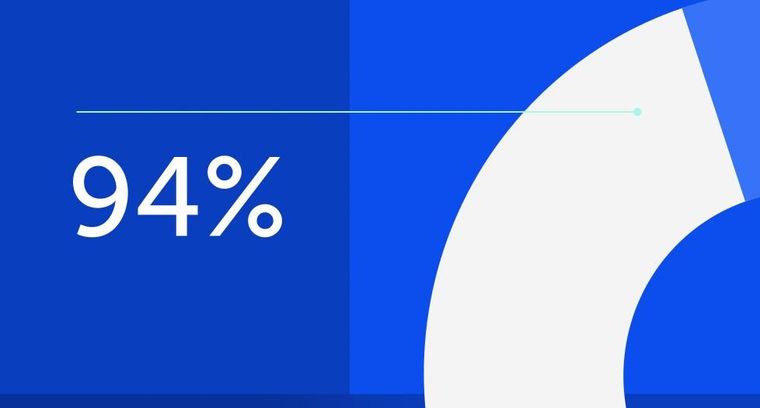
94% of researchers rate our articles as excellent or good
Learn more about the work of our research integrity team to safeguard the quality of each article we publish.
Find out more
PERSPECTIVE article
Front. Neurol., 07 September 2021
Sec. Applied Neuroimaging
Volume 12 - 2021 | https://doi.org/10.3389/fneur.2021.700833
This article is part of the Research TopicNeuroimaging for the Measurement and Management of PainView all 14 articles
Pain is a complex, multidimensional experience that emerges from interactions among sensory, affective, and cognitive processes in the brain. Neuroimaging allows us to identify these component processes and model how they combine to instantiate the pain experience. However, the clinical impact of pain neuroimaging models has been limited by inadequate population sampling – young healthy college students are not representative of chronic pain patients. The biopsychosocial approach to pain management situates a person's pain within the diverse socioeconomic environments they live in. To increase the clinical relevance of pain neuroimaging models, a three-fold biopsychosocial approach to neuroimaging biomarker development is recommended. The first level calls for the development of diagnostic biomarkers via the standard population-based (nomothetic) approach with an emphasis on diverse sampling. The second level calls for the development of treatment-relevant models via a constrained person-based (idiographic) approach tailored to unique individuals. The third level calls for the development of prevention-relevant models via a novel society-based (social epidemiologic) approach that combines survey and neuroimaging data to predict chronic pain risk based on one's socioeconomic conditions. The recommendations in this article address how we can leverage pain's complexity in service of the patient and society by modeling not just individuals and populations, but also the socioeconomic structures that shape any individual's expectations of threat, safety, and resource availability.
Neuroimaging models have significantly expanded our understanding of the neural processes that instantiate a person's subjective pain experience [for reviews see (1–3)]. Through neuroimaging, we have learned that the brain representation of pain is highly distributed and multidimensional involving sensory, cognitive, and affective components (4–7). Neuroimaging models employing multivariate [i.e., multivoxel pattern analysis or MVPA; (8)], predictive (i.e., machine learning), and network analysis techniques can, respectively, delineate multiple component processes that contribute to both acute and chronic pain (7, 9–11), predict a person's self-reported evoked pain intensity (12, 13), and localize sites of functional connectivity disruption across chronic pain phenotypes (14, 15).
Despite these important advances, neuroimaging research has yet to significantly impact the clinic. Anatomical and resting state markers lack specificity- it remains unknown whether changes are due to chronic pain or to co-morbidities like anxiety and depression [for reviews see (16, 17)]. Furthermore, most models are developed on experimental data of evoked phasic pain where participants experience a brief (under 12 s) noxious stimulus such as prick or a hot plate against the skin. This does not translate well to chronic pain which must persist 3 or more months. Acute or phasic pain is typically appraised as temporary and separate from the self, while chronic pain is typically appraised as unending and apart of one's life (18). Chronic pain is also highly personalized and embedded in spontaneous and tonic, rather than evoked and phasic, activity in the brain (19–22). Finally, population samples are not well-stratified across economic class, race, or ethnicity (23). In most cases, participant socioeconomic status (SES) is not reported nor well-measured [for a review see (24)]. Because chronic pain disproportionately affects the poor and working class across the globe (25–33), neuroimaging models of pain must take socioeconomic information into account.
The biopsychosocial approach to pain management attempts to encapsulate the broader societal issues which situate interactions among the biological, psychological, and social components of the pain experience (34). This conceptual framework states that understanding pain requires an understanding of the whole patient, their relationships, and society (35, 36). However, the biopsychosocial approach is largely theoretical and has yet to be well-integrated into pain neuroimaging research. To resolve this translational gap, this perspective formulizes the biopsychosocial approach into testable neuroimaging models intended for the diagnosis, treatment, and prevention of chronic pain. These models endeavor to predict and understand chronic pain from three levels, that of the individual, of the population, and of society.
First, recommendations are made to increase the diagnostic relevance of the population-based, or nomothetic, approach to the development of pain neuroimaging models. These recommendations include a shift in focus from evoked phasic pain to evoked tonic pain paradigms and the recruitment of larger and more diverse population samples. Second, a person-based or idiographic approach to the development of treatment-relevant models is discussed. Recommendations are made for the training and implementation of these models so that they can be used to track disease progress and treatment efficacy within individual patients. Finally, a novel society-based, or social epidemiological approach to the development of prevention-relevant models is proposed. This approach situates an individual's disease state within the socioeconomic conditions they live in. Lastly, implications for both the clinic and public policy are outlined.
Human subjects research is largely nomothetic, that is, the goal is to generate an explanation of brain activity that is “universal” and generalizable to entire populations (Figure 1A). Such models are trained on many different people sampled from the same population. Individual differences are treated as noise and intentionally minimized through careful inclusion/exclusion criteria, outlier removal, and the inclusion of confound regressors controlling for demographic variables such as age and gender identity [for a review see (37)]. The nomothetic approach is appropriate for the development of diagnostic biomarkers because inferences must be drawn from the wider population to identify pain pathologies in new patients presenting symptoms for the first time.
Figure 1. Three-level biopsychosocial approach to neuroimaging biomarker development. (A) Population-based (nomothetic) approach to diagnostic model development. Neuroimaging model weights are estimates of population-level associations between brain activity and pain outcomes (i.e., diagnostic category vs. healthy control). Population samples should be large and diversely sampled across gender identity, race, and socioeconomic identities. Models should be validated on external clinical data sets. Models can then be applied to the brain activity of a new patient to diagnosis their pain condition. (B) Person-based (idiographic) approach to treatment-relevant models. Neuroimaging model weights are estimates of person-level associations between brain activity and pain outcomes (i.e., pain severity) for the same person through time. Models weights can be regulated by nomothetic models to lessen demands on data collection from one patient. Models can be applied in the same patient at later time points to assess their disease progression or to assess treatment efficacy. Such models can be used to tailor treatment selection on a case-by-case basis. (C) Society-based (social epidemiologic) approach to prevention-relevant models. This approach requires two steps. First, participants complete a multidimensional survey that assesses both their environment (i.e., socioeconomic status) and their personal internalization of these conditions (Table 1). Then, a risk model is trained on these survey data to predict pain severity. The weights of this risk model are estimates of population-level associations between a person's socioeconomic conditions and pain outcomes. This model can be applied to the survey data of a new patient to assess their risk of pain chronification. Person-level survey data can be related to person-level pain-related brain activity, and then a neuroimaging model of the SES component of pain processing can be developed. Neuroimaging model weights are estimates of group-level associations between the socioeconomic conditions a person lives in and their pain-related brain activity. Such a model could be combined with other neuroimaging component process models of pain, such as the NPS and SIIPS1, to predict clinical outcomes in new patients.
Nomothetic neuroimaging model weights are estimates of population-level associations between brain activity and pain outcomes (i.e., self-reported pain intensity). Models are cross-validated via an iterative “leave-N-subjects-out” procedure to assess performance on out-of-sample participants [for recommendations see (38)]. Next, they are validated on held out “validation sets”; though this external validation process is not common in single neuroimaging studies due to the demand on sample size. More often, this validation process occurs over a series of papers across unique data sets collected on different scanners in varied locations [for a review see (2)]. This a slower validation process, but it is a more thorough and robust one. Once validated, the model's predictions are deemed suitable for application to a new individual drawn from the same population.
A strength of this approach is its ability to identify separable component processes of pain (7). For example, the neurologic pain signature (NPS) is a well-validated model for acute pain evoked by noxious events (13). It captures a component process that contributes to the perceived intensity of an acute painful stimulus. It includes patterns of activity in the anterior cingulate, somatosensory cortex, and periaqueductal gray. Woo et al. (7) developed a separate multivariate predictive model of pain called the stimulus intensity independent pain signature-1 (SIIPS1). SIIPS1 captures fluctuations in pain independent of noxious stimulus intensity. It includes activity in the nucleus accumbens, lateral prefrontal cortex (PFC), and parahippocampal cortex. When combined with the NPS, the two explain more variance in brain activity than either model alone. However, the combined variance explained is 30%, indicating that there are more component processes relevant to evoked pain experiencing that have yet to be discovered (Figure 1C).
Though the NPS and SIIPS1 can predict different aspects of acute pain experiencing, they cannot distinguish between chronic pain patients and controls. It is unclear whether models trained on evoked phasic pain are informative for the diagnosis of chronic pain. To distinguish between fibromyalgia patients and healthy controls, the NPS was subdivided into its positive activations and then combined with a multisensory model similar to SIIPS1 and a separate model trained to predict evoked pain in fibromyalgia patients (9). The combinatorial model performed with high accuracy within the study it was developed, however, it is unknown how it performs in external data sets. Combining models like this may be prone to overfitting, so the preregistration of model combinations is recommended.
The translational limitations of evoked phasic pain models may be due to the phasic, rather than the evoked, nature of the noxious stimuli. Recently, a tonic pain neuroimaging biomarker with clinical relevance was developed. This biomarker, called TOPS, was trained on evoked tonic pain trails in healthy controls (39). In this experiment, capsaicin was placed on the tongue to evoke pain for 1-2 min. TOPS can predict clinical pain severity and distinguish between patients and controls in two independent studies of chronic low back pain. It is possible that tonic stimulations hold greater clinical utility than phasic because longer stimulations allow for rumination and the activation of resting state networks that may play a role in the chronification of pain (22, 40, 41).
TOPS was able to track within-individual variations in pain avoidance ratings with an average correlation of r = 0.51. Though this holds promise for the clinic, there is still much variance left to be explained. Pain is an idiosyncratic experience with many dimensions; therefore, the nomothetic approach may never be able to explain the entirely of an individual's pain experience, however, a “good enough” approximation might be achieved through the development of a suite of component process models that can be combined on a person-by-person basis. As we build more models of pain components, such as social context, interoception, affect, and expectations for pain relief, we may begin to chip away at this complex neural representation.
To this end, I make the following recommendations: First, a concerted effort must be made to recruit larger, more representative samples of the population. Nomothetic models are only suitable for application on new individuals drawn from the same population in which they were trained. The NPS was trained on only 20 participants, eight of which are women and 79% are White. Sampling procedures which primarily recruit from the student pool of the universities where the research is conducted unintentionally select for young high income and high education level White participants not of Hispanic origin (23). This is not representative of the world at large, nor is it representative of populations suffering from chronic pain. In the United States, most chronic pain patients are low-education and low-income women of color over the age of 45 (26, 42, 43).
Funding agencies must provide sufficient support so that researchers can expand their recruitment, possibly by employing companies that specialize in representative sampling to stratify samples across age, gender identity, race, ethnicity, wealth and income, education level, and personality traits. Second, pain models and pain data sets should be made open and shareable to increase collective clinical impact. Patient data sets, especially those involving spontaneous pain paradigms, are difficult to collect, but are the most clinically-relevant. With increased data sharing, new pain components developed in easier to collect (i.e., evoked pain in healthy controls) diverse populations can be validated in clinically-relevant samples to improve translation and impact.
Pain is heterogeneous. The nomothetic assumption that “one-size-fits-all” ignores diversity in economic class, cultural background, gender identity, ethnicity, and personality, and limits applicability in real-world pain treatment. For example, emotional pain is positively correlated with physical pain at the group level, but this relationship is inconsistent across time within unique individuals (44). Indeed, neither SIIPS1 nor TOPS positively predicts pain in each individual the model was trained on; approximately 2-3% of the training data show effects in the opposite direction. It is possible that one's unique experiences with pain can influence the magnitude or direction of the relationship a pain component process has on their individual pain response. The idiographic approach accounts for variance across individuals by allowing for personalized predictions. Individual differences in pain expression have made it difficult for biomarkers to be developed on lower dimensional data like facial expressions, skin conductance responses, and heart rate, however, recent idiographic approaches to modeling these types of data have significantly improved their predictive power (45–47). In the clinic, such models may provide objective assessments of disease progression and treatment progress.
In the person-based approach, models are trained on many different samples from the same individual (Figure 1B). This commonly involves estimating pain-related brain activity from single trials within one experimental session. Predictive brain maps developed on one participant should be internally cross-validated to test the model's ability to predict pain outcomes on out-of-sample trials from the same participant. While it might be useful to validate the model on later timepoints, current evidence suggests that there is stability in a single individual's network-level representation of the same stimulus through time (48).
Advantages of these models include improved accuracy and the ability to capture representations at finer spatial scales [e.g., (49–53)]. Because idiographic models require hours of data acquisition from a single participant, it can be difficult to collect from patients. One way to reduce the demands on scan time is to constrain the idiographic model with nomothetic priors. For example, Lindquist et al. (52) regularized an idiographic model of acute pain in healthy controls with the NPS. The regularized model performed better than both the NPS and a purely idiographic model trained on that subject's data alone. This method of regularization is known as group-regularized individual prediction (GRIP). It combines population-based and idiographic models in proportion to their variances. It does this by applying a shrinkage factor to the model weights. The shrinkage factor penalizes idiographic activity that appears unlikely (i.e., noise) relative to group activity.
Non-regularized idiographic models are still likely to be useful if sufficient data are collected from the patient. The recommendation here is to compare the performance of regularized and non-regularized idiographic models within patients and select the best model on a patient-by-patient basis. This patient-tailored model can later be applied to their own brain activity in longitudinal follow-ups and intervention paradigms to track disease progress and treatment efficacy. It could also be deployed in real-time neurofeedback paradigms where participants can test multiple interventions and empirically validate which works best for them [see (54)]. Within this framework, a diversity of treatments (e.g., drugs, expectancy manipulations, placebo interventions, self-regulation, or mindfulness) can be tested with reduced bias.
Studies of global chronic pain prevalence suggest that societal stressors may contribute to the chronification of pain (32, 55–58). This is not surprising–the relationship between one's economic class and chronic illness has been observed as early as 1848, when Rudolph Virchow determined that treating the Typhus epidemic in Upper Silesia would require more than medicine. Virchow prescribed changes to the material conditions of the people whom the epidemic most severely impacted—the poor and working class (59). He concluded that though all illness has a biological origin, where it spreads and who is most susceptible is determined by structural factors such as housing, working conditions, diet, and sanitation (60). Similar observations have been made about chronic pain today. When controlling for age, race, and education level, a study conducted in an urban trauma center found that homelessness and low income were strongly associated with chronic pain (27).
Relationships between low economic class and chronic pain prevalence have been found across the United States (26, 61, 62) as well as across different cultures and countries including South Africa (63), Brazil (31), Iran (64), Germany (65), Austria (56), Sweden (66), Finland (67), the United Kingdom (25, 68), Japan (28), Nepal (33), and South Korea (69). Despite the long history and geographic spread of these associations, SES has largely been ignored by pain neuroimaging research. There are several reasons for this: First, there is little communication between epidemiologists and neuroimagers [an effort to correct this has begun, see (70)]. Second, the lack of socioeconomic diversity in research samples obfuscates this connection. Finally, it is difficult to mathematically relate complex social structures to functional brain activity. To the author's knowledge, only one neuroimaging study has done this to date (10). Here I propose to resolve this gap with a social epidemiologic approach to neuroimaging models of chronic pain.
Social epidemiologists study how socioeconomic structures, institutions (i.e., law, education), and social relationships influence health outcomes. A social epidemiologic approach to neuroimaging models of pain relates the structure of society to brain health and function. The primary goal of this approach is chronic pain prevention. The first step is to collect survey data assessing an individual's socioeconomic conditions and subjective experience of social status. This multidimensional assay can then be applied to pain-related brain activity to develop a neuroimaging model of socioeconomic contributions to chronic pain (Figure 1C). The resulting SES neuroimaging model may be a component process of pain useful for combinatorial models described earlier. This approach may allow us to identify patients most at risk for pain chronification because one's internalization of their socioeconomic conditions may play a role in the onset and maintenance of chronic pain (58, 61, 71).
The transition from acute to chronic pain is marked by a shift in processing from nociceptive components to socioemotional components of pain—specifically, PFC-limbic circuitry, including the NAc/striatum, amygdala, and hippocampus (72, 73), and the default mode network [DMN; (41)]. Changes to PFC-limbic circuitry may indicate a change in the valuation of pain (11, 74). Changes to DMN connectivity may change how the pain experience is construed in relation to the self (75, 76). Both of these networks are altered by poverty and socioeconomic stress (77). Activity in the PFC (78, 79) and ventral striatum (80) differs as a function of SES during both valuation and the processing of self-related information (81–83). Childhood poverty is correlated with aberrant functional connectivity within the DMN (84, 85). Interestingly, these aberrations can be reversed in people who have high income later in life (86). Relatedly, (10) found a threshold in annual income (>$25,000) that delineated vulnerability from protection in chronic pain patients. In the United States, the poverty line for a family of four is $26,200; meaning families that make less than this cannot afford food, rent, and other basic needs (87). It is unknown whether changes in income can reverse chronic pain status, however, chronic pain patients of high SES tend to have better clinical outcomes (88).
The impact of socioeconomic stress on chronic pain may not be reducible to income alone. The experience of social strain or subordination itself may contribute to chronic illness above and beyond income-level (89, 90). In non-human primates low social status is associated with immune system deficits that increase risk of infection and slow wound healing (91, 92). Chronic social stress may underlie immunosuppression in humans and animals [for a review see (93)]. People in lower social classes have a lower sense of personal control which is associated with higher levels of stress and pain (94). However, a high sense of self-efficacy is protective against chronic pain and pain severity (95). The protective effect of self-efficacy may be independent of class. For example, a large study in South Korea (N = 28,532) demonstrated that when controlling for monthly income, the presence of labor unions reduced low back pain prevalence (69). Another study in the United States found that unionized workers experience less severe pain for work-related musculoskeletal disorders (96). One interpretation of these effects is that labor unions change perceptions of self-efficacy, pain controllability, and expectations for care and safety by giving worker's the ability to advocate for themselves through collective bargaining (97).
A major barrier to the study of socioeconomic factors in chronic pain is the lack of a standardized assessment of SES. Here I propose the creation of a “Pain-Predispositions Profile Survey” (Table 1), a multidimensional assay of debt, income, property ownership, investments/savings, family wealth, education, perceived social status, environment (urban or rural), housing situation, childhood attachment, SES-related personality/evaluative traits (i.e., pain catastrophizing, controllability perceptions), as well as measures of income inequality within the city and country the patient resides in. A predisposition model of chronic pain can then be developed on these survey data that predicts patient pain status or severity. A cross-validated procedure similar to that employed by Vachon-Presseau et al. (10) can then be used to relate the survey data to functional networks in chronic pain patients (or healthy participants in evoked pain paradigms) to uncover a socioeconomic-related component process contributing to the pain experience (Figure 1C). Neuroimaging may not always be an available tool for the diagnosis and treatment of chronic pain—the survey-based model, however, is scalable and can be leveraged for treatment selection by matching people on survey similarity. Treatment programs that are validated on patients in neuroimaging studies can then be recommended to new patients with greater confidence.
An individual's valuation of a painful event (113–115), their expectations for support and health care (116–118), their beliefs about pain permanence (119, 120), personality traits (10, 121), and the socioeconomic conditions they exist in (10, 122) influence their brains' representation of pain. Pain, therefore, is a personal experience instantiated by biological processes and situated within one's socioeconomic conditions. Neuroimaging models situated within the socioeconomic structures of the population being studied are necessary for the development of a more complete understanding of the complexities of human pain. In this perspective, I discuss how three approaches to the development of pain neuroimaging models—nomothetic (population-based), idiographic (person-based), and social epidemiologic (society-based)—can be applied to the diagnosis, treatment, and prevention of chronic pain. These three approaches taken together serve to operationalize the biopsychosocial model of pain within a neuroimaging context.
It is estimated that 1% of the world's population controlled 44.8% of the world's wealth in 2018 (123). Economists from varied and opposing points on the political spectrum agree that an increasingly globalized and automated economy will heighten existing barriers to economic mobility and make income inequality more stark, widespread, and permanent (124). Therefore, it is my final recommendation that scientists and clinicians advocate for chronic pain patients at the level of public policy. In the words of Virchow, “Disease is only a manifestation of life under pathological conditions… Medicine is a social science and politics is nothing else but medicine on a large scale.”
The original contributions presented in the study are included in the article/supplementary material, further inquiries can be directed to the corresponding author/s.
The author confirms being the sole contributor of this work and has approved it for publication.
NIMH (R01 MH112560), Computational and brain predictors of emotion cue integration (PI: Zaki).
The author declares that the research was conducted in the absence of any commercial or financial relationships that could be construed as a potential conflict of interest.
All claims expressed in this article are solely those of the authors and do not necessarily represent those of their affiliated organizations, or those of the publisher, the editors and the reviewers. Any product that may be evaluated in this article, or claim that may be made by its manufacturer, is not guaranteed or endorsed by the publisher.
Special thanks to Bogdan Petre, Marta Ceko, Dean Baltiansky, and Jamil Zaki for providing comments on the ideas expanded in this paper.
1. Apkarian AV, Baliki MN, Farmer MA. Predicting transition to chronic pain. Curr Opin Neurol. (2013) 26:360–7. doi: 10.1097/WCO.0b013e32836336ad
2. Reddan MC, Wager TD. Modeling pain using fMRI: from regions to biomarkers. Neurosci Bull. (2018) 34:208-15. doi: 10.1007/s12264-017-0150-1
3. Woo CW, Chang LJ, Lindquist MA, Wager TD. Building better biomarkers: brain models in translational neuroimaging. Nat Neurosci. (2017) 20:365–77. doi: 10.1038/nn.4478
4. Coghill RC, Sang CN, Maisog JM, Iadarola MJ. Pain intensity processing within the human brain: a bilateral, distributed mechanism. J Neurophysiol. (1999) 82:1934–43. doi: 10.1152/jn.1999.82.4.1934
5. Melzack R. Pain and the neuromatrix in the brain. J Dent Educ. (2001) 65:1378–82. doi: 10.1002/j.0022-0337.2001.65.12.tb03497.x
6. Rainville P, Duncan GH, Price DD, Carrier B, Bushnell MC. Pain affect encoded in human anterior cingulate but not somatosensory cortex. Science. (1997) 277:968–71. doi: 10.1126/science.277.5328.968
7. Woo C-W, Schmidt L, Krishnan A, Jepma M, Roy M, Lindquist MA, et al. Quantifying cerebral contributions to pain beyond nociception. Nat Commun. (2017) 8:14211. doi: 10.1038/ncomms14211
8. Haxby JV. Multivariate pattern analysis of fMRI: the early beginnings. NeuroImage. (2012) 62:852–855. doi: 10.1016/j.neuroimage.2012.03.016
9. López-Solà M, Woo CW, Pujol J, Deus J, Harrison BJ, Monfort J, et al. Towards a neurophysiological signature for fibromyalgia. Pain. (2017) 158:34-47. doi: 10.1097/j.pain.0000000000000707
10. Vachon-Presseau E, Berger SE, Abdullah TB, Griffith JW, Schnitzer TJ, Apkarian AV. Identification of traits and functional connectivity-based neurotraits of chronic pain. PLoS Biol. (2019) 17:e3000349. doi: 10.1371/journal.pbio.3000349
11. Woo CW, Roy M, Buhle JT, Wager TD. Distinct brain systems mediate the effects of nociceptive input and self-regulation on pain. PLoS Biol. (2015) 13:e1002036. doi: 10.1371/journal.pbio.1002036
12. Van Oudenhove L, Kragel PA, Dupont P, Ly HG, Pazmany E, Enzlin P, et al. Common and distinct neural representations of aversive somatic and visceral stimulation in healthy individuals. Nat Commun. (2020) 11:5939. doi: 10.1038/s41467-020-19688-8
13. Wager TD, Atlas LY, Lindquist MA, Roy M, Woo CW, Kross E. An fMRI-based neurologic signature of physical pain. N Engl J Med. (2013) 368:1388-97. doi: 10.1056/NEJMoa1204471
14. Kucyi A, Davis KD. The dynamic pain connectome. Trends Neurosci. (2015) 38:86–95. doi: 10.1016/j.tins.2014.11.006
15. Mansour A, Baria AT, Tetreault P, Vachon-Presseau E, Chang PC, Huang L, et al. Global disruption of degree rank order: a hallmark of chronic pain. Sci Rep. (2016) 6:1–17. doi: 10.1038/srep34853
16. Pfannmöller J, Lotze M. Review on biomarkers in the resting-state networks of chronic pain patients. Brain Cogn. (2019) 131:4–9. doi: 10.1016/j.bandc.2018.06.005
17. Tracey I, Woolf CJ, Andrews NA. Composite pain biomarker signatures for objective assessment and effective treatment. Neuron. (2019) 101:783–800. doi: 10.1016/j.neuron.2019.02.019
18. Grichnik KP, Ferrante FM. The difference between acute and chronic pain. Mt Sinai J Med. (1991) 58:217–20.
19. Baliki MN, Baria AT, Apkarian AV. The cortical rhythms of chronic back pain. J Neurosci. (2011) 31:13981–90. doi: 10.1523/JNEUROSCI.1984-11.2011
20. Baliki MN, Chialvo DR, Geha PY, Levy RM, Harden RN, Parrish TB, et al. Chronic pain and the emotional brain: specific brain activity associated with spontaneous fluctuations of intensity of chronic back pain. J Neurosci. (2006) 26:12165–73. doi: 10.1523/JNEUROSCI.3576-06.2006
21. Huang T, Zhao Z, Yan C, Lu J, Li X, Tang C, et al. Altered spontaneous activity in patients with persistent somatoform pain disorder revealed by regional homogeneity. PLoS ONE. (2016) 11:1–17. doi: 10.1371/journal.pone.0151360
22. Spisak T, Kincses B, Schlitt F, Zunhammer M, Schmidt-Wilcke T, Kincses ZT, et al. Pain-free resting-state functional brain connectivity predicts individual pain sensitivity. Nat Commun. (2020) 11: 187. doi: 10.1038/s41467-019-13785-z
23. Lewinn KZ, Sheridan MA, Keyes KM, Hamilton A, McLaughlin KA. Sample composition alters associations between age and brain structure. Nat Commun. (2017) 8:874. doi: 10.1038/s41467-017-00908-7
24. Farah MJ. The neuroscience of socioeconomic status: correlates, causes, and consequences. Neuron. (2017) 96:56–71. doi: 10.1016/j.neuron.2017.08.034
25. Aggarwal VR, Macfarlane TV, Macfarlane GJ. Why is pain more common amongst people living in areas of low socio-economic status? A population-based cross-sectional study. Br Dent J. (2003) 194:383–7. doi: 10.1038/sj.bdj.4810004
26. Grol-Prokopczyk H. Sociodemographic disparities in chronic pain, based on 12-year longitudinal data. Pain. (2017) 158:313–22. doi: 10.1097/j.pain.0000000000000762
27. Hanley O, Miner J, Rockswold E, Biros M. The relationship between chronic illness, chronic pain, and socioeconomic factors in the ED. Am J Emerg Med. (2011) 29:286–92. doi: 10.1016/j.ajem.2009.10.002
28. Ikeda T, Sugiyama K, Aida J, Tsuboya T, Watabiki N, Kondo K, et al. Socioeconomic inequalities in low back pain among older people: the JAGES cross-sectional study. Int J Equity Health. (2019) 18:1–11. doi: 10.1186/s12939-019-0918-1
29. Jackson T, Thomas S, Stabile V, Han X, Shotwell M, McQueen KA, et al. Chronic pain without clear etiology in low- and middle-income countries. Anesth Analg. (2016) 122:2028–39. doi: 10.1213/ANE.0000000000001287
30. Qiu Y, Li H, Yang Z, Liu Q, Wang K, Li R, et al. The prevalence and economic burden of pain on middle-aged and elderly Chinese people: results from the China health and retirement longitudinal study. BMC Health Serv Res. (2020) 20:600. doi: 10.1186/s12913-020-05461-6
31. Rodrigues-De-Souza DP, Palacios-Ceña D, Moro-Gutiérrez L, Camargo PR, Salvini TF, Alburquerque-Sendín F. Socio-cultural factors and experience of chronic low back pain: a Spanish and Brazilian patients' perspective. A qualitative study. PLoS ONE. (2016) 11:1–15. doi: 10.1371/journal.pone.0159554
32. Sá KN, Moreira L, Baptista AF, Yeng LT, Teixeira MJ, Galhardoni R, et al. Prevalence of chronic pain in developing countries: systematic review and meta-analysis. Pain Rep. (2019) 4:e779. doi: 10.1097/PR9.0000000000000779
33. Sharma S, Pathak A, Jha J, Jensen MP. Socioeconomic factors, psychological factors, and function in adults with chronic musculoskeletal pain from rural Nepal. J Pain Res. (2018) 11:2385–96. doi: 10.2147/JPR.S173851
34. Gatchel RJ, Peng YB, Peters ML, Fuchs PN, Turk DC. The biopsychosocial approach to chronic pain: scientific advances and future directions. Psychol Bull. (2007) 133:581-624. doi: 10.1037/0033-2909.133.4.581
35. Darnall B. To treat pain, study people in all their complexity. Nature. (2018) 557:7–7. doi: 10.1038/d41586-018-04994-5
36. Hayes C, Hodson FJ. A whole-person model of care for persistent pain: from conceptual framework to practical application. Pain Med. (2011) 12:1738–49. doi: 10.1111/j.1526-4637.2011.01267.x
37. Salvatore S, Valsiner J. Between the general and the unique. Theory Psychol. (2010) 20:817–33. doi: 10.1177/0959354310381156
38. Varoquaux G, Raamana PR, Engemann DA, Hoyos-Idrobo A, Schwartz Y, Thirion B. Assessing and tuning brain decoders: Cross-validation, caveats, and guidelines. NeuroImage. (2017) 145:166–79. doi: 10.1016/j.neuroimage.2016.10.038
39. Lee JJ, Kim HJ, Ceko M, Park BY, Lee SA, Park H, et al. A neuroimaging biomarker for sustained experimental and clinical pain. Nat Med. (2021) 27:174–82. doi: 10.1038/s41591-020-1142-7
40. Ceko M, Frangos E, Gracely J, Richards E, Wang B, Schweinhardt P, et al. Default mode network changes in fibromyalgia patients are largely dependent on current clinical pain. NeuroImage. (2020) 216:116877. doi: 10.1016/j.neuroimage.2020.116877
41. Hemington KS, Wu Q, Kucyi A, Inman RD, Davis KD. Abnormal cross-network functional connectivity in chronic pain and its association with clinical symptoms. Brain Struct Funct. (2016) 221:4203–19. doi: 10.1007/s00429-015-1161-1
42. Walitt B, Nahin RL, Katz RS, Bergman MJ, Wolfe F. The prevalence and characteristics of fibromyalgia in the 2012 national health interview survey. PLoS ONE. (2015) 10:e0138024. doi: 10.1371/journal.pone.0138024
43. Zelaya C, Dahlhamer J, Lucas J, Connor E. Chronic pain and high-impact chronic pain among adults US 2019. In: NCHS Data Brief. vol. 390. Hyattsville, MD: The United States Centers for Disease Control and Prevention (CDC) National Center for Health Statistics (2020).
44. Frumkin MR, Haroutounian S, Rodebaugh TL. Examining emotional pain among individuals with chronic physical pain: nomothetic and idiographic approaches. J Psychosom Res. (2020) 136:110172. doi: 10.1016/j.jpsychores.2020.110172
45. Liu D, Peng F, Shea A, Rudovic O, Picard R. DeepFaceLIFT: interpretable personalized models for automatic estimation of self-reported pain. ArXiv. (2017) 66:1–16. Available online at: https://arxiv.org/abs/1708.04670
46. Lopez-Martinez D, Picard R. Multi-task neural networks for personalized pain recognition from physiological signals. ArXiv. (2017) 181–4. Available online at: https://arxiv.org/abs/1708.08755
47. Martinez DL, Rudovic O, Picard R. Personalized automatic estimation of self-reported pain intensity from facial expressions. IEEE Comput Soc Confer Comput Vis Pattern Recogn Workshops. (2017) 2017:2318–27. doi: 10.1109/CVPRW.2017.286
48. Gratton C, Laumann TO, Nielsen AN, Greene DJ, Gordon EM, Gilmore AW, et al. Functional brain networks are dominated by stable group and individual factors, not cognitive or daily variation. Neuron. (2018) 98:439–52.e5. doi: 10.1016/j.neuron.2018.03.035
49. Finn ES, Shen X, Scheinost D, Rosenberg MD, Huang J, Chun MM, et al. Functional connectome fingerprinting: Identifying individuals using patterns of brain connectivity. Nat Neurosci. (2015) 18:1664–71. doi: 10.1038/nn.4135
50. Haxby JV, Guntupalli JS, Connolly AC, Halchenko YO, Conroy BR, Gobbini MI, et al. A common, high-dimensional model of the representational space in human ventral temporal cortex. Neuron. (2011) 72:404–16. doi: 10.1016/j.neuron.2011.08.026
51. Lin Q, Li L, Liu J, Liu W, Huang G, Zhang Z. Influence of individual differences in fMRI-based pain prediction models on between-individual prediction performance. Front Neurosci. (2018) 12:1–12. doi: 10.3389/fnins.2018.00569
52. Lindquist MA, Krishnan A, López-Solà M, Jepma M, Woo C-W, Koban L, et al. Group-regularized individual prediction: theory and application to pain. NeuroImage. (2017) 145:274–87. doi: 10.1016/j.neuroimage.2015.10.074
53. Shinkareva SV, Mason RA, Malave VL, Wang W, Mitchell TM, Just MA. Using fMRI brain activation to identify cognitive states associated with perception of tools and dwellings. PLoS ONE. (2008) 3:e1394. doi: 10.1371/journal.pone.0001394
54. Reddan MC, Wager TD. Brain systems at the intersection of chronic pain and self-regulation. Neurosci Lett. (2019) 702:24-33. doi: 10.1016/j.neulet.2018.11.047
55. Chou EY, Parmar BL, Galinsky AD. Economic insecurity increases physical pain. Psychol Sci. (2016) 27:443–54. doi: 10.1177/0956797615625640
56. Dorner TE, Muckenhuber J, Stronegger WJ, Rsky É, Gustorff B, Freidl W. The impact of socio-economic status on pain and the perception of disability due to pain. Eur J Pain. (2011) 15:103–9. doi: 10.1016/j.ejpain.2010.05.013
57. Poleshuck EL, Green CR. Socioeconomic disadvantage and pain. Pain. (2008) 136:235–8. doi: 10.1016/j.pain.2008.04.003
58. Rios R, Zautra AJ. Socioeconomic disparities in pain: the role of economic hardship and daily financial worry. Health Psychol. (2011) 30:58–66. doi: 10.1037/a0022025
59. Taylor R, Rieger A. Medicine as social science: Rudolf Virchow on the typhus epidemic in upper Silesia. Int J Health Serv. (1985) 15:547–59. doi: 10.2190/XX9V-ACD4-KUXD-C0E5
60. Bhugra D. All medicine is social. J Royal Soc Med. (2014) 107:183–6. doi: 10.1177/0141076814525069
61. Goosby BJ. Early life course pathways of adult depression and chronic pain. J Health Soc Behav. (2013) 54:75–91. doi: 10.1177/0022146512475089
62. Vargas CM, MacEk MD, Marcus SE. Sociodemographic correlates of tooth pain among adults: United States, 1989. Pain. (2000) 85:87–92. doi: 10.1016/S0304-3959(99)00250-X
63. Igumbor E, Puoane T, Gansky SA. Prevalence and risk indicators of chronic pain in a rural community in South Africa. J Rural Trop Public Health. (2011) 10:61–9. Available online at: http://hdl.handle.net/10566/430
64. Zarei S, Bigizadeh S, Pourahmadi M, Ghobadifar MA. Chronic pain and its determinants: a population-based study in southern Iran. Korean J Pain. (2012) 25:245–53. doi: 10.3344/kjp.2012.25.4.245
65. Näher AF, Rummel-Kluge C, Hegerl U. Associations of suicide rates with socioeconomic status and social isolation: findings from longitudinal register and census data. Front Psychiatry. (2020) 10:1–9. doi: 10.3389/fpsyt.2019.00898
66. Kiadaliri A, Merlo J, Englund M. Complex sociodemographic inequalities in consultations for low back pain: lessons from multilevel intersectional analysis. Pain. (2021) 162:1135–43. doi: 10.1097/j.pain.0000000000002081
67. Saastamoinen P, Leino-Arjas P, Laaksonen M, Lahelma E. Socio-economic differences in the prevalence of acute, chronic and disabling chronic pain among ageing employees. Pain. (2005) 114:364–71. doi: 10.1016/j.pain.2004.12.033
68. Papageorgiou A, Croft P, Thomas E, Silman A, Macfarlane G. Psychosocial risks for low back pain: Are these related to work? Ann Rheum Dis. (1998) 59:319–20. doi: 10.1136/ard.59.4.319
69. Lee N, Sung H, Kim JH, Punnett L, Kim SS. Perceived discrimination and low back pain among 28,532 workers in South Korea: effect modification by labor union status. Soc Sci Med. (2017) 177:198–204. doi: 10.1016/j.socscimed.2017.01.057
70. LeWinn KZ, Shih EW. Social experience and the developing brain: opportunities for social epidemiologists in the era of population-based neuroimaging. Curr Epidemiol Rep. (2019) 6:466–75. doi: 10.1007/s40471-019-00222-4
71. Edwards RR. The association of perceived discrimination with low back pain. J Behav Med. (2008) 31:379–89. doi: 10.1007/s10865-008-9160-9
72. Baliki MN, Geha PY, Fields HL, Apkarian AV. Predicting value of pain and analgesia: nucleus accumbens response to noxious stimuli changes in the presence of chronic pain. Neuron. (2010) 66:149-60. doi: 10.1016/j.neuron.2010.03.002
73. Vachon-Presseau E, Tétreault P, Petre B, Huang L, Berger SE, Torbey S, et al. Corticolimbic anatomical characteristics predetermine risk for chronic pain. Brain. (2016) 139:1958–70. doi: 10.1093/brain/aww100
74. Borsook D, Upadhyay J, Chudler EH, Becerra L. A key role of the basal ganglia in pain and analgesia - insights gained through human functional imaging. Mol Pain. (2010) 6:27. doi: 10.1186/1744-8069-6-27
75. Kucyi A, Salomons TV, Davis KD. Mind wandering away from pain dynamically engages antinociceptive and default mode brain networks. Proc Natl Acad Sci. (2013) 110:18692–7. doi: 10.1073/pnas.1312902110
76. Kucyi A, Moayedi M, Weissman-Fogel I, Goldberg MB, Freeman BV, Tenenbaum HC, et al. Enhanced medial prefrontal-default mode network functional connectivity in chronic pain and its association with pain rumination. J Neurosci. (2014) 34:3969–75. doi: 10.1523/JNEUROSCI.5055-13.2014
77. Mcewen BS, Gianaros PJ. Central role of the brain in stress and adaptation: links to socioeconomic status, health, and disease. Ann N Y Acad Sci. (2010) 1186:190–222. doi: 10.1111/j.1749-6632.2009.05331.xStress
78. Muscatell KA, Dedovic K, Slavich GM, Jarcho MR, Breen EC, Bower JE, et al. Neural mechanisms linking social status and inflammatory responses to social stress. Soc Cogn Affect Neurosci. (2016) 11:915–22. doi: 10.1093/scan/nsw025
79. Muscatell KA, Morelli SA, Falk EB, Way BM, Pfeifer JH, Galinsky AD, et al. Social status modulates neural activity in the mentalizing network. NeuroImage. (2012) 60:1771–7. doi: 10.1016/j.neuroimage.2012.01.080
80. Ly M, Haynes MR, Barter JW, Weinberger DR, Zink CF. Subjective socioeconomic status predicts human ventral striatal responses to social status information. Curr Biol. (2011) 21:794–7. doi: 10.1016/j.cub.2011.03.050
81. Cascio CN, O'Donnell MB, Simons-Morton BG, Bingham CR, Falk EB. Cultural context moderates neural pathways to social influence. Cult Brain. (2017) 5:50–70. doi: 10.1007/s40167-016-0046-3
82. Gonzalez MZ, Puglia MH, Morris JP, Connelly JJ. Oxytocin receptor genotype and low economic privilege reverses ventral striatum-social anxiety association. Soc Neurosci. (2019) 14:67–79. doi: 10.1080/17470919.2017.1403954
83. Silverman ME, Muennig P, Liu X, Rosen Z, Goldstein MA. The impact of socioeconomic status on the neural substrates associated with pleasure. Open Neuroimaging J. (2009) 3:58–63. doi: 10.2174/1874440000903010058
84. Gao W, Alcauter S, Elton A, Hernandez-Castillo CR, Smith JK, Ramirez J, et al. Functional network development during the first year: Relative sequence and socioeconomic correlations. Cereb Cortex. (2015) 25:2919–28. doi: 10.1093/cercor/bhu088
85. Sripada RK, Swain JE, Evans GW, Welsh RC, Liberzon I. Childhood poverty and stress reactivity are associated with aberrant functional connectivity in default mode network. Neuropsychopharmacology. (2014) 39:2244–51. doi: 10.1038/npp.2014.75
86. Weissman DG, Conger RD, Robins RW, Hastings PD, Guyer AE. Income change alters default mode network connectivity for adolescents in poverty. Dev Cogn Neurosci. (2018) 30:93–9. doi: 10.1016/j.dcn.2018.01.008
87. Federal Register Notice. The Poverty Guidelines Updated Periodically in the Federal Register by the U.S. Department of Health and Human Services Under the Authority of 42 U.S.C. 9902(2). Washington, DC: The Federal Register (2020).
88. Fuentes M, Hart-Johnson T, Green CR. The association among neighborhood socioeconomic status, race and chronic pain in black and white older adults. J Natl Med Assoc. (2007) 99:1160–9.
89. Friedman EM, Karlamangla AS, Almeida DM, Seeman TE. Social strain and cortisol regulation in midlife in the US. Soc Sci Med. (2012) 74:607–15. doi: 10.1016/j.socscimed.2011.11.003
90. Marmot M, Wilkinson RG. Psychosocial and material pathways in the relation between income and health: a response to Lynch et al. Br Med J. (2001) 322:1233–6. doi: 10.1136/bmj.322.7296.1233
91. Archie EA, Altmann J, Alberts SC. Social status predicts wound healing in wild baboons. Proc Natl Acad Sci U.S.A. (2012) 109:9017–22. doi: 10.1073/pnas.1206391109
92. Cohen S, Line S, Manuck SB, Rabin BS, Heise ER, Kaplan JR. Chronic social stress, social status, and susceptibility to upper respiratory infections in nonhuman primates. Psychosom Med. (1997) 59:213–23. doi: 10.1097/00006842-199705000-00001
93. Takahashi A, Flanigan ME, McEwen BS, Russo SJ. Aggression, social stress, and the immune system in humans and animal models. Front Behav Neurosci. (2018) 12:56. doi: 10.3389/fnbeh.2018.00056
94. Kraus MW, Piff PK, Mendoza-Denton R, Rheinschmidt ML, Keltner D. Social class, solipsism, and contextualism: how the rich are different from the poor. Psychol Rev. (2012) 119:546–72. doi: 10.1037/a0028756
95. Jackson T, Wang Y, Wang Y, Fan H. Self-efficacy and chronic pain outcomes: a meta-analytic review. J Pain. (2014) 15:800–14. doi: 10.1016/j.jpain.2014.05.002
96. Morse T, Punnett L, Warren N, Dillon C, Warren A. The relationship of unions to prevalence and claim filing for work-related upper-extremity musculoskeletal disorders. Am J Ind Med. (2003) 44:83–93. doi: 10.1002/ajim.10234
97. Malinowski B, Minkler M, Stock L. Labor unions: a public health institution. Am J Public Health. (2015) 105:261–71. doi: 10.2105/AJPH.2014.302309
98. Adler NE, Stewart J. The MacArthur Scale of Subjective Social Status. (2007). Available online at: http://www.macses.ucsf.edu/research/psychosocial/subjective.php (accessed January 20, 2021).
99. Operario D, Adler NE, Williams DR. Subjective social status: reliability and predictive utility for global health. Psychol Health. (2004) 19:237–46. doi: 10.1080/08870440310001638098
100. Zimet GD, Dahlem NW, Zimet SG, Farley GK. The multidimensional scale of perceived social support. J Pers Assess. (1988) 52:30–41. doi: 10.1207/s15327752jpa5201_2
101. Tuomi K, Wagar K, Eskelinen L. Terveys, tyokyky ja tyoolot kunnallisissa ammattiryhmissa. Tyoterveyslaitoksen Tutkimuksia. (1985) 3:95–132.
102. Jost JT, Thompson EP. Group-based dominance and opposition to equality as independent predictors of self-esteem, ethnocentrism, and social policy attitudes among African Americans and European Americans. J Exp Soc Psychol. (2000) 36:209–32. doi: 10.1006/jesp.1999.1403
103. Lucas T, Zhdanova L, Alexander S. Procedural and distributive justice beliefs for self and others: Assessment of a four-factor individual differences model. J Indvid Dif. (2011) 32:14–25. doi: 10.1027/1614-0001/a000032
104. Dweck CS. Self-Theories: Their Role in Motivation, Personality, and Development. Philadelphia, PA: Psychology Press (1999).
105. Skevington SM. A standardised scale to measure beliefs about controlling pain (B.P.C.Q.): a preliminary study. Psychol Health. (1990) 4:221–32. doi: 10.1080/08870449008400392
106. Rotter JB. Generalized expectancies for internal versus external control of reinforcement. Psychol Monogr. (1966) 80:1. doi: 10.1037/h0092976
107. Costa PT, McCrae RR. Revised NEO Personality Inventory (NEO-PI–R) and the NEO Five-Factor Inventory (NEO-FFI) professional manual. Odessa, FL: Psychological Assessment Resources (1992).
108. Davis MH. Measuring individual differences in empathy: Evidence for a multidimensional approach. J Pers Soc Psychol. (1983) 44:113-26. doi: 10.1037/0022-3514.44.1.113
109. Feeney JA, Noller P, Hanrahan M. Attachment style questionnaire (ASQ). APA PsycTests. (1994). doi: 10.1037/t29439-000
110. Sullivan MJ, Bishop SR, Pivik J. The pain catastrophizing scale: development and validation. Psychol Assess. (1995) 7:524. doi: 10.1037//1040-3590.7.4.524
111. Spielberger CD. State-Trait Anxiety Inventory: Bibliography. 2nd ed. Palo Alto, CA: Consulting Psychologists Press (1989).
112. Beck AT, Steer RA, Brown G. Beck depression inventory–II. APA PsycTests. (1996). doi: 10.1037/t00742-000
113. Koban L, Jepma M, López-Solà M, Wager TD. Different brain networks mediate the effects of social and conditioned expectations on pain. Nat Commun. (2019) 10:1–13. doi: 10.1038/s41467-019-11934-y
114. Scott DJ, Stohler CS, Egnatuk CM, Wang H, Koeppe RA, Zubieta JK. Placebo and nocebo effects are defined by opposite opioid and dopaminergic responses. Arch Gen Psychiatry. (2008) 65:220-31. doi: 10.1001/archgenpsychiatry.2007.34
115. Zunhammer M, Bingel U, Wager TD. Placebo effects on the neurologic pain signature: a meta-analysis of individual participant functional magnetic resonance imaging data. JAMA Neurol. (2018) 75:1321–30. doi: 10.1001/jamaneurol.2018.2017
116. Coan JA, Beckes L, Gonzalez MZ, Maresh EL, Brown CL, Hasselmo K. Relationship status and perceived support in the social regulation of neural responses to threat. Soc Cogn Affect Neurosci. (2017) 12:1574-83. doi: 10.1093/scan/nsx091
117. López-Solà M, Geuter S, Koban L, Coan JA, Wager TD. Brain mechanisms of social touch-induced analgesia. Pain. (2019) 160:2072-85. doi: 10.1097/j.pain.0000000000001599
118. Reddan MC, Young H, Falkner J, López-Solà M, Wager TD. Touch and social support influence interpersonal synchrony and pain. Soc Cogn Affect Neurosci. (2020) 15:1064–75. doi: 10.1093/scan/nsaa048
119. Aytur SA, Ray KL, Meier SK, Campbell J, Gendron B, Waller N, et al. Neural mechanisms of acceptance and commitment therapy for chronic pain: a network-based fMRI approach. Front Hum Neurosci. (2021) 15:587018. doi: 10.3389/fnhum.2021.587018
120. Meier ML, Stämpfli P, Vrana A, Humphreys BK, Seifritz E, Hotz-Boendermaker S. Fear avoidance beliefs in back pain-free subjects are reflected by amygdala-cingulate responses. Front Hum Neurosci. (2015) 9:424. doi: 10.3389/fnhum.2015.00424
121. Shi Y, Huang S, Zhan H, Wang Y, Zeng Y, Cai G, et al. Personality differences of brain networks in placebo analgesia and nocebo hyperalgesia: a psychophysiological interaction (PPI) approach in fMRI. Neural Plast. (2020) 2020:1–13. doi: 10.1155/2020/8820443
122. Mechlin B. Lower socioeconomic status is associated with rating experimental pain as more intense. J Pain. (2012) 13:S52. doi: 10.1016/j.jpain.2012.01.218
123. Davies JB, Shorrocks AF. Comparing Global Inequality of Income and Wealth. WIDER Working Paper 2018/160. Helsinki: UNU-WIDER (2018). doi: 10.35188/UNU-WIDER/2018/602-9
Keywords: chronic pain, neuroimaging biomarkers, translational ability, social epidemiology, social determinants of health, machine learning, biopsychosocial pain models
Citation: Reddan MC (2021) Recommendations for the Development of Socioeconomically-Situated and Clinically-Relevant Neuroimaging Models of Pain. Front. Neurol. 12:700833. doi: 10.3389/fneur.2021.700833
Received: 26 April 2021; Accepted: 06 August 2021;
Published: 07 September 2021.
Edited by:
Flavia Di Pietro, Curtin University, AustraliaReviewed by:
Florian Rimmele, University Hospital Rostock, GermanyCopyright © 2021 Reddan. This is an open-access article distributed under the terms of the Creative Commons Attribution License (CC BY). The use, distribution or reproduction in other forums is permitted, provided the original author(s) and the copyright owner(s) are credited and that the original publication in this journal is cited, in accordance with accepted academic practice. No use, distribution or reproduction is permitted which does not comply with these terms.
*Correspondence: Marianne C. Reddan, bWNyZWRkYW5Ac3RhbmZvcmQuZWR1
Disclaimer: All claims expressed in this article are solely those of the authors and do not necessarily represent those of their affiliated organizations, or those of the publisher, the editors and the reviewers. Any product that may be evaluated in this article or claim that may be made by its manufacturer is not guaranteed or endorsed by the publisher.
Research integrity at Frontiers
Learn more about the work of our research integrity team to safeguard the quality of each article we publish.