- 1Baylor College of Medicine, Houston, TX, United States
- 2Carillion Clinic, Roanoke, VA, United States
- 3Department of Emergency Medicine, University of Rochester, Rochester, NY, United States
- 4Renaissance School of Medicine at Stony Brook University, Stony Brook, NY, United States
- 5University of Kansas Medical Center, Kansas City, MO, United States
- 6Integrative Emergency Service/John Peter Smith Health System, Fort Worth, TX, United States
- 7Detroit Medical Center, Detroit, MI, United States
- 8William Beaumont Hospital, Royal Oak, MI, United States
- 9Department of Emergency Medicine, University of Pennsylvania, Philadelphia, PA, United States
Despite an estimated 2.8 million annual ED visits, traumatic brain injury (TBI) is a syndromic diagnosis largely based on report of loss of consciousness, post-traumatic amnesia, and/or confusion, without readily available objective diagnostic tests at the time of presentation, nor an ability to identify a patient's prognosis at the time of injury. The recognition that “mild” forms of TBI and even sub-clinical impacts can result in persistent neuropsychiatric consequences, particularly when repetitive, highlights the need for objective assessments that can complement the clinical diagnosis and provide prognostic information about long-term outcomes. Biomarkers and neurocognitive testing can identify brain injured patients and those likely to have post-concussive symptoms, regardless of imaging testing results, thus providing a physiologic basis for a diagnosis of acute traumatic encephalopathy (ATE). The goal of the HeadSMART II (HEAD injury Serum markers and Multi-modalities for Assessing Response to Trauma) clinical study is to develop an in-vitro diagnostic test for ATE. The BRAINBox TBI Test will be developed in the current clinical study to serve as an aid in evaluation of patients with ATE by incorporating blood protein biomarkers, clinical assessments, and tools to measure, identify, and define associated pathologic evidence and neurocognitive impairments. This protocol proposes to collect data on TBI subjects by a multi-modality approach that includes serum biomarkers, clinical assessments, neurocognitive performance, and neuropsychological characteristics, to determine the accuracy of the BRAINBox TBI test as an aid to the diagnosis of ATE, defined herein, and to objectively determine a patient's risk of developing post-concussive symptoms.
Background
Mild traumatic brain injury (mTBI) annually affects over 23 million people worldwide (1). In 2014, there were an estimated 2.5 million TBI-related emergency department (ED) visits in the US, of which 90% were estimated to be the result of mTBI (2, 3). From 2006 to 2014, the number of TBI-related ED visits increased by 53% (4), although the real incidence is likely much higher, as mTBI is underdiagnosed in the ED (5, 6), patients may present to alternate venues (e.g., primary care), or they do not seek care at all. The majority of adults over age 40 report a history of head injury with loss of consciousness at some point in their lives (4, 7–9).
Over 90% of TBI is classified as “mild” TBI, a term that has been criticized as misleading, since many of these injuries result in significant disabilities (10), especially if they are repetitive (11). Despite a classification of “mild,” TBI can cause persistent cognitive and physical symptoms with major impacts on affected patients function and quality of life (12, 13). In 2010 an estimated 2% of the U.S. population lived with TBI-related disabilities, at an annual estimated expense of about $77 billion (4).
Despite the magnitude of this global public health problem, the diagnosis and classification of mTBI remains challenging. The terms mTBI and concussion (which has no accepted standard definition) continue to be used interchangeably, with a 2014 review reporting 50 different mTBI definitions across 101 studies (14). Although the NINDS have developed TBI common data elements (15), the lack of objective diagnostic or prognostic tools for mTBI severely limits its effectiveness.
To date, few U.S. Food and Drug Administration (FDA) cleared strategies are available to objectively diagnose, or define prognosis, in patients presenting with head injury. While anatomic imaging methods, generally with computerized tomography (CT), show TBI-related abnormalities in ~10% of patients evaluated in EDs, a significant number of patients have disabling TBI symptoms despite initial negative imaging. When neuroimaging is negative, there are few currently available objective tests to identify significant occult injury and predict future dysfunction. Consequently, while acute care physicians can exclude emergencies that require neurosurgical intervention, or neurocritical care (e.g., intracranial hemorrhage) by imaging or physical examination, and they can perform testing to exclude the need for imaging (16), they have limited ability to objectively diagnose mTBI or identify which patients will suffer longer term poor outcomes. In fact, one ED study demonstrated that the ability of physicians to prospectively identify patients who will have subsequent mTBI related symptoms 90 days after their presentation is extremely poor, with a sensitivity of only 8.1% and a specificity of 54.5% (17).
Diagnosis of mTBI in the ED is currently based on clinical findings, which can be problematic, since the medical history is often incomplete, symptoms are vague, and the physical signs are non-specific. These challenges are further confounded by factors such as intoxication or pre-existing neurologic impairments (18). The recent availability of biomarker testing may allow for more precise identification of patients with mTBI, as a manifestation of acute traumatic encephalopathy (ATE), by adding the objective evidence of injury-related leakage of brain-derived proteins into the blood (16). Although ATE testing is currently unavailable in the acute care environment, the ATE cohort is identified as having abnormal biomarkers and/or neurocognitive dysfunction, irrespective of the results of neuroimaging. Ultimately, this strategy may be applied to risk stratify patients by determining their likelihood of developing downstream symptoms caused by their TBI.
In addition to biomarkers, evidence also supports the value of adding neurocognitive (NC) assessments for the characterization of functional deficits at the time of injury (19). The use of NC testing to identify patients at risk for protracted or disabling neurological deficits following head injury, irrespective of an imaging evaluation, may allow the selection of patients who will benefit from targeted interventions that have the potential to improve patient outcomes (20–24).
BRAINBox TBI Test is a novel technology that uses a multi-marker serum panel, in conjunction with computerized neurocognitive testing, to aid in an objective diagnosis of ATE. In addition, results of this testing strategy may identify those at risk for of persistent symptoms, i.e., it provides a prognostic determination. Thus, our purpose is to determine the ability of BRAINBox TBI Test technology to identify patients with ATE, as well as to define their prognosis, in real-world patients presenting with suspected ATE.
Methods
Our primary objectives are to determine the ability of the BRAINBox TBI Test to:
1. Diagnose ATE using statistical modeling methods that combine blood biomarker values, focused patient health information, and NC/NP testing.
2. Predict persistent symptoms up to 90 days after a diagnosis of ATE by using statistical modeling combining biomarkers, focused patient health information, and NC/NP testing.
HeadSMART II (HEAD injury Serum markers and Multi-modalities for Assessing Response to Trauma) is a clinical study that was devised to provide the information for these objectives and to provide clinical validation of the test. This is a multicenter, international, observational study, NCT04423198, with an expectation of enrolling up to 2000 subjects. The study started Q1 2021, with enrollment anticipated to be completed by the end of 2022. Subjects include those with suspected ATE, and control populations. Inclusion and exclusion criteria are provided in Table 1. Data compliant with Common Data Elements; Modular Data Elements for Traumatic Brain Injury (25), as well as ICD codes, will be collected at the index visit (t = 0), and at 14-, 30- and 90-days post-injury in suspected ATE patients, at the index visit and at 14 days for trauma control patients, and only at the index visit for healthy control subjects. All participants will provide 2 blood draws (separated by 1–4 h) and NC assessments at enrollment, and with added symptom specific assessments and neuropsychological testing (patient reported outcomes, PROs) at their follow up visits. Blood draws will consist of obtaining serum, plasma (including optional collection for DNA analysis) and PAXGene tubes, which are processed according to manufacturer's recommended protocols, then stored at −80C, and shipped to a core lab for biomarker analysis. Biomarker analysis will use BRAINBox's proprietary serum/plasma biomarker assays including, but not limited to, GFAP (Glial Fibrillary Acidic Protein), NSE (Neuron Specific Enolase-2), NRGN (Neurogranin), SNCB (Beta-Synuclein), and MT3 (Metallothionein-3). These biomarkers were chosen due to their demonstrated diagnostic and prognostic utility for TBI in the HEADSMART pilot trial (26–30).
The blood biomarker values are quantified by comparing the raw signal detected from replicates to a calibrant curve that covers a concentration range greater than the clinical range of the samples tested. The replicates are evaluated for specifications including coefficient of variation that determine the technical validity of the test. All samples passing acceptance criteria are reported as a quantity (amount per unit volume, e.g., picograms/milliliter). The biomarker values will be used as predictors in the statistical/machine learning algorithms.
The BrainCheck Application will be used to provide digitized neurocognitive assessments at each visit, the details of which are given in Table 2. BrainCheck uses digitized versions of several previously validated neurocognitive pen and paper tests, each with associated metrics of function such as duration and accuracy of responses. These comprise a composite battery and that also includes measures of balance and coordination, functions that can be affected by acute brain injury. For each subject, the data are compared against a normative/reference database previously developed by BrainCheck. The normalized values will also be used as predictors in the statistical/machine learning algorithms.
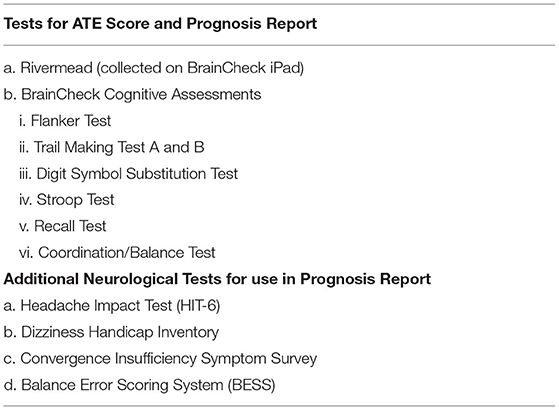
Table 2. Neuropsychiatric/neurocognitive testing (19).
The clinical data are collected through electronic CRFs and stored in REDCap Cloud Electronic Data Capture software. The NINDS common data elements for general reporting and for traumatic brain injury have been used to guide the design of the study and the CRFs.
Subjects participate in the study for up to 90 days, must enroll within 96 h of blunt head trauma, and have a Glasgow Coma Scale ≥13 at presentation. Up to 1,600 suspected ATE subjects will be enrolled through the study time points to provide sufficient subjects for separate algorithm training and validation cohorts. The demographic breakdown of the subjects will meet good clinical practice guidelines (31). For the determination of prognosis, subjects are first adjudicated as positive for TBI by a diagnostic adjudication committee, who provide an expert clinical diagnosis after reviewing the complete patient history and assessment up to 1-month post-injury. Diagnosed TBI subjects will be further categorized by the presence of symptoms at each study time point.
The Diagnostic Adjudication Committee (DAC) will consist of a panel of physicians experienced in the diagnosis and management of TBI. Two adjudicators, blinded to BRAINBox TBI Test results, will be randomly selected to review each subject's de-identified medical records, physical examination notes, neurological assessments (see Table 2), and core lab neuroimaging report, to define an expert clinical diagnosis of “TBI” or “no TBI.” The adjudication process will begin with each group after the participants have completed their respective follow up visit(s). The expert clinical diagnosis is obtained when 2 panel member's independent diagnoses agree. In the case of diagnostic disagreement, a third panel member performs an independent record review and serves as the tie breaker.
For participants who receive a head CT or MRI as part of standard care, their images are recorded and reviewed by a core lab. The neuroimaging core lab consists of a panel of board-certified neuro-radiologists, two of whom read all images and provide a report to the diagnostic adjudication committee. If the 2 readers do not agree, a 3rd will serve as a tie breaker to define the final report.
Control subjects will be enrolled for the purposes of assay development, including establishing the biomarker reference intervals, and will be cohorted into groups based on age and sex. These will include 120 trauma controls through 14 day follow up, and 120 healthy controls with a single visit. Trauma controls are defined as patients presenting to the ED for a traumatic injury and requiring an X-ray, but who do not have head trauma. Healthy controls will be subjects that report to be healthy and are not taking any prescription medications.
The BRAINBox TBI Test results determining a diagnosis of ATE, will be compared to the expert clinical diagnosis. Adjudicated patients assigned a diagnosis of TBI will be stratified for having high or low risk for post-concussive symptoms in each symptom category, based on biomarker and neuropsychiatric testing, and are defined as either “ATE with” or “ATE without” post-concussive symptoms. The high or low risk for post-concussive symptoms in each symptom category is defined using the validated interpretation guides for each test (Table 3), relevant literature with input from Key Opinion Leaders.
Algorithm Training and Testing
Data collected in this study will be used to finalize development of the BRAINBox TBI Test algorithms and to provide clinical validation of test performance. Using subjects in the training cohort, the BRAINBox diagnosis will be made by including the biomarker values, NC assessments and baseline demographics (age and sex) at the initial visit as predictors in a statistical/machine learning algorithm that estimates the ATE score. The decision threshold for ATE will be determined by identifying the ATE score that meets the diagnostic characteristic of a minimum sensitivity and specificity of 85 and 75%, respectively. To create the distributions for “ATE” and “no ATE” groups, the mean and the standard deviation of the score will be calculated on a log odds scale. For those subjects in the validation set, the final algorithm will be applied to the individual's biomarker values, neurocognitive assessments, and baseline demographics (age and sex) at the initial visit. The ATE score will be the output. If the ATE score exceeds the identified threshold, the subject will be classified as having “ATE,” otherwise, the subject will be classified as “no ATE.” A sample's log odds value will be compared to the log odds distribution of the “no ATE” subjects in the training set and a corresponding percentile will be assigned to the sample as the percentile relative to that distribution.
The performance of a prognostic ATE test will be determined by evaluating the biomarker values, neurocognitive assessments, and baseline demographics at the index visit, as predictors of 3 separate models that assign high or low risk to a subject for subsequent symptom occurrence at 14, 30, and 90 days. Symptom categories and tests performed during the study are provided in Table 3. Symptoms are evaluated as a composite at each time point, with thresholds to stratify subjects defined based on the scoring system/scale for each assessment, identifying the subject's risk for a specific symptom category at each time point. A separate statistical/machine learning algorithm will be developed for each time point that calculates the risk at the specific time after injury. In the algorithm, the definition of high or low risk for having post-concussive symptoms is defined by the decision threshold that produces the minimum sensitivity and specificity of 85 and 75%, respectively. For the validation process, the finalized algorithms will be applied to the subject's biomarker values, NC assessments, and baseline demographics (age and sex) at the index visit. If the output of the algorithm exceeds the identified decision threshold, the subject will be classified as high risk. Otherwise, the subject will be classified as low risk for post-concussive symptoms at the specified time point. The prognostic report is designed to aid the clinician in determining the subject's risk of having post-injury post-concussive symptoms at 14, 30, and 90 days.
Statistical Analysis
Sample Size Estimation
Sensitivity and specificity are used to determine performance, since both are essential in the characterization of diagnostic effectiveness of the BRAINBox TBI Test.
The following assumptions were used to calculate sample size for the primary endpoint (within 96 h of injury) with the blood specimens collected at the first-time point: a one-sample binomial test, a clinical sensitivity of 0.85 and specificity of 0.75, a “difference to detect” of 0.07, alpha of 0.05 and a power of 0.80. The assumptions for sensitivity and specificity are based on findings from HeadSMART pilot trial (30).
The sample size is based on the binomial test for a one sample design using a two-sided significance level of α = 0.05 and a power of 1-β = 0.80 (30). The following hypothesis will be tested:
Where Se (sensitivity) is the proportion of target condition positive subjects that yield a positive test result, Sp (specificity) is the proportion of target condition absent subjects that yield a negative test result, Se0 is the desired sensitivity and Sp0 is the desired specificity.
Generically for both sensitivity and specificity, let p0 represent the null value, p1 the alternative and let n and m represent the sample size and the number of observed outcomes of interest, respectively. Based on the binomial distribution, the sample size may be calculated using a numerical approach by solving two equations simultaneously. For a given two-sided significance level α (Type I error), there exists a critical value c (nonnegative integer), such that:
and
If m ≥ c, then the null hypothesis is rejected at α significance level. There exists the smallest sample size N, such that as long as n ≥ N, the power is always greater than or equal to 1-β. Then, N is the sample size from exact binomial testing.
Diagnosis of ATE
Prior to algorithm development, data will be divided into training and validation sets, with a model being derived using the training data. The training phase will include examination of potentially confounding and interacting variables (e.g., age, sex, time from injury until blood draw). Any confounding variables will be included as covariates in the model. Variables with insignificant interaction, as determined by backward selection, will be omitted. The model will provide a probability/score that a subject has ATE. The c-statistic will be reported, and a threshold selected to ensure the model meets the minimum sensitivity and specificity of 85 and 75%, respectively, with confidence intervals, positive predictive value and negative predictive value reported. Accuracy will be reported as the percentage of all suspected TBI subjects correctly classified as “ATE,” vs. “no ATE,” using the identified threshold. Validation data will be derived in an independent subject set (validation cohort), against which the developed model will be tested for generalizability. P-values comparing the AUC, sensitivity, specificity, and accuracy will be reported, with alpha defined as < 0.05. Sample size calculation was performed in PASS statistical software (32) using an assumed prevalence of 50% ATE in the subjects enrolled with blunt head injury. The final number of evaluable subjects for the “ATE,” and “no ATE” cohorts, should each not exceed 240, which makes the total number of evaluable subjects 480. An equal number of subjects are needed for algorithm validation (n = 480).
Prognosis of ATE
A separate model will be fit for symptoms at each post-enrollment time point, with data evaluated during the modeling phase, to determine the prognostic output report. Results will be grouped into low and high risk. Based on the data from the modeling/training phase, the symptom categories that are statistically supported, with sufficient prevalence to make a justified prognostic claim, will be reported. If applicable, symptoms categories may be combined into one overarching composite result, and defined as either low or high risk. Data handling for modeling will be the same as the diagnostic analysis, with the models identifying the likelihood that a subject will experience ATE symptoms at 14, 30, and 90 days.
Sample size for TBI subjects adjudicated as high or low risk for ATE symptoms at 14, 30 and 90-days post-injury is based on estimated prevalence of 60% of subjects having unresolved or emergent injury-related symptoms in the suspected ATE population at each time point (30). Using published and internal information on prevalence, as well as predefined sensitivity, specificity, confidence intervals, error, the total number of ATE subjects needed was calculated. The number of subjects with high risk of outcomes needed, the target condition for the prognosis, will be 236 evaluable subjects. The number of subjects without ATE will be 157, yielding a total number of subjects of 393 for algorithm training. With the estimated prevalence of ATE being 50%, a total of 786 blunt head trauma subjects is needed for algorithm training. This study design uses the same sample sizes for both training and validation phases, just as in the diagnostic models. Therefore, the same number of blunt head trauma subjects (n = 786) are needed for validation. To provide sufficient data for prognosis modeling in training and validation cohorts, up to 1,600 suspected ATE subjects will be needed. Enrollment numbers are subject to increase or decrease pending on true prevalence, results of data analysis and/or attrition.
The data collected in the clinical study that is directly related to study endpoints follows a BRAINBox Solutions blinding plan. Subjects from the training phase of the ATE diagnosis cohort may also be used in the training phase of the prognostic evaluation. Subjects from the validation phase of the ATE diagnosis cohort may be used in the validation phase of the prognostic evaluation. Therefore, the total number of suspected ATE patients should not exceed 1,600. In the event the actual prevalence differs from the assumed prevalence, the study design will include 2,000 blunt head trauma subjects as a maximum enrollment number.
Conclusions
This protocol proposes to collect data using a multi-modality approach, including blood biomarkers, clinical characteristics, neurocognitive and neuropsychological assessments, to develop diagnostic and prognostic algorithms for ATE. The validation phase will determine the accuracy of the BRAINBox ATE Test as an aid to the diagnosis of ATE, and as an objective determination of a patient's risk to develop post-concussive symptoms.
Ethics Statement
The studies involving human participants were reviewed and approved by the Local Ethics Committee of each participating institution. The patients/participants provided their written informed consent to participate in this study.
Author Contributions
WP wrote the first draft. All authors contributed to the article and approved the submitted version.
Funding
This study received funding from BRAINBox Solutions, Inc. The funder had the following involvement with the study: Study design. All authors were investigators, with their institutions receiving remuneration for the costs to perform this trial.
Conflict of Interest
This study received funding from Brainbox, Inc. The funder had the following involvement with the study: study design. All authors were investigators, with their institutions receiving remuneration for the costs to perform this trial.
Publisher's Note
All claims expressed in this article are solely those of the authors and do not necessarily represent those of their affiliated organizations, or those of the publisher, the editors and the reviewers. Any product that may be evaluated in this article, or claim that may be made by its manufacturer, is not guaranteed or endorsed by the publisher.
References
1. Gardner RC, Yaffe K. Epidemiology of mild traumatic brain injury and neurodegenerative disease. Mol Cell Neurosci. (2015) 66:75–80. doi: 10.1016/j.mcn.2015.03.001
2. Kay T, Harrington DE, Adams R, Anderson T, Berrol S, Cicerone K, et al. Definition of mild traumatic brain injury. J Head Trauma Rehabil. (1993) 8:86–7. doi: 10.1097/00001199-199309000-00009
3. Coronado VG, Xu L, Basavaraju SV, McGuire LC, Wald MM, Faul MD, et al. Surveillance for traumatic brain injury-related deaths–United States, 1997-2007. Centers for Disease Control and Prevention (CDC). MMWR Surveill Summ. (2011) 60:1–32.
4. Surveillance Report of Traumatic Brain Injury-related ED Visits, Hospitalizations, Deaths—United States. Centers for Disease Control Prevention. U.S. Department of Health and Human Services (2014).
5. Corwin DJ, Arbogast KB, Haber RA, Pettijohn KW, Zonfrillo MR, Grady MF, et al. Characteristics and outcomes for delayed diagnosis of concussion in pediatric patients presenting to the ED. J Emerg Med. (2020) 59:795–804. doi: 10.1016/j.jemermed.2020.09.017
6. Boutis K, Weerdenburg K, Koo E, Schneeweiss S, Zemek R. The diagnosis of concussion in a pediatric ED. J Pediatr. (2015) 166:1214–20. doi: 10.1016/j.jpeds.2015.02.013
7. Arbogast KB, Curry AE, Pfeiffer MR, Zonfrillo MR, Haarbauer-Krupa J, Breiding MJ, et al. Point of health care entry for youth with concussion within a large pediatric care network. JAMA Pediatr. (2016) 170:e160294. doi: 10.1001/jamapediatrics.2016.0294
8. Schneider ALC, Wang D, Ling G, Gottesman RF, Selvin E. Prevalence of self-reported head injury in the United States. N Engl J Med. (2018) 379:1176–8. doi: 10.1056/NEJMc1808550
9. Taylor CA, Bell JM, Breiding MJ, Xu L. Traumatic brain injury–related ED visits, hospitalizations, and deaths — United States, 2007 and 2013. MMWR Surveill Summ. (2017) 66:1–16. doi: 10.15585/mmwr.ss6609a1
10. Tenovuo O, Diaz-Arrastia R, Goldstein LE, Sharp DJ, van der Naalt J, Zasler ND. Assessing the severity of traumatic brain injury-time for a change? J Clin Med. (2021) 10:148. doi: 10.3390/jcm10010148
11. Tagge CA, Fisher AM, Minaeva OV, Gaudreau-Balderrama A, Moncaster JA, Zhang XL, et al. Concussion, microvascular injury, and early tauopathy in young athletes after impact head injury and an impact concussion mouse model. Brain. (2018) 141:422–458. doi: 10.1093/brain/awx350
12. McMahon P, Hricik A, Yue JK, Puccio AM, Inoue T, Lingsma HF, et al. Symptomatology and functional outcome in mild traumatic brain injury: results from the prospective TRACK-TBI study. J Neurotrauma. (2014) 31:26–33. doi: 10.1089/neu.2013.2984
13. Ahman S, Saveman BI, Styrke J, Björnstig U, Stålnacke BM. Long-term follow-up of patients with mild traumatic brain injury: a mixed-method study. BMJ Rehabil Med. (2013) 45:758–64. doi: 10.2340/16501977-1182
14. Kristman VL, Borg J, Godbolt AK, Salmi LR, Cancelliere C, Carroll LJ, et al. Methodological issues and research recommendations for prognosis after mild traumatic brain injury: results of the international collaboration on mild traumatic brain injury prognosis. Arch Phys Med Rehabil. (2014) 95(Suppl. 3):S265–77. doi: 10.1016/j.apmr.2013.04.026
15. NINDS Common Data Elements: Traumatic Brain Injury. National Institutes of Neurological Diseases and Stroke. Available online at: http://www.commondataelements.ninds.nih.gov/TBI.aspx-tab=Data_Standards (accessed September 10, 2021).
16. Bazarian JJ, Biberthaler P, Welch RD, Lewis LM, Barzo P, Bogner-Flatz V, et al. Serum GFAP and UCH-L1 for prediction of absence of intracranial injuries on head CT (ALERT-TBI): a multicentre observational study. Lancet Neurol. (2018) 17:782–9. doi: 10.1016/S1474-4422(18)30231-X
17. Korley FK, Peacock WF, Eckner JT, Maio R, Levin S, Bechtold KT, et al. Clinical gestalt for early prediction of delayed functional and symptomatic recovery from mild traumatic brain injury is inadequate. Acad Emerg Med. (2019) 26:1384–7. doi: 10.1111/acem.13844
18. Levin HS, Diaz-Arrastia RR. Diagnosis, prognosis, and clinical management of mild traumatic brain injury. Lancet Neurol. (2015) 14:506–17. doi: 10.1016/S1474-4422(15)00002-2
19. Yang S, Flores B, Magal R, Harris K, Gross J, Ewbank A, et al. Diagnostic accuracy of tablet-based software for the detection of concussion. PLoS ONE. (2017) 12:e0179352. doi: 10.1371/journal.pone.0179352
20. Bell KR, Hoffman JM, Temkin NR, Powell JM, Fraser RT, Esselman PC, et al. The effect of telephone counselling on reducing post-traumatic symptoms after mild traumatic brain injury: a randomised trial. J Neurol Neurosurg Psychiatry. (2008) 79:1275–81. doi: 10.1136/jnnp.2007.141762
21. Ponsford J, Willmott C, Rothwell A, Cameron P, Kelly AM, Nelms R, et al. Impact of early intervention on outcome following mild head injury in adults. J Neurol Neurosurg Psychiatry. (2002) 73:330–2. doi: 10.1136/jnnp.73.3.330
22. Hinkle JL, Alves WM, Rimell RW, Jane JA. Restoring social competence in minor head-injury patients. J Neurosci Nurs. (1986) 18:268–71. doi: 10.1097/01376517-198610000-00006
23. Mittenberg W, Tremont G, Zielinski RE, Fichera S, Rayls KR. Cognitive-behavioral prevention of postconcussion syndrome. Arch Clin Neuropsychol. (1996) 11:139–45. doi: 10.1093/arclin/11.2.139
24. Arundine A, Bradbury CL, Dupuis K, Dawson DR, Ruttan LA, Green RE. Cognitive behavior therapy after acquired brain injury: maintenance of therapeutic benefits at 6 months posttreatment. J Head Trauma Rehabil. (2012) 27:104–12. doi: 10.1097/HTR.0b013e3182125591
25. Common Data Elements; Modular Data Elements for Traumatic Brain Injury. Available online at: http://www.tbi-impact.org/cde/ (accessed December 09 2021).
26. Roy D, Peters ME, Everett A, Leoutsakos JM, Yan H, Rao V, et al. Loss of consciousness and altered mental state predicting depressive and post-concussive symptoms after mild traumatic brain injury. Brain Inj. (2019) 33:1064–9. doi: 10.1080/02699052.2019.1606447
27. Richey LN, Rao V, Roy D, Narapareddy BR, Wigh S, Bechtold KT, et al. Age differences in outcome after mild traumatic brain injury: results from the HeadSMART study. Int Rev Psychiatry. (2020) 32:22–30. doi: 10.1080/09540261.2019.1657076
28. Korley FK, Diaz-Arrastia R, Falk HJ, Peters ME, Leoutsakos JS, Roy D, et al. Prevalence of incomplete functional and symptomatic recovery among patients with head injury but brain injury debatable. J Neurotrauma. (2017) 34:1531–8. doi: 10.1089/neu.2016.4723
29. Falk H, Bechtold KT, Peters ME, Roy D, Rao V, Lavieri M, et al. A prognostic model for predicting one-month outcomes among emergency department patients with mild traumatic brain injury and a presenting glasgow coma scale of fifteen. J Neurotrauma. (2021) 38:2714–22. doi: 10.1089/neu.2021.0137
30. Peacock WF 4th, Van Meter TE, Mirshahi N, Ferber K, Gerwien R, Rao V, et al. Derivation of a three biomarker panel to improve diagnosis in patients with mild traumatic brain injury. Front Neurol. (2017) 8:641. doi: 10.3389/fneur.2017.00641
31. U.S. Department of Health and Human Services Food and Drug Administration, E6 (R2) Good Clinical Practice: Integrated Addendum to ICH E6(R1) Guidance for Industry ICH E6. (2018). Available online at: https://www.fda.gov/downloads/Drugs/Guidances/UCM464506.pdf (accessed 26 June, 2021).
Keywords: traumatic brain injury, neuropsychiatric testing for TBI, biomarkers for TBI, diagnosis of TBI, prognosis of TBI
Citation: Peacock WF, Kuehl D, Bazarian J, Singer AJ, Cannon C, Rafique Z, d'Etienne JP, Welch R, Clark C and Diaz-Arrastia R (2021) Defining Acute Traumatic Encephalopathy: Methods of the “HEAD Injury Serum Markers and Multi-Modalities for Assessing Response to Trauma” (HeadSMART II) Study. Front. Neurol. 12:733712. doi: 10.3389/fneur.2021.733712
Received: 30 June 2021; Accepted: 26 October 2021;
Published: 08 December 2021.
Edited by:
Kerry Peek, The University of Sydney, AustraliaReviewed by:
Andrew P. Lavender, Federation University Australia, AustraliaThomas George Di Virgilio, University of Stirling, United Kingdom
Copyright © 2021 Peacock, Kuehl, Bazarian, Singer, Cannon, Rafique, d'Etienne, Welch, Clark and Diaz-Arrastia. This is an open-access article distributed under the terms of the Creative Commons Attribution License (CC BY). The use, distribution or reproduction in other forums is permitted, provided the original author(s) and the copyright owner(s) are credited and that the original publication in this journal is cited, in accordance with accepted academic practice. No use, distribution or reproduction is permitted which does not comply with these terms.
*Correspondence: W. Frank Peacock, Frankpeacock@gmail.com