- 1Department of Radiology, Beijing Tiantan Hospital, Capital Medical University, Beijing, China
- 2School of Biomedical Science and Medical Engineering, Beijing Advanced Innovation Centre for Biomedical Engineering, Beihang University, Beijing, China
- 3Department of Biomedical Engineering, School of Biomedical Engineering, Capital Medical University, Beijing, China
- 4Beijing Neurosurgical Institute, Beijing Tiantan Hospital, Beijing, China
Objective: To investigate the ability of a MRI-based radiomics-clinicopathological model to predict pituitary macroadenoma (PMA) recurrence within 5 years.
Materials and Methods: We recruited 74 recurrent and 94 non-recurrent subjects, following first surgery with 5-year follow-up data. Univariate and multivariate analyses were conducted to identify independent clinicopathological risk factors. Two independent and blinded neuroradiologists used 3D-Slicer software to manually delineate whole tumors using preoperative axial contrast-enhanced T1WI (CE-T1WI) images. 3D-Slicer was then used to extract radiomics features from segmented tumors. Dimensionality reduction was carried out by the least absolute shrinkage and selection operator (LASSO). Two multilayer perceptron (MLP) models were established, including independent clinicopathological risk factors (Model 1) and a combination of screened radiomics features and independent clinicopathological markers (Model 2). The predictive performance of these models was evaluated by receiver operator characteristic (ROC) curve analysis.
Results: In total, 1,130 features were identified, and 4 of these were selected by LASSO. In the test set, the area under the curve (AUC) of Model 2 was superior to Model 1 {0.783, [95% confidence interval (CI): 0.718—.860] vs. 0.739, (95% CI: 0.665–0.818)}. Model 2 also yielded the higher accuracy (0.808 vs. 0.692), sensitivity (0.826 vs. 0.652), and specificity (0.793 vs. 0.724) than Model 1.
Conclusions: The integrated classifier was superior to a clinical classifier and may facilitate the prediction of individualized prognosis and therapy.
Introduction
Pituitary adenoma is one of the most prevalent intracranial masses that can affect adults (1, 2). The varied clinical manifestations usually result from the endocrine activity, or volume of tumors. The classification of PA is based on different criteria, such as size, immunohistochemistry (IHC), invasion, hormone secretion, and clinical manifestation (1, 3). PAs are classified into micro, macro, and giant adenomas by the MRI size. The IHC subtypes of PAs are composed of growth hormone (GH), prolactin (PRL), adrenocorticotropic hormone (ACTH), thyroid-stimulating hormone (TSH), and follicle-stimulating hormone-luteinising hormone (FSH-LH), including the monohormonal and plurihormonal adenomas. Although benign in terms of their biological behavior, 30–45% of tumors invade the cavernous or sphenoid sinus, which can be categorized into invasive and non-invasive adenomas (1, 3, 4). According to the clinical classification, PAs consist of functioning and non-functioning types (1).
The treatment strategy for most tumors is operation. The postsurgical recurrence rate of pituitary macroadenoma (PMA) within 5 years is considerably high (5). The tendency to relapse has been related to many factors, including different histotypes, tumor remnants, or the extent of invasion into adjacent anatomical structures (6). Previous research has demonstrated that many clinicopathological prognostic tools have potential to predict the recurrence of PMA, consisting of IHC characteristics, invasion of tumors, genetic expression, and markers of proliferation (7–11). However, very few attempts have been made to integrate these risk factors with a machine-learning approach.
Radiomics is a form of analysis that quantitatively extracts imaging features from medical data (12). Thus far, radiomics studies of PA have predominantly focused on two aspects: presurgical evaluation and subtype classification (13–17). Some researchers have used radiomics to explore the potential for relapse in PA. However, these previous models have been associated with small sample sizes and only cases involving non-functioning tumors (18, 19).
In the present study, we aimed to establish a comprehensive classification model that combined independent clinicopathological risk factors with preoperative radiomics signatures for the prediction of PMA recurrence within 5 years of surgery. Our goal was to provide an efficient tool for guiding clinical management and predicting prognosis.
Materials and Methods
Ethics Statement
This retrospective study involved human subjects and was approved by the Ethics Committee of Beijing Tiantan Hospital. The requirement for written informed consent was waived.
Subjects
The recurrence of adenomas was defined as incidence of enlarged remnant tumors in non-functioning PMAs, and/or endocrine biochemical recurrence in functioning PMAs (20, 21).
PMAs referred to adenomas with preoperative size > 10 mm by MRI in our study, based on Asioli et al. (20). PMAs were classified into immunonegative, monohormonal-including GH-positive, PRL-positive, ACTH-positive, FSH-LH-positive, TSH-positive, and plurihormonal by the results of IHC staining (22). The radiological signs of aggressive tumors were determined according to Knosp and Hardy—Wilson classifications on preoperative MRI by a blinded and experienced neuroradiologist. The Knosp and Hardy—Wilson criteria were used to evaluate the degree of invasion of cavernous sinus (CS) and suprasella, respectively. Knosp Scores 3 and 4 were described as adenomas extending beyond the lateral tangents of the cavernous segment of internal carotid artery (ICA) on coronal MRI and completely involving CS and ICA. Hardy—Wilson Grades 3 and 4 were represented as local and extensive invasion of the sellar floor; Stages C and D and E were characterized as total replacement of the third ventricle, intracranial adenomas, and invasion of CS (Supplementary Material 1) (5, 23). The aggressive PMAs were defined as grade of Knosp 3 or 4, and/or Hardy—Wilson Grades 3 or 4 (and/or Hardy—Wilson Stage C or D or E), and/or histological evidence of invasion of cavernous or sphenoid sinus (1). The patients who experienced subtotal resection were recognized as cases with residual tumor, and the subjects who underwent gross- or near-total resection were regarded as cases without remnants (24, 25).
A total of 168 consecutive postoperative subjects with a confirmed pathological diagnosis of PMA were acquired from our institutional medical database between January 2010 and December 2015. Analysis of medical records showed that 74 of these patients reported recurrent attacks (39 men/35 women); and 94 patients had not experienced recurrence (43 men/51 women). All the patients completed the 5-year follow-up period. The inclusion criteria were as follows: (1) available investigation for medical data; (2) underwent surgery; (3) had preoperative MRI; and (4) followed-up for duration of 5 years since first surgery. The exclusion criteria included (1) underwent other treatments for PMA before the first surgery or during the follow-up period; (2) pituitary apoplexy; (3) multiple intracranial lesions; and (4) poor-quality image or lack of contrast-enhancement MRI.
MRI Acquisition and the Segmentation of Tumors
All enrolled subjects underwent MRI of the head prior to surgery, including several different acquisition protocols [axial T1WI and T2WI, axial, coronal, and sagittal contrast-enhanced T1WI (CE-T1WI)]. The contrast agent, dimeglumine gadopentetate, was injected at a dose of.2 ml/kg, following pre-contrast T1 scanning. MRI images were obtained from four different MRI scanners with 3 T (GE Discovery MR 750, n = 59; Siemens MAGNETOM Trio TimSystem, n = 43; Siemens MAGNETOM Verio, n = 22; Philips Ingenia, n = 9), and a 1.5 T scanner (GE Medical System Genesis Signa, n = 35). Supplementary Material 2 shows the type of the contrast medium and the parameters used for axial CE-T1WI for five MRI modalities.
Whole tumors, based on preoperative axial CE-T1WI images, were identified as the region of interest (ROI). The manual delineation of each ROI was conducted by a neuroradiologist with 5 years of experience, using 3D-Slicer software (version 4.10.2 r28257, National Institutes of Health). Prior to segmentation, we applied three steps to standardize different MRI images: N4ITK bias correction, resampling with resampled voxel sizes of 1, 1, and 1, and Laplacian of Gaussian (LOG) with LOG kernel sizes being 1.5, 2, and 2.5 by 3D-Slicer.
Assessments of Intra- and Interobserver Reproducibility
Neuroradiologist 1 segmented the ROIs of 60 randomly selected cases on two occasions separated by an interval of 2 weeks. Neuroradiologist 2 with 5 years of experience independently performed the same analyses on one occasion. Intraclass correlation coefficient (ICC) was then calculated by R (version 4.0.2, http://www.R-project.org) to compare intra- and inter-observer reproducibility. The high reproducibility of these radiomics features was recognized as the ICC score for Radiologist 1 (on two occasions) or between Radiologists 1 and 2 >.75.
The Extraction of Features and Dimension Reduction
In total, 1,130 features were extracted from the segmented ROIs by 3D-Slicer software. These features encompassed eight types: first-order, shape, gray-level dependence matrix (GLDM), gray-level co-occurrence matrix (GLCM), gray-level run length matrix (GLRLM), gray-level size zone matrix (GLSZM), neighboring gray tone difference matrix (NGTDM), and wavelet-based features, which were four distinct categories: intensity histogram, texture, shape, and wavelet. The detailed information for all features is shown in Supplementary Material 3.
The features with ICC score <0.75 were excluded in the first stage, because of the poor reproducibility. Then, we performed the least absolute shrinkage and selection operator (LASSO) in the R environment to carry out dimensionality reduction in the training set. The corresponding regularization coefficient (λ) was obtained by 10-fold cross-validation in LASSO regression based on the 1-standard error of the minimum criteria (1-SE criteria).
The Establishment and Validation of a Radiomics-Clinicopathological Model
The z-score was used to normalize all features onto a similar scale. We randomly separated these subjects into a training set (including 51 recurrence and 65 non-recurrence subjects) and a test set (23 cases with relapse and 29 without relapse; based on a data-partition ratio of 7:3).
Two multilayer perceptron (MLP) classifiers were built by python (version 3.8.2, http://www.python.org) for the prediction of recurrence in PMA, including independent clinicopathological risk factors (Model 1—clinical model) and a combination of screened radiomics features and independent clinicopathological markers (Model 2—integrated model). Receiver operator characteristic (ROC) curves were performed and used to estimate the predictive performance of the two models by area under curve (AUC) analysis. This analysis allowed us to determine the accuracy, specificity, and sensitivity of each model.
MLP was composed of an input layer, a hidden layer, and an output layer. In the process of forward propagation, a series of algorithms were performed to obtain the output of each layer, which was used to be the input of the next layer. The equation was as follows:
where y represents the outcome of output, x represents the input vector, w represents the weight, b represents the bias, and f represents the activation function. Our classifiers included three hidden layers, for which the numbers of neurons were 64, 512, and 64, respectively. The dropout layer was conducted to lose 20% of neurons to reduce overfitting. We applied Rectified Linear Unit (ReLU) and Sigmoid to be activation functions for the hidden and output layers. The binary cross entropy was calculated for use as loss function. The weights were tuned by the back propagation method based on the derivation of the chain rule. In our study, the training epochs were set to 500. Before model establishment, the training cohort was shuffled. The monitoring indicators were accuracy, sensitivity, specificity, and AUC in the training set. Stochastic gradient descent (SGD) was used as the optimizer, with an initial learning rate of.1. The learning rate decay strategy was set to the reduction of 70% if the accuracy of training cohort did not improve for consecutive 100 epochs. Optimized class weights were obtained according to the numbers of recurrent and non-recurrent patients in the training set, and the batch size was default value of 32. The predictive performance of each model was validated in the test cohort and evaluated by 5-fold cross-validation. Figure 1 shows the process used for the analysis of radiomics.
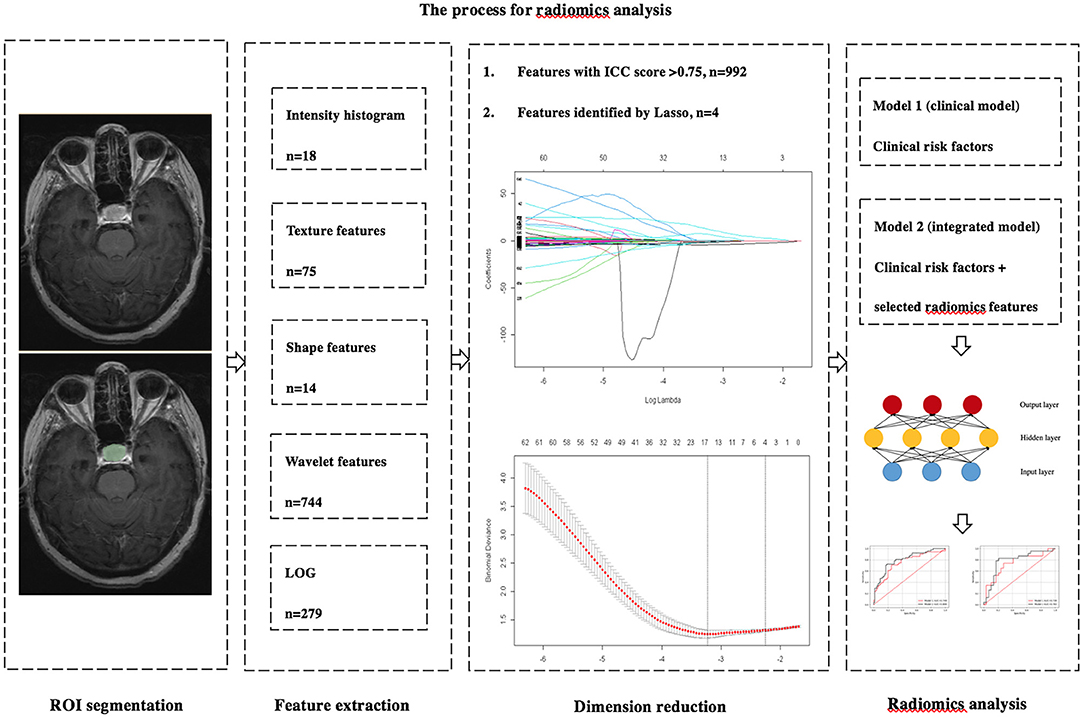
Figure 1. The process used for the analysis of radiomics. Radiomics features were extracted from the preoperative axial CE-T1WI images by 3D slicer. Dimension reductions were performed two times by ICC and LASSO. The MLP was used to build two predictive models. Model 1 included independent clinicopathological risk factors. Model 2 included the combination of radiomics features and independent risk factors.
Statistical Analysis
The normality test of the data was performed by Shapiro—Wilk. Two-sided independent sample t-test and Mann—Whitney U-test were conducted to compare the differences in continuous variates, and Pearson's χ2 test and Fisher's precision probability test were used to investigate the differences in categorical variates in the training and test sets. Univariate and multivariable logistic regression were used to identify independent clinicopathological risk factors for the recurrence of PMA in the training set. The differences of extracted radiomics features between groups of recurrence and non-recurrence in the training set were determined by Mann—Whitney U-test; these analyses were carried out with SPSS (version 23.0, IBM), and a p of < 0.05 was considered to be statistically significant.
Results
Clinical Characteristics of the Study Cohort
The baseline investigation of the study patients is shown in Table 1. The differences with regard to clinical characteristics between training and test cohorts were not statistically significant.
Univariate analysis demonstrated that age {p = 0.034; OR, 0.967 [95% confidence interval (CI), 0.937–0.997]}, height [p = 0.007; OR, 1.068 (95% CI, 1.018–1.120)], residual tumor [p = 0.009; OR, 2.963 (95% CI, 1.319–6.659)], and invasion [p = 0.009; OR, 3.359 (95% CI, 1.359–8.305)] were significant risk factors for relapse. Multivariate analysis identified two independent risk factors for the recurrence of PMA: age [p = 0.035; OR, 0.963 (95% CI, 0.930–0.997)] and residual tumor [p = 0.047; OR, 2.393 (95% CI, 1.011–5.667)] (Table 2). Our study included four clinicopathological features: age, height, residual tumor, and invasion in Models 1 and 2 based on univariate and multivariate analyses.
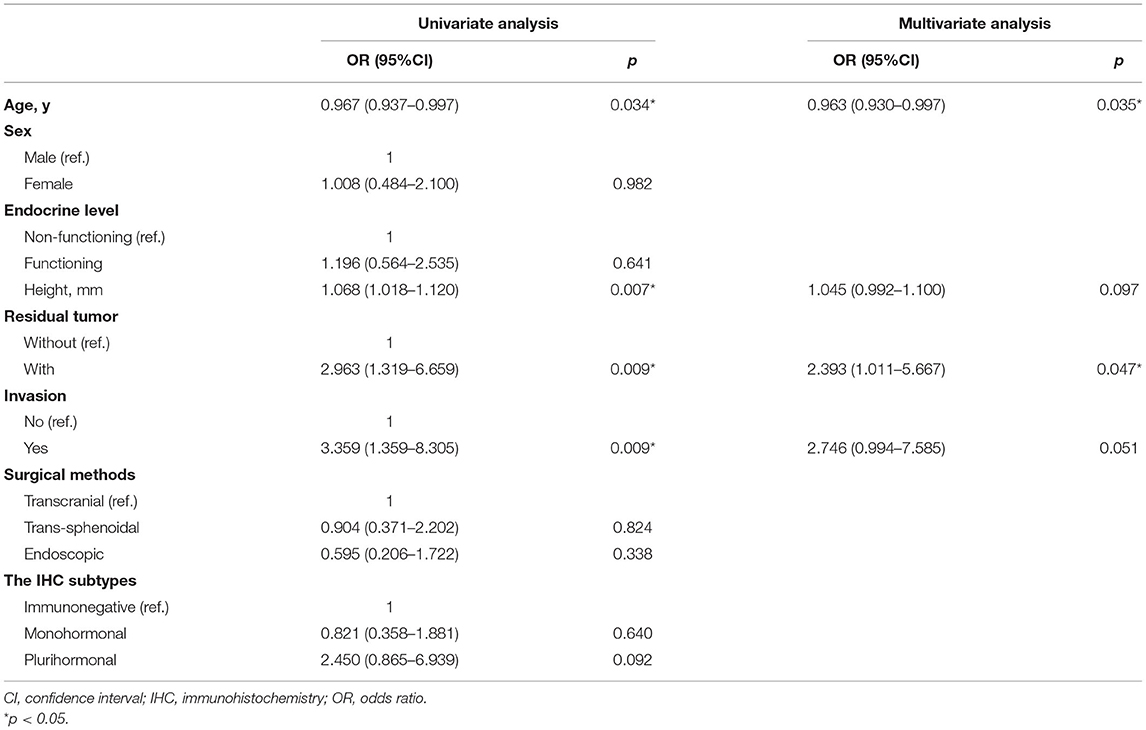
Table 2. Univariate and multivariate analysis of clinical characteristics to identify risk factors in the recurrence of PMA in the training set.
Intra- and Interobserver Analyses
The mean ICC scores for intra- (Neuroradiologist 1 on two occasions) and interobserver (Neuroradiologists 1 and 2) agreements were 0.913 ± 0.129 and 0.903 ± 0.127, respectively, for all selected patients, which showed the high agreement of these features.
Comparing the Predictive Performance of the Two Models
Of the 1,130 features, 138 with unsatisfactory agreement were excluded by the first round, and four of these radiomics features were then identified by LASSO regression, consisting of one shape feature, one LOG, and two wavelet features. All of four selected signatures showed statistically significant differences (p < 0.05) in the training set (Figure 2).
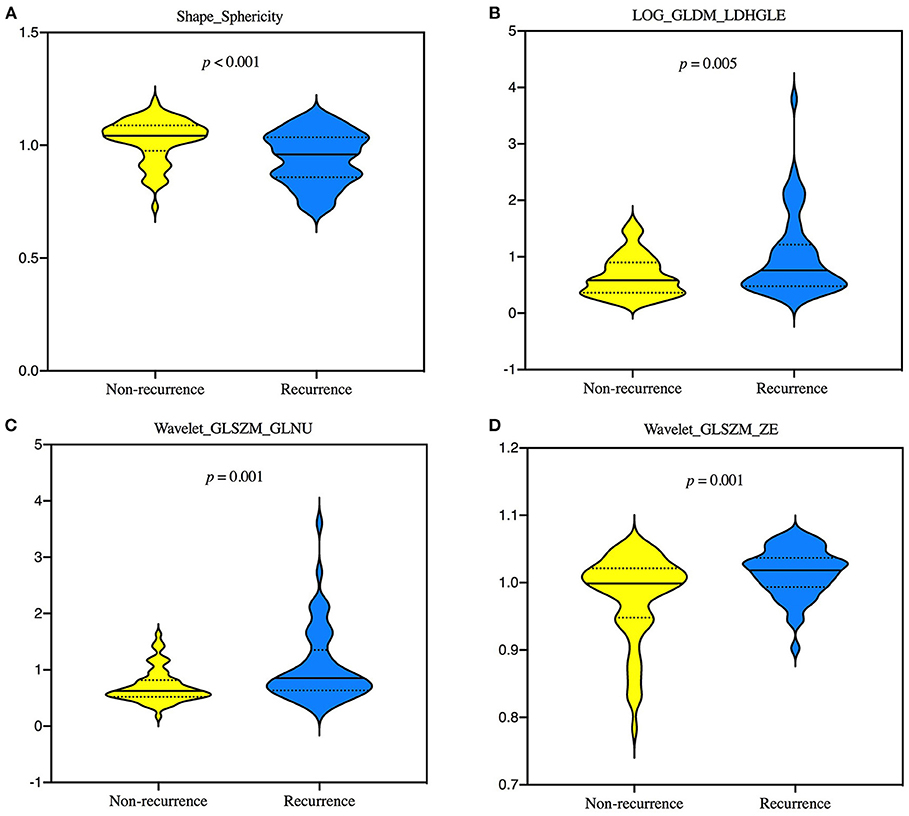
Figure 2. Violin plots showing the differences of 4 selected radiomics features, Shape_Sphericity (A), LOG_GLDM_LDHGLE (B), Wavelet_GLSZM_GLNU (C), and Wavelet_GLSZM_ZE (D) between groups of recurrence and non-recurrence in the training set by Mann—Whitney U-test. GLDM, gray-level dependence matrix; GLNU, gray-level non-uniformity; GLSZM, gray-level size zone matrix; LDHGLE, large dependence high-gray-level emphasis; LOG, laplacian of gaussian; ZE, zone entropy.
The ROC curves in the training and test sets are shown in Figure 3; the AUCs, accuracy, sensitivity, and specificity of the two models are presented in Table 3. In the test set, the AUC of Model 2 was superior to Model 1 [0.783, (95% CI: 0.718–0860) vs. 0.739, (95% CI: 0.665–0.818)]. Model 2 also yielded the higher accuracy (0.808 vs. 0.692), sensitivity (0.826 vs. 0.652), and specificity (0.793 vs. 0.724) than Model 1.
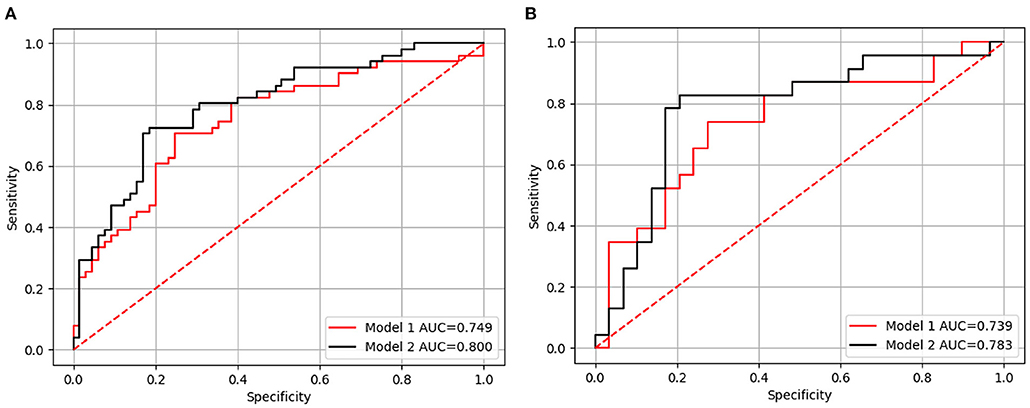
Figure 3. The receiver-operating characteristic (ROC) curve for Models 1 and 2 in the training (A) and test sets (B), respectively. Model 1 included independent clinicopathological risk factors and Model 2 included both radiomics features and independent clinicopathological risk factors.
Discussion
In this study, we constructed a diagnostic classification strategy based on the presurgical MRI to predict the recurrence risk of PMA within 5 years. This comprehensive classifier incorporates clinicopathological and radiomics features and can accurately predict the recurrence of PMA.
Various factors are known to be associated with a higher risk of PMA recurrence, which remains a significant problem for both clinicians and patients. Very few previous reports have attempted to combine clinicopathology analysis with radiomics for the prediction of PMA. MRI radiomics approaches have been described in previous literature. For example, Zhang et al. (18) and Machado et al. (19) used this method to explore the recurrence of non-functioning PA. Compared with these previous results, the Model 2 in our study presented with relative low diagnostic accuracy. This is probably because we built the test set using an independent set of subjects rather than the training group. Moreover, our study simultaneously included functioning and non-functioning PMAs. The study cohorts and enrollment criteria may lead to the different predictive performances. However, our Model 2, which had a relatively large sample size and incorporated comprehensive markers, showed a better level of classification performance than Model 1. This improved predictive efficiency demonstrates that the combination of clinicopathological data and imaging may provide more practical information and guidance for developing a treatment and prognosis strategy than clinical analysis alone.
MLP model is a feed-forward artificial neural network (ANN) model that is applicable to a non-linear inseparable issue; Almubark et al. demonstrated the predictive value of this approach in their previous study (26). The generalization and efficacy of this method have been widely confirmed in several papers (27–29). Given these characteristics, we also established an MLP classifier for the recurrence of PMA and achieved satisfactory levels of predictive performance in a test cohort. These data indicate that this deep learning algorithm is a reproducible and robust technique for classification.
Many risk factors are associated with the recurrence of PMA. In the present study, we incorporated some of the primary predictors that have been described in previous literature (30). Four clinicopathological risk factors—age, height, invasion, and residual tumor—were finally included in our comprehensive model; these factors were identified by a combination of univariate and multivariate analyses. We found that the patients in the recurrence group tended to be younger. We believe that this is because there is a greater risk of gene disorders in younger age groups (31). In a previous study, Trott concluded that young patients express elevated levels of ki-67 in non-functioning pituitary adenoma, and that this is strongly associated with relapse (32). Moreover, some ultrastructural types of PMA resulted in regrowth, such as sparsely granulated somatotroph adenoma, and are more likely to affect younger patients. The aggressive growth pattern of PMA is one of the main reasons concerned with the prognosis (33). The invasive tumors usually exhibit more rapid growth, a higher proliferative index, and larger size. Thus, the severe erosion of surrounding structures (e.g., cavernous sinus and sellar floor) and great extension of the supra- and para-sellar lead to increased rates of recurrence (34). Similar results in the present literature provide support to our conclusion that tumor remnant is also known to be significantly correlated with PMA behavior, especially the higher incidence of larger extra-sellar residuals (5, 6, 34). Height is another recognized predictive candidate. It is evident that the tumors with higher height may result in incomplete resection and invasive behavior, which consequently raise the likelihood of regrowth. These findings are consistent with previous reports (5, 6, 35). This suggests that the clinical characteristics described above are useful and reliable tools for predicting the prognosis of patients with PMA.
The tumor classification involving the transcription factor or ultrastructure showed that the presence of giant lactotroph, sparsely granulated somatotroph, crooke's cell, or silent corticotroph adenomas tends to present the recurrence nature (5, 36, 37). Our study focused on the proposed IHC subtypes that are also important and potential indicators related to the progression. The study reported by Asioli et al. showed that PRL, ACHT, and FSH-LH subunits had relapse risk with high probability (20). These indices were not statistically significant when compared between the two groups in our study; it is possible that this was owing to the small sample size compared with the previous study. Although the rate of TSH adenoma was the lowest among all cases, the trend of incidence is in line with tangible clinical practice. The morbidity of this type is low based on the demographic investigation, comprising <3% of all tumors (38). We included the plurihormonal adenoma in our study. The most common type is the co-secretion of GH and PRL (39). But the combination of different hormones tends to be more complicated. Little is known with regard to the correlation between recurrence and plurihormonal tumors.
The trans-sphenoidal and endoscopic surgical methods are extensively applied to dealing with PMA (40–42). Our study did not show the correlation between operative approaches and recurrence. According to current studies, tumor size and invasive extension were decisive factors in extent of resection. The macro or giant adenomas and extensive invasion tended to be difficult to achieve grossly complete resection, although the trans-sphenoidal or endoscopic resection was used (43–45). The residual tumor is likely to be the crucial factor that affects the prognosis, suggesting that the surgical resection may be more likely to associate with intrinsic biological characteristics of tumors, compared with an operative procedure. Patients who received total resection still have the possibility of relapse (5). This indicates that surgery alone may not enable to decrease the recurrence rate. It is also important and beneficial to combine radiotherapy or other methods (46).
The consistency of adenomas probably influences the prognostic outcomes. A study showed that texture was correlated with tumor profiles, complications, and surgical resection. The hard adenomas were at higher risk of large and aggressive behavior and subtotal removal (47). This suggests that consistency is potential to predict the recurrence of PMAs. Rui et al. confirmed the utility of the radiomics method for determining the texture of PMAs (13). Future PA studies may pay more attention to the relationship between the stiffness and recurrence by radiomics.
The proliferative biomarkers of ki-67, p53, and mitosis play an important role in tumor prognosis. Although there remains the controversy of arguments, the changes of these proliferation indices are often associated with aggressive PAs. The prognoses of the patients tended to be recurrent or poorer in the presence of ki-67 ≥3, mitoses >2, and p53 overexpression based on the study by Raverot and European Society of Endocrinology (48). Above all, the most effective and useful predictive strategies are incorporating the predictors of different fields, such as clinical, imaging, and immunohistochemical examination.
Limitations
First, the proliferations, transcription factors, ultrastructural subtypes, along with expression profiles of certain genes, were not considered in this study but may improve the performance of our classifier. Second, the pituitary scanning sequence with smaller slice thickness and interval was not applied in the study, because the protocol is not clinical routine examination; it may be considered in future studies. Third, microadenoma is another common subgroup of pituitary tumor; radiomic studies of this form of tumor are very rare. We did not include this type of tumor in the present study due to a limited sample size. Finally, this study was based in a single center and lacks external validation in multiple centers.
Conclusion
The combination of clinicopathological characteristics and imaging is useful for predicting the recurrence of PMA within 5 years. The integrated classifier was superior to a clinical classifier and may facilitate the prediction of individualized prognosis and therapy.
Data Availability Statement
The original contributions presented in the study are included in the article/Supplementary Material, further inquiries can be directed to the corresponding author/s.
Ethics Statement
The studies involving human participants were reviewed and approved by the Ethics Committee of Beijing Tiantan Hospital. The Ethics Committee obtained written informed consent from the participants.
Author Contributions
JM designed the original research. YZ conducted the research and wrote the manuscript. YZ, YLu, and XK analyzed the data. JM, TW, and YLo revised the paper. All authors contributed to the article and approved the submitted version.
Funding
This research project was funded by National Natural Science Foundation of China (No. 61771325).
Conflict of Interest
The authors declare that the research was conducted in the absence of any commercial or financial relationships that could be construed as a potential conflict of interest.
Publisher's Note
All claims expressed in this article are solely those of the authors and do not necessarily represent those of their affiliated organizations, or those of the publisher, the editors and the reviewers. Any product that may be evaluated in this article, or claim that may be made by its manufacturer, is not guaranteed or endorsed by the publisher.
Supplementary Material
The Supplementary Material for this article can be found online at: https://www.frontiersin.org/articles/10.3389/fneur.2021.780628/full#supplementary-material
References
1. Raverot G, Jouanneau E, Trouillas J. Management of endocrine disease: clinicopathological classification and molecular markers of pituitary tumours for personalized therapeutic strategies. Eur J Endocrinol. (2014) 170:R121–32. doi: 10.1530/EJE-13-1031
2. Bi WL, Greenwald NF, Ramkissoon SH, Abedalthagafi M, Coy SM, Ligon KL, et al. Clinical identification of oncogenic drivers and copy-number alterations in pituitary tumors. Endocrinology. (2017) 158:2284–91. doi: 10.1210/en.2016-1967
3. Trouillas J. In search of a prognostic classification of endocrine pituitary tumors. Endocr Pathol. (2014)25:124–32. doi: 10.1007/s12022-014-9322-y
4. Raverot G, Vasiljevic A, Jouanneau E, Trouillas J. A prognostic clinicopathologic classification of pituitary endocrine tumors. Endocrinol Metab Clin North Am. (2015) 44:11–8. doi: 10.1016/j.ecl.2014.10.001
5. Hayhurst C, Taylor PN, Lansdown AJ, Palaniappan N, Rees DA, Davies JS. Current perspectives on recurrent pituitary adenoma: the role and timing of surgery vs. adjuvant treatment. Clin Endocrinol. (2020) 92:89–97. doi: 10.1111/cen.14127
6. Raverot G, Vasiljevic A, Jouanneau E. Prognostic factors of regrowth in nonfunctioning pituitary tumors. Pituitary. (2018) 21:176–82. doi: 10.1007/s11102-017-0861-3
7. Das C, Mondal P, Mukhopadhyay M, Mukhopadhyay S, Ghosh I, Handral A. Evaluation of prognostic utility of Ki-67, P53, and O-6-methylguanine-DNA methyltransferase expression in pituitary tumors. J Lab Phys. (2019) 11:323–9. doi: 10.4103/JLP.JLP_76_19
8. Pappy AL II, Savinkina A, Bicknese C, Neill S, Oyesiku NM, Ioachimescu AG. Predictive modeling for pituitary adenomas: single center experience in 501 consecutive patients. Pituitary. (2019) 22:520–31. doi: 10.1007/s11102-019-00982-8
9. Ko CC, Chen TY, Lim SW, Kuo YT, Wu TC, Chen JH. Prediction of recurrence in solid nonfunctioning pituitary macroadenomas: additional benefits of diffusion-weighted MR imaging. J Neurosurg. (2019) 132:351–9. doi: 10.3171/2018.10.JNS181783
10. Kim JS, Lee YS, Jung MJ, Hong YK. The predictive value of pathologic features in pituitary adenoma and correlation with pituitary adenoma recurrence. J Pathol Transl Med. (2016) 50:419–25. doi: 10.4132/jptm.2016.06.30
11. Turner HE, Nagy Z, Gatter KC, Esiri MM, Wass JA, Harris AL. Proliferation, bcl-2 expression and angiogenesis in pituitary adenomas: relationship to tumour behaviour. Br J Cancer. (2000) 82:1441–5. doi: 10.1054/bjoc.1999.1074
12. Lambin P, Rios-Velazquez E, Leijenaar R, Carvalho S, van Stiphout RG, Granton P, et al. Radiomics: extracting more information from medical images using advanced feature analysis. Eur J Cancer. (2012) 48:441–6. doi: 10.1016/j.ejca.2011.11.036
13. Rui W, Wu Y, Ma Z, Wang Y, Wang Y, Xu X, et al. MR textural analysis on contrast enhanced 3D-SPACE images in assessment of consistency of pituitary macroadenoma. Eur J Radiol. (2019) 110:219–24. doi: 10.1016/j.ejrad.2018.12.002
14. Fan Y, Liu Z, Hou B, Li L, Liu X, Liu Z, et al. Development and validation of an MRI-based radiomic signature for the preoperative prediction of treatment response in patients with invasive functional pituitary adenoma. Eur J Radiol. (2019) 121:108647. doi: 10.1016/j.ejrad.2019.108647
15. Peng A, Dai H, Duan H, Chen Y, Huang J, Zhou L, et al. A machine learning model to precisely immunohistochemically classify pituitary adenoma subtypes with radiomics based on preoperative magnetic resonance imaging. Eur J Radiol. (2020) 125:108892. doi: 10.1016/j.ejrad.2020.108892
16. Niu J, Zhang S, Ma S, Diao J, Zhou W, Tian J, et al. Preoperative prediction of cavernous sinus invasion by pituitary adenomas using a radiomics method based on magnetic resonance images. Eur Radiol. (2019) 29:1625–34. doi: 10.1007/s00330-018-5725-3
17. Ugga L, Cuocolo R, Solari D, Guadagno E, D'Amico A, Somma T, et al. Prediction of high proliferative index in pituitary macroadenomas using MRI-based radiomics and machine learning. Neuroradiology. (2019) 61:1365–73. doi: 10.1007/s00234-019-02266-1
18. Zhang Y, Ko CC, Chen JH, Chang KT, Chen TY, Lim SW, et al. Radiomics approach for prediction of recurrence in non-functioning pituitary macroadenomas. Front Oncol. (2020) 10:590083. doi: 10.3389/fonc.2020.590083
19. Machado LF, Elias PCL, Moreira AC, Dos Santos AC, Murta Junior LO. MRI radiomics for the prediction of recurrence in patients with clinically non-functioning pituitary macroadenomas. Comput Biol Med. (2020) 124:103966. doi: 10.1016/j.compbiomed.2020.103966
20. Asioli S, Righi A, Iommi M, Baldovini C, Ambrosi F, Guaraldi F, et al. Validation of a clinicopathological score for the prediction of post-surgical evolution of pituitary adenoma: retrospective analysis on 566 patients from a tertiary care centre. Eur J Endocrinol. (2019) 180:127–34. doi: 10.1530/EJE-18-0749
21. Almeida JP, Tabasinejad R, Kalyvas A, Takami H, Mohan N, O'Halloran PJ, et al. The importance of long term follow up after endoscopic pituitary surgery: durability of results and tumor recurrence. Neurol India. (2020) 68(Suppl.):S92–100. doi: 10.1055/s-0040-1702474
22. Trouillas J, Jaffrain-Rea ML, Vasiljevic A, Raverot G, Roncaroli F, Villa C. How to classify the pituitary neuroendocrine tumors (PitNET)s in 2020. Cancers. (2020) 12:514. doi: 10.3390/cancers12020514
23. Shin SS, Tormenti MJ, Paluzzi A, Rothfus WE, Chang YF, Zainah H, et al. Endoscopic endonasal approach for growth hormone secreting pituitary adenomas: outcomes in 53 patients using 2010 consensus criteria for remission. Pituitary. (2013) 16:435–44. doi: 10.1007/s11102-012-0440-6
24. Juthani RG, Reiner AS, Patel AR, Cowan A, Roguski M, Panageas KS, et al. Radiographic and clinical outcomes using intraoperative magnetic resonance imaging for transsphenoidal resection of pituitary adenomas. J Neurosurg. (2020) 134:1824–35. doi: 10.3171/2020.4.JNS20178
25. Lee CC, Yang HC, Chen CJ, Lin CJ, Wu HM, Chung WY, et al. Empirical versus progression-guided stereotactic radiosurgery for non-functional pituitary macroadenomas after subtotal resection. J Neurooncol. (2019) 142:291–7. doi: 10.1007/s11060-019-03095-1
26. Almubark I, Chang LC, Shattuck KF, Nguyen T, Turner RS, Jiang X, et al. 5-min cognitive task with deep learning accurately detects early Alzheimer's disease. Front Aging Neurosci. (2020) 12:603179. doi: 10.3389/fnagi.2020.603179
27. Shehata M, Alksas A, Abouelkheir RT, Elmahdy A, Shaffie A, Soliman A, et al. A comprehensive computer-assisted diagnosis system for early assessment of renal cancer tumors. Sensors. (2021) 21:4928. doi: 10.3390/s21144928
28. Heise D, Schulze-Hagen M, Bednarsch J, Eickhoff R, Kroh A, Bruners P, et al. CT-based prediction of liver function and post-PVE hypertrophy using an artificial neural network. J Clin Med. (2021) 10:3079. doi: 10.3390/jcm10143079
29. Detmer FJ, Lückehe D, Mut F, Slawski M, Hirsch S, Bijlenga P, et al. Comparison of statistical learning approaches for cerebral aneurysm rupture assessment. Int J Comput Assist Radiol Surg. (2020) 15:141–50. doi: 10.1007/s11548-019-02065-2
30. Lv L, Yin S, Zhou P, Hu Y, Chen C, Ma W, et al. Clinical and pathologic characteristics predicted the postoperative recurrence and progression of pituitary adenoma: a retrospective study with 10 years follow-up. World Neurosurg. (2018) 118:e428–35. doi: 10.1016/j.wneu.2018.06.210
31. Tatsi C, Stratakis CA. Aggressive pituitary tumors in the young and elderly. Rev Endocr Metab Disord. (2020) 21:213–23. doi: 10.1007/s11154-019-09534-8
32. Trott G, Ongaratti BR, de Oliveira Silva CB, Abech GD, Haag T, Rech CGSL, et al. PTTG overexpression in non-functioning pituitary adenomas: Correlation with invasiveness, female gender and younger age. Ann Diagn Pathol. (2019) 41:83–9. doi: 10.1016/j.anndiagpath.2019.04.016
33. Miller BA, Rutledge WC, Ioachimescu AG, Oyesiku NM. Management of large aggressive nonfunctional pituitary tumors: experimental medical options when surgery and radiation fail. Neurosurg Clin N Am. (2012) 23:587–94. doi: 10.1016/j.nec.2012.06.013
34. Li C, Zhu H, Zong X, Wang X, Gui S, Zhao P, et al. Experience of trans-nasal endoscopic surgery for pituitary tumors in a single center in China: Surgical results in a cohort of 2032 patients, operated between 2006 and 2018. Clin Neurol Neurosurg. (2020) 197:106176. doi: 10.1016/j.clineuro.2020.106176
35. Monsalves E, Larjani S, Loyola Godoy B, Juraschka K, Carvalho F, Kucharczyk W, et al. Growth patterns of pituitary adenomas and histopathological correlates. J Clin Endocrinol Metab. (2014) 99:1330–8. doi: 10.1210/jc.2013-3054
36. Farrell CJ, Garzon-Muvdi T, Fastenberg JH, Nyquist GG, Rabinowitz MR, Rosen MR, et al. Management of nonfunctioning recurrent pituitary adenomas. Neurosurg Clin N Am. (2019) 30:473–82. doi: 10.1016/j.nec.2019.05.006
37. Walsh MT, Couldwell WT. Symptomatic cystic degeneration of a clinically silent corticotroph tumor of the pituitary gland. Skull Base. (2010) 20:367–70. doi: 10.1055/s-0030-1253579
38. Luo P, Zhang L, Yang L, An Z, Tan H. Progress in the pathogenesis, diagnosis, and treatment of tsh-secreting pituitary neuroendocrine tumor. Front Endocrinol. (2020) 11:580264. doi: 10.3389/fendo.2020.580264
39. Rasul FT, Jaunmuktane Z, Khan AA, Phadke R, Powell M. Plurihormonal pituitary adenoma with concomitant adrenocorticotropic hormone (ACTH) and growth hormone (GH) secretion: a report of two cases and review of the literature. Acta Neurochir. (2014) 156:141–6. doi: 10.1007/s00701-013-1890-y
40. Miller BA, Ioachimescu AG, Oyesiku NM. Contemporary indications for transsphenoidal pituitary surgery. World Neurosurg. (2014) 82(6 Suppl.):S147–51. doi: 10.1016/j.wneu.2014.07.037
41. Vasudevan K, Saad H, Oyesiku NM. The role of three-dimensional endoscopy in pituitary adenoma surgery. Neurosurg Clin N Am. (2019) 30:421–32. doi: 10.1016/j.nec.2019.05.012
42. Khalafallah AM, Liang AL, Jimenez AE, Rowan NR, Oyesiku NM, Mamelak AN, et al. Trends in endoscopic and microscopic transsphenoidal surgery: a survey of the international society of pituitary surgeons between 2010 and 2020. Pituitary. (2020) 23:526–33. doi: 10.1007/s11102-020-01054-y
43. Hoang N, Tran DK, Herde R, Couldwell GC, Osborn AG, Couldwell WT. Pituitary macroadenomas with oculomotor cistern extension and tracking: implications for surgical management. J Neurosurg. (2016) 125:315–22. doi: 10.3171/2015.5.JNS15107
44. Dallapiazza RF, Grober Y, Starke RM, Laws ER Jr, Jane JA Jr. Long-term results of endonasal endoscopic transsphenoidal resection of nonfunctioning pituitary macroadenomas. Neurosurgery. (2015) 76:42–52. doi: 10.1227/NEU.0000000000000563
45. Schult D, Hölsken A, Siegel S, Buchfelder M, Fahlbusch R, Kreitschmann-Andermahr I, et al. EZH2 is highly expressed in pituitary adenomas and associated with proliferation. Sci Rep. (2015) 5:16965. doi: 10.1038/srep16965
46. Taussky P, Kalra R, Coppens J, Mohebali J, Jensen R, Couldwell WT. Endocrinological outcome after pituitary transposition (hypophysopexy) and adjuvant radiotherapy for tumors involving the cavernous sinus. J Neurosurg. (2011) 115:55–62. doi: 10.3171/2011.2.JNS10566
47. Rutkowski MJ, Chang KE, Cardinal T, Du R, Tafreshi AR, Donoho DA, et al. Development and clinical validation of a grading system for pituitary adenoma consistency. J Neurosurg. (2020) 134:1800–7. doi: 10.3171/2020.4.JNS193288
Keywords: pituitary macroadenoma, recurrence, predictive model, deep learning, multilayer perceptron
Citation: Zhang Y, Luo Y, Kong X, Wan T, Long Y and Ma J (2022) A Preoperative MRI-Based Radiomics-Clinicopathological Classifier to Predict the Recurrence of Pituitary Macroadenoma Within 5 Years. Front. Neurol. 12:780628. doi: 10.3389/fneur.2021.780628
Received: 14 October 2021; Accepted: 02 December 2021;
Published: 05 January 2022.
Edited by:
Zhixiong Liu, Central South University, ChinaReviewed by:
S. Ottavio Tomasi, Paracelsus Medical University, AustriaAntonino F. Germano, University of Messina, Italy
Teresa Somma, Federico II University Hospital, Italy
Copyright © 2022 Zhang, Luo, Kong, Wan, Long and Ma. This is an open-access article distributed under the terms of the Creative Commons Attribution License (CC BY). The use, distribution or reproduction in other forums is permitted, provided the original author(s) and the copyright owner(s) are credited and that the original publication in this journal is cited, in accordance with accepted academic practice. No use, distribution or reproduction is permitted which does not comply with these terms.
*Correspondence: Jun Ma, dr.junma@foxmail.com