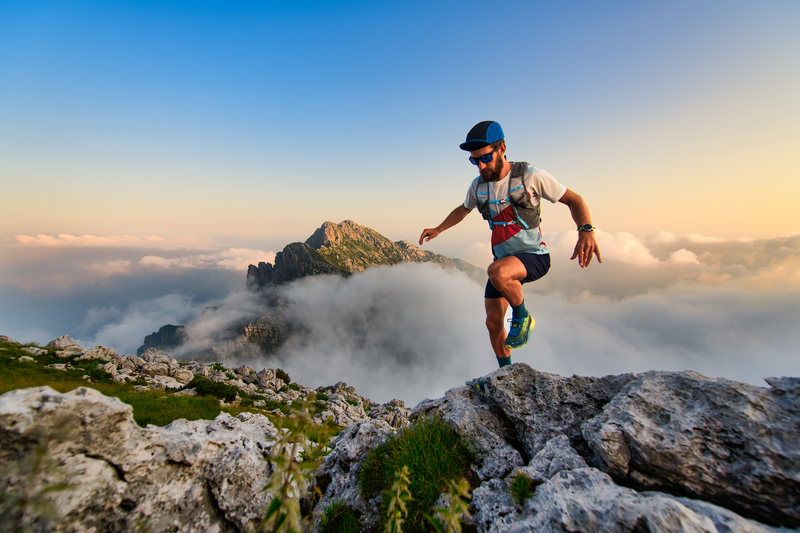
94% of researchers rate our articles as excellent or good
Learn more about the work of our research integrity team to safeguard the quality of each article we publish.
Find out more
ORIGINAL RESEARCH article
Front. Neurol. , 15 December 2021
Sec. Epilepsy
Volume 12 - 2021 | https://doi.org/10.3389/fneur.2021.767165
Objectives: To investigate the association between radiomics features and epilepsy in patients with unruptured brain arteriovenous malformations (bAVMs) and to develop a prediction model based on radiomics features and clinical characteristics for bAVM-related epilepsy.
Methods: This retrospective study enrolled 176 patients with unruptured bAVMs. After manual lesion segmentation, a total of 858 radiomics features were extracted from time-of-flight magnetic resonance angiography (TOF-MRA). A radiomics model was constructed, and a radiomics score was calculated. Meanwhile, the demographic and angioarchitectural characteristics of patients were assessed to build a clinical model. Incorporating the radiomics score and independent clinical risk factors, a combined model was constructed. The performance of the models was assessed with respect to discrimination, calibration, and clinical usefulness.
Results: The clinical model incorporating 3 clinical features had an area under the curve (AUC) of 0.71. Fifteen radiomics features were used to build the radiomics model, which had a higher AUC of 0.78. Incorporating the radiomics score and clinical risk factors, the combined model showed a favorable discrimination ability and calibration, with an AUC of 0.82. Decision curve analysis (DCA) demonstrated that the combined model outperformed the clinical model and radiomics model in terms of clinical usefulness.
Conclusions: The radiomics features extracted from TOF-MRA were associated with epilepsy in patients with unruptured bAVMs. The radiomics-clinical nomogram, which was constructed based on the model incorporating the radiomics score and clinical features, showed favorable predictive efficacy for bAVM-related epilepsy.
Brain arteriovenous malformations (bAVMs) are tangles of malformed vessels without capillary networks and commonly present with intracranial hemorrhage, epilepsy, or neurological deficits (1, 2). Although hemorrhage prevention is the primary aim of bAVM treatment, seizure control should also be at the forefront of therapeutic management (3). The accurate prediction of epilepsy may open novel therapeutic possibilities for patients with bAVMs. Patients can present initially with epilepsy or experience epilepsy after intracranial hemorrhage (4). Although hemorrhage history has long been considered a strong predictor for bAVM-related epilepsy (5), this factor is not applicable for unruptured bAVMs. Additionally, epilepsy caused by the rupture of bAVMs may affect the identification of factors that correlate with epilepsy in patients without hemorrhage (6). A reliable tool to predict epilepsy specific to patients with unruptured bAVMs is needed for clinical decision-making.
Radiomics is a research branch in the field of medical imaging. Much lesion information that is not recognized by the human eye remains unmined. Radiomics can extract high-dimensional radiomic features from medical images to fully exploit the in-depth information of lesions (7, 8). Based on the rapid development of machine learning and image processing techniques, radiomic analyses have been successfully applied in epilepsy prediction (9, 10). Considering the potential of radiomics analysis to provide a more accurate prediction for bAVM-related epilepsy, the development of a prediction model combining clinical and quantitative imaging features is a necessary step in enhancing care and treatment for patients with unruptured bAVMs.
Time-of-flight magnetic resonance angiography (TOF-MRA) has been regarded as a first-line non-invasive diagnostic tool in the evaluation of cerebrovascular diseases. It is frequently one of the first examinations performed when there is an initial diagnosis of bAVMs (11, 12). TOF-MRA is easy to perform and provides satisfactory images with the simultaneous presentation of vessels and brain tissues. In our study, a total of 176 patients with bAVMs who underwent TOF-MRA imaging were enrolled. We sought to analyze and explore the performance of clinical factors and radiomics features extracted from TOF-MRA in predicting bAVM-related epilepsy. Then, we built and validated a radiomics-clinical nomogram as a useful clinical tool for the individualized preoperative prediction of epilepsy in patients with unruptured bAVMs.
A total of 176 patients with unruptured bAVMs were retrospectively reviewed from our bAVM database of two prospective clinical trials (ClinicalTrials.gov Identifier: NCT01758211 and NCT02868008) between July 2012 and August 2020. Patients with bAVMs were clinically diagnosed by neuroradiological data, and unruptured bAVMs were defined based on the combination of medical history and radiological findings as reported previously (13). All recruited patients had no clinical history of surgical, endovascular, or stereotactic radiosurgical treatment for bAVMs. Meanwhile, detailed clinical records were available for the evaluation of epilepsy. This study was approved by the ethics committee of Beijing Tiantan Hospital, Capital Medical University, and written informed consent was obtained from all patients. The flow chart of the study is illustrated in Figure 1.
The diagnosis of epilepsy was clarified according to the classification and terminology of the International League Against Epilepsy based on the patient's observed behavior (14). Moreover, a patient was determined to have suffered from bAVM-related epilepsy when a history of at least one seizure with the presence of an enduring alteration (i.e., bAVM) in the brain was reported (15).
The MR images were obtained using a 3.0-T MR scanner (SIEMENS Trio). Axial TOF-MRA images were obtained for all the patients. The sagittal T1 anatomical image acquired was a gradient-echo sequence: TR, 2,300 msec; TE, 2.98 msec; slice thickness, 1 mm; slices, 176; FOV, 256 mm; flip angle, 9°; matrix, 64 × 64; voxel size, 1 × 1 × 1 mm3; and bandwidth, 240. Axial TOF-MRA was performed using a three-dimensional (3D) TOF gradient-echo acquisition sequence: TR, 22 msec; TE, 3.86 msec; slice thickness, 1 mm; slices, 36 × 4; FOV, 220 × 220 mm2; flip angle, 120°; and matrix, 512 × 512 (16).
Masks of lesions were drawn on each patient's TOF-MRA image using 3D Slicer software (www.slicer.org) by two neurosurgeons (Y.M.J. and H.L.) who were blinded to the patients' clinical information. We delineated the lesion area according to the texture of light and dark reflected by vessels and tissues. The lesion masks were combined when there was less than a 5% discrepancy between the individual masks identified by the two neurosurgeons, and the masks we used were determined by a senior neurosurgeon (Y.C.) when the individual masks from the two neurosurgeons were inconsistent (>5%).
Based on PyRadiomics, a total of 858 radiomic features were extracted, including 7 location features, 18 first-order statistics (FOS) features, 14 shape- and size-based features, 75 texture features, and 744 wavelet features. The location features were extracted based on the method applied in the research of Liu et al. (10). In brief, a coordinate system with the anterior commissure (AC) as the origin point was developed in the Montreal Neurological Institute (MNI) space and the lesion segmentation of each patient was registered to the MNI template. Based on the coordinate system, the polar coordinates (γ, θ, and Φ) of the centroid of the bAVM and the distance (Cityblock, Chebyshev, Mahalanobis, and Cosine distance) from the AC to the centroid of the bAVM were calculated. The FOS features reflected the distribution of voxel values within the 3D matrix of the region of interest and the overall information of the bAVMs. The shape- and size-based features reflected the volume, surface area, and shape of the lesions. The texture features describing the internal and surface textures of the bAVMs included 24 gray-level co-occurrence matrix (GLCM) features, 14 gray-level dependence matrix (GLDM) features, 16 gray-level run-length matrix (GLRLM) features, 16 gray-level size zone matrix (GLSZM) features, and 5 neighborhood gray-tone difference matrix (NGTDM) features. The wavelet features were calculated by FOS, GLCM, GLDM, GLRLM, GLSZM, and NGTDM features through Coiflet 1 3D wavelet transform.
Epilepsy-related radiomic features were screened by the least absolute shrinkage and selection operator (LASSO) method, which is suitable for the regression of high-dimensional data. Meanwhile, multivariate logistic regression analysis was used to select independent predictors for epilepsy among clinical features. Three logistic regression models were constructed based on the screened features. The selected radiomics features were applied to build a radiomics model, and a radiomics score was calculated for each patient through a linear combination of the selected features weighted by their respective coefficients. A clinical model was developed based on the screened clinical features. Finally, a combined model was constructed by incorporating the significant variables of the clinical factors and the radiomics score.
Receiver operating characteristic (ROC) curve analysis based on 5-fold cross-validation was used to assess the model performance, and the area under the ROC curve (AUC) was calculated for quantification. Accuracy, sensitivity, specificity, positive predictive value, and negative predictive value were also used to assess the predictive performance. Additionally, calibration curves were plotted to assess the calibration of the combined model, accompanied by the Hosmer-Lemeshow test. Finally, to assess the clinical usefulness of the models, decision curve analysis (DCA) was performed by calculating the net benefits for a range of threshold probabilities in the whole cohort.
Statistical analyses of patients' baseline characteristics were performed with the SPSS statistical package (version 22.0.0). Continuous variables are summarized as the means ± SD, and categorical variables are summarized as frequency counts and percentages. Variables were compared between patients with or without epilepsy. Wilcoxon rank-sum tests, t-tests, and chi-squared tests were used as appropriate. Statistical tests were considered significant at P < 0.05, and variables with P < 0.05 in the univariate analysis were then used in the multivariate analysis. LASSO logistic analysis and figures with ROC curves were generated by Python (version 3.7). Nomogram construction, calibration plot construction, the Hosmer-Lemeshow test, and DCA were performed using R (version 4.0.2).
Between July 2012 and August 2020, a total of 176 patients with unruptured bAVMs were included in this study. The baseline characteristics of all patients are summarized in Table 1. Of 176 patients, 100 (56.8%) suffered from epilepsy and 76 (43.2%) did not. The ages of patients with or without epilepsy were 26.6 ± 11.6 years and 30.7 ± 11.9 years (P = 0.023), respectively. The lesion size of patients with epilepsy was 46.5 ± 12.6 mm, and that of patients without epilepsy was 40.8 ± 12.1 mm (P = 0.003). The number of niduses involving the frontal lobe was 55 for patients with epilepsy and 20 for patients without epilepsy (P < 0.001). There were no significant differences in the other characteristics mentioned in Table 1 between patients with epilepsy and those without epilepsy.
We extracted 12 clinical features and 858 quantitative radiomics features from a single patient. For clinical features, multivariable logistic regression analyses showed that patient age (P = 0.048), lesion size (P = 0.022), and frontal lobe involvement (P = 0.001) were independent risk factors for epilepsy (Supplementary Table 1). A clinical model was constructed based on these 3 clinical risk factors. Meanwhile, the radiomic model was constructed based on the 15 radiomics features screened by LASSO logistic analysis, including polar coordinates γ, polar coordinates Φ, WLLH.firstorder.Mean, WLLH.glcm.ClusterShade, WLLH.glcm.Correlation, WLHL.firstorder.Mean, WLHL.ngtdm.Strength, WLHH.firstorder.Mean, WHLL.gldm.DependenceVariance, WHLH.glszm.SmallAreaLowGrayLevelEmphasis, WHHL.glcm.Imc2, WHHL.glszm.SizeZoneNonUniformity Normalized, WHHH.firstorder.Skewness, WHHH.gldm.DependenceVariance, and WHHH.glszm.SmallAreaLowGrayLevelEmphasis. Finally, the combined model was constructed incorporating the clinical risk factors and the radiomics score calculated by the radiomic model.
ROC analysis was used to assess the ability of the 3 models to predict epilepsy. The ROC curves showed that the model built by the combination of clinical features and the radiomic score had favorable performance. The AUC for the combined model was 0.82 (95% CI 0.74–0.90), which was higher than that of the clinical model [AUC 0.71 (95% CI 0.62–0.80)] and radiomics model [AUC 0.78 (95% CI 0.71–0.85)] (Figure 2) Detailed results regarding the predictive performance of the 3 models are provided in Table 2.
Figure 2. Comparison of ROC curves based on 5-fold cross-validation among the three models. The clinical model is marked with an orange curve, and the AUC is 0.71 (95% CI 0.62–0.80). The radiomics model is marked with a blue curve, and the AUC is 0.78 (95% CI 0.71–0.85). The combined model is marked with a green curve, and the AUC is 0.82 (95% CI 0.74–0.90).
Based on the combined model, a radiomics-clinical nomogram was developed for visualization (Figure 3). The calibration curve of the nomogram demonstrated favorable agreement between the ground truth and the predicted probabilities of epilepsy (Figure 4A). The Hosmer-Lemeshow test yielded non-significant statistics (P = 0.243), which suggested that there was no departure from the perfect fit.
Figure 3. The radiomics-clinical nomogram derived from the combined model. The nomogram was built based on patient age, lesion size, involvement of the frontal lobe, and radiomics score. With the nomogram, the probability of epilepsy for each patient could be calculated on the basis of a logistic regression formula using the total points.
Figure 4. Calibration curve for the nomogram and decision curve analysis for the three models. (A) The calibration curve indicates the goodness-of-fit of the nomogram. The 45° gray dotted line represents the ideal prediction, and the green curve represents the predictive performance of the nomogram. The closer the green curve is to the ideal prediction line, the better the predictive efficacy of the nomogram. (B) Decision curve analysis of the three models in the whole cohort. The green curve, the blue curve, and the orange curve represent the net benefits of the combined model, the radiomics model, and the clinical model, respectively. The combined model had a higher net benefit than the other two models and simple diagnoses, such as all epilepsy patients (gray curve) or no epilepsy patients (black curve), across a large range of threshold probabilities at which a patient would be diagnosed with epilepsy.
The clinical utility of the 3 models was all evaluated, DCA was performed by quantifying the net benefits under different threshold probabilities. The DCA showed that the combined model had a higher overall net benefit in predicting epilepsy than the clinical model and the radiomics model across a large range of reasonable threshold probabilities, which demonstrated that the combined model had a relatively good performance in terms of clinical application (Figure 4B).
The accurate prediction of epilepsy could allow the customization of treatment options for patients with unruptured bAVMs. In this study, we developed and presented a quantitative and individualized epilepsy prediction model incorporating a series of clinical and TOF-MRA-based radiomics features associated with bAVM-related epilepsy. The results showed that the radiomics-clinical nomogram could successfully stratify patients according to their epilepsy risk. This easy-to-use nomogram may be a powerful clinical tool for assisting clinicians with personalized therapeutic decisions.
Recent developments in the field of radiomics have allowed the extraction of high-throughput imaging features, followed by automated analysis to assist clinical decision-making. Several studies have developed radiomics-based predictive models for various clinical characteristics, including survival outcomes (17), lymph node metastasis (18), and treatment responses (19). As epilepsy was among the important clinical characteristics associated with unruptured bAVMs, we applied the radiomics approach for the personalized prediction of epilepsy risk. Our results indicated that by incorporating the radiomics score and clinical characteristics, the risk of bAVM-related epilepsy could be evaluated with favorable accuracy.
Lesion location is an influential factor associated with bAVM-related epilepsy. Previous studies have shown that the frontal and temporal lobes are related to epilepsy in patients with bAVMs (4, 6). Moreover, at the brain subregion level, Zhang et al. found that the right precentral gyrus and the right superior longitudinal fasciculus tended to be epileptogenic, and the damage percentages to these regions of the lesions were considered location features and added into radiomic analyses for the prediction of bAVM-related epilepsy (9). Recently, a coordinate system was developed to quantitatively describe the location of brain lesions and was successfully applied to the prediction of tumor-related epilepsy by radiomics analysis (10, 20). In our study, we utilized this approach to obtain the location features of bAVMs. The polar coordinates based on the centroid of the bAVM and the distances from the AC to the centroid accurately described the lesion location, which provided more detailed information for the radiomic prediction models in the current study.
Studies of general epilepsy populations show that in 49–91% of patients with epilepsy, at least one seizure precipitant factor, including stress, lack of sleep, fatigue, emotions, and flickering lights, could be identified (21–24). It has been claimed that the prevention of seizure precipitants could improve the management of epilepsy (25–27). Therefore, patients with a high risk of epilepsy can benefit from behavior and lifestyle education if they could be screened out correctly with an imaging biomarker. This work is of great clinical significance considering that the radiomics-clinical nomogram showed favorable predictive efficacy in bAVM-related epilepsy, which could assist clinical treatment decision-making and achieve precision treatment.
The present study also has some limitations. First, given the stereotactic electroencephalographic data were not available, the diagnosis of the bAVM-related seizures was based on the clinical presentation. Second, the interpretability of radiomic features has remained an intractable task in the study of radiomics. Finally, although to our best knowledge, this study is the largest study termed patients with unruptured bAVMs, and our internal validation with a cross validation method showed favorable diagnostic performance, further external validation studies are needed to confirm our findings.
The radiomics features extracted from TOF-MRA were associated with bAVM-related epilepsy. Combining the radiomics and clinical features, the radiomics-clinical nomogram could be a reliable tool for personalized treatment in patients with unruptured bAVMs, assisting in clinical treatment decision-making and achieving precision treatment.
The raw data supporting the conclusions of this article will be made available by the authors, without undue reservation.
The studies involving human participants were reviewed and approved by the Ethics Committee of Beijing Tiantan Hospital, Capital Medical University. Written informed consent to participate in this study was provided by the patients/participants.
SZ designed the study, acquired the data, performed statistical analysis, and drafted the manuscript for intellectual content. QZ analyzed the data and revised the manuscript for intellectual content. YJ and HL masked the bAVMs on patients' TOF-MRA image and revised the manuscript for intellectual content. JWeng, RH, and JWang analyzed, interpreted the data, and revised the manuscript for intellectual content. HX and JZhang collected the data. YL, ZW, SW, and JZhao designed the study and revised the manuscript for intellectual. YC provided overall oversight of the research. All authors contributed to the article and approved the submitted version.
This study was supported by the National Natural Science Foundation of China (81901175), the National Key Research and Development Program of China during the 13th Five-Year Plan Period (2016YFC1301803).
The authors declare that the research was conducted in the absence of any commercial or financial relationships that could be construed as a potential conflict of interest.
All claims expressed in this article are solely those of the authors and do not necessarily represent those of their affiliated organizations, or those of the publisher, the editors and the reviewers. Any product that may be evaluated in this article, or claim that may be made by its manufacturer, is not guaranteed or endorsed by the publisher.
We are grateful to Feng Su from the Peking-Tsinghua Center for Life Sciences, Academy for Advanced Interdisciplinary Studies in the study design and manuscript revision.
The Supplementary Material for this article can be found online at: https://www.frontiersin.org/articles/10.3389/fneur.2021.767165/full#supplementary-material
AC, Anterior commissure; AUC, Area under the receiver operating curves; bAVM, Brain arteriovenous malformation; DCA, Decision curve analysis; FOS, First-order statistics; GLCM, Gray-level co-occurrence matrix; GLDM, Gray-level dependence matrix; GLRLM, Gray-level run-length matrix; GLSZM, Gray-level size zone matrix; LASSO, Least absolute shrinkage and selection operator; MNI, Montreal Neurological Institute; NGTDM, Neighborhood gray-tone difference matrix; ROC, Receiver operating characteristic; TOF-MRA, Time-of-flight Magnetic Resonance Angiography.
1. Hartmann A, Mohr JP. Acute management of brain arteriovenous malformations. Curr Treat Options Neurol. (2015) 17:346. doi: 10.1007/s11940-015-0346-5
2. Lawton MT, Rutledge WC, Kim H, Stapf C, Whitehead KJ, Li DY, et al. Brain arteriovenous malformations. Nat Rev Dis Primers. (2015) 1:15008. doi: 10.1038/nrdp.2015.8
3. Soldozy S, Norat P, Yagmurlu K, Sokolowski JD, Sharifi KA, Tvrdik P, et al. Arteriovenous malformation presenting with epilepsy: a multimodal approach to diagnosis and treatment. Neurosurg Focus. (2020) 48:E17. doi: 10.3171/2020.1.FOCUS19899
4. Hoh BL, Chapman PH, Loeffler JS, Carter BS, Ogilvy CS. Results of multimodality treatment for 141 patients with brain arteriovenous malformations and seizures: factors associated with seizure incidence and seizure outcomes. Neurosurgery. (2002) 51:303–9; discussion 9–11. doi: 10.1097/00006123-200208000-00004
5. Schramm J. Seizures associated with cerebral arteriovenous malformations. Handb Clin Neurol. (2017) 143:31–40. doi: 10.1016/B978-0-444-63640-9.00004-7
6. Benson JC, Chiu S, Flemming K, Nasr DM, Lanzino G, Brinjikji W. MR characteristics of unruptured intracranial arteriovenous malformations associated with seizure as initial clinical presentation. J Neurointerv Surg. (2020) 12:186–91. doi: 10.1136/neurintsurg-2019-015021
7. Mao N, Dai Y, Lin F, Ma H, Duan S, Xie H, et al. Radiomics nomogram of DCE-MRI for the prediction of axillary lymph node metastasis in breast cancer. Front Oncol. (2020) 10:541849. doi: 10.3389/fonc.2020.541849
8. Lambin P, Leijenaar RTH, Deist TM, Peerlings J, de Jong EEC, van Timmeren J, et al. Radiomics: the bridge between medical imaging and personalized medicine. Nat Rev Clin Oncol. (2017) 14:749–62. doi: 10.1038/nrclinonc.2017.141
9. Zhang Y, Yan P, Liang F, Ma C, Liang S, Jiang C. Predictors of epilepsy presentation in unruptured brain arteriovenous malformations: a quantitative evaluation of location and radiomics features on T2-weighted imaging. World Neurosurg. (2019) 125:e1008–15. doi: 10.1016/j.wneu.2019.01.229
10. Liu Z, Wang Y, Liu X, Du Y, Tang Z, Wang K, et al. Radiomics analysis allows for precise prediction of epilepsy in patients with low-grade gliomas. NeuroImage Clinical. (2018) 19:271–8. doi: 10.1016/j.nicl.2018.04.024
11. Yu S, Yan L, Yao Y, Wang S, Yang M, Wang B, et al. Noncontrast dynamic MRA in intracranial arteriovenous malformation (AVM), comparison with time of flight (TOF) and digital subtraction angiography (DSA). Magn Reson Imaging. (2012) 30:869–77. doi: 10.1016/j.mri.2012.02.027
12. Hadizadeh DR, Kukuk GM, Steck DT, Gieseke J, Urbach H, Tschampa HJ, et al. Noninvasive evaluation of cerebral arteriovenous malformations by 4D-MRA for preoperative planning and postoperative follow-up in 56 patients: comparison with DSA and intraoperative findings. Am J Neuroradiol. (2012) 33:1095–101. doi: 10.3174/ajnr.A2921
13. Mohr JP, Parides MK, Stapf C, Moquete E, Moy CS, Overbey JR, et al. Medical management with or without interventional therapy for unruptured brain arteriovenous malformations (ARUBA): a multicentre, non-blinded, randomised trial. Lancet. (2014) 383:614–21. doi: 10.1016/S0140-6736(13)62302-8
14. Fisher RS, Cross JH, French JA, Higurashi N, Hirsch E, Jansen FE, et al. Operational classification of seizure types by the International League Against Epilepsy: position Paper of the ILAE Commission for Classification and Terminology. Epilepsia. (2017) 58:522–30. doi: 10.1111/epi.13670
15. Fisher RS, van Emde Boas W, Blume W, Elger C, Genton P, Lee P, et al. Epileptic seizures and epilepsy: definitions proposed by the International League Against Epilepsy (ILAE) and the International Bureau for Epilepsy (IBE). Epilepsia. (2005) 46:470–2. doi: 10.1111/j.0013-9580.2005.66104.x
16. Jiao Y, Lin F, Wu J, Li H, Wang L, Jin Z, et al. A supplementary grading scale combining lesion-to-eloquence distance for predicting surgical outcomes of patients with brain arteriovenous malformations. J Neurosurg. (2018) 128:530–40. doi: 10.3171/2016.10.JNS161415
17. Aerts HJ, Velazquez ER, Leijenaar RT, Parmar C, Grossmann P, Carvalho S, et al. Decoding tumour phenotype by noninvasive imaging using a quantitative radiomics approach. Nat Commun. (2014) 5:4006. doi: 10.1038/ncomms5006
18. Huang YQ, Liang CH, He L, Tian J, Liang CS, Chen X, et al. Development and validation of a radiomics nomogram for preoperative prediction of lymph node metastasis in colorectal cancer. J Clin Oncol. (2016) 34:2157–64. doi: 10.1200/JCO.2015.65.9128
19. Nie K, Shi L, Chen Q, Hu X, Jabbour SK, Yue N, et al. Rectal cancer: assessment of neoadjuvant chemoradiation outcome based on radiomics of multiparametric MRI. Clin Cancer Res. (2016) 22:5256–64. doi: 10.1158/1078-0432.CCR-15-2997
20. Wang Y, Wei W, Liu Z, Liang Y, Liu X, Li Y, et al. Predicting the type of tumor-related epilepsy in patients with low-grade gliomas: a radiomics study. Front Oncol. (2020) 10:235. doi: 10.3389/fonc.2020.00235
21. Samsonsen C, Sand T, Brathen G, Helde G, Brodtkorb E. The impact of sleep loss on the facilitation of seizures: a prospective case-crossover study. Epilepsy Res. (2016) 127:260–6. doi: 10.1016/j.eplepsyres.2016.09.014
22. Balamurugan E, Aggarwal M, Lamba A, Dang N, Tripathi M. Perceived trigger factors of seizures in persons with epilepsy. Seizure. (2013) 22:743–7. doi: 10.1016/j.seizure.2013.05.018
23. Ferlisi M, Shorvon S. Seizure precipitants (triggering factors) in patients with epilepsy. Epilepsy Behav. (2014) 33:101–5. doi: 10.1016/j.yebeh.2014.02.019
24. Sperling MR, Schilling CA, Glosser D, Tracy JI, Asadi-Pooya AA. Self-perception of seizure precipitants and their relation to anxiety level, depression, and health locus of control in epilepsy. Seizure. (2008) 17:302–7. doi: 10.1016/j.seizure.2007.09.003
25. Schmid-Schönbein C. Improvement of seizure control by psychological methods in patients with intractable epilepsies. Seizure. (1998) 7:261–70. doi: 10.1016/S1059-1311(98)80017-4
26. Wolf P, Okujava N. Possibilities of non-pharmacological conservative treatment of epilepsy. Seizure. (1999) 8:45–52. doi: 10.1053/seiz.1998.0243
Keywords: brain arteriovenous malformations, radiomics analysis, epilepsy, machine learning, time-of-flight magnetic resonance angiography
Citation: Zhao S, Zhao Q, Jiao Y, Li H, Weng J, Huo R, Wang J, Xu H, Zhang J, Li Y, Wu Z, Wang S, Cao Y and Zhao J (2021) Radiomics Analysis for Predicting Epilepsy in Patients With Unruptured Brain Arteriovenous Malformations. Front. Neurol. 12:767165. doi: 10.3389/fneur.2021.767165
Received: 30 August 2021; Accepted: 22 November 2021;
Published: 15 December 2021.
Edited by:
Mohd Farooq Shaikh, Monash University, MalaysiaReviewed by:
S. Ottavio Tomasi, Paracelsus Medical University, AustriaCopyright © 2021 Zhao, Zhao, Jiao, Li, Weng, Huo, Wang, Xu, Zhang, Li, Wu, Wang, Cao and Zhao. This is an open-access article distributed under the terms of the Creative Commons Attribution License (CC BY). The use, distribution or reproduction in other forums is permitted, provided the original author(s) and the copyright owner(s) are credited and that the original publication in this journal is cited, in accordance with accepted academic practice. No use, distribution or reproduction is permitted which does not comply with these terms.
*Correspondence: Yong Cao, Y2FveW9uZ0BianR0aC5vcmc=
Disclaimer: All claims expressed in this article are solely those of the authors and do not necessarily represent those of their affiliated organizations, or those of the publisher, the editors and the reviewers. Any product that may be evaluated in this article or claim that may be made by its manufacturer is not guaranteed or endorsed by the publisher.
Research integrity at Frontiers
Learn more about the work of our research integrity team to safeguard the quality of each article we publish.