schultheis MT, Chute DL. An ecologically valid driving assessment program: The neurocognitive driving test. Arch Clini Neuropsychol. (1999) 14:794–5. doi: 10.1093/arclin/14.8.794a
48. Wynne RA, Beanland V, Salmon PM. Systematic review of driving simulator validation studies. Saf Sci. (2019) 117:138–51. doi: 10.1016/j.ssci.2019.04.004
49. Uc EY, Rizzo M. Driving and neurodegenerative diseases. Curr Neurol Neurosci Rep. (2008) 8:377–83. doi: 10.1007/s11910-008-0059-1
50. Rizzo M, Uc EY, Dawson J, Anderson S, Rodnitzky R. Driving difficulties in Parkinson's disease. Mov Disord. (2010) 25:136–40. doi: 10.1002/mds.22791
51. Dubinsky RM, Gray C, Husted D, Busenbark K, Vetere-Overfield B, Wiltfong D, et al. Driving in Parkinson's disease. Neurology. (1991) 41:517–20. doi: 10.1212/WNL.41.4.517
52. Ratnapradipa KL, Pope CN, Nwosu A, Zhu M. Older driver crash involvement and fatalities, by age and sex, 2000-2017. J Appl Gerontol. (2021) 40:1314–9. doi: 10.1177/0733464820956507
53. Uc EY, Rizzo M, Johnson AM, Dastrup E, Anderson SW, Dawson JD. Road safety in drivers with Parkinson disease. Neurology. (2009) 73:2112–9. doi: 10.1212/WNL.0b013e3181c67b77
54. Singh R, Pentland B, Hunter J, Provan F. Parkinson's disease and driving ability. J Neurology, Neurosurg Psychiat. (2007) 78:363–6. doi: 10.1136/jnnp.2006.103440
55. Emerson JL, Johnson AM, Dawson JD, Uc EY, Anderson SW, Rizzo M. Predictors of driving outcomes in advancing age. Psychol Aging. (2012) 27:550–9. doi: 10.1037/a0026359
56. Toepper M, Schulz P, Beblo T, Driessen M. Predicting on-road driving skills, fitness to drive, and prospective accident risk in older drivers and drivers with mild cognitive impairment: the importance of non-cognitive risk factors. J Alzheimers Dis. (2021) 79:401–14. doi: 10.3233/JAD-200943
57. Baird JF, Cederberg KL, Sikes EM, Jeng B, Sasaki JE, Sandroff BM, et al. Changes in cognitive performance with age in adults with multiple sclerosis. Cogn Behav. (2019) 32:201–7. doi: 10.1097/WNN.0000000000000200
58. Beckerman H, Kempen JC, Knol DL, Polman CH, Lankhorst GJ, Groot V de. The first 10 years with multiple sclerosis: the longitudinal course of daily functioning. J Rehabil Med. (2013) 45:68–75. doi: 10.2340/16501977-1079
Keywords: multiple sclerosis (MS), driving, on-road driving, driving simulator, cognition
Citation: Seddiq Zai S, Heesen C, Buhmann C, das Nair R and Pöttgen J (2022) Driving ability and predictors for driving performance in Multiple Sclerosis: A systematic review. Front. Neurol. 13:1056411. doi: 10.3389/fneur.2022.1056411
Received: 28 September 2022; Accepted: 07 November 2022;
Published: 30 November 2022.
Edited by:
Emilio Portaccio, Careggi University Hospital, ItalyReviewed by:
Abiodun E. Akinwuntan, University of Kansas Medical Center, United StatesElisabeth Gulowsen Celius, Oslo University Hospital, Norway
Copyright © 2022 Seddiq Zai, Heesen, Buhmann, das Nair and Pöttgen. This is an open-access article distributed under the terms of the Creative Commons Attribution License (CC BY). The use, distribution or reproduction in other forums is permitted, provided the original author(s) and the copyright owner(s) are credited and that the original publication in this journal is cited, in accordance with accepted academic practice. No use, distribution or reproduction is permitted which does not comply with these terms.
*Correspondence: Susan Seddiq Zai, cy5zZWRkaXEtemFpQHVrZS5kZQ==