- 1Department of Psychology, University of California, Los Angeles, Los Angeles, CA, United States
- 2Department of Neurosurgery, Brain Injury Research Center (BIRC), UCLA Brain Injury Research Center, David Geffen School of Medicine, University of California, Los Angeles, Los Angeles, CA, United States
While electroencephalogram (EEG) burst-suppression is often induced therapeutically using sedatives in the intensive care unit (ICU), there is hitherto no evidence with respect to its association to outcome in moderate-to-severe neurological patients. We examined the relationship between sedation-induced burst-suppression (SIBS) and outcome at hospital discharge and at 6-month follow up in patients surviving moderate-to-severe traumatic brain injury (TBI). For each of 32 patients recovering from coma after moderate-to-severe TBI, we measured the EEG burst suppression ratio (BSR) during periods of low responsiveness as assessed with the Glasgow Coma Scale (GCS). The maximum BSR was then used to predict the Glasgow Outcome Scale extended (GOSe) at discharge and at 6 months post-injury. A multi-model inference approach was used to assess the combination of predictors that best fit the outcome data. We found that BSR was positively associated with outcomes at 6 months (P = 0.022) but did not predict outcomes at discharge. A mediation analysis found no evidence that BSR mediates the effects of barbiturates or propofol on outcomes. Our results provide initial observational evidence that burst suppression may be neuroprotective in acute patients with TBI etiologies. SIBS may thus be useful in the ICU as a prognostic biomarker.
Introduction
Biomarkers of recovery are greatly needed in coma following traumatic brain injury (TBI) for prognosis and to inform crucial medical and management decisions, including withdrawal of life-sustaining care (1). Associating electroencephalogram (EEG) patterns such as burst-suppression with patient outcomes may allow for the discovery of prognostic biomarkers. EEG burst-suppression patterns are commonly observed in coma patients, but are challenging to interpret since, in different contexts, their presence may be considered benign, therapeutic, or life-threatening (2). Pharmacologically-induced burst-suppression (i.e., deep anesthesia) is generally regarded as safe and is often induced intentionally in the intensive care unit (ICU) to reduce the cerebral metabolic rate and intracranial pressure (ICP) in patients with severe traumatic brain injury (TBI) (3, 4) and/or nonconvulsive status epilepticus (5). However, even in a therapeutic context, Niedermeyer and colleagues described burst-suppression as “incompatible with normal brain functioning” and therefore harmful, albeit allowing for vast recovery (2). Yet, to date, no study has specifically investigated the association between this EEG pattern and chronic outcome in patients surviving moderate-to-severe TBI. In what follows, we examine sedation-induced burst-suppression (SIBS) and assess the association between EEG burst-suppression ratio (BSR) at the acute time-point (i.e., ~3 weeks post-injury) and global outcome, as measured by the Glasgow Outcome Scale extended (GOSe) (6), at discharge from the hospital (2–51 days post-injury) and at a chronic (~6 month) follow-up.
Methods
Subjects
Our sample consisted of 32 patients with usable data admitted at the UCLA Ronald Reagan University Medical Center Neuroscience/Trauma ICU from December 2015 to January 2020 following moderate-to-severe TBI. Inclusion criteria were an admission Glasgow Coma Scale (GCS) (7) score ≤ 8 or an admission GCS score of 9–14 with computed tomography (CT) evidence of intracranial bleeding. Exclusion criteria were a GCS > 14 with non-significant head CT, history of neurologic disease or TBI, and brain death. Our sample size was not determined a priori but rather constrained by the number of available patients. For a flowchart illustrating how many patients were excluded at each step of the data processing pipeline and why, see Supplementary Figure 1. The study was approved by the UCLA Institutional Review Board; informed assent and consent were obtained per state regulations. Multiday EEG was recorded continuously (Cz reference) throughout each patient's stay in the ICU. Data were acquired using Nicolet (Natus Medical, Inc., Pleasanton, CA, USA) or Moberg ICU Solutions (Moberg Research, Inc., Ambler, PA, USA) EEG systems. Persyst software (Persyst Development Corporation, Solana Beach, CA, USA) was used to de-identify and export EEG data in European Data Format (EDF) to MATLAB (version R2019b, The MathWorks, Inc., Natick, MA, USA) for analysis. Anesthetic medications, administered to manage symptoms and/or reduce cerebral metabolism, were noted on a daily basis and sorted into two categories (barbiturates and propofol). In particular, burst suppression was induced using barbiturates in patients with therapy refractory ICP elevation, following evidence (4) showing efficacy for treatment of ICP, and to treat increased brain edema.
Behavioral Assessments
During ICU hospitalization, behavioral assessments were performed several times daily using the GCS. Global outcomes were assessed with the GOSe in-person interview protocol at hospital discharge 2–51 days post-injury (22 ± 10 days, mean ± std) and either in-person or by phone to patients and/or family members at a chronic follow-up 161 – 318 days post-injury (193 ± 34.5 days, mean ± std; 4 patients had chronic assessments more than 7 months post-injury, see Table 1).
EEG Section Selection
Our analysis of burst suppression was data-driven (i.e., we measured burst suppression using the BSR computed directly from EEG data rather than inferring bust suppression from other records). The goal of our EEG selection was therefore to include the most discontinuous EEG from all patients by extracting those sections of EEG that corresponded to low behavioral responsiveness. If burst suppression was not used in patients or they otherwise did not show discontinuous EEG, their data were still included and benefited the analysis by increasing the between-subjects variance in BSR. To analyze patients during periods of minimal responsiveness when SIBS was used, we extracted 10 min of EEG from 13 channels common to all patients (Figure 1A) from timepoints corresponding to low GCS scores, with EEG sections spaced a minimum of 24 h apart (Supplementary Figure 2). Note that although low GCS scores do not necessarily imply sedation, we expected sedation to only coincide with low GCS scores and therefore used GCS scores to guide EEG extraction. Specifically, EEG sections were extracted by sorting each patient's GCS scores from low to high, appending the lowest score to a second list, and then crawling down the first list of GCS scores and adding each timepoint that was at least 24 h from any timepoint on the second list to the second list. EEG sections were then extracted from the second list's timepoints.
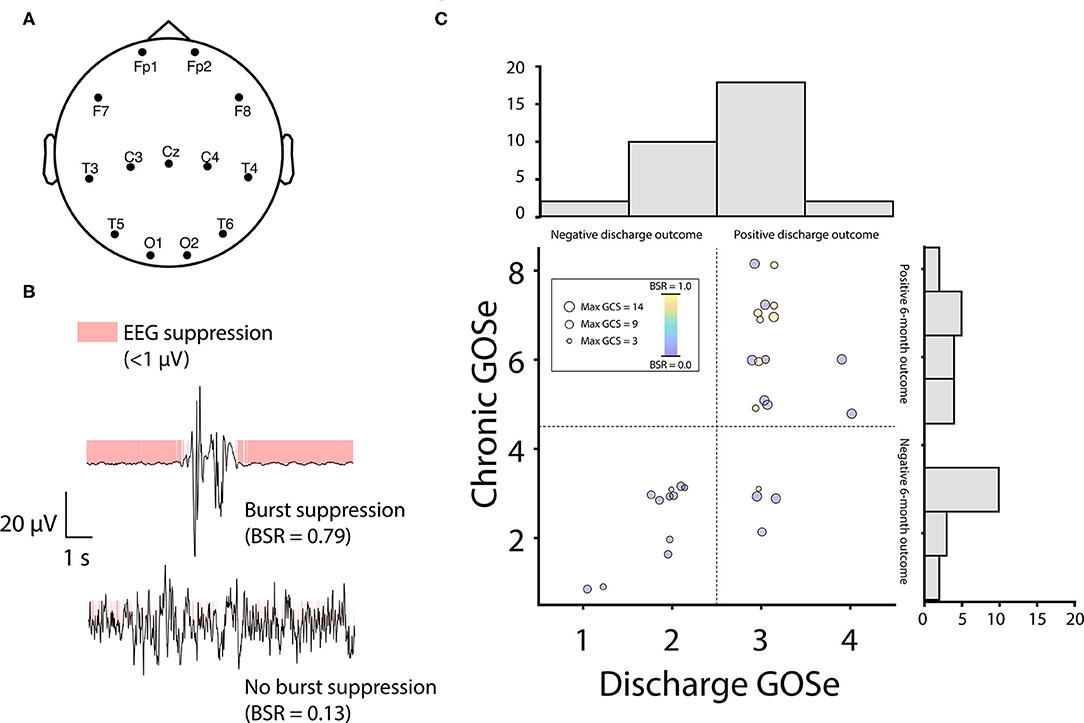
Figure 1. EEG burst-suppression positively predicts outcomes at chronic follow up. (A) EEG signals were recorded from 13 channels common to all patients. Channel placement was frequently modified from standard positions (shown above) to accommodate bone flaps and injury sites in individual patients. (B) The EEG burst suppression ratio (BSR) was computed as the proportion of clean signal with a rectified amplitude < 1 μV (mode across channels). (C) Outcomes were assessed using the Glasgow Outcome Scale extended (GOSe) and were divided at discharge and chronic timepoints using median splits (dotted lines, GOSe scores are scattered with jitter to avoid overlap). Patients with burst-suppression (red circles) were overrepresented in the upper half of each median split (blue circles = no burst-suppression; circle size is proportional to patient's highest Glasgow Coma Scale score). Note that 2 patients missing chronic GOSe scores (Patients 4 and 10, Table 1) are not shown in the scatter plot but are represented in the discharge GOSe histogram. GOSe scores at discharge showed low spread, with most patients either scoring 2 (vegetative state) or 3 (low severe disability). Similarly, chronic GOSe scores showed a bimodal distribution with peaks flanking a large dip at 4 (upper severe disability). Owing to this fact, we opted for a median split on scores (discharge: 1-2 vs. 3-4; chronic: 1-4 vs. 5-8) to create dichotomous outcome variables for logistic regression. In the upper half of both the discharge and chronic GOSe median split, 40% of patients displayed BSR > 0.5, vs. 0% (discharge) and 6.7% (chronic) of patients in the lower half of the GOSe distribution.
EEG Data Processing
EEG extraction covered time points most likely to contain burst suppression (as determined by low GCS, see above) and was unbiased with respect to patient outcomes. Data were extracted from 13 channels common to all patients (Figure 1A), resulting in 215 EEG sections from 40 patients that entered preprocessing (corrupted data from one patient were rejected prior to preprocessing). After importing, all EEG data were re-referenced to average and bandpass filtered 0.5–45 Hz to attenuate low frequency drift and high frequency muscle artifacts and line noise. EEG data were further artifact reduced using a combination of manual exclusion of bad segments and independent component analysis (ICA) (10). EEG sections corresponding to excessive noise or disconnected equipment were discarded.
Burst Suppression Ratio
We computed the BSR of usable EEG sections as the proportion of the total data with an amplitude < 1 μV [in line with the range recommended by Westover and colleagues (11); see Figure 1B and Supplementary Figure 3]. Data were binarized using this threshold (as chosen according to the method described below) after rectifying the signal (12), and the modal binary value across channels was used to construct a single time series on which the proportion was based (Figure 1B). Higher proportions correspond to more discontinuous EEG patterns and lower proportions correspond to more continuous EEG patterns. Note that we did not compute time spent in burst suppression, as we were only interested in the greatest depth of burst suppression (i.e., maximum BSR) for each patient and, moreover, computing total time spent in burst suppression would be impractical as it would require processing and analyzing several days of EEG recordings for each patient.
To determine the proper threshold for computing the BSR, we performed a sensitivity analysis by computing BSR on all usable EEG sections from all 32 patients in Table 1, plus 2 additional patients excluded from other analyses due to missing GOSe (74-year-old male) and medication (23-year-old female) data whose EEG data were nonetheless perfectly suitable for this particular analysis. This yielded 154 EEG sections from 34 patients. We used several different thresholds (1, 5, and 10 μV; Supplementary Figure 3) and then compared the resulting BSR values to 1) the concurrent GCS score and 2) the log-transformed kurtosis of voltage amplitudes, which should scale linearly with BSR (with the exception of BSR = 1.0 or isoelectric EEG, in which the complete absence of EEG bursts yields low kurtosis values).
Statistical Analysis
In order to relate each patient's greatest depth of burst suppression to global outcome, we extracted the maximum BSR across all EEG sections for each patient. Owing to their non-normal and non-linear characteristics (13), GOSe scores were binarized according to a median split (Figure 1C). Model selection and averaging were performed using the Multi-Model Inference package in R (version 3.6.2) (14). Multiple logistic regression models were fitted to predict outcome using any of the following: maximum BSR, maximum GCS score, sex, and age at injury (all models included an intercept). We covaried for the maximum GCS given that it reflects the patient's overall behavioral recovery in the ICU. Note that the maximum GCS was computed from the patient's entire ICU stay and not just those timepoints for which EEG data were extracted (which corresponded to GCS minima rather than maxima). Model averaged parameters were derived using all models within 5 small-sample corrected Akaike information criterion (AICc) units of the leading model for each dependent variable (discharge and chronic outcome, see Table 2).
Mediation Analysis
Given that burst suppression was induced by sedatives, we asked whether BSR mediates effects of barbiturates and/or propofol on outcomes. Mediation analyses were performed in R (version 3.6.2) using the Mediation package (15). Specifically, we ran nonparametric mediation analyses using 1000 Monte Carlo simulation per analysis. Four separate analyses were run with barbiturates/propofol as the independent variable and discharge/chronic outcome as the dependent variable. In addition to these, we also ran analyses with BSR as the independent variable and medication as the mediator. Medications were coded as binary variables according to whether the patient was administered the medication on the same day as the patient's maximum BSR. Each analysis included a regression model predicting the mediator from the independent variable and another regression model predicting outcome from the independent variable and mediator; logistic regression was used when predicting binary variables. Analyses also controlled for each patient's maximum GCS score, as this was the only significant covariate in any analysis (see section Results).
Missing Data Analysis
To investigate whether n = 8 patients who were excluded due to excessive noise, technical artifacts, or missing medication data (see Supplementary Figure 1 for an illustration of where each patient was excluded in the workflow) were different in their outcomes than patients whose data were retained, we compared the proportion of patients with outcomes above and below the median split cutoff for the GOSe at discharge (0 if < 3, otherwise 1) and chronic (0 if < 5, otherwise 1) assessments using a chi-squared test.
Results
Prior to analysis, data from 8 patients that had entered preprocessing were excluded entirely (Supplementary Figure 1) for the following reasons: 1) data quality (e.g., due to disconnected equipment or unremovable technical artifacts; 6 patients), 2) no GOSe assessments (1 patient), 3) no medication data (1 patient). Of the 32 remaining patients (Table 1), 2 patients were missing chronic GOSe scores and excluded from its prediction; these patients were also excluded from mediation analyses. Patients in our sample were predominantly male (84%), reflecting a frequently reported greater risk in males for TBI (8, 9). EEG processing yielded 4.6 ± 1.8 usable EEG sections per patient, or 88 ± 17% of extracted EEG sections (mean ± std).
Burst Suppression Ratio Threshold
Of three different voltage threshold values (1, 5, and 10 μV), we selected a 1 μV threshold given that this threshold 1) only yielded high BSR values for EEG sections coinciding with GCS = 3 (i.e., the lowest possible responsiveness score) and 2) yielded BSR values that correlated strongly with the log10(kurtosis) of the signal (r = 0.88) (see Supplementary Figure 3 for further details).
EEG Patterns
Ten patients (31%) displayed visual evidence of an isoelectric or burst suppression EEG (Supplementary Figure 4); however, BSR was treated as a continuous variable and was not thresholded or otherwise transformed to create categories of burst suppression and non-burst suppression. EEG patterns followed an expected progression from continuous EEG (low BSR) to burst suppression (high BSR), followed by isoelectric EEG (BSR ~ 1). See Supplementary Figure 4 for representative examples of EEG occurring at maximum BSR for all patients. The proportion of patients sedated with barbiturates increased with BSR (Supplementary Figure 5), reaching 90% at BSR > 0.15 and 100% at BSR > 0.45. EEG channels showed nearly perfect agreement regarding whether the rectified signal exceeded the 1 μV threshold as measured using intraclass correlations (ICCs) derived from each patient's EEG section corresponding to the maximum BSR (mean ICC = 0.999, min ICC = 0.992, max ICC = 1.0).
Multi-Model Inference
GOSe scores were not correlated with number of post-injury days to assessment (discharge: r = −0.24, P = 0.18; chronic: r = 0.05, P = 0.79). Using multi-model inference, model parameters were averaged across four models each to predict discharge and chronic outcomes. No variable significantly predicted outcome at discharge. Maximum BSR (P = 0.022) and maximum GCS (P = 0.011) significantly predicted outcome at 6 months (Table 3). Next, medications were examined as binary variables indicating whether the patient was given the medication on the day of maximum BSR. No significant mediation effects were detected after modeling barbiturates and propofol separately as independent variables and BSR as a mediator variable and vice versa.
Missing Data Analysis
We compared outcomes from excluded patients (n = 8, see Supplementary Figure 1) to all patients with discharge GOSe scores (n = 32) and chronic GOSe scores (n = 30). In the case of discharge outcomes, 62.5 and 75% of patients fell above the median split (i.e., GOSe ≥ 3) in the retained and excluded samples, respectively. In the case of chronic outcomes, 50% of patients fell above the median split (i.e., GOSe ≥ 5) in both the retained and excluded samples. Proportions of outcomes above the median split did not significantly differ between retained and excluded patients at discharge (χ2 = 0.44, P =0.51) or chronic (χ2 = 0, P = 1.0) assessments.
Discussion
In this study, we show that the use of SIBS in the ICU is associated with favorable outcome (i.e., GOSe ≥ 5) at > 5 months post-injury in TBI patients, after controlling for patients' maximum behavioral recovery (i.e., highest GCS score) in the ICU. Moreover, while all patients with SIBS were administered barbiturates (see Supplementary Figure 5), we did not find evidence that BSR mediated an effect of sedatives on outcome. Our results suggest that the use of SIBS in the setting of moderate-to-severe TBI should not automatically imply a poor prognosis, and may in fact be a biomarker of the brain's ability to respond to decreased metabolic demand.
Our finding that SIBS predicts chronic outcomes is particularly important in light of our recent finding that other EEG variables, such as oscillatory power and the presence/absence of power spectral peaks, do not predict chronic outcomes in the same cohort of patients with moderate-to-severe TBI (16). Our current analysis—which examined a subset of this cohort—taken together with our other recent findings cited above, demonstrates that SIBS predicts chronic outcomes in TBI better than conventional models, such as the ABCD model of mesocircuit recovery (16), which infers thalamocortical integrity from spectral peaks and does not explicitly take EEG burst suppression into account (17, 18). Furthermore, our results herein were achieved using sparse spatial sampling (i.e., 13 channels) with clinical, rather than research-grade, EEG, and we observed near-perfect correlations in the presence/absence of suppression between EEG channels, with an average ICC of 0.999 across patients. The foregoing suggests that our results are not dependent on spatial location and could be reproduced using many other EEG systems, including montages as simple as a single bipolar channel.
One implication of our findings is that coma recovery may follow one of two main trajectories. The first trajectory is the previously reported association between early recovery, as captured by the GCS, and better outcome (19, 20). The second trajectory is characterized by deep burst-suppression coma and a state of low maximum responsiveness in the ICU. Because this trajectory is also associated with positive outcomes, one may infer that SIBS is likely, from a mechanistic standpoint, neuroprotective and, from an observational standpoint, a biomarker of later recovery that may inform decisions to sustain care, contrary to published guidelines in other coma etiologies (21–23). Along these lines, a randomized multi-site study conducted several decades ago by Eisenberg and colleagues (4) demonstrated that treatment by high-dose barbiturates acutely improves ICP in patients with severe head injury by a factor of at least 2:1, increasing to 4:1 after controlling for prerandomization cardiac factors.
Study Limitations
Several possible limitations of our study should be noted. 1) It cannot be ruled out that our method of extracting local portions of EEG may have missed burst-suppression in some patients. However, our sampling was unbiased such that there is no reason to believe that our sensitivity varied with patients' outcomes. 2) After exporting EEG files to EDF format to be analyzed for our study, it was brought to our attention that discontinuities in EEG recordings (e.g., when EEG equipment is temporarily disconnected) may not be reflected in timestamps. Thus, it is possible EEG may have been sampled at times asynchronously to the targeted portions defined by GCS scores. However, we observed that burst suppression/muscle activity coincided with low/high GCS scores, suggesting that GCS and EEG data were well aligned. 3) Because we did not have access to the time of day at which medications were given, it was not possible to confirm that anesthetic drugs were administered before burst suppression in EEG. However, it is likely that burst suppression was sedative-induced, given the causal relationship between sedatives and burst suppression and the close temporal proximity of the two events.
Conclusions
SIBS might be a candidate biomarker of prognosis that relates positively with desirable outcomes in coma recovery following TBI. Future work is needed to clarify the neural mechanisms underlying this association and to determine why this same EEG pattern is, in other etiologies, generally associated with the opposite (i.e., poor) outcome (2, 24). Furthermore, the extent to which the therapeutic effect of SIBS is dose-dependent remains unknown and will require a future prospective study.
Data Availability Statement
The datasets presented in this article are not readily available because researchers seeking to access data must first complete a material transfer agreement with UCLA. Requests to access the datasets should be directed to Joel Frohlich, am9lbGZyb2hsaWNoQGdtYWlsLmNvbQ==.
Ethics Statement
The studies involving human participants were reviewed and approved by University of California Los Angeles Institutional Review Board. Informed consent for participation was obtained from patients' family members.
Author Contributions
JF and MM conceived of the idea for this study and wrote the original draft of the manuscript. CR, VS, and JR collected and curated the data. EL and NS organized behavioral and medication data from patients. JD provided computing resources. JF processed and analyzed the data and also generated figures and tables. MJ and DM contributed to the statistical analysis. PV and MM acquired funding and supervised the project. All authors contributed to reviewing and editing the manuscript.
Funding
This work was supported by grants from the Tiny Blue Dot Foundation (MM), the National Institute of Neurological Disorders and Stroke (PV, Grant Numbers NS058489, NS100064, and NS049471), and the State of California Neurotrauma Initiative (PV). Sponsors had no influence over any aspect of the work.
Conflict of Interest
PV received personal fees from Ceribell and UCB Pharma outside the submitted work.
The remaining authors declare that the research was conducted in the absence of any commercial or financial relationships that could be construed as a potential conflict of interest.
Publisher's Note
All claims expressed in this article are solely those of the authors and do not necessarily represent those of their affiliated organizations, or those of the publisher, the editors and the reviewers. Any product that may be evaluated in this article, or claim that may be made by its manufacturer, is not guaranteed or endorsed by the publisher.
Acknowledgments
We are grateful to the patients who participated in this research and their families. We are also grateful to Joerg Hipp and Pilar Garces for sharing EEG processing code.
Supplementary Material
The Supplementary Material for this article can be found online at: https://www.frontiersin.org/articles/10.3389/fneur.2021.750667/full#supplementary-material
References
1. Giacino JT, Fins JJ, Laureys S, Schiff ND. Disorders of consciousness after acquired brain injury: the state of the science. Nat Rev Neurol. (2014) 10:99. doi: 10.1038/nrneurol.2013.279
2. Niedermeyer E, Sherman DL, Geocadin RJ, Hansen HC, Hanley DF. The burst-suppression electroencephalogram. Clin Electroencephalogr. (1999) 30:99–105. doi: 10.1177/155005949903000305
3. Doyle P, Matta B. Burst suppression or isoelectric encephalogram for cerebral protection: evidence from metabolic suppression studies. Brit J Anaesth. (1999) 83:580–4. doi: 10.1093/bja/83.4.580
4. Eisenberg HM, Frankowski RF, Contant CF, Marshall LF, Walker MD. High-dose barbiturate control of elevated intracranial pressure in patients with severe head injury. J Neurosurg. (1988) 69:15–23. doi: 10.3171/jns.1988.69.1.0015
5. Jordan KG, Hirsch LJ. Nonconvulsive Status Epilepticus (NCSE), treat to burst-suppression: pro and con. Epilepsia. (2006) 47:41–5. doi: 10.1111/j.1528-1167.2006.00659.x
6. Jennett B, Snoek J, Bond M, Brooks N. Disability after severe head injury: observations on the use of the Glasgow Outcome Scale. J Neurol Neurosurg Psychiatry. (1981) 44:285–93. doi: 10.1136/jnnp.44.4.285
7. Teasdale G, Jennett B. Assessment of coma and impaired consciousness: a practical scale. Lancet. (1974) 304:81–4. doi: 10.1016/S0140-6736(74)91639-0
8. Javouhey E, Guerin A-C, Chiron M. Incidence and risk factors of severe traumatic brain injury resulting from road accidents: a population-based study. Accid Anal Prevent. (2006) 38:225–33. doi: 10.1016/j.aap.2005.08.001
9. Ottochian M, Salim A, Berry C, Chan LS, Wilson MT, Margulies DR. Severe traumatic brain injury: is there a gender difference in mortality? Am J Surg. (2009) 197:155–8. doi: 10.1016/j.amjsurg.2008.09.008
11. Westover MB, Shafi MM, Ching S, Chemali JJ, Purdon PL, Cash SS, et al. Real-time segmentation of burst suppression patterns in critical care EEG monitoring. J Neurosci Methods. (2013) 219:131–41. doi: 10.1016/j.jneumeth.2013.07.003
12. Chemali J, Ching S, Purdon PL, Solt K, Brown EN. Burst suppression probability algorithms: state-space methods for tracking EEG burst suppression. J Neural Eng. (2013) 10:56017. doi: 10.1088/1741-2560/10/5/056017
13. Crone JS, Lutkenhoff ES, Vespa PM, Monti MM. A systematic investigation of the association between network dynamics in the human brain and the state of consciousness. Neurosci Consciou. (2020) 2020:niaa008. doi: 10.1093/nc/niaa008
15. Tingley D, Yamamoto T, Hirose K, Keele L, Imai K. Mediation: R Package for Causal Mediation Analysis. (2014) doi: 10.18637/jss.v059.i05
16. Frohlich J, Crone JS, Johnson MA, Lutkenhoff ES, Spivak NM, Dell'Italia J, et al. Neural oscillations track recovery of consciousness in acute traumatic brain injury patients. Hum Brain Mapp. (2021) 1–17. doi: 10.1002/hbm.25725
17. Schiff ND. Mesocircuit mechanisms underlying recovery of consciousness following severe brain injuries: model and predictions. In: Brain Function and Responsiveness in Disorders of Consciousness. Springer. p. 195–204. doi: 10.1007/978-3-319-21425-2_15
18. Forgacs PB, Frey H, Velazquez A, Thompson S, Brodie D, Moitra V, et al. Dynamic regimes of neocortical activity linked to corticothalamic integrity correlate with outcomes in acute anoxic brain injury after cardiac arrest. Ann Clin Transl Neurol. (2017) 4:119–29. doi: 10.1002/acn3.385
19. Foreman BP, Caesar RR, Parks J, Madden C, Gentilello LM, Shafi S, et al. Usefulness of the abbreviated injury score and the injury severity score in comparison to the Glasgow Coma Scale in predicting outcome after traumatic brain injury. J Trauma Acute Care Surg. (2007) 62:946–50. doi: 10.1097/01.ta.0000229796.14717.3a
20. Martino C, Russo E, Santonastaso DP, Gamberini E, Bertoni S, Padovani E, et al. Long-term outcomes in major trauma patients and correlations with the acute phase. World J Emerg Surg. (2020) 15:1–7. doi: 10.1186/s13017-020-0289-3
21. Callaway CW, Donnino MW, Fink EL, Geocadin RG, Golan E, Kern KB, et al. Part 8: post–cardiac arrest care: 2015 American Heart Association guidelines update for cardiopulmonary resuscitation and emergency cardiovascular care. Circulation. (2015) 132:S465–82. doi: 10.1161/CIR.0000000000000262
22. Nolan JP, Soar J, Cariou A, Cronberg T, Moulaert VR, Deakin CD, et al. European resuscitation council and European society of intensive care medicine 2015 guidelines for post-resuscitation care. Intens Care Med. (2015) 41:2039–56. doi: 10.1007/s00134-015-4051-3
23. Rossetti AO, Rabinstein AA, Oddo M. Neurological prognostication of outcome in patients in coma after cardiac arrest. Lancet Neurol. (2016) 15:597–609. doi: 10.1016/S1474-4422(16)00015-6
Keywords: traumatic brain injury (TBI), burst suppression, barbiturates, coma, disorders of consciousness, EEG biomarker, Glasgow Coma Scale (GCS), Glasgow Outcome Scale extended (GOSe)
Citation: Frohlich J, Johnson MA, McArthur DL, Lutkenhoff ES, Dell'Italia J, Real C, Shrestha V, Spivak NM, Ruiz Tejeda JE, Vespa PM and Monti MM (2021) Sedation-Induced Burst Suppression Predicts Positive Outcome Following Traumatic Brain Injury. Front. Neurol. 12:750667. doi: 10.3389/fneur.2021.750667
Received: 31 July 2021; Accepted: 19 November 2021;
Published: 22 December 2021.
Edited by:
Nicole Osier, University of Texas at Austin, United StatesReviewed by:
Eugene Golanov, Houston Methodist Hospital, United StatesPatrick Czorlich, University Medical Center Hamburg-Eppendorf, Germany
Copyright © 2021 Frohlich, Johnson, McArthur, Lutkenhoff, Dell'Italia, Real, Shrestha, Spivak, Ruiz Tejeda, Vespa and Monti. This is an open-access article distributed under the terms of the Creative Commons Attribution License (CC BY). The use, distribution or reproduction in other forums is permitted, provided the original author(s) and the copyright owner(s) are credited and that the original publication in this journal is cited, in accordance with accepted academic practice. No use, distribution or reproduction is permitted which does not comply with these terms.
*Correspondence: Joel Frohlich, am9lbGZyb2hsaWNoQGdtYWlsLmNvbQ==