- Department of Neurology, Shanghai Tenth People's Hospital, Tongji University School of Medicine, Shanghai, China
Objective: Currently, the risk of occult atrial fibrillation (AF) could not be predicted in patients with acute ischemic stroke (AIS) using a simple scoring system. Therefore, in this study, we developed and externally validated a nomogram to predict occult AF in patients with AIS.
Methods: In this study, we prospectively conducted a development cohort study with data collected at our stroke center from July 2017 to February 2018, and an external validation cohort from March 2019 to December 2019.
Results: Follow-up data were collected from 177 participants (56.5% older than 65 years, 29.4% female) for generating the nomogram model. Multivariate logistic regression analysis was performed with AF as the dependent variable indicated that age >65 years, heart rate >100, C-reactive protein (CRP), N-terminal pro-B-type natriuretic peptide (NT-proBNP) >270, hemorrhagic transformation (HT) as independent variables for predicting the development of AF, and a nomogram was generated based on these factors. The area under the receiver operating characteristic curve (AUC-ROC) for the model was 0.937, the C-index was 0.926, and the AUC-ROC for the validation cohort was 0.913.
Conclusion: To our knowledge, this is the first nomogram developed and externally validated in a stroke center cohort for individualized prediction of risk of developing AIS in patients with occult AF. This nomogram could provide valuable information for the screening of occult AF after a stroke.
Introduction
Atrial fibrillation (AF) increases the risk of stroke by four to five times (1), and according to early estimates of the prevalence of AF, 15%−20% of strokes are caused by AF (2). AF-related stroke generally has a poor clinical outcome, although anticoagulation is largely preventable (64% lower risk of stroke and 25% lower mortality) (3). Currently, 12 million ischemic stroke cases are reported each year, and one in four strokes and half of transient ischemic attacks (TIAs) have no established cause after standard diagnostic testing and are marked as “cryptogenic” (4). Undiagnosed AF is believed as the cause of many cryptogenic strokes (CSs); however, anticoagulation is not indicated unless AF is proven. Because patients with AF have an increased risk of recurrent stroke, improved detection and treatment strategies for AF are expected to reduce the burden of recurrent stroke. The detection of AF has a substantial impact on the treatment decisions of patients with ischemic stroke. Subclinical AF is one of the pathogenesis of embolic stroke of unknown origin (ESUS) (5). According to statistics, AF accounts for approximately 8%−15% of ESUS cases (5, 6); even with direct oral anticoagulation, empirical anticoagulation is no better than aspirin in preventing recurrent strokes without documented AF (7, 8). Therefore, in the absence of documented AF, aspirin remains the standard antithrombotic therapy (9). Nevertheless, in patients with ESUS, the annual recurrence rate with standard antithrombotic therapy remains as high as 5% (10). Therefore, early etiological classification of patients with acute ischemic stroke (AIS) can select a reasonable secondary prevention program for the patients. However, the screening of some types of AF is not easy, and accurate etiological classification is highly dependent on the results of complete auxiliary examinations. Occult AF is often transient and asymptomatic, so there are challenges in clinical screening work (11, 12). The Chinese National Stroke Registry data showed that the proportion of stroke or TIA patients with AF in China is only 6.7% (13), while a large-scale registration study conducted in Europe showed that the proportion is 38% (14); thus, it can be seen that the detection rate of AF in China is significantly lower than that in Europe. Similarly, in terms of secondary prevention in patients with AIS complicated by AF, the situation in China is also significantly worse than that in developed countries. Compared with foreign countries, in China, the diagnosis rate of AF is low, and the missed diagnosis rate is high. Screening for this risk factor makes the diagnosis of occult AF difficult. If a reliable screening method can be found to preliminarily predict the possibility of cerebral embolism caused by occult AF in patients with AIS, it can guide further targeted examinations to determine whether it is occult AF, which will greatly improve the diagnosis rate of occult AF. At present, there is no well-recognized and very effective primary screening method for differential diagnosis. Therefore, the importance of screening AF is obvious. Screening high-risk patients, examining them more accurately, and improving the etiological diagnosis of occult AF will not only affect the direction of treatment but also guide the choice of stroke prevention programs in this group.
Methods
Study design and patient enrollment
This is a longitudinal study aimed at developing and validating prediction models. A total of 401 patients with AIS who were hospitalized in the Stroke Center of the Tenth People's Hospital affiliated with Tongji University in Shanghai from July 2017 to February 2019 were consecutively recruited. Participants met the following criteria: (1) 18 years of age or older and (2) diagnosed with AIS based on diffusion-weighted magnetic resonance imaging (MRI) within 1 week, and patients were excluded if key data were missing. The validation cohort included 65 stroke subjects without previous documented AF who were admitted from the same stroke center between March 2019 and December 2019. The inclusion/exclusion criteria for the development cohort also apply to the external validation cohort. AF was defined as a prior history or diagnosis of AF after hospital discharge. A history of a previous stroke was defined as a history of a previous ischemic stroke or TIA. According to our stroke center clinical reference value range, a high heart rate on admission was defined as a heart rate >100 beats/min, high TnT was defined as a TnT >0.014 ng/ml, and high NT-proBNP was defined as an NT-proBNP >270 pg/ml. Age and AF-related ROC curves showed an optimum sensitivity and specificity trade-off at age 65 years, so patients were grouped according to the optimal age cutoff of 65 years, the elderly patients are defined as those of age >65 years. HT is defined as the first head CT/MRI after AIS without bleeding, and the second head CT/MRI examination hemorrhagic infarction can be identified by the finding of intracranial hemorrhage or by the first CT/MRI findings. Multiple ischemic lesions in MRI are defined as infarcts caused by the occlusion of two or more different cerebral vessels in the blood supply system. Shanghai Tenth People's Hospital Ethics Committee approved this study, and all participants and their carers provided written informed permission.
Data collection
Demographic data such as sex and age, current medical history, vascular risk factors, and the National Institutes of Health Stroke Scale (NIHSS) admission scores were collected. Laboratory test data such as routine blood count, blood biochemistry, and coagulation function were collected from all patients based on medical records during hospitalization. Relevant imaging data, including brain imaging, routine electrocardiogram, transthoracic echocardiography, and 24-h Holter heart rate test were also collected. All patients included in the external examination of the model were examined by a 24-h dynamic electrocardiogram. Based on whether AF was present or not, patients were divided into AF and non-AF groups.
Statistical analysis
Continuous quantitative variables are shown as mean and standard deviation, while categorical variables are shown as frequencies and ratios. First, the normal distribution of quantitative variables was determined using the Kolmogorov–Smirnov test. Student's t-test was then used to compare normally distributed quantitative data, while the chi-square test was employed to compare qualitative variables. The Mann–Whitney U-test was used to compare non-normally distributed variables. Based on the odds ratio (OR) and 95% confidence interval (CI), we conducted a logistic regression analysis with a probability of entry set to 0.05 and a probability of removal set to 0.10. To build the prediction model, variables with a p-value of < 0.05 in the multivariate analysis were incorporated into the R language. AUC-ROC curves were used to calculate the predictive accuracy of nomogram models to differentiate patients with AF. Then, we used the bootstrap method (1,000 resamplings) for internal verification and to calculate a revised C-index, which is equivalent to a range of 0.5–1.0 for AUC-ROC. The higher the score, the more accurate the prediction. Models built from the development cohort were then applied to an external validation cohort, and performance was evaluated by AUC-ROC. Calibration of the risk prediction model in the development cohort was performed by comparing the observed AF probabilities according to the nomogram-based total score with the nomogram-based predicted probabilities and assessed using the Hosmer–Lemeshow test whether event rates observed in patients with AIS match expected rates. In all cases, a P-value of < 0.05 is considered statistically significant. We used R software version 3.6.2 (2019 R Foundation for Statistical Computing platform), SPSS 24 (IBM Corporation, New York, USA), and GraphPad Prism 7 (GraphPad Software, La Jolla, CA, USA) for the analysis.
Results
Development cohort characteristics
Of all 401 patients, 224 were excluded from data analysis due to incomplete examination and lack of baseline data; finally, 177 patients were analyzed. Compared with included patients, those excluded due to the lack of baseline data had no significant difference in terms of age >65 (59.8 vs. 56.5%), female gender (30.8 vs. 29.4%), NIHSS on admission (3.53 ± 3.88 vs. 3.48 ± 3.84), hypertension (66.1 vs. 74%), diabetes mellitus (37.5 vs. 40.1%), AF (29.0 vs. 37.9%), and p >0.05 for all (Supplementary Table S1). As shown in Table 1, the mean age was 68.7 ± 12.1 years, 52 patients (29.3%) were women, and the mean NIHSS score at presentation was 3.5 ± 3.1. A total of 67 patients (49 were previously diagnosed, and 18 were diagnosed after discharge) were identified as having AF. Univariate analysis found that age >65, sex, NIHSS score, hypertension, history of the previous stroke, heart rate >100, aortic sinus diameter, left atrial diameter, CRP, platelet count, D-D dimer, triglyceride, high-density lipoprotein, TnT > 0.014, NT-proBNP > 270, HT, and multiple ischemic lesions in MRI were significantly different between AF and non-AF groups (P < 0.05 for all). Multivariate logistic regression was conducted with these factors.
The development of an individualized prediction model
In binary logistic analysis, sex, NIHSS score, hypertension, history of previous stroke, heart rate, aortic sinus diameter, left atrial diameter, platelet count, D-D dimer, triglyceride, high-density lipoprotein, TnT > 0.014, and multiple ischemic lesions in MRI were excluded because they were not statistically significant. As shown in Table 2, for the development of the model, five potential predictors were generated by multivariate logistic regression (LR method): age > 65 (OR, 4.95, 95% CI, 1.18–20.76; p = 0.029), heart rate > 100 (OR, 8.04, 95% CI, 1.99–32.48; p = 0.003), CRP (OR, 1.06, 95% CI, 1.00–1.11; p = 0.042), NT-proBNP > 270 (OR, 20.01, 95% CI, 4.27–93.74; p = 0.0001), and HT (OR, 32.24, 95% CI, 2.47–420.79; p = 0.008). The nomogram was used to build a prediction model. The point values of each factor used to calculate the total score are summarized as shown in Figure 1. The area under the ROC curve for the developed validation was 0.937, with a sensitivity of 95.5% and a specificity of 85.5% (Figure 2).
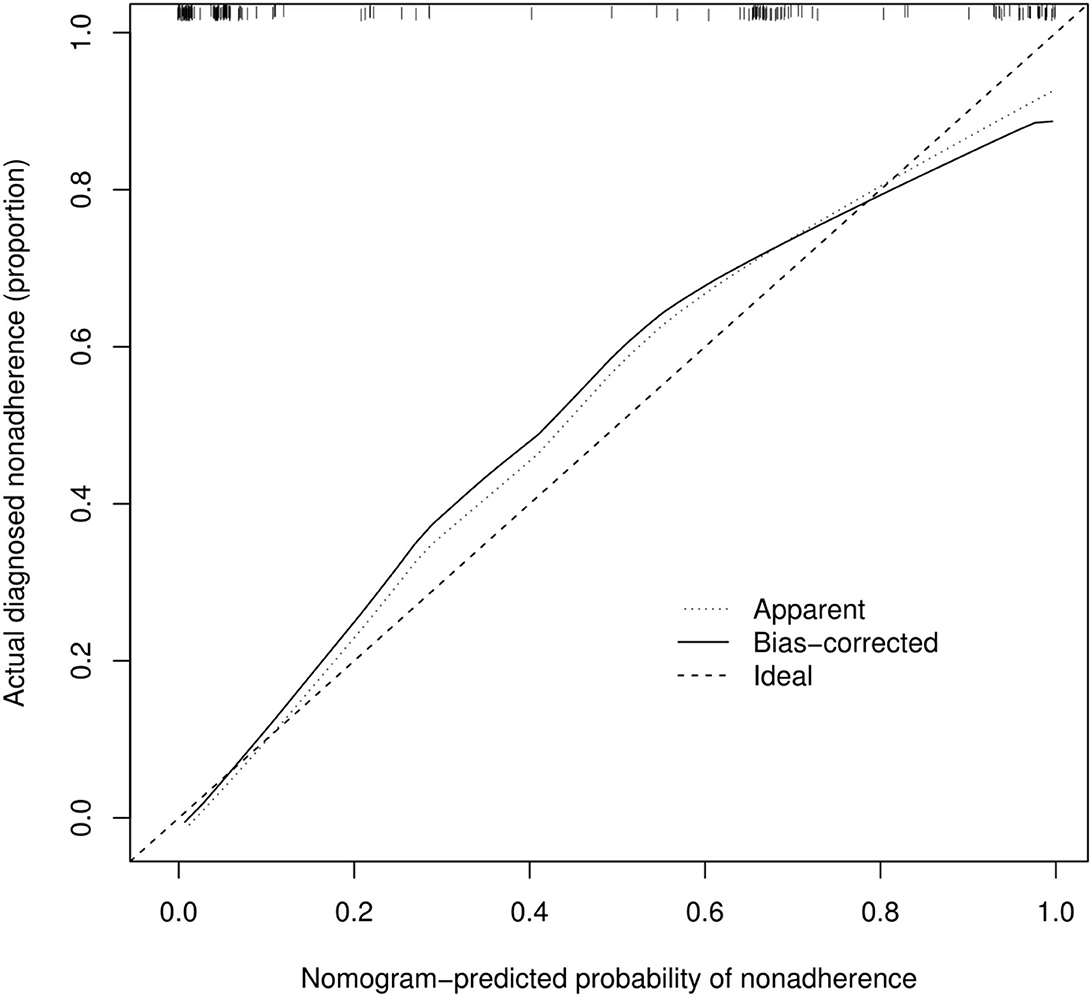
Figure 2. Predictive model based on logistic analysis for early diagnosis of AF in stroke in development and validation cohorts.
External validation and apparent performance of the occult AF risk nomogram
According to Figure 3, the calibration curve of the nomogram for predicting occult AF in patients with AIS in this cohort showed good agreement. The resulting estimated AUC value of 1,000 bootstrap samples is 0.937, indicating that the model has good discrimination. The validation cohort's area under the ROC curve was 0.913, as illustrated in Figure 2, with a sensitivity of 86.7% and a specificity of 87.8%. Therefore, in both the internal and external variations, our nomogram had excellent prediction performance, suggesting the nomogram based on the five existing risk factors has a high degree of generalizability.
Discussion
In this study, the risk factors associated with AF in patients with stroke were identified using logistic regression analysis. In addition, we developed and externally validated a screening model for predicting occult AF in patients with stroke to facilitate the screening of high-risk patients with occult AF.
Studies have shown that cryptogenic stroke (CS) accounts for about 35% of all ischemic strokes (15), and the literature shows that approximately 67% of patients initially diagnosed with CS are found to have evidence of underlying cardioembolic stroke after a period of follow-up (16). Meanwhile, the main cause of cardioembolic stroke is AF. Compared with paroxysmal AF, persistent AF and permanent AF are easier to diagnose. We have observed from clinical work that paroxysmal AF is more likely to be missed during hospitalization. Many studies have reported that the risk of AIS due to paroxysmal AF is not significantly different from persistent AF and permanent AF (17). In addition, ECG monitoring for patients with AIS lasting >48 h can increase the detection rate of AF by 17.5–21.3% (18). However, the detection of AF, especially paroxysmal AF, remains limited due to the uneven distribution of medical resources.
Hence, we established a nomogram based on age, heart rate, CRP, NT-proBNP, and HT to accurately predict the probability of occult AF in patients with AIS. By combining readily available clinical information with this model, clinicians can predict occult AF in patients with AIS rapidly and personally.
Both our findings and earlier research indicate that the association of AF with stroke increases with age (14, 19). In clinical work, the CHA2DS2-VASc score is widely used clinically to evaluate whether patients with a definite diagnosis of AF need anticoagulation, and factors such as age >65 years in the scoring system are considered to be relatively higher risk. Apart from advanced age, some studies have shown that NT-proBNP is also an independent predictor of paroxysmal AF (20). NT-proBNP has the advantages of a long half-life and good stability and is more suitable for clinical use. Serum NT-proBNP levels were considerably greater in patients with AF than in sinus rhythm controls (21). Lucie Garnie et al. (22) suggested that post-stroke AF was independently predicted by NT-proBNP. Our data further demonstrate the close association of NT-proBNP with AF in patients with AIS. The STAF scoring system developed by Suissa et al. (19) found that higher NIHSS scores, left atrial enlargement, asymptomatic extracranial stenosis (I > 50%), or cavitary infarction syndrome were associated with AF in patients with stroke. However, a prospective study from China showed that the STAF score has only moderate sensitivity and specificity in detecting AF in patients with stroke, with a relatively limited ability to predict AF, especially paroxysmal and new-onset AF (23).
In addition to the aforementioned factors, higher CRP at admission was also a strong modifiable predictor of AF. A previous study found that atrial inflammation is an important factor in the pathogenesis of AF. In long-term persistent/permanent AF, inflammatory infiltration and CRP levels in blood were significantly increased, and it was found that the degree of atrial inflammation was closely related to CRP blood levels (24), and the significance of CRP in predicting occult AF in individuals with AIS is further supported by our investigation. In our model, the strongest predictor of AF was HT. In fact, few studies have explored the relationship between HT and stroke complicated with AF, whereas a recent article published in Stroke pointed out that AF is associated with cerebral parenchymal hematoma and symptomatic intracranial hemorrhage after AIS (25). In multivariate analysis, wide 95% confidence intervals of HT and NT-proBNP suggest that these patients have a lower overall incidence of events and a higher incidence of AF. The blood biochemical, ultrasonography, imaging, and other indicators used for screening AIS complicated with occult AF have the characteristics of non-invasiveness, simple detection operation, and low price, which are more convenient for clinical application and promotion, and provide a new alternative diagnostic method for the diagnosis of cardiogenic embolism. Therefore, for high-risk groups, more accurate examinations such as long-term electrocardiography can be carried out in a targeted manner, observe the condition closely, repeat multiple examinations, increase the detection rate of AF, choose a more appropriate antithrombotic plan, thereby reducing the risk of cardioembolic stroke and death.
There are several limitations that need to be emphasized. First, in the developed validation, we included all patients with stroke in the study, not just those with cryptogenic stroke. We did this because the sample size is small and the number of ESUS is relatively small, and it is difficult to establish a validation model in the development cohort if we focus only on patients with ESUS, but external validation of the predictive model for occult AF in the ESUS patient cohort further confirmed the reliability of the model, which may compensate for the shortcomings introduced by our experimental design. Second, due to the limited conditions and the number of days the patients were in the hospital, we only performed 24-h dynamic electrocardiogram and not long-term ECG monitoring for all patients in the validation cohort, which leaves our prediction model somewhat deficient in detecting occult atrial AF. However, due to the limited conditions at our stroke center and the number of days the patients were in the hospital, it was difficult for us to complete long-term ECG monitoring such as 14 vs. 30 days of external cardiac monitoring during hospitalization, and the long-term ECG follow-up will be refined as much as possible in future clinical work. Third, compared with included patients, those excluded due to lack of baseline data showed a trend toward lower hypertension and AF (both p < 0.10), and there may be an inadvertent selection bias in place. Finally, this study is a single-center study with the problems of a single population and a small sample size, which may cause natural bias. If possible, external validation should be considered in a multicenter cohort with a large number of patients.
Conclusion
This study provides a simple screening model for occult AF in acute stroke patients. This externally validated nomogram provides clinicians with a new tool that is more useful than traditional patient consultation risk scores because it makes it easy and fast to visualize each stroke survivor's risk.
Data availability statement
The original contributions presented in the study are included in the article/Supplementary material, further inquiries can be directed to the corresponding authors.
Ethics statement
The studies involving human participants were reviewed and approved by Shanghai Tenth People's Hospital, Tongji University School of Medicine, 301 Middle Yanchang Road, Shanghai 200072, China. The patients/participants provided their written informed consent to participate in this study. Written informed consent was obtained from the individual(s) for the publication of any potentially identifiable images or data included in this article.
Author contributions
YZ and XL conceived and designed the study. XW collected and analyzed the data. LM and XW drafted the article. All authors read and approved the version of the final manuscript.
Funding
This work was supported by grants from the National Natural Science Foundation of China (Nos. 82101537 and 82071206) and the Science and Technology Commission of Shanghai Municipality (Nos. 22Y31900204 and 20142202900).
Acknowledgments
The authors are grateful to the study participants.
Conflict of interest
The authors declare that the research was conducted in the absence of any commercial or financial relationships that could be construed as a potential conflict of interest.
Publisher's note
All claims expressed in this article are solely those of the authors and do not necessarily represent those of their affiliated organizations, or those of the publisher, the editors and the reviewers. Any product that may be evaluated in this article, or claim that may be made by its manufacturer, is not guaranteed or endorsed by the publisher.
Supplementary material
The Supplementary Material for this article can be found online at: https://www.frontiersin.org/articles/10.3389/fneur.2022.1034350/full#supplementary-material
References
1. Wolf PA, Abbott RD, Kannel WB. Atrial fibrillation as an independent risk factor for stroke: the Framingham study. Stroke. (1991) 22:983–8. doi: 10.1161/01.STR.22.8.983
2. Ericson L, Bergfeldt L, Bjorholt I. Atrial fibrillation: the cost of illness in Sweden. Eur J Health Econ. (2011) 12:479–87. doi: 10.1007/s10198-010-0261-3
3. Saposnik G Gladstone D Raptis R Zhou L Hart RG Investigators Investigators of the Registry of the Canadian Stroke N. Atrial fibrillation in ischemic stroke: predicting response to thrombolysis and clinical outcomes. Stroke. (2013) 44:99–104. doi: 10.1161/STROKEAHA.112.676551
4. Bal S, Patel SK, Almekhlafi M, Modi J, Demchuk AM, Coutts SB. High rate of magnetic resonance imaging stroke recurrence in cryptogenic transient ischemic attack and minor stroke patients. Stroke. (2012) 43:3387–8. doi: 10.1161/STROKEAHA.112.671172
5. Gladstone DJ, Spring M, Dorian P, Panzov V, Thorpe KE, Hall J, et al. Atrial fibrillation in patients with cryptogenic stroke. N Engl J Med. (2014) 370:2467–77. doi: 10.1056/NEJMoa1311376
6. Sanna T, Diener HC, Passman RS, Di Lazzaro V, Bernstein RA, Morillo CA, et al. Cryptogenic stroke and underlying atrial fibrillation. N Engl J Med. (2014) 370:2478–86. doi: 10.1056/NEJMoa1313600
7. Diener HC, Sacco RL, Easton JD, Granger CB, Bernstein RA, Uchiyama S, et al. Dabigatran for prevention of stroke after embolic stroke of undetermined source. N Engl J Med. (2019) 380:1906–17. doi: 10.1056/NEJMoa1813959
8. Hart RG, Sharma M, Mundl H, Kasner SE, Bangdiwala SI, Berkowitz SD, et al. Rivaroxaban for stroke prevention after embolic stroke of undetermined source. N Engl J Med. (2018) 378:2191–201. doi: 10.1056/NEJMoa1802686
9. Powers WJ, Rabinstein AA, Ackerson T, Adeoye OM, Bambakidis NC, Becker K, et al. 2018 Guidelines for the Early Management of Patients with Acute Ischemic Stroke: A Guideline for Healthcare Professionals from the American Heart Association/American Stroke Association. Stroke. (2018) 49:e46–e110. doi: 10.1161/STR.0000000000000158
10. Mozaffarian D, Benjamin EJ, Go AS, Arnett DK, Blaha MJ, Cushman M, et al. Heart disease and stroke statistics–2015 update: a report from the American Heart Association. Circulation. (2015) 131:e29–e322. doi: 10.1161/cir.0000000000000152
11. Yoshida Y, Jin Z, Russo C, Homma S, Nakanishi K, Ito K, et al. Subclinical left ventricular systolic dysfunction and incident stroke in the elderly: long-term findings from cardiovascular abnormalities and brain lesions. Eur Heart J Cardiovasc Imaging. (2022). doi: 10.1093/ehjci/jeac145
12. Camm AJ, Corbucci G, Padeletti L. Usefulness of continuous electrocardiographic monitoring for atrial fibrillation. Am J Cardiol. (2012) 110:270–6. doi: 10.1016/j.amjcard.2012.03.021
13. Wang Y, Jing J, Meng X, Pan Y, Wang Y, Zhao X, et al. The Third China National Stroke Registry (Cnsr-Iii) for patients with acute Ischaemic stroke or transient Ischaemic attack: design, rationale and baseline patient characteristics. Stroke Vasc Neurol. (2019) 4:158–64. doi: 10.1136/svn-2019-000242
14. Bjorck S, Palaszewski B, Friberg L, Bergfeldt L. Atrial fibrillation, stroke risk, and warfarin therapy revisited: a population-based study. Stroke. (2013) 44:3103–8. doi: 10.1161/STROKEAHA.113.002329
15. Carrazco C, Golyan D, Kahen M, Black K, Libman RB, Katz JM. Prevalence and risk factors for paroxysmal atrial fibrillation and flutter detection after cryptogenic ischemic stroke. J Stroke Cerebrovasc Dis. (2018) 27:203–9. doi: 10.1016/j.jstrokecerebrovasdis.2017.08.022
16. Yaghi S, Kamel H, Elkind MSV. Atrial cardiopathy: a mechanism of cryptogenic stroke. Expert Rev Cardiovasc Ther. (2017) 15:591–9. doi: 10.1080/14779072.2017.1355238
17. Furie KL, Kasner SE, Adams RJ, Albers GW, Bush RL, Fagan SC, et al. Guidelines for the prevention of stroke in patients with stroke or transient ischemic attack: a guideline for healthcare professionals from the American Heart Association/American Stroke Association. Stroke. (2011) 42:227–76. doi: 10.1161/STR.0b013e3181f7d043
18. Fujii S, Shibazaki K, Kimura K, Sakai K, Aoki J. A simple score for predicting paroxysmal atrial fibrillation in acute ischemic stroke. J Neurol Sci. (2013) 328:83–6. doi: 10.1016/j.jns.2013.02.025
19. Suissa L, Bertora D, Lachaud S, Mahagne MH. Score for the targeting of atrial fibrillation (Staf): a new approach to the detection of atrial fibrillation in the secondary prevention of Ischemic stroke. Stroke. (2009) 40:2866–8. doi: 10.1161/STROKEAHA.109.552679
20. Patton KK, Ellinor PT, Heckbert SR, Christenson RH, DeFilippi C, Gottdiener JS, et al. N-Terminal pro-B-type natriuretic peptide is a major predictor of the development of atrial fibrillation: the cardiovascular health study. Circulation. (2009) 120:1768–74. doi: 10.1161/CIRCULATIONAHA.109.873265
21. Shelton RJ, Clark AL, Goode K, Rigby AS, Cleland JG. The diagnostic utility of N-terminal pro-B-type natriuretic peptide for the detection of major structural heart disease in patients with atrial fibrillation. Eur Heart J. (2006) 27:2353–61. doi: 10.1093/eurheartj/ehl233
22. Garnier L, Duloquin G, Meloux A, Benali K, Sagnard A, Graber M, et al. Multimodal approach for the prediction of atrial fibrillation detected after stroke: Safas study. Front Cardiovasc Med. (2022) 9:949213. doi: 10.3389/fcvm.2022.949213
23. Liu XY Li YX, Fu YG, Cai YY, Zhang YS, Min JY, et al. The value of the score for the targeting of atrial fibrillation (Staf) screening in acute stroke patients. J Stroke Cerebrovasc Dis. (2017) 26:1280–6. doi: 10.1016/j.jstrokecerebrovasdis.2017.01.022
24. Wu L, Emmens RW, van Wezenbeek J, Stooker W, Allaart CP, Vonk ABA, et al. Atrial inflammation in different atrial fibrillation subtypes and its relation with clinical risk factors. Clin Res Cardiol. (2020) 109:1271–81. doi: 10.1007/s00392-020-01619-8
Keywords: acute ischemic stroke, occult atrial fibrillation, nomogram, secondary prevention, cardioembolism
Citation: Wang X, Meng L, Zhao Y and Liu X (2023) Development and external validation of a prognostic model for occult atrial fibrillation in patients with ischemic stroke. Front. Neurol. 13:1034350. doi: 10.3389/fneur.2022.1034350
Received: 01 September 2022; Accepted: 05 December 2022;
Published: 18 January 2023.
Edited by:
Wenbo Zhao, Capital Medical University, ChinaReviewed by:
Deep Pujara, University Hospitals Cleveland Medical Center, United StatesChristoph Stretz, Brown University, United States
Copyright © 2023 Wang, Meng, Zhao and Liu. This is an open-access article distributed under the terms of the Creative Commons Attribution License (CC BY). The use, distribution or reproduction in other forums is permitted, provided the original author(s) and the copyright owner(s) are credited and that the original publication in this journal is cited, in accordance with accepted academic practice. No use, distribution or reproduction is permitted which does not comply with these terms.
*Correspondence: Yanxin Zhao, emhhb195YW54aW4mI3gwMDA0MDsxMjYuY29t; Xueyuan Liu,
bGl1eHkmI3gwMDA0MDt0b25namkuZWR1LmNu
†These authors have contributed equally to this work