- 1School of Nursing, University of Texas at Austin, Austin, TX, United States
- 2Department of Pediatrics, Baylor College of Medicine, Houston, TX, United States
- 3Department of Psychiatry and Behavioral Sciences, Stanford University, Palo Alto, CA, United States
Objective: We aimed to characterize local brain network connectivity in long-term breast cancer survivors compared to newly diagnosed patients.
Methods: Functional magnetic resonance imaging (fMRI) and subjective cognitive and psychological function data were obtained from a group of 76 newly diagnosed, pre-treatment female patients with breast cancer (mean age 57 ± 7 years) and a separate group of 80, post-treatment, female breast cancer survivors (mean age 58 ± 8; mean time since treatment 44 ± 43 months). The network-based statistic (NBS) was used to compare connectivity of local brain edges between groups. Hubs were defined as nodes with connectivity indices one standard deviation or more above network mean and were further classified as provincial (higher intra-subnetwork connectivity) or connector (higher inter-subnetwork connectivity) using the participation coefficient. We determined the hub status of nodes encompassing significantly different edges and correlated the centralities of edges with behavioral measures.
Results: The post-treatment group demonstrated significantly lower subjective cognitive function (W = 3,856, p = 0.004) but there were no group differences in psychological distress (W = 2,866, p = 0.627). NBS indicated significantly altered connectivity (p < 0.042, corrected) in the post-treatment group compared to the pre-treatment group largely in temporal, frontal-temporal and temporal-parietal areas. The majority of the regions projecting these connections (78%) met criteria for hub status and significantly less of these hubs were connectors in the post-treatment group (z = 1.85, p = 0.031). Subjective cognitive function and psychological distress were correlated with largely non-overlapping edges in the post-treatment group (p < 0.05).
Conclusion: Widespread functional network alterations are evident in long-term survivors of breast cancer compared to newly diagnosed patients. We also demonstrated that there are both overlapping and unique brain network signatures for subjective cognitive function vs. psychological distress.
Introduction
Cancer and its treatments are associated with risk for cognitive dysfunction, most commonly in the domains of executive functioning, memory, attention, and processing speed (1, 2). Neuroimaging studies suggest that cancer-related cognitive impairment (CRCI) results from injury to brain structure and function. We previously showed that breast cancer survivors have altered brain network connectivity compared to healthy controls and chemotherapy naïve survivors (3–9). Other groups have subsequently observed similar findings (10–20).
Most of these studies, including our own, have focused largely on global brain network characteristics and/or local connectivity among a limited number of discrete regions. The advantages of these approaches include the provision of single, parsimonious metrics that summarize the vast complexity of the entire brain network (global properties) and the testing of specific hypotheses (limited local connectivity). Examination of all potential differences among local brain network connections is agnostic and requires larger samples but can provide a broader profile of potential biomarker features for modeling applications.
For example, we have previously utilized brain connectivity (connectome) features in machine learning models for predicting future cognitive outcome following breast cancer chemotherapy treatment (21, 22) and identifying neurophysiological clusters associated with cognitive impairment subtypes (8). However, these required complex feature selection/dimension reduction techniques due to limited a priori data regarding important local features. Additionally, there are emerging methods for deriving transcriptome profiles of connectome topology (23–25). These methods integrate region-specific transcriptomes with regional connectome properties (24). For example, Zhao et al. (26) used transcriptome-connectome correlation analysis to identify gene signatures of age-related brain functions. Transcriptome methods could provide unique insights regarding the genetic and epigenetic mechanisms underlying CRCI.
In terms of additional molecular mechanisms, our preclinical studies indicated that chemotherapy treatment leads to significant mitochondrial damage resulting in decreased respiratory activity (27). Hubs are highly connected regions responsible for the majority of information processing and exchange (28, 29). As a result, they require much greater metabolic resources than non-hub regions (30–32). Hubs are believed to play a role in the transmission of neuropathology (33) as well as the maintenance of brain network resilience (34). Our prior studies have examined hub status within the global network but not within local networks (3, 4, 21). Few other studies have examined hub status and these have also focused exclusively on the global network (13, 35).
One of the difficulties in clinical management of CRCI is disentangling cognitive effects from psychological distress and fatigue. Since subjective cognitive outcomes are typically correlated with distress while objective outcomes are not (36), clinicians and researchers often conclude that objective measures reflect neuropathology while subjective reports represent distress. However, there is limited empirical evidence to support this theory. On the contrary, several studies, including our own have shown that self-report measures of cognitive function do correlate with neuroimaging metrics (22, 37–39), suggesting that subjective cognitive impairments may represent a unique phenotype of CRCI. Distress is more often “controlled” statistically in neuroimaging studies of CRCI, leaving gaps in knowledge concerning the neural underpinnings of distress within the context of CRCI.
In this study we aimed to compare local functional brain connectivity in patients seen pre-treatment with those seen post-treatment. This included determining the hub status of local connectivity differences and examining local connectivity profiles associated with subjective cognitive function and psychological distress.
Methods
Participants
In this retrospective study, we examined neuroimaging and self-rating questionnaire data that was commonly acquired across all participants. These included 76 newly diagnosed female patients with breast cancer and 80 female breast cancer survivors. Participants were age 43-81 years, newly diagnosed patients were evaluated prior to primary cancer treatment (surgery with general anesthesia, chemotherapy, radiation) and survivors were evaluated at least 6 months post primary cancer treatment (Table 1) in order to allow for medical stabilization and to focus on patients who may have longer-term, more persistent cognitive deficits. Newly diagnosed patients (PRE-TX group) were assessed between 2012 and 2020 and survivors (POST-TX group) were assessed between 2008 and 2016, all at a single university. This study was approved by the Stanford University Institutional Review Board, was conducted in accordance with the ethical standards of the Declaration of Helsinki and all participants provided written informed consent.
Self-Report Data
Self-ratings of psychological function were obtained using the Total Score of the Clinical Assessment of Depression (CAD), which measures, depression, anxiety and fatigue (40). Subjective executive function was measured with the Global Executive Score of the Behavioral Rating Inventory of Executive Function-Adult Version (BRIEF-A) (41). Raw scores were converted to T scores (mean = 50 ± 10) based on the published normative data for each test. The clinical cutoff score for BRIEF-A is 65 (41) and is 69 for CAD (40). Participants also completed objective cognitive testing but these measures were different for PRE vs. POST participants and therefore could not be combined.
Neuroimaging Acquisitions
Functional magnetic resonance imaging (fMRI) data were obtained while participants rested with eyes closed using a T2* weighted (42) gradient echo spiral pulse sequence: TR = 2,000 ms, TE = 30 ms, flip angle = 80° and 1 interleave, FOV = 22 cm, matrix = 64 x 64, in-plane resolution = 3.4375, number of volumes = 216, oblique prescription with a 3T GE Signa HDx whole body scanner (GE Medical Systems, Milwaukee, WI). A high-order shimming method was employed to reduce field heterogeneity. A high-resolution, 3D IR prepared FSPGR scan was also acquired and used for spatial normalization of fMRI: TR: 8.5, TE: minimum, flip: 15°, TI: 400 ms, BW: ±31.25 kHz, FOV: 22 cm, Phase FOV: 0.75, slice thickness: 1.5 mm, 124 slices, 256 x 256 @ 1 NEX, scan time: 4:33. Data were visually inspected for quality.
Neuroimaging Preprocessing
Resting state fMRI were preprocessed with Statistical Parametric Mapping 12 and CONN Toolboxes (43, 44) implemented in Matlab v2019b (Mathworks, Inc, Natick, MA). Briefly, this involved realignment, coregistration with the segmented anatomic volume, spatial normalization, and artifact detection followed by band-pass filtering (0.008-0.09 Hz). The CompCor correction method was used to reduce physiological and other non-neuronal noise artifacts (45). Motion parameters from realignment were included as regressors and images identified as motion or signal outliers using Artifact Detection Tools (46) were excluded (global signal = 3.0 standard deviations, motion = 1.0 mm, rotation = 0.05 mm). We determined a priori that participants with more than 10% outlier volumes would be excluded from the analysis though none met this threshold. Temporal correlations between all possible pairs of 90 cortical and subcortical bilateral regions (47) were computed based on the mean corrected fMRI signal. This resulted in a 90 x 90 functional connectome matrix for each participant where regions were defined as nodes and the temporal correlations between nodes were defined as edges (48).
Statistical Analyses
We compared BRIEF-A and CAD scores between groups using two-tailed Wilcoxon rank-sum tests given inhomogeneity of variance. We also examined the difference in frequency of clinically significant scores (based on published cutoff scores) using a two-tailed z test for proportions.
We compared local connectivity within connectomes using the Network-based Statistic (NBS) Toolbox v1.2 (49), which identifies connected substructures, or components, within the larger network, similar to the cluster-based thresholding approach used in traditional voxel-wise neuroimaging analyses (49). Permutation testing with 5,000 permutations was then used to determine edge differences in components between the groups, controlling for multiple comparisons using family-wise error (FWE) and covarying for age. NBS results were visualized using BrainNet Viewer (50).
Brain regions identified as having significant NBS differences between groups were then evaluated for network hub status based on degree, betweenness centrality and/or clustering coefficient > 1 standard deviation above network mean (51). Hubs were further classified as provincial or connector type based on module participation coefficient. First, modularity analysis was conducted at minimum connection density across groups, which refers to the highest threshold where all nodes remain connected (no isolated nodes) (52). Modularity was calculated using the method described by Newman (53) and module decomposition was visually inspected for quality assurance. Hubs with module participation coefficient P < 0.3 were classified as provincial hubs, and hubs with P > 0.3 were classified as connector hubs (51). Hub results were visualized using BrainNet Viewer (50).
To explore relationships between connectome edges and self-rating scores, we first calculated the edge betweenness centrality for each edge identified as significantly different between groups by NBS. Betweenness centrality indicates the importance of each edge for the network's integration (54). We calculated centrality at the minimum connection density across groups. We then correlated the centralities with BRIEF-A and CAD scores using Spearman two-tailed correlations (p < 0.05) in the POST-TX group only given that BRIEF-A scores were significantly higher in this group (indicating greater executive dysfunction). Given that these were exploratory correlations, we did not correct for multiple comparisons. We also correlated BRIEF-A and CAD scores within the POST-TX group.
Results
The POST-TX group demonstrated significantly greater executive dysfunction as measured by the BRIEF-A (W = 3,856, p = 0.004) but the groups did not differ in terms of CAD scores (W = 2,866, p = 0.627, Table 1). Further, there were 30 participants in the POST-TX group (38%) who had clinically significant BRIEF-A scores compared to 0 (0%) in the PRE-TX group, which was a significant difference (z = 5.94, p < 0.001). There were 7 (9%) participants in the POST-TX group with clinically significant CAD scores compared to 4 (5%) in the PRE-TX group. This was not a significant difference (z = 1.02, p = 0.154).
As shown in Figure 1, NBS revealed that two main network components were significantly different between the groups. Component 1 was hypo-connected in the POST-TX group and consisted of edges connecting left supramarginal gyrus with right insula and right Rolandic operculum, right supramarginal gyrus with bilateral insula, right superior temporal pole with left Rolandic operculum and bilateral supramarginal gyrus, and right superior temporal gyrus with right Heschl's gyrus, left Rolandic operculum and left superior temporal gyrus (10 edges). The t statistics for these edges ranged from 3.11 to 4.22 (p < 0.042, FWE corrected, Table 2).
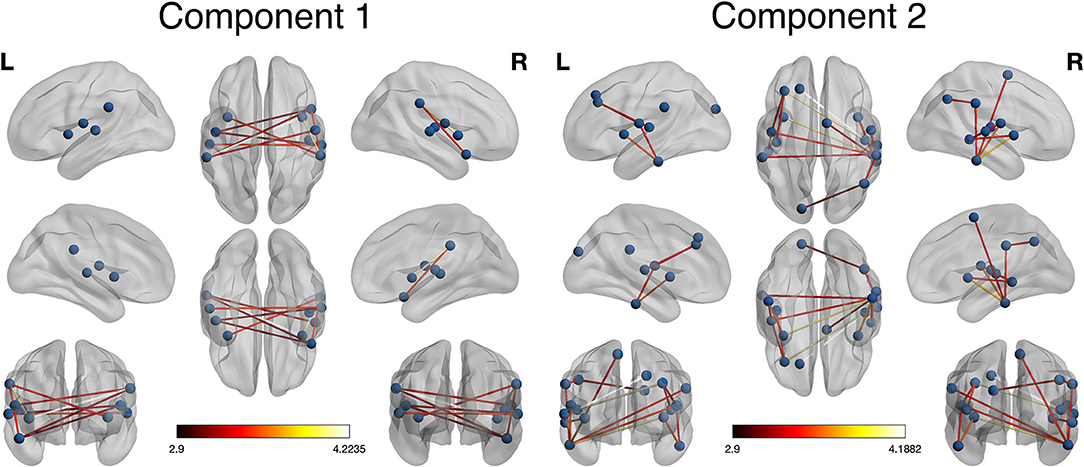
Figure 1. Network-based statistic (NBS) visualization. Two components were identified by NBS that differed significantly between groups. Component 1 included connections among lateral temporal and parietal regions. These connections were hypo-connected in the POST-TX compared to the PRE-TX group (colorbar indicates t statistic, p = 0.042, FWE corrected). Component 2 included connections in the frontal, temporal, parietal, and occipital regions. These connections were hyper-connected in the POST-TX group (colorbar indicates t statistic, p = 0.006, FWE corrected).
Component 2 was hyper-connected in the POST-TX group and included edges connecting left Heschl's gyrus with left middle and superior frontal gyri, right Heschl's with left middle frontal gyrus, left superior occipital gyrus with right angular gyrus, left Rolandic operculum with left middle frontal gyrus, right supramarginal gyrus with right angular gyrus, left inferior temporal gyrus with left insula and left Rolandic operculum, right inferior temporal gyrus with bilateral insula, bilateral Rolandic operculum, right supplementary motor area and bilateral supramarginal gyri, right middle temporal gyrus with right insula, right Rolandic operculum and right superior temporal gyrus with right middle temporal gyrus (18 edges). The t statistics ranged from 3.11 to 4.19 (p = 0.006, FWE corrected, Table 2).
Most (78%) of the nodes that these altered edges connected were classified as hubs and 52% of these hubs were connector type, connecting to other functional subnetworks, in the PRE-TX group compared to 30% in the POST-TX group (Figure 2) (79). This difference was significant (z = 1.85, p = 0.031).
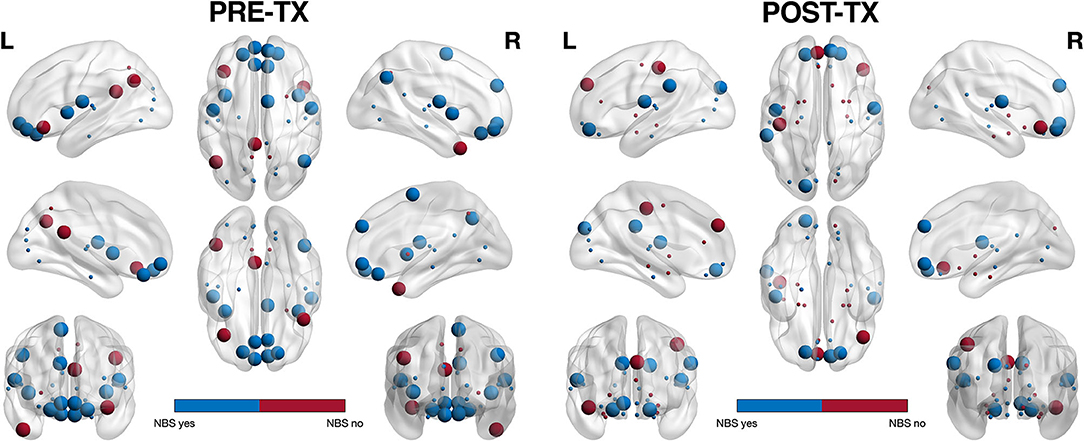
Figure 2. Hub profile visualization. Of the regions connected by NBS significant edges (shown in blue), 78% were classified as hubs. In the PRE-TX group, 52% of hub regions were connector (larger spheres) vs. provincial (smaller spheres) whereas in the POST-TX group, 70% were provincial. This difference was significant (z = 1.85, p = 0.031).
BRIEF-A and CAD scores were significantly correlated in the POST-TX group (r = 0.52, p < 0.001). Centralities for the significant NBS edges were not correlated with BRIEF-A or CAD scores in the POST-TX group. However, given that brain function tends to be non-linear and hierarchical, we further explored correlations among all edges for the significant NBS matrices (i.e., alternate connections between nodes encompassing significant NBS edges). For component 1, CAD correlated with five edges (p < 0.050, uncorrected) and BRIEF-A with three edges (p < 0.041, uncorrected) and two of these edges overlapped (i.e., were correlated with both scores). Component 2 showed the opposite profile with five edges correlating with BRIEF-A (p < 0.043, uncorrected) and two with CAD (p < 0.022, uncorrected) with no overlap (Figure 3).
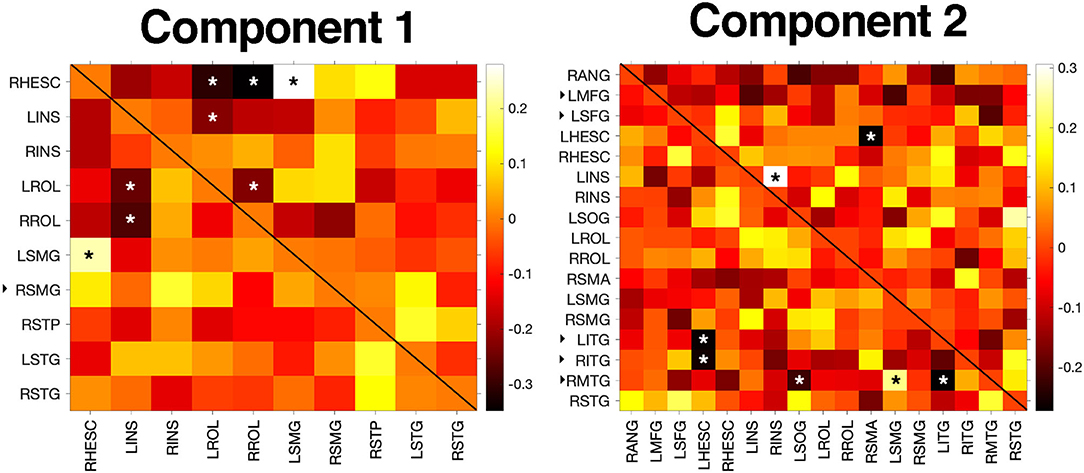
Figure 3. Correlation results. BRIEF-A and CAD scores were not correlated with centralities of significant NBS edges but were associated with centralities of several alternate edges connecting significant NBS nodes (p < 0.05). In the heatmaps, significant correlations with CAD are denoted with an asterisk in the upper triangle while correlations with BRIEF-A are denoted in the lower triangle. Colorbar indicates correlation coefficient. The black, right facing triangle next to a node label indicates a non-hub. All other nodes were hubs. L/RHESC, right Heschl's gyrus; L/RINS, left/right insula; L/RROL, left/right Rolandic operculum; L/RSMG, left/right supramarginal gyrus; RSTP, right superior temporal pole; L/RSTG, left/right superior temporal gyrus; RANG, right angular gyrus; LMFG, left middle frontal gyrus; LSFG, left superior frontal gyrus; LSOG, left superior occipital gyrus; RSMA, right supplementary motor area; L/RITG, left/right inferior temporal gyrus; RMTG, right middle temporal gyrus; RSTG, right superior temporal gyrus.
Discussion
Compared to the PRE-TX group, POST-TX breast cancer survivors demonstrated significantly altered local functional brain network connectivity. Specifically, our findings indicated group differences in the functional connections within temporal regions and among temporal-parietal and frontal-temporal regions. Since this study focused on connectivity, these findings suggest that information exchange between these regions may be altered following breast cancer treatment. Connections between temporal pole and supramarginal gyrus, as well as those between temporal pole and insula have been shown to be associated with word comprehension (55). Word comprehension per se has not been studied among breast cancer survivors, although potentially related problems such as difficulties with reading have been reported (56).
Inferior temporal gyrus is connected to supramarginal and angular gyri by inferior longitudinal fasciculus (ILF) and arcuate fasciculus (AF). AF connects frontal, parietal and temporal areas and is believed to be involved in language, praxis and visual spatial processing (57). ILF also connects to hippocampus, amygdala and cingulate to subserve vision, memory, emotion and language including the ventral-semantic stream (57). Insula connections with other temporal areas, including Heschl's gyrus, tend to be involved in cognition, emotion and interoception (58). Left middle and left superior frontal connections with left Rolandic operculum, left superior occipital, right superior temporal and right middle temporal regions likely involve attention and executive function integration with verbal memory, selective visual attention and somatosensory processing (59). Taken together, these findings may reflect the deficits in verbal memory, verbal fluency, attention, executive function and emotion regulation that are frequently observed among breast cancer patients and survivors (60–62).
Our findings may help direct future studies involving predictive modeling. A critical aspect of CRCI management is determining which patients are at highest risk. Neuroimaging data tend to be uniquely accurate in predicting future clinical and behavioral outcomes (63) but involve high dimensionality. Depending on the image resolution and parcellation scheme, neuroimaging data can provide potential features numbering in the tens of thousands or more. This is impractical for many clinical studies involving modest sample sizes. Therefore, findings such as ours can provide a smaller set of potential features for training predictive models. Similarly, a limited set of regions of interest would make interrogating cortical gene expression profiles more feasible, especially in a smaller sample (64).
Such studies involve examining the spatial correlations between brain-wide messenger RNA expression and regional neuroimaging metrics (25). Although many studies have demonstrated that primary breast cancer and/or its treatments significantly alter brain function and structure, it remains unclear how or why. Several studies, including our own, suggest genetic and/or epigenetic risk factors may play an important role (9, 39, 65–69). However, these findings have been limited to the candidate variants selected and have shown some inconsistent results, which may relate to what brain regions were examined. Regions of altered connectivity associated with CRCI could be correlated with an existing transcriptional atlas, such as the Allen Human Brain Atlas (70), to determine the gene expression profiles of those regions. This would provide more specific insights regarding the molecular mechanisms underlying altered connectivity profiles compared to the candidate genetic variant studies conducted thus far.
Most of the nodes that encompassed the altered edges in the POST-TX group were hub regions. This is consistent with prior literature suggesting that hub areas of the brain tend to be more vulnerable to injury and disease (32). Hubs have high activity and metabolism and are therefore believed to play a critical role in neurodegeneration. For example, amyloid-beta deposition in Alzheimer's disease has been shown to be associated with cortical hub connectivity (71). We have previously shown that connectivity of default mode network hubs can accurately distinguish chemotherapy treated breast cancer survivors from chemotherapy naïve survivors and healthy controls, suggesting that hubs may be preferentially vulnerable to chemotherapy (38). Our present results further indicate hub vulnerability across different subnetworks. Specifically, the hubs identified here are members of various functional subnetworks including default mode but also dorsal-attention and salience networks (72).
Further, the POST-TX group had significantly fewer connector hubs compared to the PRE-TX group. Connector hubs connect functional subnetworks to each other and are thus important for integrating information processing across the brain. Provincial hubs have higher intra-subnetwork connectivity and are therefore more important for local information exchange, or segregation (51). Lesioning of connector hubs tends to have a more widespread effect on brain network organization and efficiency (51, 73). Therefore, a greater proportion of provincial hubs following breast cancer therapy may reflect reorganization of neural resources to protect local processing in response to a reduction in global integration. Since cognitive domains such as executive function rely on interactions among various, anatomically distant regions, this may help explain the greater executive dysfunction endorsed by the POST-TX group. In fact, BRIEF-A scores were significantly correlated with the centralities of several edges and all of these edges connected one or more hubs (Figure 3).
BRIEF-A and CAD scores were significantly correlated with each other in the POST-TX group, consistent with prior reports (36, 74). However, BRIEF-A scores indicated significantly higher subjective executive dysfunction in the POST-TX group despite no significant difference in psychological distress. These results suggest that self-reported cognitive dysfunction cannot be sufficiently explained by distress. BRIEF-A and CAD scores did not correlate with the significant NBS edges. However, the non-linear nature of brain function (75, 76) in combination with potential brain network reorganization following injury can result in indirect effects of altered connectivity on clinical and behavioral outcomes. Accordingly, we found that centralities for alternate edges in the same component matrices were correlated with both BRIEF-A and CAD scores in the POST-TX group. Interestingly, there was limited overlap in these correlations. These results suggest that psychological distress and subjective cognitive dysfunction may have unique neurophenotypes.
However, correlations were exploratory and not corrected for multiple comparisons. Therefore, these findings should be interpreted with caution and further research regarding the neural correlates of subjective cognitive function vs. distress is warranted.
The present study is not without limitations including the cross-sectional design. Consistent with other such studies in this field, the POST-TX group was heterogenous for treatment regimen and there were not sufficient subsample sizes to examine effects of individual treatments. While longitudinal studies are optimal for examining individual cognitive trajectories and evaluating the effects of specific treatments, “broad” studies with larger samples are useful for identifying neuropsychiatric phenotypes (8, 77). Our study was also limited by its retrospective nature in terms of data availability. Objective cognitive function may have distinct neural correlates from those of subjective cognitive function. We did not have sufficient overlap in objective testing batteries for our retrospective cohorts to evaluate these effects. However, research regarding the neural correlates of subjective cognitive impairment has been very limited to date and therefore our findings make a significant contribution to this literature. The BRIEF-A measures executive function, which is very commonly affected by breast cancer and its therapies but the ability to assess other subjective cognitive domains would have strengthened our results. There was large variability in time since primary treatment completion of participants in the POST-TX group and a majority were undergoing hormone blockade therapy during this interval. It is possible that network structure changes over time across the post-treatment phase. Also, the rate of recovery after treatment could potentially alter hub status as hub profiles are dynamic. Therefore, assessment at multiple time points following breast cancer treatment could provide additional insights (21, 78).
Despite these limitations, our results provide further support that breast cancer and/or its treatments are associated with brain network alterations. This work provides further insights regarding the neural correlates of common cognitive-behavioral deficits observed in breast cancer survivors. These results could potentially inform predictive modeling applications and/or transcriptome studies, among others. Our findings also uniquely add to the growing body of work that patient reported outcomes are linked to measurable biomarkers. Our results suggest that self-reported CRCI and psychological symptoms may have distinct neurophenotypes, further indicating that CRCI and distress can co-occur and be jointly caused by post-treatment brain injury. This evidence includes guidance for future investigations of and potential interventions for subjective CRCI.
Data Availability Statement
The raw data supporting the conclusions of this article will be made available by the authors, without undue reservation.
Ethics Statement
The studies involving human participants were reviewed and approved by the Stanford University Institutional Review Board. The patients/participants provided their written informed consent to participate in this study.
Author Contributions
SRK and OP designed and conducted the study. SRK conducted data analyses. SRK, TT, and AMH wrote the manuscript. SRK, MW, MWG, and OP reviewed/edited the manuscript. All authors contributed to the article and approved the submitted version.
Funding
This research was supported by funding from the National Institutes of Health (R01CA226090 and R01CA172145 to SRK/OP and K01NR018970 to AMH).
Conflict of Interest
The authors declare that the research was conducted in the absence of any commercial or financial relationships that could be construed as a potential conflict of interest.
Publisher's Note
All claims expressed in this article are solely those of the authors and do not necessarily represent those of their affiliated organizations, or those of the publisher, the editors and the reviewers. Any product that may be evaluated in this article, or claim that may be made by its manufacturer, is not guaranteed or endorsed by the publisher.
Acknowledgments
The authors wish to thank the staff and faculty of the Richard M. Lucas Center at Stanford University for assistance with neuroimaging protocols.
References
1. Janelsins MC, Kesler SR, Ahles TA, Morrow GR. Prevalence, mechanisms, and management of cancer-related cognitive impairment. Int Rev Psychiatry. (2014) 26:102–13. doi: 10.3109/09540261.2013.864260
2. Mayo SJ, Lustberg M, H MD, Nakamura ZM, Allen DH, Von Ah D, et al. Cancer-related cognitive impairment in patients with non-central nervous system malignancies: an overview for oncology providers from the MASCC Neurological Complications Study Group. Support Care Cancer. (2020) 29:2821-40. doi: 10.1007/s00520-020-05860-9
3. Bruno J, Hosseini SM, Kesler S. Altered resting state functional brain network topology in chemotherapy-treated breast cancer survivors. Neurobiol Dis. (2012) 48:329–38. doi: 10.1016/j.nbd.2012.07.009
4. Hosseini SM, Koovakkattu D, Kesler SR. Altered small-world properties of gray matter networks in breast cancer. BMC Neurol. (2012) 12:28. doi: 10.1186/1471-2377-12-28
5. Kesler SR, Watson CL, Blayney DW. Brain network alterations and vulnerability to simulated neurodegeneration in breast cancer. Neurobiol Aging. (2015) 36:2429–42. doi: 10.1016/j.neurobiolaging.2015.04.015
6. Kesler SR, Blayney DW. Neurotoxic effects of anthracycline- vs nonanthracycline-based chemotherapy on cognition in breast cancer survivors. JAMA Oncol. (2016) 2:185–92. doi: 10.1001/jamaoncol.2015.4333
7. Kesler SR, Rao V, Ray WJ, Rao A, Alzheimer's Disease Neuroimaging Initiative. Probability of Alzheimer's disease in breast cancer survivors based on gray-matter structural network efficiency. Alzheimers Dement. (2017) 9:67–75. doi: 10.1016/j.dadm.2017.10.002
8. Kesler SR, Petersen ML, Rao V, Harrison RA, Palesh O. Functional connectome biotypes of chemotherapy-related cognitive impairment. J Cancer Surviv. (2020) 14:483–93. doi: 10.1007/s11764-020-00863-1
9. Harrison RA, Rao V, Kesler SR. The association of genetic polymorphisms with neuroconnectivity in breast cancer patients. Sci Rep. (2021) 11:6169. doi: 10.1038/s41598-021-85768-4
10. Chen X, He X, Tao L, Li J, Wu J, Zhu C, et al. The working memory and dorsolateral prefrontal-hippocampal functional connectivity changes in long-term survival breast cancer patients treated with tamoxifen. Int J Neuropsychopharmacol. (2017) 20:374–82. doi: 10.1093/ijnp/pyx008
11. Cheng H, Li W, Gong L, Xuan H, Huang Z, Zhao H, et al. Altered resting-state hippocampal functional networks associated with chemotherapy-induced prospective memory impairment in breast cancer survivors. Sci Rep. (2017) 7:45135. doi: 10.1038/srep45135
12. Mo C, Lin H, Fu F, Lin L, Zhang J, Huang M, et al. Chemotherapy-induced changes of cerebral activity in resting-state functional magnetic resonance imaging and cerebral white matter in diffusion tensor imaging. Oncotarget. (2017) 8:81273–84. doi: 10.18632/oncotarget.18111
13. Xuan H, Gan C, Li W, Huang Z, Wang L, Jia Q, et al. Altered network efficiency of functional brain networks in patients with breast cancer after chemotherapy. Oncotarget. (2017) 8:105648. doi: 10.18632/oncotarget.22358
14. Apple AC, Schroeder MP, Ryals AJ, Wagner LI, Cella D, Shih PA, et al. Hippocampal functional connectivity is related to self-reported cognitive concerns in breast cancer patients undergoing adjuvant therapy. Neuroimage Clin. (2018) 20:110–8. doi: 10.1016/j.nicl.2018.07.010
15. Li TY, Chen VC, Yeh DC, Huang SL, Chen CN, Chai JW, et al. Investigation of chemotherapy-induced brain structural alterations in breast cancer patients with generalized q-sampling MRI and graph theoretical analysis. BMC Cancer. (2018) 18:1211. doi: 10.1186/s12885-018-5113-z
16. Chen BT, Jin T, Patel SK, Ye N, Ma H, Wong CW, et al. Intrinsic brain activity changes associated with adjuvant chemotherapy in older women with breast cancer: a pilot longitudinal study. Breast Cancer Res Treat. (2019) 176:181-9. doi: 10.1007/s10549-019-05230-y
17. Feng Y, Tuluhong D, Shi Z, Zheng LJ, Chen T, Lu GM, et al. Postchemotherapy hippocampal functional connectivity patterns in patients with breast cancer: a longitudinal resting state functional MR imaging study. Brain Imaging Behav. (2019) 14:1456-67. doi: 10.1007/s11682-019-00067-x
18. Shen CY, Chen VC, Yeh DC, Huang SL, Zhang XR, Chai JW, et al. Association of functional dorsal attention network alterations with breast cancer and chemotherapy. Sci Rep. (2019) 9:104. doi: 10.1038/s41598-018-36380-6
19. Chen VC, Lin KY, Tsai YH, Weng JC. Connectome analysis of brain functional network alterations in breast cancer survivors with and without chemotherapy. PLoS ONE. (2020) 15:e0232548. doi: 10.1371/journal.pone.0232548
20. Bekele BM, Luijendijk M, Schagen SB, De Ruiter M, Douw L. Fatigue and resting-state functional brain networks in breast cancer patients treated with chemotherapy. Breast Cancer Res Treat. (2021) 89:787–96. doi: 10.1007/s10549-021-06326-0
21. Kesler SR, Rao A, Blayney DW, Oakley-Girvan IA, Karuturi M, Palesh O. Predicting long-term cognitive outcome following breast cancer with pre-treatment resting state fMRI and random forest machine learning. Front Hum Neurosci. (2017) 11:555. doi: 10.3389/fnhum.2017.00555
22. Henneghan AM, Gibbons C, Harrison RA, Edwards ML, Rao V, Blayney DW, et al. Predicting patient reported outcomes of cognitive function using connectome-based predictive modeling in breast cancer. Brain Topogr. (2020) 33:135–42. doi: 10.1007/s10548-019-00746-4
23. Arnatkeviciute A, Fulcher BD, Fornito A. A practical guide to linking brain-wide gene expression and neuroimaging data. Neuroimage. (2019) 189:353–67. doi: 10.1016/j.neuroimage.2019.01.011
24. Fornito A, Arnatkeviciute A, Fulcher BD. Bridging the gap between connectome and transcriptome. Trends Cogn Sci. (2019) 23:34–50. doi: 10.1016/j.tics.2018.10.005
25. Selvaggi P, Rizzo G, Mehta MA, Turkheimer FE, Veronese M. Integration of human whole-brain transcriptome and neuroimaging data: practical considerations of current available methods. J Neurosci Methods. (2021) 355:109128. doi: 10.1016/j.jneumeth.2021.109128
26. Zhao X, Chen J, Xiao P, Feng J, Nie Q, Zhao X, et al. Identifying age-specific gene signatures of the human cerebral cortex with joint analysis of transcriptomes and functional connectomes. Brief Bioinform. (2020) 22:1-12. doi: 10.1093/bib/bbaa388
27. Manchon J, Uzor N, Kesler S, Weffeel J, Townley A, Pradeep S, et al. TFEB ameliorates the impairment of the autophagy-lysosome pathway in neurons induced by doxorubicin. Aging. (2016) 8:3507–19. doi: 10.18632/aging.101144
28. Guimera R, Amaral LA. Cartography of complex networks: modules and universal roles. J Stat Mech. (2005) 2005:nihpa35573. doi: 10.1088/1742-5468/2005/02/P02001
29. Sporns O, Betzel RF. Modular brain networks. Annu Rev Psychol. (2016) 67:613–40. doi: 10.1146/annurev-psych-122414-033634
30. Albert R, Jeong H, Barabasi A-L. Error and attack tolerance of complex networks. Nature. (2000) 406:378–82. doi: 10.1038/35019019
31. Lord LD, Expert P, Huckins JF, Turkheimer FE. Cerebral energy metabolism and the brain's functional network architecture: an integrative review. J Cereb Blood Flow Metab. (2013) 33:1347–54. doi: 10.1038/jcbfm.2013.94
32. Crossley NA, Mechelli A, Scott J, Carletti F, Fox PT, Mcguire P, et al. The hubs of the human connectome are generally implicated in the anatomy of brain disorders. Brain. (2014) 137:2382–95. doi: 10.1093/brain/awu132
33. Zhou J, Gennatas ED, Kramer JH, Miller BL, Seeley WW. Predicting regional neurodegeneration from the healthy brain functional connectome. Neuron. (2012) 73:1216–27. doi: 10.1016/j.neuron.2012.03.004
34. Achard S, Salvador R, Whitcher B, Suckling J, Bullmore E. A resilient, low-frequency, small-world human brain functional network with highly connected association cortical hubs. J Neurosci. (2006) 26:63–72. doi: 10.1523/JNEUROSCI.3874-05.2006
35. Zeng Y, Cheng ASK, Song T, Sheng X, Cheng H, Qiu Y, et al. Changes in functional brain networks and neurocognitive function in Chinese gynecological cancer patients after chemotherapy: a prospective longitudinal study. BMC Cancer. (2019) 19:386. doi: 10.1186/s12885-019-5576-6
36. Bray VJ, Dhillon HM, Vardy JL. Systematic review of self-reported cognitive function in cancer patients following chemotherapy treatment. J Cancer Surviv. (2018) 12:537-59. doi: 10.1007/s11764-018-0692-x
37. Kesler SR, Kent JS, O'hara R. Prefrontal cortex and executive function impairments in primary breast cancer. JAMA Neurol. (2011) 68:1447–53. doi: 10.1001/archneurol.2011.245
38. Kesler SR, Wayfull JS, Hosseini SM, Cheung M, Watson CL, Hoeft F. Default mode network connectivity distinguishes chemotherapy-treated breast cancer survivors from controls. Proc Natl Acad Sci USA. (2013) 110:11600–5. doi: 10.1073/pnas.1214551110
39. Mcdonald BC, Conroy SK, Smith DJ, West JD, Saykin AJ. Frontal gray matter reduction after breast cancer chemotherapy and association with executive symptoms: a replication and extension study. Brain Behav Immun. (2013) 30(Suppl 0):S117–25. doi: 10.1016/j.bbi.2012.05.007
40. Aghakhani A, Chan EK, Test reviews: Bracken BA, Howell K. (2004). Clinical assessment of depression. Odessa, FL: Psychological Assessment Resources. J Psychoeduc Assess. (2007) 25:416–22. doi: 10.1177/0734282907300383
41. Roth RM, Lance CE, Isquith PK, Fischer AS, Giancola PR. Confirmatory factor analysis of the behavior rating inventory of executive function-adult version in healthy adults and application to attention-deficit/hyperactivity disorder. Arch Clin Neuropsychol. (2013) 28:425–34. doi: 10.1093/arclin/act031
42. Glover GH, Lai S. Self-navigated spiral fMRI: interleaved versus single-shot. Magn Reson Med. (1998) 39:361–8. doi: 10.1002/mrm.1910390305
43. Ashburner J. SPM: a history. Neuroimage. (2012) 62:791–800. doi: 10.1016/j.neuroimage.2011.10.025
44. Whitfield-Gabrieli S, Nieto-Castanon A. Conn: a functional connectivity toolbox for correlated and anticorrelated brain networks. Brain Connect. (2012) 2:125–41. doi: 10.1089/brain.2012.0073
45. Behzadi Y, Restom K, Liau J, Liu TT. A component based noise correction method (CompCor) for BOLD and perfusion based fMRI. NeuroImage. (2007) 37:90–101. doi: 10.1016/j.neuroimage.2007.04.042
46. Chai XJ, Ofen N, Gabrieli JD, Whitfield-Gabrieli S. Selective development of anticorrelated networks in the intrinsic functional organization of the human brain. J Cogn Neurosci. (2014). 26:501–13. doi: 10.1162/jocn_a_00517
47. Tzourio-Mazoyer N, Landeau B, Papathanassiou D, Crivello F, Etard O, Delcroix N, et al. Automated anatomical labeling of activations in SPM using a macroscopic anatomical parcellation of the MNI MRI single-subject brain. Neuroimage. (2002) 15:273–89. doi: 10.1006/nimg.2001.0978
48. Bassett DS, Bullmore ET. Small-world brain networks revisited. Neuroscientist. (2017) 23:499–516. doi: 10.1177/1073858416667720
49. Zalesky A, Fornito A, Bullmore ET. Network-based statistic: identifying differences in brain networks. Neuroimage. (2010) 53:1197–207. doi: 10.1016/j.neuroimage.2010.06.041
50. Xia M, Wang J, He Y. BrainNet viewer: a network visualization tool for human brain connectomics. PLoS ONE. (2013) 8:e68910. doi: 10.1371/journal.pone.0068910
51. Sporns O, Honey CJ, Kotter R. Identification and classification of hubs in brain networks. PLoS ONE. (2007) 2:e1049. doi: 10.1371/journal.pone.0001049
52. Hosseini SM, Hoeft F, Kesler SR. GAT: a graph-theoretical analysis toolbox for analyzing between-group differences in large-scale structural and functional brain networks. PLoS ONE. (2012) 7:e40709. doi: 10.1371/journal.pone.0040709
53. Newman ME. Modularity and community structure in networks. Proc Natl Acad Sci USA. (2006) 103:8577–82. doi: 10.1073/pnas.0601602103
54. Kaiser M. A tutorial in connectome analysis: topological and spatial features of brain networks. Neuroimage. (2011) 57:892–907. doi: 10.1016/j.neuroimage.2011.05.025
55. Bonilha L, Hillis AE, Hickok G, Den Ouden DB, Rorden C, Fridriksson J. Temporal lobe networks supporting the comprehension of spoken words. Brain. (2017) 140:2370–80. doi: 10.1093/brain/awx169
56. Downie FP, Mar Fan HG, Houede-Tchen N, Yi Q, Tannock IF. Cognitive function, fatigue, and menopausal symptoms in breast cancer patients receiving adjuvant chemotherapy: evaluation with patient interview after formal assessment. Psychooncology. (2006) 15:921–30. doi: 10.1002/pon.1035
57. Lin YH, Young IM, Conner AK, Glenn CA, Chakraborty AR, Nix CE, et al. Anatomy and white matter connections of the inferior temporal gyrus. World Neurosurg. (2020) 143:e656–66. doi: 10.1016/j.wneu.2020.08.058
58. Nomi JS, Schettini E, Broce I, Dick AS, Uddin LQ. Structural connections of functionally defined human insular subdivisions. Cereb Cortex. (2018) 28:3445–56. doi: 10.1093/cercor/bhx211
59. Briggs RG, Lin YH, Dadario NB, Kim SJ, Young IM, Bai MY, et al. Anatomy and white matter connections of the middle frontal gyrus. World Neurosurg. (2021) 150:e520-9. doi: 10.1016/j.wneu.2021.03.045
60. Syarif H, Waluyo A, Afiyanti Y, Mansyur M. Executive function in breast cancer survivors and the influencing factors. Enferm Clin. (2019) 29(Suppl. 2):280–5. doi: 10.1016/j.enfcli.2019.04.034
61. Muzzatti B, Bomben F, Flaiban C, Piccinin M, Annunziata MA. Quality of life and psychological distress during cancer: a prospective observational study involving young breast cancer female patients. BMC Cancer. (2020) 20:758. doi: 10.1186/s12885-020-07272-8
62. Sousa H, Almeida S, Bessa J, Pereira MG. The developmental trajectory of cancer-related cognitive impairment in breast cancer patients: a systematic review of longitudinal neuroimaging studies. Neuropsychol Rev. (2020) 30:287–309. doi: 10.1007/s11065-020-09441-9
63. Gabrieli JD, Ghosh SS, Whitfield-Gabrieli S. Prediction as a humanitarian and pragmatic contribution from human cognitive neuroscience. Neuron. (2015) 85:11–26. doi: 10.1016/j.neuron.2014.10.047
64. Diez I, Sepulcre J. Neurogenetic profiles delineate large-scale connectivity dynamics of the human brain. Nat Commun. (2018) 9:3876. doi: 10.1038/s41467-018-06346-3
65. Li W, Zhao J, Ding K, Chao HH, Li CR, Cheng H, Shen L. Catechol-O-Methyltransferase Gene Polymorphisms and the Risk of Chemotherapy-Induced Prospective Memory Impairment in Breast Cancer Patients with Varying Tumor Hormonal Receptor Expression. Med Sci Monit. (2020) 26:e923567. doi: 10.12659/MSM.923567
66. Mandelblatt JS, Small BJ, Luta G, Hurria A, Jim H, Mcdonald BC, et al. Cancer-related cognitive outcomes among older breast cancer survivors in the thinking and living with cancer study. J Clin Oncol. (2018) 36:1800140. doi: 10.1200/JCO.18.00140
67. Merriman JD, Sereika SM, Conley YP, Koleck TA, Zhu Y, Phillips ML, et al. Exploratory study of associations between dna repair and oxidative stress gene polymorphisms and cognitive problems reported by postmenopausal women with and without breast cancer. Biol Res Nurs. (2019) 21:50–60. doi: 10.1177/1099800418799964
68. Yao S, Hu Q, Kerns S, Yan L, Onitilo AA, Misleh J, et al. Impact of chemotherapy for breast cancer on leukocyte DNA methylation landscape and cognitive function: a prospective study. Clin Epigenetics. (2019) 11:45. doi: 10.1186/s13148-019-0641-1
69. Yang GS, Mi X, Jackson-Cook CK, Starkweather AR, Lynch Kelly D, Archer KJ, et al. Differential DNA methylation following chemotherapy for breast cancer is associated with lack of memory improvement at one year. Epigenetics. (2020) 15:499–510. doi: 10.1080/15592294.2019.1699695
70. Hawrylycz MJ, Lein ES, Guillozet-Bongaarts AL, Shen EH, Ng L, Miller JA, et al. An anatomically comprehensive atlas of the adult human brain transcriptome. Nature. (2012) 489:391–9. doi: 10.1038/nature11405
71. Buckner RL, Sepulcre J, Talukdar T, Krienen FM, Liu H, Hedden T, et al. Cortical hubs revealed by intrinsic functional connectivity: mapping, assessment of stability, and relation to Alzheimer's disease. J Neurosci. (2009) 29:1860–73. doi: 10.1523/JNEUROSCI.5062-08.2009
72. Buckner RL, Krienen FM, Yeo BT. Opportunities and limitations of intrinsic functional connectivity MRI. Nat Neurosci. (2013) 16:832–7. doi: 10.1038/nn.3423
73. Hart MG, Price SJ, Suckling J. Connectome analysis for pre-operative brain mapping in neurosurgery. Br J Neurosurg. (2016) 30:506–17. doi: 10.1080/02688697.2016.1208809
74. Hutchinson AD, Hosking JR, Kichenadasse G, Mattiske JK, Wilson C. Objective and subjective cognitive impairment following chemotherapy for cancer: a systematic review. Cancer Treat Rev. (2012) 38:926–34. doi: 10.1016/j.ctrv.2012.05.002
75. Rabinovich MI, Muezzinoglu MK. Nonlinear dynamics of the brain: emotion and cognition. Physics-Uspekhi. (2010) 53:357–72. doi: 10.3367/UFNe.0180.201004b.0371
76. Kesler SR, Gugel M, Huston-Warren E, Watson C. Atypical structural connectome organization and cognitive impairment in young survivors of acute lymphoblastic leukemia. Brain Connect. (2016) 6:273–82. doi: 10.1089/brain.2015.0409
77. Cornblath EJ, Lydon-Staley DM, Bassett DS. Harnessing networks and machine learning in neuropsychiatric care. Curr Opin Neurobiol. (2019) 55:32–9. doi: 10.1016/j.conb.2018.12.010
78. Mcdonald BC, Conroy SK, Ahles TA, West JD, Saykin AJ. Alterations in brain activation during working memory processing associated with breast cancer and treatment: a prospective functional magnetic resonance imaging study. J Clin Oncol. (2012) 30:2500–8. doi: 10.1200/JCO.2011.38.5674
Keywords: breast cancer, fMRI, cognition, effective connectivity, functional connectivity, Bayesian network, graph theory
Citation: Kesler SR, Tang T, Henneghan AM, Wright M, Gaber MW and Palesh O (2021) Cross-Sectional Characterization of Local Brain Network Connectivity Pre and Post Breast Cancer Treatment and Distinct Association With Subjective Cognitive and Psychological Function. Front. Neurol. 12:746493. doi: 10.3389/fneur.2021.746493
Received: 23 July 2021; Accepted: 05 October 2021;
Published: 29 October 2021.
Edited by:
Jaymin Upadhyay, Boston Children's Hospital and Harvard Medical School, United StatesReviewed by:
Linda Douw, VU University Amsterdam, NetherlandsBo Gao, Affiliated Hospital of Guizhou Medical University, China
Copyright © 2021 Kesler, Tang, Henneghan, Wright, Gaber and Palesh. This is an open-access article distributed under the terms of the Creative Commons Attribution License (CC BY). The use, distribution or reproduction in other forums is permitted, provided the original author(s) and the copyright owner(s) are credited and that the original publication in this journal is cited, in accordance with accepted academic practice. No use, distribution or reproduction is permitted which does not comply with these terms.
*Correspondence: Shelli R. Kesler, c3JrZXNsZXJAYXVzdGluLnV0ZXhhcy5lZHU=